Social Epidemiology of Psychosis and Bipolar PDF
Document Details
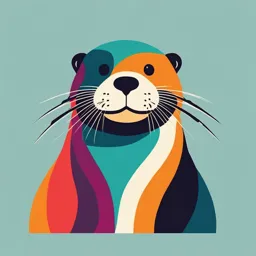
Uploaded by AmenableHurdyGurdy5261
University College London, University of London
Tags
Summary
This document explores the social epidemiology of psychosis and bipolar disorder. It examines historical contexts, key findings, and discusses the role of immigration and ethnicity in the development of these conditions. The document also touches on environmental impacts on mental health, using studies and research.
Full Transcript
**Key Preparatory Lecture** **Social Epidemiology** *"the branch of epidemiology that studies the social distribution and social determinants of health" Berkman & Kawachi* Incidence based studies can be used to inform us about the frequency of diseases across subgroupings of the population. Coho...
**Key Preparatory Lecture** **Social Epidemiology** *"the branch of epidemiology that studies the social distribution and social determinants of health" Berkman & Kawachi* Incidence based studies can be used to inform us about the frequency of diseases across subgroupings of the population. Cohort studies and cross-sectional studies have been used to measure the incidence of psychotic disorder in different groups. Predominantly by place and according to migrant/ethnic status. - Combining frequency, distribution and determinants allows to study diseases from a population level rather than an individual level. **Historical Context** *The psychiatric epidemiologist's Christopher Columbus →* **Ørnulv** **Ødegård** - Set up a study in 1932 - Raised rates of "insanity" in Norwegians in Minnesota cf Norway - Ødegård argued for selection effects - One of the first to compare the rates of a disorder with a comparison control group (Norwegians and immigrants to US) - Found higher rates of "insanity" (psychotic disorder) among immigrants from Norway to US - Argued it was due to selection patterns due to certain pressures on the migrants that not only forced them to migrate but made it more likely to develop psychosis **Major Findings** Immigration & Ethnicity → Mass migration following advent of industrial revolution → Mass population movements to cities *(Guanzhou)* - Published in 1939 - Analysis of 30,000 first admissions for psychosis in Chicago in 1920s - Pre-admission census tract address obtained - Incidence rates estimated for each tract Neighbourhood Risk - Highest rates of schizophrenia in inner city tracts - These tracts tended to be more socially disorganized, socially isolated - No comparable association with bipolar disorder - Also observed elsewhere: Mannheim (Germany), Ireland, London, Bristol, Nottingham - **Pre-lecture background reading: Lewis et al. 2020** Association between Urban Environment, IQ, and Nonaffective Psychosis Study Overview - Prospective cohort study of Swedish men born 1982-1988 - Sample: 227,429 men conscripted at age 18 - Follow-up: Until December 31, 2016 Key Findings - 1,596 men (0.7%) diagnosed with nonaffective psychosis - Increased odds of psychosis per 1-SD increase in: - Population density: OR 1.07 (95% CI 1.04-1.14) - Deprivation: OR 1.09 (95% CI 1.02-1.13) - IQ negatively associated with deprivation (-0.70 points per 1-SD increase) - 23% of deprivation\'s effect on psychosis mediated by IQ - No effect modification by IQ on associations between urban factors and psychosis Implications - Being born in deprived areas may increase psychosis risk partly through effects on cognitive development - Supports neurodevelopmental model of psychotic disorders - Identifying specific factors in deprived environments could inform prevention strategies Methodology - Exposures: Small area-level population density and socioeconomic deprivation at birth - Outcome: First diagnosis of nonaffective psychosis (ICD-10) from age 18 - IQ assessed at conscription (age 18) - Mediation analysis using potential outcomes framework This study provides evidence for the role of early-life urban environments and cognitive development in psychosis risk, highlighting potential pathways for intervention. **Notes: How our environments affect mental health** **Recap & Discussion** **Exposure ---------------------------\> Outcome** Migration Schizophrenia Cities **Threats to causality: *Migration leading to/causality of Schizophrenia*** **Chance** **Bias** **Confounding** **Reverse