Summary of Babbie Book SSR (PDF)
Document Details
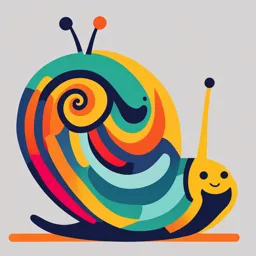
Uploaded by LuxuriousOmaha
University of Twente
Tags
Summary
This document provides a summary of a book on social research methods. It covers fundamental concepts, paradigms, research design, and data analysis techniques. The document is likely meant to be a study guide or outline.
Full Transcript
**Summary Babbie Book SSR and Summary of the units materials:** **Key Features and Structure:** 1. **Foundations of Inquiry:** - Explains human inquiry, science, and the nature of social research. - Discusses paradigms, theory, and ethics in research. 2. **Structuring Rese...
**Summary Babbie Book SSR and Summary of the units materials:** **Key Features and Structure:** 1. **Foundations of Inquiry:** - Explains human inquiry, science, and the nature of social research. - Discusses paradigms, theory, and ethics in research. 2. **Structuring Research:** - Focuses on conceptualization, measurement, research design, and sampling. - Addresses quantitative and qualitative methodologies. 3. **Modes of Observation:** - Covers experiments, surveys, qualitative field research, and unobtrusive methods. - Includes evaluation research and how to analyze existing statistics. 4. **Data Analysis:** - Explores qualitative and quantitative data analysis. - Introduces multivariate analysis and statistical methods. 5. **Applications:** - Guidance on reading, writing, and proposing social research. - Appendices provide additional resources like using libraries and random numbers. **Highlights:** - Engages readers with real-world examples and data, offering insights into practical applications. - Includes boxed features such as \"Issues and Insights,\" \"How to Do It,\" and \"Applying Concepts to Everyday Life\" for enhanced learning. - Updated statistical data and examples reflecting contemporary research challenges. **Chapter 1: Human Inquiry and Science** - **Overview:** Introduces the fundamentals of social research and its distinction from everyday human inquiry. Emphasizes avoiding errors like overgeneralization, selective observation, and illogical reasoning. - **Key Concepts:** - **Ordinary Human Inquiry vs. Scientific Inquiry:** Science employs systematic methods to understand patterns and predict social phenomena. - **Foundations of Social Science:** Built on theory (explaining phenomena), social regularities, and studying aggregates (groups) rather than individuals. - **Ethics in Inquiry:** Ethical principles are central to conducting human research. **Chapter 2: Paradigms, Theory, and Research** - **Overview:** Explores the theoretical underpinnings of social research and the importance of paradigms and theories. - **Key Concepts:** - **Social Science Paradigms:** Includes positivism, conflict theory, symbolic interactionism, feminist theory, and critical race theory. - **Theory Construction:** Deductive (starting from a theory to test hypotheses) vs. inductive (building theory from observations). - **The Role of Paradigms:** Paradigms shape how researchers view and approach social phenomena. **Chapter 4: Research Design** - **Overview:** Focuses on planning and structuring research to address specific questions or hypotheses. - **Key Concepts:** - **Research Purposes:** Exploration, description, and explanation. - **Units of Analysis:** Includes individuals, groups, organizations, and social artifacts. - **Time Dimensions:** Cross-sectional (single point in time) vs. longitudinal (over time). - **Causal Relationships:** Discusses criteria for causality---association, time order, and nonspuriousness. **Chapter 5: Conceptualization, Operationalization, and Measurement** - **Overview:** Covers the steps of defining and measuring concepts in research. - **Key Concepts:** - **Conceptualization:** Defining concepts and their dimensions. - **Operationalization:** Translating concepts into measurable variables. - **Measurement Quality:** Emphasizes reliability (consistency) and validity (accuracy). **Chapter 6: Indexes, Scales, and Typologies** - **Overview:** Explores tools for combining and measuring variables. - **Key Concepts:** - **Indexes and Scales:** An index summarizes multiple indicators, while a scale considers intensity patterns. - **Scale Types:** Includes Likert scales, Guttman scaling, and semantic differentials. - **Validation:** Ensuring reliability and validity in constructed indexes and scales. **Chapter 7: The Logic of Sampling** - **Overview:** Discusses techniques for selecting representative samples. - **Key Concepts:** - **Sampling Types:** Probability sampling (e.g., random sampling) vs. nonprobability sampling (e.g., convenience sampling). - **Sampling Frames:** Defines the population of interest and ensures representativeness. - **Sampling Errors:** Explains biases and errors in sampling methods. **Chapter 8: Experiments** - **Overview:** Introduces the experimental method in social research. - **Key Concepts:** - **Components:** Includes independent/dependent variables, control groups, pretesting, and posttesting. - **Experimental Designs:** Classical experiments, quasi-experiments, and natural experiments. - **Strengths and Weaknesses:** Experiments are strong in internal validity but may lack generalizability. **Chapter 11: Unobtrusive Research** - **Overview:** Examines methods that do not involve direct interaction with subjects. - **Key Concepts:** - **Content Analysis:** Analyzing texts, media, and artifacts. - **Analyzing Existing Statistics:** Utilizing pre-existing datasets for secondary analysis. - **Comparative and Historical Research:** Comparing data across time and contexts. - **Strengths:** Reduces researcher influence on subjects; ethical concerns are minimal. **Chapter 14: Quantitative Data Analysis** - **Overview:** Focuses on analyzing numerical data. - **Key Concepts:** - **Quantification:** Organizing data into categories and codebooks. - **Descriptive Statistics:** Summarizing data through measures of central tendency (mean, median) and dispersion (range, standard deviation). - **Bivariate Analysis:** Exploring relationships between two variables. - **Data Cleaning:** Ensuring accuracy in data entry and analysis. **Chapter 15: The Logic of Multivariate Analysis** - **Overview:** Introduces methods for analyzing relationships between multiple variables. - **Key Concepts:** - **Elaboration Model:** Explains relationships while controlling for additional variables. - **Replication, Explanation, Interpretation:** Techniques to refine findings. - **Multivariate Techniques:** Includes regression analysis and factor analysis. Unit 1: Empirical Research **Empirical Research and the Wheel of Science** **Empirical research** involves the systematic collection and analysis of data to answer questions about real-world phenomena. It relies on observation, experimentation, and evidence rather than theory or intuition. The **Wheel of Science** (also called the empirical cycle) is a framework that outlines the iterative process of scientific research. The steps in the wheel of science are: 1. **Observation**: Identifying phenomena or trends that warrant investigation. 2. **Induction**: Formulating a hypothesis based on observations. 3. **Deduction**: Deriving predictions or testable statements from the hypothesis. 4. **Testing**: Collecting data through experiments or observations to test the predictions. 5. **Evaluation**: Analyzing results and refining or rejecting the hypothesis, leading to further observations. **Induction vs. Deduction** - **Induction**: - Moves from specific observations to broader generalizations. - Example: Observing that many students perform better when studying in groups, and concluding that group study improves academic performance. - **Deduction**: - Moves from general theories to specific predictions. - Example: Starting with a theory that group study improves academic performance, then predicting that a specific group of students will score higher if they study together. **Steps in the Decision-Making Process** 1. **Problem & Need Analysis**: - Identify and clearly define the problem. - Analyze needs and prioritize them to guide decisions. 