Resilient Control for Macroeconomic Models PDF
Document Details
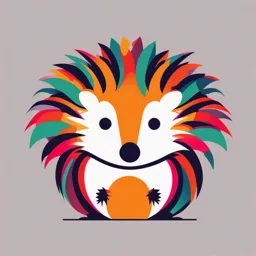
Uploaded by ValuableIndianapolis7374
Texas A&M University–Corpus Christi
2022
David Hudgins, Patrick M. Crowley
Tags
Summary
This research paper develops a framework for resilient macroeconomic control. It analyzes optimal feedback fiscal and monetary policies in response to large negative incidents using a discrete-time soft-constrained linear-quadratic dynamic game. The authors highlight the importance of resilience in policymaking, particularly in response to events such as the 2020 economic crisis.
Full Transcript
Computational Economics (2023) 61:1403–1431 https://doi.org/10.1007/s10614-022-10246-6 Resilient Control for Macroeconomic Models David Hudgins1 · Patrick M. Crowley2 Accepted: 22 February 2022 / Published online: 2 April 2022 © The Author(s), under exclusive licence to Springer Science+Business...
Computational Economics (2023) 61:1403–1431 https://doi.org/10.1007/s10614-022-10246-6 Resilient Control for Macroeconomic Models David Hudgins1 · Patrick M. Crowley2 Accepted: 22 February 2022 / Published online: 2 April 2022 © The Author(s), under exclusive licence to Springer Science+Business Media, LLC, part of Springer Nature 2022 Abstract This paper derives a macroeconomic resilient control framework that provides the optimal feedback fiscal and monetary policy responses in response to a potentially large negative external incident. We simulate the model for the U.S. under the con- ditions that prevailed throughout the 2020 economic crisis that occurred due to the government lockdown that was caused by the coronavirus pandemic. We develop a discrete-time soft-constrained linear-quadratic dynamic game under a worst-case design with multiple disturbances. Within this context, we introduce a resilience feed- back response and compare the case where the policymakers counter in response the external incident with the case when they do not counter. This framework is especially applicable to large-scale macroeconomic tracking control models and wavelet-based control models when formulating the magnitudes of the policy changes necessary for the unemployment rate and national output variables to maintain acceptable track- ing errors in the periods following a major disruption. Our policy recommendations include the maintenance of “rainy day” funds at appropriate levels of government to mitigate the effects of large adverse events. Keywords Linear-quadratic · Minimax · Resilience control · Wavelet analysis JEL Classification C61 · C73 · E58 · E61 B David Hudgins [email protected] Patrick M. Crowley [email protected] 1 Department of Decision Sciences and Economics, College of Business, OCNR 375, 6300 Ocean Drive, Texas A & M University – Corpus Christi, Corpus Christi, TX 78412, USA 2 Department of Decision Sciences and Economics, College of Business, OCNR 323, 6300 Ocean Drive, Texas A & M University – Corpus Christi, Corpus Christi, TX 78412, USA 123 1404 D. Hudgins, P. M. Crowley 1 Introduction The triad of modern control systems properties includes resilience, robustness, and security (Huang et al., 2020). Resiliency, or in model terminology resilient control, refers to policy approaches aimed at restoring a system in response to unforeseen rare external incidents1 (or what some economists might refer to as “shocks”2 ). If resiliency is the ability, ex-post, to rebound from the effects of an adverse incident, then robustness is the setting of macroeconomic policy, ex-ante, so as to encompass as many probable future scenarios as possible. One variation on robustness is minimax worst-case robust control which aims to formulate optimal policy that operates functionally under a given range of adverse disturbances. Neither resilience nor robustness are general properties of a system, however, since a system may be either resilient or robust to one set of disturbances, yet may be highly vulnerable to others (Mili, 2011). There is generally a tradeoff between the two control aspects of robustness and resilience. In order to achieve resilience, the policymakers use control policy resources to bring the system back into normal operation following the impact of a negative incident. Robustness, on the other hand, requires that policymakers utilize resources to obtain optimal performance in each specified operating state (Zhu & Basar, 2011).3 Centralized systems are typically more robust but less resilient than decentralized systems, but may fail to respond to unexpected events, while decentralized systems are typically more resilient to unexpected incidents or attacks (Zhu & Basar, 2011). Lastly, security refers to the ability of the system to resist negative external events or malicious attacks (Huang et al., 2020). There are many examples of the tradeoff between resilience and robustness in macroeconomics, but here we just provide two. The severe economic downturn during the “great recession” of 