Causation** ----------------------------------------------------------------------------------------------------------------------------------------------------------- ------------------------------------------------------------------------------------------------------------------------------------------------------------ ------------------------------------------------------------------------------------------------------------------------------------------ ------------------------------------------------------------------------------------ How robust are the findings? i.e. sample size - if the study is too small, won't be able to detect an effect. Or it will detect an effect that isn't true Who is at risk? Selection bias. Variation by ethnic groups / migrant status Migrants more likely to be younger (healthy migrant effects) and men (more likely to be economic migrants and more at risk of psychosis) Are people who migrate (more likely to be) predisposed to psychosis? Validity of diagnostic criteria - ?based on biomedical model (developed in the US) is it valid for other populations? Migration reasons vary Are people who live in urban areas (more likely to be) predisposed to psychosis? Higher rates in country of origin - is it due to the migration factor or do people from their country of origin have a higher rate of psychosis regardless Migrants more likely to be from lower social classes(?)/urban living Genetic confounding Case finding procedure Substance abuse - more likely to have increased availability in urban areas "Do people with schizophrenia migrate more?" - does the outcome drive the exposure Estimation of denominator More likely to experience major life events - known risk for Schz Generalisability Childhood traumas Assess confounding factors to sample and control group and adjust statistical measure. Could **restrict** analysis to eliminate confounding variable - though may reduce generalisability Could **match** number of people in sample to number in control group with confounding variable For some migrant groups, they have an elevated risk of psychosis - not all. **Migration, Ethnicity & Psychotic Disorder** **Where is the risk increased?** *Hogerzeil et al. 2016 -* Turkish migrants to Netherlands *Weiser et al. 2008; Zolkowska et al. 2001, Cantor-Graae et al. 2003, Tarricone et al. 2012; Dykxhoorn et al. 2018* Red: show increased risk in psychosis from migrant populations Green: show a lower risk in psychosis from migrant populations (more green space than urbanised Europe & Canada and Australia founded on migration histories - so most people from these places are migrants --- more diverse mix which may allow people to migrate and incorporate themselves in these countries). **How big are the effect sizes?** Meta-analysis: Grand mean = around 3 though massive variation across research (variation might be explained by different migrant groups being investigated) - Mean RR for schizophrenia & immigrant status: 2.9 (95% CI: 2.5-3.4) (Cantor-Graae & Selten, 2005) - RR\~5 in black African & Caribbean migrant groups (Kirkbride et al 2012, Tortelli et al 2015) - In the UK context & Europe - more at risk than White British group - RR\~ 2-4 for Asian women (UK) & North African groups - About 2-4x more at risk - Men seem to be at a similar risk - RR\~1.5-2 white migrants - Still slightly more at risk - so not completely ethnically influenced **What factors account for variation in risk by ethnicity & migrant status?** **Control strategies:** - Regression adjustment - Matching - Restriction If there is still an effect after controlling cofounder - we have stronger evidence that the outcome is not caused by the confounder. Looking into the causal structure: "we believe there is a causal relationship between X & Y but recognise C could influence this pathway" ***Example 1. Simple Causal Mechanism*** *Also shown that these excesses tend to persist for BME groups living in both urban and rural parts of the region.* Confounding effect of income - increased risk of psychosis goes down. Suggesting the initial risk are not necessarily associated to country but more so the age, sex, time period and income. Though still higher in African populations. ***Example 2. Complex Causal Mechanism*** *Conditioning on mediator(s) could still lead to valid estimates of the causal direct effect as long as there are no unmeasured confounders of X\>Y, X\>M and M\>Y.* *Therefore in this example, conditioning on a range of potential mediators and confounders may allow us to understand if there is a direct causal effect of ethnicity on psychotic disorder. Presumably, there would not be, if we could correctly identify all confounders and mediators.