2. **Design and Decision Making**: - Develop possible solutions or interventions. - Evaluate alternatives systematically (e.g., through ex ante evaluation). 3. **Implementation**: - Execute the chosen solution or intervention. - Monitor the process (process evaluation). 4. **Evaluation**: - Assess outcomes and impacts (ex post evaluation or effect research). - Determine if the decision solved the problem effectively. **Systematic Decision Making and Systematically Answering Empirical Questions** Systematic decision-making involves structured steps to ensure informed and evidence-based decisions. Similarly, systematically answering empirical questions involves a structured process to ensure valid and reliable findings. Both require: - Clear objectives or research questions. - Well-designed data collection and analysis plans. - Rigorous testing and evaluation of hypotheses or decisions. The alignment between these processes ensures that decisions are grounded in empirical evidence. **Formulating Research Questions in Decision-Making Contexts** Research questions should be relevant to the phase of the decision-making process. Examples: - **Problem & Need Analysis**: \"What are the primary causes of declining employee satisfaction?\" - **Ex Ante Evaluation**: \"What is the expected impact of implementing a flexible work schedule on productivity?\" - **Process Evaluation**: \"Are the resources allocated for the training program being used effectively?\" - **Ex Post Evaluation**: \"What has been the impact of the new marketing strategy on sales over the past year?\" **Examples of Confirmation Bias** Confirmation bias occurs when individuals seek or interpret evidence to support their pre-existing beliefs. Examples: 1. A manager who believes a new policy is effective only notices instances where the policy works well and ignores failures. 2. A researcher selectively reports data that align with their hypothesis while disregarding contradictory data. 3. A doctor focuses on symptoms that confirm their initial diagnosis and dismisses other possibilities. **How Systematic Empirical Research Helps Avoid Confirmation Bias** 1. **Structured Data Collection**: Ensures that evidence is collected systematically, reducing selective attention. 2. **Objective Analysis Methods**: Statistical tests and standardized methods help ensure findings are not swayed by personal biases. 3. **Peer Review**: Independent evaluation of research by others challenges subjective interpretations. 4. **Transparent Reporting**: Publishing all results, including negative findings, prevents selective reporting. Systematic empirical research promotes rigor and objectivity, making it less likely for confirmation bias to influence conclusions. Unit 2: Clear research questions **1. Identifying Units, Variables, and Settings in Empirical Research Questions** - **Units**: These are the entities being studied or observed. Units can be individuals, groups, organizations, countries, etc. - **Variables**: Characteristics of the units that vary and are of interest in the study. Variables have attributes (categories) or values (numerical levels). - **Settings**: The context or environment in which the research takes place (e.g., geographic location, time period, population). **Example:** Descriptive Research Question:\ *\"What is the average income of individuals in urban areas?\"* - **Unit**: Individuals. - **Variable**: Income (measured in currency, e.g., dollars). - Attributes/Values: Specific income levels (e.g., \$40,000, \$50,000). - **Setting**: Urban areas. Explanatory Research Question:\ *\"How does education level influence income in urban areas?\"* - **Unit**: Individuals. - **Independent Variable**: Education level (e.g., high school, bachelor's, master's). - Attributes: Different levels of education. - **Dependent Variable**: Income (measured in currency). - **Setting**: Urban areas. **2. Distinguishing Empirical, Normative, and Conceptual Questions** - **Empirical Questions**:\ These are questions that can be answered using observations, experiments, or evidence.\ Example: *"What percentage of voters support the new policy?"* - **Normative Questions**:\ These involve value judgments and address what \"should\" or \"ought\" to be. They cannot be answered solely with empirical evidence.\ Example: *"Should the government increase the minimum wage?"* - **Conceptual Questions**:\ These focus on clarifying the meaning of terms or concepts.\ Example: *"What is the definition of democracy?"* **3. Differentiating Explanatory and Descriptive Empirical Questions** - **Descriptive Questions**:\ Aim to describe characteristics or trends without establishing causal relationships.\ Example: *"What is the literacy rate in rural areas?"* - Goal: Provide information or summarize a phenomenon. - **Explanatory Questions**:\ Aim to explain why or how phenomena occur, focusing on causal relationships.\ Example: *"Why is the literacy rate lower in rural areas compared to urban areas?"* - Goal: Identify underlying causes or mechanisms. **Key Terms in Context** - **Unit (of Analysis)**: The entity being studied (e.g., individuals, cities, countries). - **Variable**: A measurable characteristic that varies across units (e.g., income, education level). - **Attributes/Values**: Categories or numerical levels of a variable. - **Setting**: The context or environment of the study (e.g., time, location, population). - **Research Question**: A focused question guiding the study (e.g., descriptive or explanatory). - **Empirical**: Based on observed or experimental evidence. - **Normative**: Based on values or judgments about what \"ought\" to be. - **Conceptual**: Related to the meaning and definitions of ideas or terms. - **Descriptive**: Focused on describing characteristics or trends. - **Explanatory**: Focused on identifying causes and mechanisms behind phenomena. Unit 3: What are data?: **1. Recognizing Units of Analysis and Units of Observation in a Study** - **Units of Analysis**: The primary entities being studied or analyzed. These are the \"what\" or \"who\" you are trying to draw conclusions about (e.g., individuals, households, organizations, countries). - **Units of Observation**: The entities from which data is collected. Sometimes these overlap with units of analysis, but not always. **Example**:\ In a study about the effect of school-level policies on student performance: - **Units of Analysis**: Schools (policies are being studied at the school level). - **Units of Observation**: Individual students (data collected through their test scores). **2. How Mixing Up Units of Analysis and Units of Observation May Lead to the Ecological Fallacy** The **ecological fallacy** occurs when conclusions about individuals are drawn from data aggregated at a group level. This happens when researchers mistake the unit of analysis for the unit of observation. **Example**: - A study shows that countries with higher average incomes tend to have higher literacy rates. - Assuming that an individual with a higher income in one of these countries is also more literate is an ecological fallacy because the relationship at the group level does not necessarily apply to individuals. **3. Identifying Variables with Their Attributes/Values in a Study** - **Variables**: Characteristics being measured or observed. - **Attributes/Values**: Specific categories or numerical measures associated with a variable. **Example**: - **Variable**: Gender. - **Attributes**: Male, Female, Nonbinary. - **Variable**: Income. - **Values**: \$20,000, \$30,000, \$40,000, etc. **4. Determining if Attributes/Values Are Exhaustive and Mutually Exclusive** - **Exhaustive**: All possible categories or values are included. - Example: For the variable \"marital status,\" categories like \"married,\" \"single,\" \"divorced,\" and \"widowed\" must cover all potential statuses. - **Mutually Exclusive**: No overlap exists between categories or values. - Example: If "marital status" has overlapping categories like \"married\" and \"cohabiting,\" they are not mutually exclusive because someone could fall into both. **5. Differentiating Between Levels of Measurement** 1. **Nominal**: Categorical data with no inherent order. - Example: Eye color (blue, brown, green). 