Session 5 - Intro to Epidemiology PDF
Document Details
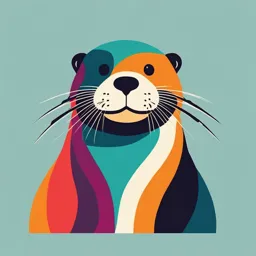
Uploaded by AmenableHurdyGurdy5261
University College London
Maddie Davies-Kellock
Tags
Summary
These lecture notes provide an introduction to epidemiology, focusing on the study of disease distribution and determinants in human populations. The material covers key concepts like study designs, research critiques, and the history of epidemiology. The reading list also suggests PSBS0002: Core Principles of Mental Health Research as a further resource.
Full Transcript
Session5: 11th Oct - pt1 Created @September 24, 2024 2:56 PM Tags Intro to Epidemiology: Lead Teachers: Maddie Davies-Kellock To be covered: Identify the major types of study designs and summaries their strengths and...
Session5: 11th Oct - pt1 Created @September 24, 2024 2:56 PM Tags Intro to Epidemiology: Lead Teachers: Maddie Davies-Kellock To be covered: Identify the major types of study designs and summaries their strengths and weaknesses. Understand how to critique a research study along three key lines of investigation - chance, bias & confounding. Consider whether associations are casual Reading list: PSBS0002: Core Principles of Mental Health Research | University College London (talis.com) Class Prep: NOTES: Preliminary Slides What is epidemiology? “The study of the distribution and determinants of disease frequency in human populations” — MacMahon & Pugh, adapted by Hennekens & Buring 💡 The study of the distributions & determinants of health-relates states or events in specified populations, & the application of this study to the control of health problems. Disease in Human Population Session5: 11th Oct - pt1 1 Frequency: Quantification of existence/occurrence of disease - i.e. incidence/prevalence (Descriptive epidemiology) & rate/risk of disease in a population. Determinants: Systematic scientific identification of risk factors for disease, informed by knowledge of frequency & distribution (Analytical epidemiology) Distribution: Who gets the disease? Comparisons between populations or across subgroups or over time. Essential to describe patterns & formulate hypotheses (Descriptive & analytical epidemiology) What is the frequency of depression in the population? Total people in population = 20 What is the prevalence (proportion) of depression in the population in 2023? 4/20 = 20% How many people at risk of depression in the population in 2024? Population at risk = 20 - 4 = 16 What is the incidence (proportion of new cases) of depression in the population in 2024? Incidence = 2 / 16 = 12.5% Why do we need epidemiology? Alleviate suffering Plan health services Treatment Identify high risk groups Improve health & well-being Identify causes Prevention History of epidemiology and psychiatric epidemiology? What is psychiatric epidemiology? Session5: 11th Oct - pt1 2 “The study of the distribution and determinants of mental health outcomes in human populations” History of epidemiology? Hippocrates (born ~460 B.C.) Father of modern medicine John Snow Cholera outbreak common and deadly in 19th century London. Prevailing theory: Miasma Snow used advances in population enumeration, statistics & disease surveillance to populate a different theory. Frequency Death rates from cholera, 1853-4 Source: Snow (1855). On the Mode of Communication of Cholera (2nd ed) Distribution The first psychiatric epidemiology Emile Durkheim, 1858-1917, French Sociologist. Session5: 11th Oct - pt1 3 Seminal monograph “Suicide” (1897) Hypothesised stronger social control among Catholics vs Protestants led to lower suicide rates. Exposure: predominant country-level social control Attempted to operationalise an outcome: “we must inquire whether, among the different varieties of death, some have common qualities objective enough to be recognized by all honest observers, specific enough not to be found elsewhere and also sufficiently [in] kin to those commonly called suicides” Durkheim, 1897 To do: Preliminary Slides: Intro to Epidemiology Read: Epidemiology for the Unititated - chpt 1-4 & 12 Watch: Intro video to Epidemiology Prep Notes: Read: Psychoses, Ethnicity and Socio-Economic Status Read: Airs, Waters, Places Read: The Environment and Disease: Association or Causation? Notes: Outcomes: Be familiar with the history of epidemiology and psychiatric epidemiology Understand the core principles of epidemiological research and differences between key measures of disease frequency. Understand how to critique a research study along three key lines of investigation – chance, bias (selection & measurement), confounding and reverse causality How to discuss whether associations are causal Session5: 11th Oct - pt1 4 Core Principles of Epidemiological Research Uses of Epidemiology Historical study - to investigate prevalence of disease, influence healthcare policy Population studies to establish prevalence of disease Calculating individual risks For Health Services Research To help clinical understanding and the identification and labelling of disease To suggest possible causes Investigating Causes