* X ---\> M suggests it's a mediating factor - not confounding Indirect = outcome is influenced by mediating factor We can adjust for M (though not encouraged - this biases the total causal effect) As the pathway X→M→Y is part of the total cause *Umbrella term for several of these mediators might be what Michael Marmot has called psychosocial disempowerment as a hypothesis for the mechanism linking social adversity to poor health outcomes.* The Mediator to Outcome relationship may of course be confounded by several factors as well, which we would need to condition on, including: Examples of M→Y confounders: Sex/gender Childhood trauma Cannabis use Parental history of psychosis Paternal age Relationship status Living arrangements If we control for M with the knowledge of C - if we have confounders that we don't measure but exist, we don't block the path. We make it an alternative explanation for Y, making a collider bias. To account for this, we must condition/block for C too so we can investigate the direct effect of X→Y. - Does the social experience following migration affect psychosis risk? - Minority position may increase likelihood of psychosocial disempowerment *(Marmot, 2004, 2006)* - Psychosocial disempowerment involves loss or denial of agency over one's life/environment - May arise when more socially & culturally distanced from majority population - **Hypothesis**: Elevated psychosis risk in Black, Asian & Minority Ethnic groups would be attributable to greater sociocultural distance *(Jongsma et al, 2021)* - Data from EU-GEI case-control study - 1130 FEP cases & 1497 controls from 17 centres - EU funded study: Brasil, UK, Netherlands, France, Spain, Italy - Social distance: education, parental SES, social isolation - Cultural & linguistic distance: measured via language trees & fluency - To measure socio-cultural distance to the general population - E.g. similarities in language and culture (i.e. Italy & Spain) - Disempowerment: discrimination - Logistic regression, multiple imputation Further work suggests it is cultural distance, not discrimination which predicts the raised rates in migrant groups While discrimination might represent acute, perceived experiences, social and cultural distance may reflect more chronic, structural effects of discrimination deeply embedded in society Case/control Ns shown on slide Regression adjustment: Not much difference in risk between Model 1 and adjustment for Social distance. Suggests all those factors combined are explanations for raised rate of psychosis for people in ethnic minority groups Model 4 = not much of an effect of discrimination with linguistic distance. **Urbanicity & Psychotic Disorder** **What is the evidence for increased risk?** The AESOP study made several important contributions to understanding the epidemiology of psychotic disorders: 1. The study used population-based case finding, moving beyond hospital-based settings to identify cases in the community. 2. It was conducted across three centres in the UK - South London, Nottinghamshire, and Bristol - allowing for geographical comparisons. 3. Over a two-year period, 568 cases of first-episode psychosis (FEP) were identified using consensus-based diagnoses. 4. The study examined geographical variation in incidence rates after adjusting for key factors like age, sex, and ethnicity. 5. The results showed more variation in incidence rates for non-affective psychoses compared to affective psychoses across the different locations. 6. This approach addressed limitations of previous studies by accounting for important variables like ethnicity and individual socioeconomic status, which had often been overlooked. 7. The AESOP study aimed to clarify whether differences in psychosis risk remained across geographical areas even after accounting for these demographic and socioeconomic factors. This methodological approach represented an advancement in psychiatric epidemiology by providing a more comprehensive and community-based assessment of psychosis incidence across diverse UK settings. *Bipolar doesn't show the same relationship with the urban environments.