Lecture -8- MSc-1 PDF
Document Details
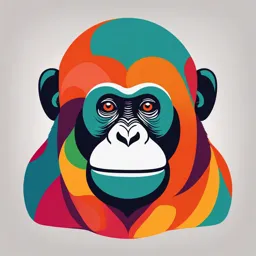
Uploaded by AlluringActionPainting
Al-Ahliyya Amman University
Dr. Walhan ALSHAER
Tags
Summary
This lecture covers research methods and biostatistics, focusing on statistical analysis techniques. It explores descriptive and inferential statistics, confidence intervals, hypothesis testing, and parametric and non-parametric tests. The lecture also touches on correlation and regression analyses, explaining how they can be applied in various fields like medical research, business analytics, and environmental science.
Full Transcript
Research Methods and Biostatistics طرق البحث العلمي واإلحصاء الحيوي For Undergraduate students Dr. Walhan ALSHAER Director of Pharmacological and Diagnostic Research Center Al-Ahliyya Amman University (AAU...
Research Methods and Biostatistics طرق البحث العلمي واإلحصاء الحيوي For Undergraduate students Dr. Walhan ALSHAER Director of Pharmacological and Diagnostic Research Center Al-Ahliyya Amman University (AAU) And Senior Research Scientist Cell Therapy Center The University of Jordan 1 Lecture: Introduction to Biostatistics Basic Statistical Concepts A. Descriptive Statistics: Measures of central tendency and variability. B. Inferential statistics: Population vs. sample, hypothesis testing. 2 Descriptive Statistics vs. Inferential Statistics Descriptive: Summarizing data (e.g., mean, median, mode). Inferential: Drawing conclusions or making predictions about a population based on sample data. Importance of Inferential Statistics Helps make evidence-based decisions. Essential in research, clinical trials, quality control, and more. 3 Key Concepts in Inferential Statistics Population vs. Sample Population: The entire group of interest in a study. Sample: A smaller group selected from the population. Example: Population: All diabetic patients in a city. Sample: 150 diabetic patients chosen randomly. 4 Inferential Statistical Methods 1. Estimation and Confidence Intervals Point Estimate: Single value used to estimate a population parameter (e.g., sample mean). Confidence Interval (CI): A range of values, derived from the sample, that likely contains the population parameter. Formula for Confidence Interval for the Mean: Example: If the sample mean of exam scores is 70 with a standard deviation of 5 (n=100), a 95% CI is: 70±1.96⋅5/√100=[69.02,70.98] 5 Inferential Statistical Methods 2. Hypothesis Testing Definition: A method to test claims or hypotheses about population parameters. Steps of Hypothesis Testing: 1. State the null (H₀) and alternative hypotheses (H₁). 2. Set the significance level (α = 0.05). 3. Select the test statistic: 4. Compute the test statistic and p-value. 5. Compare the p-value with α: If p < α, reject H₀. 6. Interpret the result. Example: Testing if a new drug reduces blood pressure. H₀: The drug has no effect (µ = µ₀). H₁: The drug reduces blood pressure (µ < µ₀). 6 Applications of Inferential Statistic 1. Medical Research: Example: Clinical trials to test a new vaccine. 2. Business Analytics: Example: Estimating customer satisfaction from survey data. 3. Environmental Science: Example: Checking pollution levels in a city using sample air quality data. 4. Quality Control in Manufacturing: Example: Testing if a factory produces defect-free products. 7 Advantages and Limitations of Inferential Statistics Advantages: Allows decision-making with incomplete data. Provides estimates and confidence about population characteristics. Limitations: Accuracy depends on sampling methods. Sampling errors and biases can mislead results. 8 Discussion How would you interpret a p-value of 0.03? How would you interpret a p-value of 0.06? 9 Statistical Analysis Techniques Parametric vs. Non-Parametric Tests T-tests, ANOVA, and regression analysis Chi-square tests and non-parametric alternatives Correlation and Regression Pearson vs. Spearman correlation Simple and multiple regression analysis 10 Parametric vs. Non-Parametric Tests Definition: Methods to summarize, explore, and analyze data. Types of Statistical Techniques: Parametric Tests: Assume underlying data follows a specific distribution (usually normal). Non-Parametric Tests: No assumptions about data distribution. When to Use Each? Parametric tests are more powerful if assumptions are met. Non-parametric tests are suitable for small samples, skewed data, or ordinal variables. 