2. **Ordinal**: Categorical data with a meaningful order but no consistent intervals. - Example: Education level (high school, bachelor's, master's). 3. **Interval**: Numerical data with consistent intervals but no true zero point. - Example: Temperature in Celsius (0°C does not indicate "no temperature"). 4. **Ratio**: Numerical data with consistent intervals and a true zero point. - Example: Income (zero income means the absence of income). **Special Case**: - **Dichotomy (Dummy Variable)**: A variable with only two attributes/values, often coded as 0 and 1. - Example: Employed (1 = yes, 0 = no). **6. Explaining What a Data Matrix Looks Like** A **data matrix** is a table where: - **Rows** represent individual cases (units of observation). - **Columns** represent variables. - **Cells** contain the value of a variable for a specific case. **Example of a Data Matrix**: **Case ID** **Gender** **Age** **Income** **Employed** ------------- ------------ --------- ------------ -------------- 1 Male 25 40000 1 2 Female 30 50000 1 3 Male 22 30000 0 **7. Codebook** A **codebook** is a document that explains the structure and coding of a dataset. It includes: - **Variable names** and labels. - **Descriptions** of what each variable measures. - **Values and their meanings** (e.g., 1 = Yes, 0 = No for a dichotomy). - **Measurement levels** (nominal, ordinal, interval, ratio). Unit 5: Conceptualizing constructs **1. Distinguishing Constructs from Observables** - **Constructs**: Abstract ideas or phenomena that cannot be directly observed or measured (e.g., intelligence, satisfaction, democracy). - **Observables**: Measurable and concrete phenomena or indicators used to infer constructs (e.g., IQ test scores for intelligence, survey responses for satisfaction). **Example**: - Construct: Academic performance. - Observables: Test scores, grades, class attendance. **2. Identifying Constructs in Research Questions and Theories** Constructs can appear in research as: 1. **Variables**: Characteristics or attributes that vary across units. - Example: \"What is the relationship between *motivation* and *performance*?\" - Motivation and performance are constructs treated as variables. 2. **Sets of Variables**: When constructs are multifaceted or multidimensional. - Example: The construct \"job satisfaction\" could include variables like work-life balance, pay, and coworker relationships. 3. **Units of Analysis**: Constructs can also represent the \"who\" or \"what\" being studied. - Example: In a study about \"democratic governance,\" the construct of \"democracy\" could represent the unit of analysis (countries). **3. Conceptualization, Operationalization, and Measurement** - **Conceptualization**: Defining what a construct means in a theoretical context. - Example: Defining \"stress\" as the perception of pressure and inability to cope with demands. - **Operationalization**: Turning a construct into measurable variables by specifying how it will be observed. - Example: Measuring \"stress\" through self-reported survey items or cortisol levels. - **Measurement**: Collecting data based on the operational definition. - Example: Administering the survey or analyzing cortisol samples. **4. Conceptualizing a Construct Using Relationships Between Traits** Constructs often consist of **dimensions, facets, or traits**, which are specific aspects of the construct. **Example**: The construct \"intelligence\" includes dimensions like: - Logical reasoning. - Verbal ability. - Spatial awareness. **Relationships Between Traits**: - Traits may be additive (summed together for a composite score). - Traits may represent different but interrelated facets of the same construct. **5. How Composite Measures Are Used to Measure a Single Construct** A **composite measure** combines multiple indicators to represent a single construct. This is useful when constructs are complex or multidimensional. **Example**:\ To measure \"social capital,\" you might combine: 1. Trust in institutions. 2. Participation in community activities. 3. Number of social connections. **6. Distinguishing Composite Measures as Typology, Index, or Scale** 1. **Typology**: Classifies cases into categories based on combinations of attributes or dimensions. - Example: Classifying organizations as \"small, medium, or large\" based on size and industry. 2. **Index**: Aggregates multiple variables into a single score, often with equal or weighted contributions. - Example: Human Development Index (HDI), which combines life expectancy, education, and income. 3. **Scale**: Measures the intensity or degree of a construct, typically based on responses to items that reflect underlying traits. - Example: Likert scale measuring attitudes toward climate change (e.g., 1 = Strongly Disagree, 5 = Strongly Agree). **Key Terms Explained** - **Construct/Concept/Term**: Abstract idea or phenomenon of interest. - **Dimension/Facet/Trait**: Specific aspects or components of a construct. - **Conceptualization**: Defining the construct theoretically. - **Operationalization**: Developing a method to observe or measure the construct. - **Measurement**: Collecting data for the construct. - **Types**: Refers to different categories of composite measures (typology, index, scale). - **Typology**: Categorizes cases based on attributes. - **Index**: Aggregates multiple indicators into one score. - **Scale**: Measures the degree or intensity of a construct. UNIT 6: **1. The Relationship Between \"Conceptualization,\" \"Operationalization,\" and \"Measurement\"** - **Conceptualization**:\ Defining what a construct means in theoretical terms. It clarifies the idea or concept being studied. - Example: \"Health\" could be conceptualized as physical, mental, and social well-being. - **Operationalization**:\ Translating the conceptual definition into specific, measurable variables or indicators. - Example: Measuring physical health using variables like BMI, exercise frequency, and heart rate. - **Measurement**:\ Collecting data based on the operationalized variables, often using specific tools or instruments. - Example: Conducting surveys or using medical devices to collect BMI and heart rate data. **Relationship**: - Conceptualization provides the theoretical definition. - Operationalization specifies how the concept will be observed. - Measurement is the process of collecting data based on the operational definition. **2. Recognizing Various Data Collection Methods** Common methods of data collection include: 1. **Surveys/Questionnaires**: Collecting structured data from respondents. - Example: Likert scales to measure satisfaction. 2. **Interviews**: Open-ended or semi-structured discussions to gather in-depth data. - Example: Asking participants about their career motivations. 3. **Observation**: Systematic recording of behaviors or events. - Example: Watching customer interactions in a store. 4. **Experiments**: Manipulating variables to observe causal effects. - Example: Testing the impact of a new teaching method on student performance. 