* - Place of residence at first contact for psychosis recorded - Models estimate incidence of non-affective psychotic disorders in different neighbourhoods, adjusted for age, sex, ethnicity - Darker colours show neighbourhoods with statistically significantly higher rates than the study centre average; lighter = lower risk - Non-affective psychosis risk associated with neighbourhood social capital - No variation in affective psychoses consistent with other studies - Repeated in more rural area - All contacts with Early Intervention Psychosis \[EIP\] services recorded over 3.5 years, 2009-12 - 687 FEP cases identified, 16-35 yrs (ICD-10 F10-33) - Neighbourhood at first contact recorded - Combined with detailed Census data on 530 neighbourhoods - Multilevel Poisson regression - Adjusted for age, sex, ethnicity & SES & other neighbourhood factors - How do such factors operate in rural communities? - When people in rural areas are exposed to factors like deprivation, do we also see increased rates of disorder? - Urbanicity: less confident of risk *After additional conditioning on C2 the direct association between urbanicity & NAPD becomes weaker in Richardson et al (2018)* In a DAG, there only appears to be an association between urbanicity & non-affective psychoses, but no effect for the affective psychoses Conditioning for age, sex, ethnicity & SES does not appear to remove the direct effect of X\>Y Further conditioning (SEPEA) for deprivation, ethnic diversity and social isolation may explain that effect But there is a bigger problem at hand here, to do with the direction of the effect ***Can you think of a bigger issue with the way the previous studies were conducted that limits our understanding of whether urbanicity causes non-affective psychotic disorder?*** *Hint: when was urbanicity measured & why is this important?* - Instead of exposure to urban environments causing psychosis, could psychosis be causing (leading to) exposure to urban environments? - This is called reverse causation - May happen if pathology of disease affects "exposure" - Here, psychosis could lead to job loss, lower income & therefore move to more urban (deprived) neighbourhoods *How could we remove / minimise reverse causality from our study? - longitudinal study* Formally reverse causation is a type of unmeasured confounding, here were subclinical disease status, U, influences urbanicity after onset and risk of clinical disorder. If we measure urbanicity at some time **before** the onset of symptoms, we can still estimate the total causal effect of urbanicity on non-affective disorders, unconfounded by subclinical psychotic symptoms. ***Example 1. Simple Causal Mechanism*** *Note, we might not agree all these are confounders of the relationship in this study, i.e. sex is unlikely to influence urban birthplace, while age at birth is obviously zero, though in this study they adjusted for age as what we call a time-varying covariate. Still, age and sex are unlikely to confound here, though are predictors of* - Cohort study of 1.75m people in Denmark - Followed from birth until diagnosis of schizophrenia (N=2,669) - Birthplace categorised in 5 groups from rural to capital city - Poisson regression - Adjusted for age, sex, parental age, family history of schizophrenia & season of birth Shows link between where you're born and risk of psychosis - higher risk in being born in the Capital. ***Example 2. Complex Causal Mechanism*** *What factors might be represented by 'U'?* This may deal with reverse causation of the individual, but still leaves open the possibility that intergenerational reverse causation may explain urbanicity at birth associations with NAPD - Higher polygenric risk score for schizophrenia (PRS) predicts living in more densely populated areas in adulthood - 4 data sets from UK, Netherlands and Australia *(Colodro-Conde et al, 2018)* - Similar results at age 15 in Denmark, but not at birth *(Paksarian et al, 2018)* - We investigated PRS, neighbourhood at birth & psychotic experiences in ALPSAC birth cohort (N=11,879) - *(Solmi et al. 2020)* **Conclusion** - Incidence of psychotic disorders vary according to urban exposure & migrant/ethnic status - Risk of all psychotic disorders elevated for many ethnic minority & migrant groups - Only non-affective psychoses appear to vary by urban exposure - Ethnicity, migrant status & urban living are only proxies for other exposures - Epidemiology (and DAGs) can be useful for testing causal role of environmental factors on health outcomes (including psychosis) - We used DAGs to think about two epidemiological concepts -- confounding & reverse causation - For both urbanicity and ethnicity/migration, new research suggest psychosocial disempowerment may be relevant (sociocultural distance, social capital), but stronger causal evidence needed to address roles of: - Disempowerment, sociocultural distance & discrimination - Genetic confounding