Introduction to Epidemiology 1 PDF
Document Details
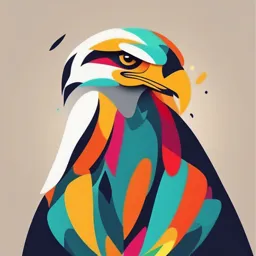
Uploaded by WorldFamousZombie1045
UCL
Tags
Summary
This document provides an introduction to epidemiology, focusing on core principles and different research methodologies used in epidemiology, potentially in mental health. It also covers issues like chance, bias, confounding, and reverse causality.
Full Transcript
**[Core Principles of Mental Health Research]** **[Introduction to Epidemiology 1]** [Learning Outcomes:] - Be familiar with the history of epidemiology and psychiatry epidemiology - Understand the core principles of epidemiology research and differences between key measures of disea...
**[Core Principles of Mental Health Research]** **[Introduction to Epidemiology 1]** [Learning Outcomes:] - Be familiar with the history of epidemiology and psychiatry epidemiology - Understand the core principles of epidemiology research and differences between key measures of disease frequency - Understand how to critique a research study along three key lines of investigation -- chance, bias (selection & measurement), confounding and reverse causality - How to discuss whether associations are casual [Investigating causes:] - Hypothesis: Physical activity reduces the likelihood of depression - Outcome: Depression - Exposure: Physical activity - Study Design: Cross Sectional survey Come from: - Lab studies - Disease surveillance - Case reports - Theoretical speculation - Clinical observation Outcome happens after exposure [Epidemiological Reasoning:] Study Design: - Identify the question + - Systematic collection of data + - Systematic analysis of data + Interpretation - Assess threats to validity - - Casual interference -- [Making Inferences from associations:] - Association does not mean causation - When deciding if a relationship is casual, these issues must be considered: - Chance (statistics) - Bias (selection bias, measurement bias) - Confounding - Reverse causality (cause opposite to what we assume -- e.g., depression causes less exercise) - Must then weigh up likelihood that association found is actually causal (i.e., causal inference) [Making inferences from epidemiological observations:] - Estimated from the sample are not necessarily the same as the (true) value from the population Why does chance matter? - Can't test everyone - Since we must sample, we are faced with uncertainty -- we need to quantify this uncertainty using statistics (confidence intervals & probability) - Conversely, to save us studying everyone, we can use adequately sized samples to make statistically robust inferences about the population [Chance:] - Need to consider if the association results by chance, as a result of random error - Statistical inference (p-values and confidence intervals) help make a judgement about whether sampling variation can explain the result - Type 1 error: "statistically significant" result occurs by chance (p-value) accept null when its false - Type 2 error: real result not "statistically significant" because of chance (1-P) reject null when its true - Confidence intervals -- give you information about likely values and whether your study has excluded an important result [95% Confidence Intervals:] - The CI and p-value are both derived from the size of the difference between groups and its standard error, so they are closely related - The standard error decreases as sample size increases so the width of the CI and size of p are as dependent on sample size as they are on the size of the difference - The larger the sample, the narrower the CI and the smaller the p-value (more precise) - in confidence intervals mid-point is odds ratio value - If confidence interval ranges between 0.3 -- 1.5 (aka crosses 1) means we have failed to reject the null hypothesis (results found by chance) [Bias: ] - Systematic error introduced during the design or conduct of a study - If there is bias your estimate of association is not the true value as observed in the population, you are studying - Bias can reduce and increase the size of association - It is difficult or impossible to quantify bias -- but you can identify likely sources and discuss possible impact [Selection Bias:] - Bias that arises from the procedures used to select individuals into the study or analysis - It is about the people in your study (or not in your study) - The sample of participants selected differs on characteristics than the target population with respect to factors that are associated both with the exposure and the outcome [Examples:] - Low response rate in a survey - Participants in a clinical trial drop out of follow up - Case-control studies are particularly sensitive to selection bias [Measurement Bias:] - Bias that results from the measurement of exposure, outcome or other information obtained