5. **Document Analysis**: Reviewing existing texts, records, or media. - Example: Analyzing government reports for economic trends. **3. Telling If a Data Collection Method Is (Un)obtrusive and (Non)verbal** - **Obtrusive**: The researcher's presence or data collection process is noticeable and may influence participants\' behavior. - Example: Face-to-face interviews. - **Unobtrusive**: The researcher does not directly interact with participants or influence their behavior. - Example: Analyzing archival records or observing through a hidden camera. - **Verbal**: Relies on spoken or written language. - Example: Interviews, surveys, open-ended questions. - **Nonverbal**: Focuses on behaviors, physiological measures, or visual cues. - Example: Observing body language or using biometric data. **4. Differentiating Primary Data From Secondary Data** - **Primary Data**: Data collected directly by the researcher for the specific study. - Example: Conducting a survey on consumer preferences. - **When to Use**: When specific, current, and targeted data is required. - **Secondary Data**: Data originally collected by someone else for a different purpose. - Example: Using census data to study demographic trends. - **When to Use**: When existing data is sufficient, time or resources are limited, or historical comparisons are needed. **5. Operationalizing a Construct** **Operationalization** involves defining how to measure a construct using specific, observable variables. **Example**: - **Construct**: Job satisfaction. - **Operationalization**: - Use a survey with Likert-scale items like: - \"I feel valued at work\" (1 = Strongly Disagree, 5 = Strongly Agree). - \"My work environment is conducive to productivity.\" - Observe retention rates or absenteeism as indirect indicators. **Key Terms Explained** - **Conceptualization**: Theoretical definition of a construct. - **Operationalization**: Translating a conceptual definition into measurable variables. - **Measurement**: Collecting and recording data to assess variables. - **Data Collection**: Methods used to gather information for research. - **Primary Data**: Data collected firsthand for a specific study. - **Secondary Data**: Pre-existing data collected for another purpose. - **Obtrusive Research**: Methods where the researcher's presence is evident. - **Unobtrusive Research**: Methods where participants are unaware of being studied. - **Verbal Measurement**: Relies on spoken or written responses. - **Nonverbal Measurement**: Focuses on behaviors, physiological signals, or other non-linguistic cues. **UNIT 7:** **1. Operationalizing a Construct Using Content Analysis of Primary Documents** To operationalize a construct through content analysis: - **Step 1**: **Define the Construct**: Clearly conceptualize the abstract idea. - Example: \"Media bias\" could be defined as unequal representation of political parties in news coverage. - **Step 2**: **Develop a Coding Scheme**: Create categories or codes for observable indicators of the construct. - Example: Count the number of positive, neutral, and negative mentions of political parties in news articles. - **Step 3**: **Analyze Primary Documents**: Apply the coding scheme to analyze primary documents like news articles, policy documents, or transcripts. **2. Explaining How, Why, and When Data Can Be Collected by Means of Content Analysis** - **How**: 1. Select appropriate texts or media (e.g., speeches, policies, articles). 2. Define codes or categories based on the research question. 3. Analyze documents systematically, assigning codes to relevant parts. - **Why**: 1. Content analysis is unobtrusive, allowing researchers to study phenomena without influencing them. 2. It provides insights into patterns, trends, and hidden meanings in communication. - **When**: 1. When studying communication, representation, or cultural artifacts (e.g., how gender is portrayed in advertising). 2. When historical or archival analysis is needed. **3. Coding Texts or Recordings Using a Coding Scheme** - **Coding**: The process of systematically categorizing qualitative data into themes or patterns. - **Steps to Code**: 1. **Develop a Coding Scheme**: Define categories or themes relevant to the research question. - Example: For customer reviews, create codes like \"positive sentiment,\" \"negative sentiment,\" and \"neutral sentiment.\" 2. **Apply Codes**: Read or listen to the material and assign codes to sections of the text or recordings. 3. **Refine the Scheme**: Adjust codes based on emerging patterns or new insights. 4. **Analyze the Data**: Identify themes, trends, or relationships based on coded data. **4. When to Use Content Analysis Compared to Other Methods** - **Use Content Analysis When**: - Research focuses on textual or media data (e.g., analyzing political speeches). - An unobtrusive method is needed to study communication without direct interaction with participants. - Large amounts of archival or documentary evidence need to be systematically analyzed. - **Other Methods May Be Better When**: - Direct interaction is required to understand individual experiences (e.g., interviews). - Observing behavior in real-time is the focus (e.g., ethnographic research). **5. Differentiating Between Inductive and Deductive Coding** - **Inductive Coding**: - Codes emerge from the data without pre-defined categories. - Often used in exploratory research. - Example: Reading interview transcripts to identify recurring themes organically. - **Deductive Coding**: - Codes are defined before analyzing the data, based on theory or prior research. - Often used in confirmatory research. - Example: Using predefined codes like \"social interaction\" and \"self-care\" to analyze patient diaries. **6. Explaining Inter-Coder Reliability** - **Definition**: Inter-coder reliability refers to the degree of agreement between two or more independent coders analyzing the same data. It ensures that the coding process is consistent and not biased by individual interpretations. - **Why It's Important**: - Confirms the reliability of the coding scheme. - Enhances the credibility of the research findings. - **How to Measure**: - Use statistical methods like Cohen's Kappa or Krippendorff's Alpha to assess agreement levels. **Key Terms Explained** - **Unobtrusive Research**: Research methods that do not involve direct interaction with subjects, minimizing influence on behavior. - **Content Analysis**: Systematic analysis of text or media to uncover patterns, themes, or meanings. - **Primary Documents**: Original texts or materials analyzed during research (e.g., transcripts, policy papers). - **Coding**: Categorizing data into themes or variables for analysis. - **Coding Scheme**: A structured set of categories or codes used to analyze data. - **Inductive Coding**: Letting themes emerge organically from the data. - **Deductive Coding**: Applying pre-defined codes based on existing theories or hypotheses. - **Inter-Coder Reliability**: Agreement among coders analyzing the same data, ensuring consistency. **UNIT 8:** **1. Differentiating Between Reliability and Validity of a Measurement Instrument** - **Reliability**: Refers to the **consistency** of a measurement instrument. If the instrument produces the same results under consistent conditions, it is reliable. - **Example**: A thermometer giving the same reading when used repeatedly on the same person in stable conditions. - **Validity**: Refers to the **accuracy** of a measurement instrument. It assesses whether the instrument measures what it is intended to measure. - **Example**: A thermometer should measure body temperature, not ambient temperature, to be valid. **Key Difference**: Reliability is about consistency; validity is about accuracy. A measurement can be reliable but not valid (e.g., a broken scale consistently giving the wrong weight). **2. Why the Quality of a Measurement Instrument Depends on Its (Intended) Use** - **Purpose-Driven Quality**: A measurement instrument's quality is judged based