2008–2009 was far outside the feasible predictions of common macroeconomic forecasting methods, such as DSGE models and the BVAR models (Lindé, 2018). Moreover, these models do not account for the asymmetry where the negative effects of recessionary gaps are far more damaging than the positive effects of unexpectedly strong growth. These two aspects demonstrate the need to seriously consider worst-case robust and resilient approaches when formulating policy. The economic decline in the second quarter of 2020 that resulted from the coron- avirus lockdowns led to a projected 10% quarterly drop in real GDP, which represents 1 By “external”, we mean external to the economy, and not necessarily international (such as a terrorist attack, military conflict or public health pandemic), and by “incident” we mean any event that is a novel development that has significant ramifications for the economy. 2 We have avoided the use of the term “shock” in this paper, given the wide variety of connotations that this word has taken on in the subject of economics, and due to the fact that there is some controversy as to whether recessions are always the result of shocks. This is borne out by the fact that the 2020 recession was caused by government public health recommendations in response to a public health pandemic. Indeed, some policymakers have referred to the 2020 economic downturn as “recession by design” and not directly caused by economic shocks at all. The term “event” is therefore utilized, which then also encompasses the usual definition of “shock” in economics. 3 This tradeoff also occurs in econometric studies, such as (Klimek, Poledna, and Thurner, 2019) which uses linear response theory (LTR) to estimate responses to “shocks” in a macroeconomic Leontief input–output (IO) model. An external “shock” causes a new nonequilibrium state to arise that is influenced by two opposing forces that attempt to achieve a balance between a relaxation to the original equilibrium state and the new equilibrium state implied by the external “shock”. 123 Resilient Control for Macroeconomic Models 1405 the largest single-quarter drop since 1947. This decline is four times larger than the GDP decline in 1958 quarter 1, during the Eisenhower Recession, which previously was the worst quarter recorded.4 This unprecedented 2020 economic decline repre- sented an unexpected external incident that moved the economy away from equilibrium and beyond any forecasted projections. Since the economic system failed in terms of security and robustness, this incident demonstrates the need to examine resilience when formulating policy. The purpose of this paper is to develop a resilient control framework for obtaining optimal feedback fiscal and monetary policy responses under large negative exter- nal incidents. First, we construct a discrete-time soft-constrained LQ dynamic game under a worst-case design with multiple disturbances. This framework is especially applicable to large-scale macroeconomic tracking control models and wavelet-based control (WBC) models when the distribution of the disturbances is unknown, when there may be model misspecification, or when the policymakers are concerned with obtaining maximum disturbance robustness. Next, we simulate a WBC version of the model for the U.S. under the conditions that prevailed throughout the 2020 economic crisis. Within this context, we introduce a resilience feedback response and compare the case where policymakers counter against the external incident with the case when they do not. 1.1 Minimax Robust Control Under worst-case modeling, the policymaker is concerned about the effects on the economy if the reference model is misspecified and/or the probability distributions of the disturbances are unknown. Typically, robust models account for this by including additive disturbances that are determined through feedback from the entire state-space model (Leitemo & Soderstrom, 2008). Since the disturbances impact the model in the same way as multiplicative parameter uncertainty or omitted variables, robust control represents a general approach to model uncertainty (Hansen & Sargent, 2007; Leitemo & Soderstrom, 2008). The robust methods of Hansen and Sargent (2008) that use entropy to measure model misspecification in the time and frequency domains have been widely employed in macroeconomics; these methods, however, do not address the optimal tracking control problem. Minimax approaches to linear-quadratic (LQ) H∞ -optimal control achieve robust designs by minimizing a performance index under the worst possible disturbances, where those disturbances maximize that same performance index. Although adaptive control and linear-quadratic Gaussian (LQG) models produce optimal policies when models are correctly specified, these polices can result in poor performance and insta- bility when the model or disturbances are misspecified (Tornell, 2000). H∞ -optimal control has been widely applied in engineering and economics, with many examples such as Basar and Bernhard (1991), Basar (1992), Hansen et al. (1999), Rustem and Howe (2002), Zakovic et al. (2007), Hansen and Sargent (2008), Hudgins and Chan (2008), Hudgins and Na (2016), and Hudgins and Crowley (2019). 