of Illness Suspicion about a possible risk factor for disease → Where do they come from: clinical observation, lab studies, disease surveillance, case report, theoretical speculation 1. Comparison groups 2. Measure the exposure and 3. Measure outcome (e.g. via surveys, observational) 4. Analyse the association statistically Investigating cause example: Hypothesis: Physical activity reduces the likelihood of depression Outcome: depression (via diagnosis/validated measure of symptoms) Exposure (risk factor): physical activity (via self-report, data from technology) Study Design: cross sectional survey Session5: 11th Oct - pt1 5 Risk ratio example - those expose divided by total Risk ratio = 2.35 = more than twice the risk of outcome Risk = 0.3 = 30% of people the exercise 5x a week have depression Risk ratio = 0.6 = if you exercise 5x a week, you are 0.6 less likely to be depressed Odds ratio example - used when total not available Odds ratio = 3.56 = odds of outcome are 3.5 times higher in those with exposure than without exposure Interpretation below one = less at risk / lower odds Interpretation above one = more at risk / higher odds Epidemiological Reasoning Session5: 11th Oct - pt1 6 Chance, Bias (Selection or Measurement), Confounding, Reverse Causality Making Inferences from Associations 💡 Association does not mean causation. When deciding if a relationship is causal, these issues must be considered: Chance (statistics) Bias (selection bias, measurement bias) Confounding Reverse causality Must then weigh up likelihood that association found is actually causal i.e. causal inference. We want to answer questions about mental health in the population. We wish to “infer” values in the population, using the values from our sample which represents the population. The estimate from the sample is not necessarily the same as the (true) value from the population. Quantify uncertainty - confidence intervals & p-values Why does chance matter? Can’t test everyone Conversely, to save us studying everyone, we can use adequately sized samples to make statistically robust inferences about the population. Chance Session5: 11th Oct - pt1 7 Need to consider if the association results by chance, as a result of random error. Statistical inference (p values and confidence intervals) help make a judgement about whether sampling variation can explain the results. Type 1 error – a “statistically significant” result occurs by chance Type 2 error – a real result is not “statistically significant” because of chance - E.g. false negative (Note: More on this in the “sample size calculation lecture”) Confidence intervals – also give you information about likely values and whether your study has excluded an important result. Since we must sample, we are faced with uncertainty. We need to quantify this uncertainty using statistics (confidence intervals and p values) Lower CI = 0.4 Lower CI = 0.5 Upper CI = 0.9 Upper CI = 0.7 Wide confidence interval - due to small Same risk ratio. sample size The CI is narrower — can be more certain Null = 1 that the estimate is close to the true value in the population. Session5: 11th Oct - pt1 8 More on 95% Confidence Interval The CI and p value are both derived from the size of the difference between groups and its standard error so they are closely related The standard error decreases as sample size increases So the width of the CI and size of p are as dependent on sample size as they are on the size of the difference The larger the sample, the narrower the CI and the smaller the p-value A → statistically significant and clinically meaningful C → True value in the population is likely to be clinically meaningful but less certainty due to the wider CI (overlaps with A, suggesting it’s capturing the same variation) D → not statistically significant (wide CI) and suggests the true value in the population could include harm (E.g. exercise causing depression) E → statistically significant but not clinically meaningful F → suggests no association but lots of uncertainty due to the wide CI - could be clinically meaningful or cause harm. Bias Introducing systematic error during the design or conduct of a study If there is bias, your estimate of association is not the true value as observed in the population you are studying Session5: 11th Oct - pt1 9 Bias can reduce and increase the size of association It is difficult or impossible to quantify bias – but important to can identify likely sources and discuss possible impact 1. Selection Bias Arises from the procedures used to select individuals into the study or analysis. It is about the people in your study and not in your study The sample of participants selected differs on characteristics than the target population with respect to factors that are associated both with the exposure and the outcome Examples: Low response rate in a survey Participants in a clinical trial drop out of follow up Case-control studies are particularly sensitive to selection bias (discussed in pt2.) 