on whether it meets the needs of its intended use. - Example: A questionnaire for assessing anxiety in children might not be appropriate for adults due to differences in language comprehension. - **Context Matters**: The same instrument may perform well in one context but fail in another. - Example: A survey designed for urban populations might not yield valid results in rural areas if it uses urban-centric language or examples. **3. Two Aspects of Measurement Reliability: Stability and Consistency** - **Stability**: Refers to the ability of a measurement instrument to produce consistent results over time (also known as test-retest reliability). - **Assessment**: Administer the same test to the same group after a time interval and compare results. - **Consistency**: Refers to the uniformity of results across items within the instrument (also known as internal consistency). - **Assessment**: Use statistical tests like Cronbach's alpha to evaluate how well items on a scale measure the same construct. **4. Why Measurement Validity Cannot Be Observed Directly** - Validity assesses whether an instrument measures what it is supposed to measure. However, this is an **abstract concept** and cannot be directly observed or measured. - **Example**: You cannot directly observe \"intelligence\" to confirm whether an IQ test truly measures it. **5. Methods to Assess Measurement Validity** 1. **Content Validity**: - **Definition**: Assesses whether the measurement instrument covers the full range of the construct. - **Example**: A job performance survey should include items about teamwork, leadership, and productivity if those are part of the construct. - **Assessment**: Use expert judgment to evaluate coverage. 2. **Construct Validity**: - **Definition**: Assesses whether the instrument is consistent with theoretical expectations of the construct. - **Example**: If a test measures \"motivation,\" scores should correlate with related behaviors like persistence or goal-setting. - **Assessment**: Use correlational studies to see if the instrument aligns with other measures of the same construct. 3. **Criterion-Related Validity**: - **Definition**: Assesses whether the instrument correlates with an external criterion known to measure the construct. - **Types**: - **Predictive Validity**: The instrument predicts future outcomes. - Example: SAT scores predicting college success. - **Concurrent Validity**: The instrument correlates with another measure taken at the same time. - Example: A new anxiety scale correlates with an established one. **Key Terms Explained** - **Measurement Instrument**: Tools used to collect data, such as surveys, tests, or observation checklists. - **Reliability**: Consistency of a measurement instrument; freedom from random error. - **Random Error**: Unpredictable fluctuations that affect measurement reliability. - **Measurement Validity**: Accuracy of the instrument in measuring the intended construct; freedom from systematic error. - **Systematic Error**: Biases or consistent inaccuracies in measurement, reducing validity. - **Data Collection Bias**: Errors introduced by the data collection process, such as leading questions or researcher influence. - **Construct Validity**: How well a measurement aligns with the theoretical concept. - **Content Validity**: How well a measurement covers the full scope of the construct. - **Criterion-Related Validity**: How well a measurement correlates with an external benchmark. **UNIT 15:** **Research Designs** 1. **Correlational Research / Cross-Sectional Research**: - **Definition**: Examines relationships between variables at a single point in time. - **Example**: Studying the relationship between screen time and academic performance using a survey conducted once. - **Limitation**: Cannot establish causality, only associations. 2. **Longitudinal Research**: - **Definition**: Collects data from the same subjects over a period of time to observe changes and trends. - **Example**: Following a group of students over 10 years to study the effect of early education on career outcomes. - **Strength**: Can show changes over time and help infer causality. - **Limitation**: Time-consuming and susceptible to participant attrition. 3. **Interrupted Time Series Design**: - **Definition**: Observes a dependent variable repeatedly over time, with a specific event or intervention (\"interruption\") occurring during the period. - **Example**: Tracking crime rates before and after implementing a new policing strategy. - **Strength**: Can show trends before and after an intervention. - **Limitation**: Difficult to rule out external influences (threats to internal validity). 4. **(Classical) Experiment**: - **Definition**: A controlled study where participants are randomly assigned to experimental and control groups, with a clear manipulation of an independent variable. - **Example**: Testing the effect of a new drug on blood pressure. - **Key Components**: Random assignment (R), treatment (X), observation (O), pretests, and posttests. - **Strength**: High internal validity; establishes causality. 5. **Quasi-Experimental Design**: - **Definition**: Similar to experiments but lacks random assignment. Groups are pre-existing or selected based on specific criteria. - **Example**: Studying the effect of a training program in one school while another school serves as a comparison group. - **Strength**: Useful when random assignment is unethical or impractical. - **Limitation**: Reduced internal validity due to potential selection biases. **Key Experimental Components** 1. **Experimental Group**: The group receiving the treatment or intervention (X). 2. **Control Group**: The group not receiving the treatment, used for comparison. 3. **Double-Blind Experiment**: - Both participants and researchers are unaware of who is in the experimental or control group. - **Purpose**: Reduces biases such as placebo effects and observer bias. 4. **Random Assignment (R)**: - Participants are randomly assigned to experimental or control groups. - **Purpose**: Ensures group equivalence and increases internal validity. 5. **Pretest**: An observation (O) made before the treatment is applied. - **Purpose**: Measures baseline levels of the dependent variable. 6. **Posttest**: An observation (O) made after the treatment is applied. - **Purpose**: Measures the effect of the treatment on the dependent variable. 7. **Treatment (X)**: The independent variable being manipulated in the experiment. - **Example**: A new drug or a training program. 8. **Observation (O)**: The act of measuring the dependent variable in an experimental context. 9. **Placebo**: A substance or procedure with no therapeutic effect given to control groups to mimic the experience of the experimental group. - **Purpose**: Controls for placebo effects, where participants experience changes because they believe they received the treatment. **Validity in Research** 1. **Internal Validity**: - Refers to the extent to which an experiment establishes a causal relationship between the independent and dependent variables. - **Threats**: Confounding variables, selection bias, maturation, and history effects. - **Strengthened By**: Random assignment, control groups, and consistent measurement. 