4 See the U.S. CBO macroeconomic variable projections in the document for April, 2020, available at https://www.cbo.gov/publication/56335. 123 1406 D. Hudgins, P. M. Crowley There are four limitations for using H∞ -optimal control in economics. First, if the system is formulated incorrectly, then optimizing the wrong controller makes perfor- mance worse, instead of better. Secondly, many analyses model the interdependent prices and agent’s decisions separately, thus H∞ -methods cannot be applied (Tor- nell, 2000). Thirdly, the computational solution can be difficult. Lastly, most of the macroeconomic policy forecasting models, such as Kendrick and Shoukry (2014), Taylor (1993), and the various central bank forecasting models are large-scale, with multiple equations and disturbances, where the policymaker’s preference for robust- ness varies across equations. Wavelet-based control (WBC) models, which contain variables that are wavelet- decomposed to account for cycles in the time–frequency domain, create additional equations and disturbances, thus further expanding the size of the model and the com- putational complexity (Crowley & Hudgins, 2015). But the ability to assign different disturbance weights across different targets, frequencies, and equations is particularly useful for policy modeling (Hudgins & Crowley, 2019; Leitemo & Soderstrom, 2008). In terms of the recent literature using these methods in macroeconomics, Hudgins and Na (2016) simulated a multiple-parameter design and a mixed stochastic/single param- eter H∞ -optimal control design for an aggregate model, and then Hudgins and Crowley (2019) was the first paper to integrate minimax robust optimal control policies and dynamic non-cooperative game theory with a WBC model. The optimal policy stance in robust control designs can be more cautious or more aggressive than in alternate model specifications (Bernhard, 2002). Hudgins and Na (2016) and Hudgins and Crowley (2019) find that fiscal policy is more aggressive under the minimax robust design. Onatski and Stock (2002) and Dennis et al. (2009) also find that robust monetary policy is relatively more active. These results contradict the less active monetary policy rules obtained in Zakovic et al. (2007) and Barlevy (2011), which uphold the Brainard principle (Brainard, 1967), which states that increased uncertainty decreases monetary and fiscal policy activism. Leitemo and Soderstrom (2008) partially reconcile the more aggressive versus more passive robust policies by finding that the type of external shock and source of misspecification determine whether monetary policy is more aggressive or more cautious. 1.2 Resilient Control Concepts Resilient control is an ex-post incident concept that models the system’s ability to recover after negative events occur (Huang et al., 2020). Resilient control policies are designed to restore performance after robustness and security fail under unexpected adverse external incidents, so that the system can self-recover from the impact of the deterioration. Whereas the term “resilience” was originally studied in the early 1970s in the fields of ecology and psychology, resilience is now often used to describe the efforts of businesses, communities, and governments to enhance their ability to rapidly recover from crises such as natural disasters, terrorist incidents, or cyberattacks (Wei & Ji, 2010). Smith, Pereyda, and Gammel (2016) explain eight cybersecurity best practice for resilient control systems, where practices 4 – 7 are applicable to economic policy: have 123 Resilient Control for Macroeconomic Models 1407 a contingency plan; patch, update, and maintain; don’t forget physical security; and learn from events. In terms of a contingency plan, the study points out that something will go wrong, so that it is not a matter of “if”, but rather “when”. Thus, a good control defender will always have a plan, or plans, to respond to a crisis. Wei and Ji (2010) give four properties of resilient industrial control systems: (1) the incidence of undesirable incidents can be minimized; (2) most of the undesirable incidents can be mitigated; (3) adverse impacts of undesirable incidents can be min- imized; (4) ability to recover from the adverse impacts of undesirable incidents to normal operation in the shortest time. Yang and Sydnor (2012) stress the importance of viewing the resilient control system as a large coupled system designed by using multiple input-multiple output (MIMO) methods, such as optimal control, and H∞ - control. That study emphasizes that robust control design improves the survivability of resilient control systems since it mitigates the adverse effects of modeling