on participants -- measurement bias is sometimes called "information bias" [Many types including:] - Observer bias: error introduced by observer expectations - Recall bias: if the illness (outcome) affects memory for past events [Confounding:] - Alternative explanation of the association between an exposure and outcome - A confounder is a "third" variable that is associated with the exposure and outcome and results in a change in the observed association - Confounding can lead to spurious associations or eliminate real ones [Methods for dealing with confounding:] - You have to collect data on potential confounders when you carry out a study so need to think about them at the design stage [Main methods for taking account of confounding are:] 1. Randomisation: the best way if you can but often not possible 2. Adjusting using multivariable methods: after adjustment for a confounder the association between exposure and outcome will change Residual Confounding: you can almost never take account of confounding perfectly and there are unknown confounders, so there is always the possibility of confounding even after adjustment [Confounding or Bias?] - Confounding still occurs even if the study is perfectly designed (which is impossible) - Confounding is an alternative explanation for the association that is present in the study population - Bias is introduced by the investigator (not intentionally) as a result of carrying out the study [Reverse Causality:] - Main way of addressing this is to carry out a longitudinal study where you measure the exposure the exposure in people without the outcome and then follow them up to see who becomes ill [Dealing with chance, bias & confounding] - Chance - Sample Size - Confidence Intervals - Bias: - Sampling Strategy - Measures Used - Design of study - Retrospective vs Prospective - Confounding: - Restriction (Design) - Matching (Design) - Adjustment (Analysis) [Models of Causation:] - "Neither necessary nor sufficient": disease can occur without the cause - E.g., elderly woman who had smoked thousands of cigarettes in their life being fine [Bradford-Hill Considerations on causality:] - Temporality: exposure precedes outcome - Strength: stronger associations are more likely to be casual -- usually taken to be \> Relative Risk of 1.5 to 2 - Dose-Response: as the level of exposure increases so does the risk of the outcome - Consistency: different studies in different populations find the association - Specificity: exposure produces a specific effect - Coherence: triangulation across different sources of evidence - Plausibility: aligns with known biological or other processes - Analogy: similar exposures lead to the outcome - Experimental evidence: animal work or experimental medicine studies support the hypothesis [Study Designs:] - Observational vs Experimental (choice of study design will depend on whether the investigator assigned the exposure [Descriptive Epidemiology: Cross-sectional studies] - Conducted on individuals - Exposure & outcome status ascertained at a "cross-sectional" point in time - Often survey based i.e., questionnaire - Chiefly concerned with assessing frequency of disease occurrence - i.e., prevalence - and distribution i.e., by sex, age, race, social class - less useful for determinants of health [Strengths & Limitations] [Descriptive Epidemiology: Ecological Studies] - Conducted on populations - Disease frequencies compared between different groups in same period - Or, in the same population at different times - Often use routine data - Can reveal patterns at the population level - As before, limited in terms of determinants [Strengths & Limitations] [Analytical Epidemiology: Case-Control Studies ] - Conducted at the individual level - Starting point is case status - Identification of people with the disease outcome i.e., cases - A sample of controls (without the disease) are recruited & compared with respect to exposure of interest [Strengths & Limitations] [Analytical Epidemiology: Cohort Studies] - A population is identified without the disease outcome - Risk factors measured at points during follow up - Followed over time to see who develops disease [Strengths & Limitations] [Intervention Studies: Randomised Controlled Trials ] - A form of prospective cohort study - People identified based on exposure status & followed to see who experiences outcome - What is the major strength of a randomised trial? - Random allocation to exposure will, on average, ensure balance of other factors which could affect disease risk [Summary:] - No study design is optimal, all have strengths & weaknesses - Is some circumstances only certain designs will be possible? - In terms of casual inference: - RCT Very Strong - Cohort Study Moderate/Quite Strong - Case-Control Moderate - Cross-Sectional Weak - Ecological Very Weak