ITE353 Data Scalability and Analytics PDF
Document Details
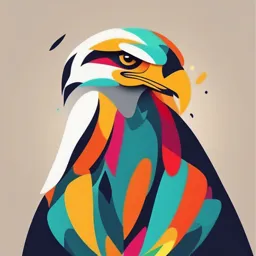
Uploaded by SustainableMagenta438
PHINMA EDUCATION
Tags
Summary
This document is a student activity sheet for an ITE353 course on Data Scalability and Analytics. It introduces correlation and regression analysis techniques for data analysis and provides examples.
Full Transcript
ITE353: Data Scalability and Analytics Student Activity Sheet Module #7 Name: _________________________________________________________ Class n...
ITE353: Data Scalability and Analytics Student Activity Sheet Module #7 Name: _________________________________________________________ Class number: _______ Section: ____________ Schedule: _____________________________________ Date: _______________ Lesson Title: Performing Data Analysis Materials: Lesson Objectives: Learning Modules, Online sites for At the end of the session, you will be able to: statistical calculations 1. Define statistical, correlation, and regression analysis. 2. Differentiate correlation and regression analysis References: 3. Perform data analysis using correlation and regression https://www.statology.org/ A. LESSON PREVIEW/REVIEW Introduction Good day, everyone! Were you able to pass your first-period quiz? I hope you are still into our data analysis topics. For today’s session, you will explore how to identify patterns and relationships in data, as well as how to draw conclusions based on your findings. Specifically, we will focus on the concepts of correlation and regression, which are powerful tools for understanding the relationships between variables and making predictions about the future. By the end of this course, you will be equipped with the skills and knowledge needed to conduct data analysis on your own and draw meaningful insights from the results. Let's get started! B. MAIN LESSON Content and Skill-Building Understanding the Goal of Data Analysis In the previous discussions, you learned that data analysis is defined as a process of cleaning, transforming, and modeling data to discover useful information for business decision-making. The goal of data analysis is to gain insights and knowledge from data in order to make informed decisions, solve problems, and improve outcomes. By using statistical and computational methods to analyze data, we can identify patterns, relationships, and trends that may not be immediately apparent through simple observation. 1 This document is the property of PHINMA EDUCATION ITE353: Data Scalability and Analytics Student Activity Sheet Module #7 Name: _________________________________________________________ Class number: _______ Section: ____________ Schedule: _____________________________________ Date: _______________ A simple example of data analysis is that whenever we take any decision in our day-to-day lives, we think about what happened last time or what will happen if we make that particular decision. This is nothing but analyzing our past or future and making decisions based on it. For that, we gather memories of our past or dreams of our future. Statistical Analysis In the previous topics, we mentioned that there are two analytical methods we can utilize in collecting data: quantitative and qualitative research methods. One of the fundamental components of quantitative research methods is statistical analysis, which involves investigating trends, patterns, and relationships using quantitative data. Statistical analysis is based on the principles and methods of statistics. Correlation and Regression for Data Patterns Correlation and regression analysis are statistical techniques used to identify patterns and relationships in data. By analyzing the relationship between two or more variables, we can gain insights into how they are related and make predictions about their future behavior. Correlation analysis is used to measure the strength and direction of the relationship between two or more variables. It examines how much two variables are related to each other, and whether their relationship is positive, negative, or non-existent. A positive correlation occurs when the E.g., is a positive correlation between height values of two variables increase or and weight, because taller people tend to weigh decrease together. more. 2 This document is the property of PHINMA EDUCATION ITE353: Data Scalability and Analytics Student Activity Sheet Module #7 Name: _________________________________________________________ Class number: _______ Section: ____________ Schedule: _____________________________________ Date: _______________ A negative correlation occurs when the E.g., is a negative correlation between studying values of two variables move in opposite and watching TV, because the more time directions. someone spends watching TV, the less time they have for studying. A zero correlation occurs when there is no relationship between the values of two variables. Regression analysis is used to model the relationship between two or more variables. There are two main types of regression: simple linear regression and multiple regression. Simple linear regression is used when Multiple regression is used when there there is a single independent variable are two or more independent variables that is used to predict a single dependent that are used to predict a single variable. dependent variable. Correlation vs. Regression 3 This document is the property of PHINMA EDUCATION ITE353: Data Scalability and Analytics Student Activity Sheet Module #7 Name: _________________________________________________________ Class number: _______ Section: ____________ Schedule: _____________________________________ Date: _______________ Performing Data Analysis Using Correlation Performing a statistical analysis using correlation involves several steps, which are as follows: For example, suppose we have the following dataset that contains two variables: (1) Hours studied and (2) Exam Scores received for 20 different students: Research question: Is there a relationship between the number of hours spent studying and exam scores? Data: A sample of 20 students, where the number of hours spent studying and the corresponding exam scores are recorded for each student on the left side. Calculation: If you are investigating the relationship between two continuous variables, you can use correlation analysis to measure the strength and direction of the relationship. Pearson's correlation coefficient is commonly used for this purpose. Use statistical software or a calculator to calculate Pearson's correlation coefficient. This is a value that ranges from -1 to 1, where -1 indicates a perfect negative correlation, 0 indicates no correlation, and 1 indicates a perfect positive correlation. You may utilize this online site to calculate this value and just follow the on-screen instructions. http://www.socscistatistics.com/tests/pearson/ By entering the sample values at the left, and using the online calculator, we can find that the correlation between these two variables is r = 0.915. 