error and unpredicted disturbances under normal operating conditions, and thus reduces the chance of penetrating multiple defensive layers. Zhu and Basar (2011) develop a hybrid framework for a cyber-physical system that integrates a minimax worst-case continuous-time H∞ -control system with a discrete- time stochastic uncertainty that models external incident shocks, or cyberattacks, that cause a change in the system operation at random times. In this framework, the defense against cyberattacks involves human decision-making, and thus involves a longer time-scale than the attacks. The dynamic-game model parameters yield a stationary saddle-point solution strategy where the defender is always defending, and the attacker should not be attacking. Our approach follows both Zhu and Basar (2011) and Yang and Sydnor (2012) by integrating resilient control within a robust optimal control model. To our knowledge, this paper is the first to explore resilient control within a macroeconomic context. Klimek, Poledna, and Thurner (2019) explore resilience in an econometric model impulse-response model, but not in an optimal control framework. Martini (2020) sur- veys the socio-economic literature on regional resilience, and explores the relationship between economic structure and regional resilience. Our procedure is designed for macroeconomic tracking models where the policymakers are interested in robustness to worst-case disturbances in the normal business cycle, and also achieving resilience to large negative incidents that might occur irregularly. This is therefore also the first paper to integrate wavelets and resilient control. 2 Model Specification The policymakers’ objective is to minimize the discrete-time quadratic tracking index in Eq. (1), where y denotes a state vector, z denotes a policy vector, the target vectors are defined with a superscript (*), k denotes the time period (or stage), and the superscript (T ) represents the matrix transpose. T min E(J ) y K +1 − y∗K +1 f y K +1 − y∗K +1 z K T T (1) + y K − y∗K k y K − y∗K + z K − z∗K Rk z K − z∗K k1 123 1408 D. Hudgins, P. M. Crowley The first term in Eq. (1) penalizes the policymakers for the tracking errors in the final state vector (with penalty matrix f ), and the second term penalizes the tracking errors in the state vector in each period (with penalty matrix k ). The last term penalizes the tracking errors for the control vector (with penalty matrix Rk ). In macroeconomic models, the state variables may include a variety of variables, such as real GDP and/or some of its components, inflation, unemployment, and the exchange rate, while the policy variables may include variables such as government spending, taxes, interest rates, and the money supply (Hudgins & Na, 2016; Kendrick & Shoukry, 2014; Leitemo & Soderstrom, 2008). Our paper expands the large open-economy WBC model of Crowley and Hudgins (2019), where the policy vector includes the interest rate and government spending at each of five wavelet frequency ranges, and the state vector includes a variety of domestic and foreign variables, such as real GDP. The tracking index is subject to the linearized difference equation system in Eq. (2): yk+1 α 0,k + α 1,k yk + · · · + α ρ yk−ρ+1 + β 1,k z k + · · · + β θ,k z k−θ+1 + δ k (εk + ψ k ); y (1) y1 (2) The state vector contains ρ lags, and the policy vector contains θ lags, since most time-series models and WBC models consist of econometric equations with more than one-lag. In the last term, ε is a stochastic disturbance vector, where some elements may have unknown distributions. Following the typical robust modeling specifications, as in Leitemo and Soderstrom (2008), ψ is a vector containing unknown equation mis- specification errors that enters the equation as an additive term, where the policymaker is unable to observe the individual values of ε and ψ. Equation (2) can be specified as a time-variant system where the coefficients change over time, or as a time-invariant sys- tem with fixed coefficients. The equations in this paper are modeled as time-invariant with fixed coefficient estimates.5 In order to make the minimax worst-case design tractable, the LQ-tracking problem given by Eqs. (1) and (2) must first be converted into a LQ-regulator problem via four transformations. First, the state vector needs to be augmented by embedding the constants (α0 ) from the econometric equations, using the procedures presented in Crowley and Hudgins (2015, 2017). This is achieved by introducing a new variable ck , where ck ck−1 for all k 1,…,K, and c1 1. The system state vector must also be further augmented by embedding the state target vector. These two procedures increase the dimensions of the augmented state vector. 5 The authors have created a MATLAB program that allows the user to input any given coefficient, or set of coefficients, as a function of the time index k, thus allowing the chosen coefficients to vary over time. Since this analysis focuses on the computational capability to analyze resilient control policies, the time-varying comparisons are beyond the scope of this paper. 