2. Measurement Bias Results from the measurement of exposure, outcome or other information obtained on participants. Measurement bias is sometimes called “information bias” Observer bias: error introduced by observer expectations e.g. an observer rates someone as less active because of existing views about motivation and depression - may guide the intertidal towards a particular answer Recall bias: if the illness (outcome) affects memory for past events e.g. people with depression are more likely to remember traumatic events that have occurred in the past than an individual without depression Social desirability bias: respond in a way that makes the individual appear ‘better’ or more likely to give the ‘right’ or ‘assumed’ answer e.g. say they exercise more than you do / or say you drink less than you do Confounding Alternative explanation of the association between an exposure and outcome. Session5: 11th Oct - pt1 10 A confounder is a “third” variable that is associated with the exposure and outcome and results in a change in the observed association. Confounding can lead to spurious associations or eliminate real ones. Confounder must be associated with the exposure and outcome. Methods for Dealing with Confounding You have to collect data on potential confounders when you carry out a study. Confounder = socio- Need to think about them at the design stage. economic status The main methods for taking account of confounding are: Exposure: physical 1. Randomisation – the best way if you can but often not activity → Outcome: possible depression 2. Adjusting using multivariable methods – after adjustment Example of potential for a confounder the association between exposure and confounder: outcome will change Better physical health Residual confounding – you can almost never take account of Access to green space confounding perfectly and there are unknown confounders, in local area so there is always the possibility of confounding even after adjustment Confounding or Bias Confounding still occurs even if the study is perfectly designed (which is impossible). Confounding is an alternative explanation for the association that is present in the study population. Bias is introduced by the investigator (not intentionally) as a result of carrying out the study. Reverse Causality The hypothesis is that the exposure causes the outcome. E.g. physical activity reduces the likelihood of depression. Session5: 11th Oct - pt1 11 However, it is possible sometimes that the outcome causes the exposure. The main way of addressing this possibility: Carry out a longitudinal study Where you measure the exposure in people without the outcome and then follow them up to see who becomes ill. Dealing with Chance, Bias & Confounding We have now learnt about 3 major threats to validity: Chance – Addressed at the design stage only Ensure the sample is of sufficient size to detect the effect you hope to observe Bias –Only addressed at the design phase, By designing representative or random samples, and minimising issues of selection and information bias Confounding – At the design and analysis phase of the study. At the design stage via restriction or matching At the analysis stage by adjusting the statistical model for confounders. You cannot adjust statistical models for biases. Causal Inference Session5: 11th Oct - pt1 12 💡 “Is there any other way of explaining the set of facts before us, is there any other answer equally, or more, likely than cause and effect?” Bradford-Hill What is a cause? In public health, a cause is something that if taken away would reduce the incidence of a disease. There are multiple causes for almost all common diseases – a “multifactorial aetiology”. And also for most psychiatric disorders. Causes are almost always neither necessary or sufficient. Models of Causation “Neither necessary nor sufficient” Disease can occur without the cause People without the cause can get the disease Bradford-Hill Considerations on Causality - How to judge if something is causal Temporality – exposure precedes outcome Strength – stronger associations are more likely to be causal. Usually taken to be (more than) > Relative Risk of 1.5 to 2. Dose-response – as the level of exposure increases so does the risk of the outcome Consistency – different studies in different populations find the association Specificity – exposure produces a specific effect Coherence – triangulation across different sources of evidence Lots of different studies/types of evidence points to the same thing Plausibility – aligns with known biological or other processes Explanation of the process for how the exposure leads to the outcome Analogy – that similar exposures lead to the outcome Session5: 11th Oct - pt1 13 Experimental evidence – animal work or experimental medicine studies support the hypothesis Summary Epidemiology is the science of population health. Five non-causal explanations for an association: Chance Selection Bias Measurement Bias Confounding Reverse causality Proving a causal association in observational data is challenging (perhaps impossible) but there are “guidelines” we can use to discuss the possibility of causation. Session5: 11th Oct - pt1 14