4 This document is the property of PHINMA EDUCATION ITE353: Data Scalability and Analytics Student Activity Sheet Module #7 Name: _________________________________________________________ Class number: _______ Section: ____________ Schedule: _____________________________________ Date: _______________ Interpretation: Since this value is close to 1, it confirms that there is a strong positive correlation between the two variables. This is a strong positive correlation, which means that high X variable scores go with high Y variable scores (and vice versa). Performing Data Analysis using Regression For conducting regression analysis, we implement the following steps: Using the example given in the correlation analysis which is finding the relationship between the number of hours spent studying and exam scores, we continue the analysis steps: Regression model: Since we are examining the relationship between two variables, hours of study and exam scores, a simple linear regression model would be appropriate. This model assumes that there is a linear relationship between the two variables. Calculation: Assuming that the regression model is a simple linear regression model, the regression equation would be: Exam Score = b0 + b1*(Hours of Study) where: Exam Score is the dependent variable (the variable we are trying to predict) Hours of Study is the independent variable (the variable we are using to predict the dependent variable) b0 is the intercept term, which is the predicted value of the dependent variable when the independent variable is zero. b1 is the slope coefficient, which indicates the rate of change in the dependent variable for each unit increase in the independent variable 5 This document is the property of PHINMA EDUCATION ITE353: Data Scalability and Analytics Student Activity Sheet Module #7 Name: _________________________________________________________ Class number: _______ Section: ____________ Schedule: _____________________________________ Date: _______________ Using a linear regression calculator (https://www.graphpad.com/quickcalcs/linear1/), that you can access online, we find that the following equation best describes the relationship between these two variables: Predicted exam score = 65.47 + 2.58*(hours studied) The way to interpret this equation is as follows: The predicted exam score for a student who studies zero hours is 65.47. The average increase in exam scores associated with one additional hour studied is 2.58. We can also use the equation to predict the score that a student will receive based on the number of hours studied. For example, a student who studies 6 hours is expected to receive a score of 80.95: Predicted exam score = 65.47 + 2.58*(6) = 80.95. Interpretation: We can also plot this equation as a line on a scatterplot. The linear regression calculator also generated the graph. 6 This document is the property of PHINMA EDUCATION ITE353: Data Scalability and Analytics Student Activity Sheet Module #7 Name: _________________________________________________________ Class number: _______ Section: ____________ Schedule: _____________________________________ Date: _______________ We can see that the regression line “fits” the data quite well. Recall earlier that the correlation between these two variables was r = 0.915. It turns out that we can square this value and get a number called “r-squared” which describes the total proportion of variance in the response variable that can be explained by the predictor variable. In this example, r2 = 0.9152 = 0.837. This means that 83.7% of the variation in exam scores can be explained by the number of hours studied. Skill-Building Activity (20PTS) It's time to put your data-analysis skills to use. Sit in groups of two, and perform correlation and regression analyses. One of you will conduct the correlation analysis, while the other will conduct the regression analysis. You’ll base your calculations on the information provided. Below are the height and weight data sets of individuals: Research question: Is there a relationship between height and weight in a sample of individuals? Calculate the correlation coefficient, perform a simple linear regression analysis, and interpret your findings. 7 This document is the property of PHINMA EDUCATION ITE353: Data Scalability and Analytics Student Activity Sheet Module #7 Name: _________________________________________________________ Class number: _______ Section: ____________ Schedule: _____________________________________ Date: _______________ Check for Understanding (5PTS) Based on the content notes, provide what is being asked in each number. 1. TRUE/FALSE. Regression analysis can only be used to analyze linear relationships between variables. ______________________________________________________________________ 2. Which of the following is the correct form of the regression analysis equation for a simple linear regression model? Encircle the letter of the correct answer. A. y = a + bx B. y = a - bx C. y = b + ax D. y = b - ax 3. Based on the correct answer in item no. 2, what does y represent? Encircle the letter of the correct answer. A. Intercept B. Independent variable C. Slope D. Dependent variable 4. What is a positive relationship in correlation analysis? Encircle the letter of the correct answer. A. When two variables have an inverse relationship and increase or decrease in opposite directions B. When there is no relationship between the two variables C. When two variables have a direct relationship and increase or decrease together D. When two variables are completely unrelated and have no effect on each other 5. What are statistics? ______________________________________________________________________ ______________________________________________________________________ C. LESSON WRAP-UP Summary / Frequently Asked Questions 1. What are predictor and response variables? A predictor variable (also known as an independent variable) is a variable that is used to predict or explain the variation in another variable. In statistical analysis, predictor 8 This document is the property of PHINMA EDUCATION ITE353: Data Scalability and Analytics Student Activity Sheet Module #7 Name: _________________________________________________________ Class number: _______ Section: ____________ Schedule: _____________________________________ Date: _______________ variables are usually denoted by "X" and are used to predict the response variable (or dependent variable), denoted by "Y". In the example we’ve used for today’s lesson on determining the relationship between students' hours of study and their exam scores, the predictor variable is the number of hours studied and the response variable is the exam score. 2. Can I use both correlation and regression in my data analysis? Yes, it is common to use both correlation and regression analysis in data analysis. Correlation analysis is often used as a first step to explore the relationship between two variables, while regression analysis is used to develop a more detailed model of the relationship and to make predictions about one variable based on the other variable(s). Thinking About Learning Congratulations! You are done! Shade the number of the module that you have finished today. Fill out the table below and write about your learning experiences for today’s session. Learning Scores Action Plan Date Target/Topic What module# did you do? What were What contributed to the quality of your What’s What were the learning performance today? What will you do the date your scores in targets? What next session to maintain your today? the activities? activities did you performance or improve it? do? 9 This document is the property of PHINMA EDUCATION