2. **External Validity**: - Refers to the extent to which the findings of a study can be generalized to other settings, populations, or times. - **Threats**: Sample biases, artificial settings, or non-representative populations. - **Strengthened By**: Using representative samples and replicating studies in different contexts. **UNIT 11:** **1. Summarizing Ratio Variables Using Measures of Central Tendency and Spread** - **Ratio Variables**: These are variables where both differences and ratios are meaningful, and they have a true zero point (e.g., height, weight, income). - **Central Tendency**: Measures that represent the center of a distribution of data: - **Mean**: The average of all values. - **Median**: The middle value when the data is ordered. - **Mode**: The most frequent value. - **Spread (or Dispersion)**: Measures that show how spread out the values are: - **Range**: The difference between the maximum and minimum values. - **Variance**: Measures how much the data points differ from the mean (i.e., the average squared deviation). - **Standard Deviation**: The square root of the variance, representing how much the values deviate from the mean on average. It\'s in the same units as the original data, making it more interpretable than variance. **2. Explaining How Standardizing Variables Works and Why You Would Want to Apply This** - **Standardization**: Standardizing a variable means transforming the data to have a mean of 0 and a standard deviation of 1. This is often done using the **z-score** formula. - **Why Standardize?**: - **Comparability**: Standardization allows you to compare variables measured on different scales or with different units. For example, you can compare test scores in mathematics and reading, even if the scales are different (e.g., 0-100 vs. 0-10). - **Normalization**: It normalizes the data, which can be important for techniques like regression or machine learning that assume normally distributed data. - **Standardization Formula**: - ZZ is the z-score (the standardized value). - XX is the original value. - μμ is the mean of the dataset. - σσ is the standard deviation of the dataset. **3. Using the Standard Deviation to Compute Z-Scores in Order to Standardize Variables with Different Variances** - **Z-Scores**: A z-score tells you how many standard deviations a data point is from the mean. - If a z-score is **0**, the value is exactly at the mean. - If a z-score is **positive**, the value is above the mean. - If a z-score is **negative**, the value is below the mean. - **Formula for Z-Score**: - **X**: A raw score or data point. - **μμ**: Mean of the dataset. - **σσ**: Standard deviation of the dataset. - **Why Z-Scores Are Useful**:\ Z-scores allow you to compare values across different datasets that might have different units of measurement or scales, by transforming them to a common scale. - Example: You might want to compare someone\'s income and test scores. If you standardize both variables, you can directly compare how \"far\" they are from the average within each respective distribution, even though the units are different (dollars vs. test scores). **4. Using Statistical Software to Compute the Standard Deviation, Variance, and Mean of Variables** - **Using Software (e.g., R, SPSS, Python, Excel)**: Most statistical software packages allow you to easily compute the mean, variance, and standard deviation of your variables. Here\'s how this can be done in a general sense: - **Mean**: In software: mean(variable) - **Variance**: In software: var(variable) - **Standard Deviation**: In software: sd(variable) **5. Using Statistical Software to Add Variables with Standardized Values to the Dataset** - Once you have standardized a variable (using the z-score formula), you may want to add these standardized values (z-scores) as a new variable in your dataset. **Key Terms:** - **Standard Deviation**: A measure of how much the values in a dataset deviate from the mean. It is expressed in the same units as the data. - **Variance**: The square of the standard deviation. It represents the spread of the data but is in squared units of the original data, which can make it harder to interpret. - **Distributions**: The way data is spread or arranged. Common distributions include normal distribution, uniform distribution, and binomial distribution. - **Z-Scores / Standardized Values**: These indicate how many standard deviations an observation is from the mean of its distribution. A z-score of 1 means the value is one standard deviation above the mean, while a z-score of -1 means it\'s one standard deviation below the mean. **UNIT 16:** **1. Constructing Clear Tables to Confirm or Reject a Hypothesis** - **Purpose of Tables**: Tables are used to present data systematically to evaluate whether the hypothesis is supported. - **Steps**: 1. **State the Hypothesis**: Clearly articulate the null and alternative hypotheses. - Example: *Null Hypothesis*: There is no relationship between education level and income. - *Alternative Hypothesis*: Higher education levels are associated with higher income. 2. **Choose Variables**: Identify the independent variable (education level) and dependent variable (income). 3. **Organize Data**: Use rows and columns to represent categories or levels of the variables. Include percentages for clarity. 4. **Interpret Results**: Assess trends or differences to evaluate the hypothesis. **Example of a Table**: **Education Level** **Low Income (%)** **Medium Income (%)** **High Income (%)** --------------------- -------------------- ----------------------- --------------------- High School 40 35 25 Bachelor's Degree 20 30 50 Master's Degree 10 20 70 **2. Why Confirmation Relates to the Association Aspect of a Hypothesis** - **Association**: Confirming a hypothesis using tables often shows that there is a relationship (correlation) between variables, but this does not prove causality. - Example: A table showing a positive association between education and income does not confirm that education *causes* higher income; other factors (e.g., family background, economic conditions) might be involved. - **Third Variable Aspect**: Tables can partially address whether a third variable might influence the observed association. Including a **test variable** (a third variable) helps determine if the original association holds or changes. **3. Understanding Causality in the Context of Replication and Addition Models** - **Causality**: To infer causality, you must eliminate alternative explanations. The **elaboration model** helps explore relationships between variables: 1. **Replication**: The original association between variables remains consistent across different levels of a test variable. - Example: Education and income are positively associated regardless of gender (test variable). 2. **Addition**: Incorporating new variables to refine understanding of the relationship. - Example: Adding \"work experience\" reveals that the effect of education on income is partly explained by differences in experience. **4. Creating a Contingency Table with Two Layers (2x2x2 Table)** - **Contingency Table**: A table used to show the relationship between two or more categorical variables. A 2x2x2 table introduces a third variable (layer) to explore interactions. **Steps**: 1. Define three variables: - **Dependent Variable (e.g., Support for Policy: Yes/No)** - **Independent Variable (e.g., Income Level: High/Low)** - **Test Variable (e.g., Gender: Male/Female)** 2. Construct the table: Each \"layer\" represents a level of the test variable. **Example of a 2x2x2 Table**: **Layer 1 (Male)**: **Income Level** **Support Policy: Yes (%)** **Support Policy: No (%)** ------------------ ----------------------------- ---------------------------- High Income 70 30 Low Income 40 60 **Layer 2 (Female)**: **Income Level** **Support Policy: Yes (%)** **Support Policy: No (%)** ------------------ ----------------------------- ---------------------------- High Income 80 20 Low Income 50 50 - **Interpretation**: Compare the relationships within and between layers to determine if gender affects the association between income and policy support. **Key Terms Explained** 1. **Elaboration Model**: A framework to refine relationships between variables by introducing a third variable (test variable) to check for replication, explanation, or other patterns. 2. **Test Variable**: The third variable introduced in the elaboration model to evaluate its effect on the relationship between the independent and dependent variables. 