123 Resilient Control for Macroeconomic Models 1409 Secondly, the state-space system must be formulated as a first-order matrix differ- ence equation by further augmenting the state vector with each of the lags of the state and control vectors, as in Chow (1975, pp. 227 – 238). Thirdly, the state vector must further be augmented by including the state and control target vectors, as in Crowley and Hudgins (2015, 2017). These augmentations to the original state vector substan- tially increase the number of state variables when there are multiple state and control variables and/or multiple lags. The new augmented state vector is given by Eq. (3): T x k ck ; ykT ; yk−1 T ;... ; yk−ρ T T ; z k−1 T ; z k−2 ;... ; z k−θ T ; y∗kT ; z∗k−1 T (3) Fourthly, a new control vector uk needs to be defined as in Crowley and Hudgins (2015), where the new vector embeds the original control tracking errors as follows: T uk u 1,k ; u 2,k ;... ; u m,k u j,k z j,k − z ∗j,k for j 1,... , m (4) The control target variables are then added to each of the state equations that contain these variables, where the values of the original control variables (zj, k ) can be recovered with a one-period lag by embedding them within the augmented state vector (xk ) in Eq. (3). Upon completion of the above transformations, the LQ tracking model can be written in a standard LQ-regulator format, where the policy objective is to minimize the performance index in Eq. (5) subject to the state Eq. (6), where xk is the new augmented state vector, and wk is a vector of disturbance terms that contain the sum of indistinguishable random variable errors and model misspecification errors. The matrices f and k are augmented to become Qf and Qk so that they can incorporate the new expanded state and control vectors. K min E(J ) x TK +1 Q f x K +1 + x kT Q k x k + ukT Rk uk (5) u k1 x k+1 Ak x k + B k uk + Dk w k x(1) x 1 (6) Qf is a positive semi-definite matrix, Qk is a positive semi-definite matrix, for all k 1, 2, …, K Rk is positive definite, for all k 1, 2, …, K k ∈ [1, K] x ∈ Rn u ∈ U ⊂ U w ∈ W ⊂ W , U is the control constraint set, which is a subset of the Hilbert space U. W is the disturbance constraint set, which is a subset of the Hilbert space W. Assume that U and W are compact. The resilient control model in this paper contains 138 state equations, which includes wavelet-decomposed versions of real GDP and its components, inflation, money supply, unemployment, lags of some of the variables, and the embedded tar- gets. As in Kendrick (1981), the penalty weights that form the elements of the Qf , Qk , and Rk matrices are chosen by the policymaker, and can be time variant or invariant. The policymaker is required to calibrate a large number of penalty elements due to the 123 1410 D. Hudgins, P. M. Crowley large size of matrices. The penalty parameters used in the different simulation cases in this paper are provided in the appendix.6 2.1 Minimax Robust Design In minimax control, the objective is formulated as a 2-player zero-sum dynamic non- cooperative game. The policymaker is player 1, and uses fiscal and/or monetary policy to minimize the objective function Jγ. Nature is player 2, and nature chooses the disturbance values so as to maximize the objective function. The multiple-parameter minimax system reformulates the optimal control problem in (5) and (6) as a soft- constrained dynamic game where the disturbance vector is weighted by a positive definite penalty matrix, G, so that a distinct weight can be chosen for each of the disturbance terms in the w vector. The minimax game is then defined as follows: K min max Jγ x TK +1 Q f x K +1 + x TK Q k x k + ukT Rk uk − wkT Γ wk (7) u w k1 subject to Eq. (6), where ⎡ ⎤ γ1 0 0 0 ⎢0 γ2 0 0 ⎥ ⎢ ⎥ ⎢. ⎥ γ1 , γ2 , γ p... , > 0 (8) ⎣0 0.. 0 ⎦ 0 0 0 γp The model specification is more general than the designs in Basar and Bernhard (1991), which does not consider the tracking design, restricts the control variable penalty matrix Rk to be an identity matrix, and does not allow for multiple distinct disturbance weights. Our specification is also more general than the systems explored by Hudgins and Na (2016), which again restricts the control variable penalty matrix Rk to be an identity matrix. Hudgins and Crowley (2019) considers the case where only a subset of the disturbances are modeled by a worst-case design in a WBC model, while another set of disturbances is stochastic. Thus, the method explored in this paper contributes to the literature in both the generalization of the minimax setup and in terms of the resilient control design. The generalization to include multiple robust disturbance weights in the minimax index is crucial in economic modeling. Leitemo and Soderstrom (2008) emphasize the importance of assigning different disturbance weights