11 Parametric vs Non-Parametric Tests 1. Parametric Tests a) T-Tests Purpose: Compare means between two groups. Types: Independent t-test: Comparing means of two independent samples. Paired t-test: Comparing means within the same group (before vs. after). Example: Comparing blood pressure before and after administering a drug. 12 Parametric vs Non-Parametric Tests b) ANOVA (Analysis of Variance) Purpose: Compare means across three or more groups. Types: One-Way ANOVA: One independent variable with multiple groups. Two-Way ANOVA: Two independent variables to assess interaction effects. Example: Comparing exam scores among students from three teaching methods. 13 Parametric vs Non-Parametric Tests c) Regression Analysis Purpose: Examine relationships between variables and predict outcomes. Types: Simple Regression: One independent variable predicts one dependent variable. Multiple Regression: Two or more independent variables predict one dependent variable. Example: Predicting weight (Y) based on height (X) and diet score (X2). 14 Parametric vs Non-Parametric Tests 2. Non-Parametric Tests a) Chi-Square Tests Purpose: Test the association between two categorical variables. Types: Chi-square goodness of fit: Compares observed vs expected frequencies. Chi-square test for independence: Tests the association between two categorical variables. Example: Testing if smoking status (yes/no) is associated with gender. 15 Parametric vs Non-Parametric Tests b) Non-Parametric Alternatives When parametric test assumptions are violated: Mann-Whitney U test: Alternative to the independent t-test. Wilcoxon Signed-Rank test: Alternative to the paired t-test. Kruskal-Wallis test: Alternative to ANOVA. Spearman correlation: Non-parametric alternative to Pearson correlation. Example: Comparing median exam scores between two groups when data is skewed. 16 Correlation and Regression Analysis 1. Correlation Purpose: Measure the strength and direction of the relationship between two variables. a) Pearson Correlation Coefficient (r) Used for: Continuous data (interval/ratio) that meets normality assumptions. Range: -1 to +1. Positive r: Positive relationship. Negative r: Negative relationship. r = 0: No correlation. 17 Correlation and Regression Analysis b) Spearman Rank Correlation Used for: Ordinal data or when assumptions of normality are violated. Based on ranks of data rather than raw values. Example: Pearson: Correlation between height and weight. Spearman: Correlation between rank in a class and hours of study. 18 Correlation and Regression Analysis 2. Regression Analysis a) Simple Linear Regression Purpose: Model the relationship between one independent variable (X) and one dependent variable (Y). Equation: Y=β0+β1X+ϵ β₀: Intercept β₁: Slope ε: Error term Example: Predicting salary based on years of experience. 19 Correlation and Regression Analysis b) Multiple Regression Purpose: Model the relationship between multiple independent variables (X1, X2, …) and one dependent variable (Y). Equation: Y=β0+β1X1+β2X2+...+ϵ Example: Predicting disease risk based on age, BMI, and exercise level. Interpretation of Coefficients: Each β shows the effect of one predictor while holding others constant. Significance: Based on p-values and confidence intervals. 20 Practical Applications of Statistical Techniques Medical Research: Using t-tests and regression for clinical outcomes. Social Sciences: Chi-square tests for survey analysis. Business Analytics: Multiple regression for sales predictions. Environmental Studies: Correlation analysis for pollutant levels and health outcomes. 21 Discussion Summary Parametric tests are powerful but require assumptions (e.g., normality). Non-parametric tests are flexible for non-normal or ordinal data. Correlation quantifies relationships between variables. Regression analysis predicts outcomes and determines variable impacts. Q&A and Discussion What assumptions must be met for a t-test? When would you use Spearman instead of Pearson correlation? 22 Next lecture: Interpreting and Presenting Data The following topics will be delivered as practical and training lectures. Statistical Software Applications Introduction to software Data entry and management Data Visualization Creating graphs and charts Effective presentation of statistical findings Interpreting Results Understanding p-values and confidence intervals Making inferences from data 23