3. **Partial Relationship**: The relationship between the independent and dependent variables within levels of the test variable. 4. **Replication (Elaboration Model)**: The original relationship holds across all levels of the test variable, supporting its robustness. 5. **Addition (Elaboration Model)**: Adding variables to a model to understand better or refine causal relationships. 6. **Spurious Relationship**: An apparent association between variables caused by a third variable, not a direct relationship. - Example: Ice cream sales and drowning rates are associated, but the third variable (summer weather) explains the relationship. 7. **Explanation**: A test variable explains the original relationship entirely, making it spurious. 8. **Interpretation**: The test variable mediates the relationship between the independent and dependent variables, providing additional insight. 9. **Specification**: The relationship between variables is only present under certain conditions defined by the test variable. 10. **Suppressor Variable**: A variable that conceals the true relationship between the independent and dependent variables. Adding this variable reveals the suppressed relationship. **UNIT 19:** **1. Differentiate Between Non-Probability Sampling and Probability Sampling** - **Non-Probability Sampling**: - **Definition**: A sampling method where not all members of the population have an equal chance of being selected. The selection process is subjective or based on convenience. - **Examples**: - **Convenience Sampling**: Selecting individuals who are easiest to reach. - **Judgmental/Purposive Sampling**: Selecting individuals based on specific criteria or researcher judgment. - **Snowball Sampling**: Recruiting participants through referrals from initial subjects. - **Quota Sampling**: Ensuring specific quotas for different subgroups but without random selection. - **Advantages**: Easy, quick, and cost-effective. - **Disadvantages**: Not representative of the population; limited generalizability. - **Probability Sampling**: - **Definition**: A sampling method where every member of the population has a known, non-zero chance of being selected, ensuring randomness. - **Examples**: - **Simple Random Sampling**: Every individual has an equal chance of being selected. - **Systematic Sampling**: Selecting every kthkth individual from a list. - **Stratified Sampling**: Dividing the population into strata and randomly sampling from each. - **Cluster Sampling**: Randomly selecting clusters (groups) and then sampling individuals within those clusters. - **Advantages**: Produces representative samples; results can be generalized. - **Disadvantages**: Can be more time-consuming and expensive. **2. The Relationship Between Population, Sampling Frame, and Sample for Probability Sampling** - **Population**: - The entire group you want to study or make inferences about. - Example: All university students in a country. - **Sampling Frame**: - A list or database that represents the population and is used to draw the sample. - Example: A list of all enrolled students at the universities. - **Sample**: - A subset of the population chosen from the sampling frame to participate in the study. - Example: 1,000 randomly selected students from the list. **Relationship**: - The sampling frame should accurately reflect the population to ensure representativeness. If the frame is incomplete or biased, the sample will not represent the population well. **3. Consequences of Sampling Error, Sampling Bias, and Non-Response** - **Sampling Error**: - **Definition**: The natural variability that occurs because only a subset of the population is sampled, not the entire population. - **Consequence**: Results may differ from the true population values, but error can be minimized with larger or more representative samples. - **Sampling Bias**: - **Definition**: Systematic error in the sampling process that causes certain groups to be over- or underrepresented. - **Example**: Using a phone survey might exclude people without access to phones. - **Consequence**: Results may be skewed and not generalizable to the population. - **Non-Response**: - **Definition**: When selected individuals do not or cannot participate in the study. - **Example**: If busy professionals are less likely to respond, their perspectives might be missing. - **Consequence**: Non-response can lead to bias if non-respondents differ systematically from respondents. **4. Compute the Response Rate** The **response rate** is the percentage of individuals from the sample who complete the survey or participate in the study. It is calculated as follows: Response Rate=(Number of ResponsesTotal Number of Individuals Contacted)×100Response Rate=(Total Number of Individuals ContactedNumber of Responses)×100 - **Example**: - Number of responses: 500 - Total individuals contacted: 1,000 - Response rate:Response Rate=(5001,000)×100=50%Response Rate=(1,000500)×100=50% A high response rate is desirable to reduce non-response bias. **Key Terms** 1. **Population**: The entire group you aim to study or make conclusions about. 2. **Sampling Frame**: A list or database representing the population from which the sample is drawn. 3. **Non-Probability Sampling**: Sampling methods where not all population members have an equal chance of selection. 4. **Probability Sampling**: Sampling methods ensuring all population members have a known and equal chance of selection. 5. **Simple Random Sampling**: A method where every individual has an equal chance of being selected. 6. **Representativeness/Representative Sample**: A sample that accurately reflects the population's characteristics. 7. **Sampling Error**: Variability in sample results due to studying a subset rather than the entire population. 8. **Sampling Bias**: Systematic error leading to an unrepresentative sample. 9. **Non-Response**: Selected individuals fail to participate, potentially biasing results. 10. **Response Rate**: The percentage of individuals from the sample who participate in the study. **UNIT 25:** **1. Explain the Different Types of Validity Threats** **Validity threats** refer to factors that can compromise the accuracy of study results. They are typically divided into threats to **internal validity** (affecting causality) and **external validity** (generalizability). **Internal Validity Threats** These affect the ability to infer a causal relationship between the independent and dependent variables. 1. **History**: - **Definition**: Events outside the study that occur during the research period and affect participants. - **Example**: A policy change or natural disaster during a longitudinal study on education outcomes. 2. **Maturation**: - **Definition**: Changes within participants over time due to natural growth or development rather than the treatment. - **Example**: Improved reading skills in children simply because they age, not because of an intervention. 3. **Testing and Reactivity**: - **Definition**: When taking a pretest influences participants\' behavior in the posttest. - **Example**: Students perform better in the second round of testing because they're familiar with the test format. 4. **Instrument Change**: - **Definition**: Changes in the measurement tool or method over time that affect consistency. - **Example**: Switching to a different test midway through a study on academic performance. 5. **Selection**: - **Definition**: Systematic differences between groups before the intervention begins. - **Example**: Comparing two classes where one is already more motivated than the other. 6. **Attrition (Mortality)**: - **Definition**: Participants dropping out of the study in a non-random manner. - **Example**: High-achieving students drop out of a study on educational interventions, skewing results. 