across different targets and equations. Additionally, WBC models typically utilize political cycle targeting that allocates relative more importance to economic performance at the political cycle 6 In the LQ tracking design, only the relative size of the parameters has meaning, since a relatively larger penalty weight on a given tracking error causes a larger relative emphasis on achieving that target compared to achieving the other targets in the model. In most macroeconomic control models, some of the final period parameters have larger values than in the other periods, so that there is more emphasis on achieving the objective at the end of the time horizon. A set of calibrated penalty values for a large WBC closed-economy model of the U.S. economy are given in the appendix of Crowley and Hudgins (2019). 123 Resilient Control for Macroeconomic Models 1411 frequency ranges of 2 to 8 years, which requires that the policy variables and the disturbances be weighted differently across frequency ranges (Crowley & Hudgins, 2015). The formulation presented here specifies a “minimax game”, where policymak- ers are minimizing the expected disutility, given that nature is generating the worst possible disturbances. The policymakers are minimizing the control effort, while the disturbances are assigned their worst-case values such that the system still has a solu- tion. Let the model assume that both players have access to closed-loop perfect state information with memory. Define the recursive matrix sequences M k and 2k , k ∈ [1, K], where 2k is invertible, by Eqs. (8) and (9): −1 M k Q k + AkT M k+1 k Ak ; M k+1 Q f (9) k I + B k R−1 k B T K − D k Γ −1 T D k M k+1 (10) As derived in Basar and Bernhard (1991, pp. 28 – 47), the saddle-point solution to this game is found by recursively computing Eqs. (9) and (10). The two matrices M k and 2k are thus computed offline in retrograde time. The existence of a saddle-point solution requires that: Γ − DkT M k+1 Dk > 0 ; k 1, 2,... , K (11) A unique (and global) feedback saddle-point solution exists if, and only if, the condition in Eq. (11) is satisfied. If the matrix given by Eq. (11) has one or more negative eigenvalues, and therefore is not positive definite, then the game does not have a saddle-point solution, and its upper value is unbounded. The MATLAB program we created for this research issues a warning to the user, and it will cease calculation if Eq. (11) has any negative eigenvalues for any period. The optimal control and the optimal disturbance trajectories are given by Eqs. (12) and (13), respectively7 : −R−1 −1 O ptimal T uk k B k M k+1 k Ak x k (12) −1 w∗k Γ −1 DkT M k+1 k Ak x k (13) 2.2 Disturbance Attenuation Policymakers achieve a robust design by selecting relatively smaller values for the penalty weights on the diagonal of the G matrix. When the diagonal elements of 7 This solution is derived in detail by Basar and Bernhard (1991, pp. 28 – 47) for the case where G is a scalar and R is an identity matrix. The solution for the current specification is the same, except that G has been generalized to a square matrix with the appropriate dimensions, and the matrix R is positive definite matrix that is not restricted to be an identity matrix. 123 1412 D. Hudgins, P. M. Crowley the G matrix are each equal to a given constant γ H , then the problem reduces to an H∞ -optimal control design (Hudgins & Na, 2016). The H∞ -optimal control design with disturbance attenuation provides the maximum possible robustness by generating the optimal control under the worst-case overall error structure as measured by the Euclidean norm of the disturbance vector w. Robust control seeks to stabilize the system under the worst-case disturbances, which occurs when the magnitudes of the errors are the largest. This can be achieved by choosing a small value for γ H , which causes the disturbances to be as disruptive as possible under the game specification. Disturbance attenuation refers to the process of choosing the value of γ H so that the errors are generating a maximum disruption, thus forcing the policymaker to choose controls that attenuate the worst-case performance of the system, as discussed in Basar and Bernard (1991), Tornell (2000), and Hudgins and Crowley (2019). Disturbance attenuation requires the value γ H * > 0 to be chosen for γ H , such that γ H * is the infimum, which is the smallest value of γ H that still allows for a saddle-point solution of the minimax game. Whereas γ H is a free parameter in the general minimax game, γ H * must be set at the infimum of γ H for disturbance attenuation so that the optimal control stabilizes the system in the worst-case.8 Pareto attenuation replaces H∞ -disturbance attenuation in the multiple-parameter minimax framework when the penalty weights for the disturbances are different num- bers. The policymaker must specify the DPR (disturbance penalty ratio) so that each disturbance is assigned a penalty weight relative to the weight of an arbitrarily chosen diagonal element of the G matrix. Then, Pareto attenuation is achieved by selecting the smallest set of penalty weights where the saddle-point conditions in (9) – (11) are satisfied (Hudgins & Na, 2016). The disturbance attenuation value for the game depends upon the diagonal elements of the G matrix, and will change if any of the diagonal elements change. After the DPR is chosen, the penalty disturbance weights are no longer free parameters, and cannot be arbitrarily chosen. 