7. **Regression to the Mean**: - **Definition**: Extreme scores tend to move closer to the average upon retesting, unrelated to treatment effects. - **Example**: Students who performed exceptionally poorly in the first test improve in the second test due to random fluctuation. **2. Consequences of Validity Threats** - **Distorted Results**: - Study findings might reflect external factors or random variability rather than the intended relationship between variables. - **Misleading Conclusions**: - Researchers may incorrectly infer causality or overgeneralize results. - Example: Assuming an intervention is effective when improved outcomes are due to maturation. - **Reduced Generalizability**: - External validity threats can limit the ability to apply findings to broader populations or contexts. - **Ineffective Policies or Treatments**: - When studies inform real-world decisions, validity threats can lead to ineffective or harmful interventions. **3. How to Reduce the Risk of Validity Threats** **Design Strategies to Minimize Threats** 1. **History**: - Use a control group exposed to the same external conditions. - Shorten the study duration to reduce the risk of intervening events. 2. **Maturation**: - Include a control group to distinguish between natural development and treatment effects. - Randomize participants to ensure comparable maturation rates across groups. 3. **Testing and Reactivity**: - Avoid pretests when possible or use alternative designs (e.g., Solomon Four-Group Design). - Use different but equivalent versions of the test for pretest and posttest. 4. **Instrument Change**: - Ensure consistent measurement tools and procedures throughout the study. - Train researchers to maintain consistency in data collection. 5. **Selection**: - Randomly assign participants to groups to ensure equivalence. - Use matching techniques to create similar groups. 6. **Attrition (Mortality)**: - Minimize dropouts by maintaining participant engagement. - Conduct analyses to determine whether attrition is random or systematic. 7. **Regression to the Mean**: - Use random assignment to reduce its impact. - Avoid basing interventions on extreme scores alone. **Key Terms Explained** 1. **History**: External events affecting participants during the study. 2. **Maturation**: Natural changes within participants over time. 3. **Testing and Reactivity**: Influence of testing on participant behavior. 4. **Instrument Change**: Inconsistent measurement tools or methods. 5. **Selection**: Pre-existing differences between groups. 6. **Attrition (Mortality)**: Participants dropping out of the study. 7. **Regression to the Mean**: Extreme scores moving closer to the average over time. **UNIT 26:** **How, Why, and When Data Can Be Collected by Observation** **How Observation is Conducted** - **Structured Observation**: - Predetermined categories or variables guide the data collection process. - Example: Recording the number of times a child shares toys during a 10-minute observation. - **Less-Structured Observation**: - Data collection is more flexible, often without a strict framework. - Example: Descriptive field notes documenting how children interact during free play. **Why Use Observation** - **Direct Data Collection**: Captures real-world behavior without relying on self-reports or recollections. - **Rich Detail**: Provides contextual information that might be missed in surveys or experiments. - **Unobtrusiveness**: Can observe natural behaviors without participants\' awareness in certain contexts. **When to Use Observation** - To study behaviors that are difficult to measure through other means (e.g., non-verbal communication). - When contextual or situational factors are critical (e.g., group dynamics). - In exploratory research to generate hypotheses. **2. Advantages and Disadvantages of Observational Research** **Advantages** 1. **Ecological Validity**: Reflects natural behavior, particularly in naturalistic observation. 2. **Unfiltered Insights**: Captures behaviors participants might not self-report. 3. **Flexibility**: Suitable for structured and unstructured data collection. 4. **Visual and Contextual Data**: Observers can document non-verbal cues, surroundings, and interactions. **Disadvantages** 1. **Observer Bias**: Observers\' interpretations might affect data. 2. **Hawthorne Effect**: Participants may alter behavior if they know they\'re being observed. 3. **Time-Consuming**: Can require significant time and resources for training and data collection. 4. **Limited Scope**: Hard to generalize findings if observations are context-specific. 5. **Reliability Challenges**: Consistency in coding or interpretation can be difficult to maintain. **3. Creating an Observation Schedule** An **observation schedule** is a structured framework for systematically recording behaviors or events. **Steps to Create an Observation Schedule** 1. **Define the Variable**: - Decide on the specific behaviors or actions to observe. - Example: Observing instances of helping behavior in a classroom. 2. **Determine Categories**: - Break down the variable into observable, measurable components. - Example: Helping behavior might include picking up dropped items, assisting with tasks, or offering encouragement. 3. **Select Sampling Method**: - **Event Sampling**: Record every occurrence of the behavior of interest. - **Time Sampling**: Observe at specific intervals (e.g., every 5 minutes for an hour). 4. **Establish Coding Guidelines**: - Use clear, unambiguous criteria for recording observations. - Example: Define \"helping\" as \"providing unsolicited assistance to another person.\" 5. **Pilot-Test the Schedule**: - Test it on a small group to ensure clarity and reliability. **4. New Technologies in Observational Research** - **Wearable Cameras**: Provide a first-person perspective for recording behavior unobtrusively. - **Mobile Apps**: Allow observers to record data in real-time using pre-designed templates. - **AI and Machine Learning**: Analyze video recordings to detect and classify behaviors automatically. - **Eye-Tracking Devices**: Monitor where participants look during specific tasks or situations. - **Sensors**: Capture physiological data (e.g., heart rate, movement) during observations. **5. General Threats to Reliability and Validity in Observational Research** **Threats to Reliability** 1. **Observer Bias**: - Observers may interpret behaviors differently based on their expectations or prior knowledge. 2. **Inconsistent Coding**: - Variations in how different observers record or interpret the same behavior. 3. **Environmental Variability**: - Uncontrolled changes in the environment might affect observations. **Threats to Validity** 1. **Hawthorne Effect**: - Participants alter their behavior because they know they\'re being observed. 2. **Limited Generalizability**: - Results from a specific context or group may not apply to others. 3. **Observer Influence**: - The presence of the observer might inadvertently affect participants\' behaviors. 4. **Sampling Bias**: - Choosing unrepresentative events or times for observation. **Key Terms** 1. **Naturalistic Observation**: Observing behavior in its natural environment without interference. 2. **Contrived Observation**: Observing in a controlled, artificial setting created by the researcher. 3. **(Non-)Participatory Observation**: - **Participatory**: The observer becomes part of the group being studied. - **Non-Participatory**: The observer does not interact with participants. 4. **Observation or Rating**: - **Observation**: Directly recording behaviors as they occur. - **Rating**: Assigning subjective scores based on observed behaviors. 5. **Observation Schedules**: Structured tools for recording specific behaviors systematically. 6. **Event Sampling**: Recording every occurrence of a specific behavior. 7. **Time Sampling**: Observing and recording behaviors at set intervals.