2.3 Resilient Control Modeling This section discusses how resilience is integrated into our model. There is a large of amount of literature that has previously explored robust control applications. The topic of resilient control is recent, however, and the literature is currently limited. In fact, since the literature on resilient control is still in its infancy, the approaches and terminologies have not been standardized. The terms resilience, robustness, adap- tiveness, survivability, and fault-tolerance have been used interchangeably (Wei & Ji, 2010). These terms are do not refer to exactly identical concepts, even though they do share some common elements (Wei & Ji, 2010). It is important to distinguish between resilience and robustness in order to highlight the resilience aspect of the control model. 8 In order to achieve a global solution for disturbance attenuation, the initial value for the state vector must be set at x1 0. Since this is generally not the case for macroeconomic models, the problem can be solved by adding one additional initial period where x0 0, and x1 x0 + x1 e + w0 , and then solving the problem. See Basar and Bernard (1991, Chapter 3) for details. 123 Resilient Control for Macroeconomic Models 1413 Huang et al., (2020) limit the definition of resilient control to only refer to control policies that return the system to normal after the system breaks down following a catastrophic external event that exceeds the robustness and security tolerances of the system. Wei and Ji (2010) employ a broader definition, whereby the proactive resilient control design strategy spans the considerations from design through implementation stages. In this approach, resilience control minimizes the number of negative inci- dences that shock the system, avoids the negative impacts that results from most of the negative incidents that still occur, minimizes the damage from the adverse impacts of undesirable incidents, and returns the system to normal operation in the shortest time. Resilience specifically models how quickly the industrial control system recov- ers to normal operation following a system breach; whereas robustness, adaptiveness, survivability and fault-tolerance do not. Resilience is thus a superset of all the above properties, which are aspects of resilience (Wei & Ji, 2010). Although the system is designed to be robust against various shocks, it cannot be designed to resist every cyberattack or physical shock. In the absence of resiliency considerations, the ability to return the system to normal may be very costly, and may take a very long time. When the robustness and security fail due to an external incident, resilient control minimizes the adverse impacts by preventing further deterioration and restoring system performance. Zhu and Basar (2011) implement a holistic resilient control approach to cyber physical power generation systems where a single cost function simultaneously balances between resilience and robustness. In that holistic dynamic game approach, the cyber attacker attempts to minimize the overall system performance while the defending controller attempts to maximize the overall system performance. The cyberattack is modelled by a discrete probability process set within a continuous-time dynamic game. Wei and Ji (2010) model resiliency in terms of (1) the superior response to an individual incident, and (2) a set of system failures. System A is more i-resilient than system B with respect to that given incident i if the performance and financial loss that results is less under system A than in system B. Similarly, system A is more resilient than system B if the overall potential financial loss is less under system A than in system B. That study plots a “resilience curve” that plots performance over time. The resilience curve shows the degradation that results after the negative incident, and the return to normal system operation. Note that this can only be defined for systems that have degraded past their robust tolerances due to negative incidents, and are then using the resilience control features to return to normal operation. No literature as of yet measures the level of resiliency for an industrial control system, although there have been proposals to measure resilience performance by buffering capacity, margin, and tolerance (Wei & Ji, 2010). However, these metrics do not illustrate the speed at which the system can recover from the breakdowns (Wei & Ji, 2010). Our approach utilizes aspects of both the analyses in Zhu and Basar (2011) and Wei and Ji (2010). It is similar to Zhu and Basar (2011) in that it seeks to balance the cost of tracking the economic variables at their current post-incident operating state with the cost of returning the variables back to their pre-incident tracking trajectories. It is also similar in that we employ a minimax control design to make the system more robust to anticipated disturbances, which both expands the stress tolerances of 123 1414 D. Hudgins, P. M. Crowley the model, and reduces the performance loss arising from the large negative incidents that occur. This method is similar to Wei and Ji (2010) since it attempts to produce a resilience curve that is characterized by a shorter catastrophic episode with a smaller economic performance loss before more quickly returning close to its normal pre- incident operation. Extreme macroeconomic incidents, however, are not characterized by the constant threat level posed by potential cyberattacks. Thus, we model a resilient approach where policymakers utilize additional control resources following an extreme negative incident. The advantage to this approach is that policymakers can simulate the effects of various sizes of extreme incidents that occur at different times, and then model the costs of the optimal responses required to return the economy near to its original desired state. This allows for planners to allocate resources for dealing with potential incidents, and formulate a plan for how those resources will be deployed throughout the post-event recovery. The specifics of our macroeconomic resilient control method are as follows: (1) Develop a large-scale state-space deterministic control system that optimally tracks the variable target trajectories with certainty. (2) Introduce uncertainty in the system through disturbances that capture model mis- specification and additive errors. Robustness is integrated within this aspect by employing minimax game where the policymakers minimize the variable track- ing errors while nature maximizes the economic disruption. This produces the best possible policy options under the maximum stress tolerance that the system will endure before breaking down. (3) The parameters on the disturbances in the state-space equations and the perfor- mance index can be expanded to include additional entropy to make the system even more robust (with a policy cost increase) to some of the anticipated distur- bances. (4) We compute the minimax policies when the fiscal budget deficit is restricted and compare the deterioration in macroeconomic performance with case where fiscal policy is not restricted. This allows for comparing the benefits more aggressive policy to the costs of the increased government financing. (5) The control system, however, cannot be made so robust that it is impervious to every type of catastrophic shock. Systems that are robust to some sets of disturbances are often more susceptible to breakdowns that arise from different types of shocks. Thus, the system cannot be made so robust a priori that it can be assured that it will never fail. In other words, every control system may encounter large catastrophic shocks that cause the system to periodically fail to operate normally. (6) We introduce a large negative catastrophic incident in the middle of the planning horizon. We then plot resilience curves that simulate the primary economic vari- ables’ trajectories when a resilience plan with fiscal policy measures is employed to counter against the negative incident verses the case where there are no counter measures. This is consistent with the approach of Wei and Ji (2010) that seeks 123 Resilient Control for Macroeconomic Models 1415 to minimize performance loss by enacting a policy design that reduces the dete- rioration in performance and increases the speed at which the variables return to their targets following the incident. (7) We also plot the cost of the resilience counter policy measures across the time horizon following the negative external incident. By using a WBC model, we are able to analyze the desirable spending at each frequency range, which provides policymakers a more politically feasible implementation strategy. A negative external incident could occur at any point in the planning horizon. Our procedure introduces the possibility of such an incident as follows. In the discrete time index, let ζ denote the time of a large negative incident, so that the economy follows a pre-incident trajectory from k 1, …, ζ – 1, and then follows an altered post-incident trajectory from k ζ, …, K. The control vector in Eq. (4) can be augmented by adding a new subset consisting of resilience transitional control variables associated with system recovery, as defined in Eq. (14). Then, the state vector in Eq. (3) can augmented to accommodate the new variables. u R E S : u j,k 0 for k 1,... , ζ−1; u j,k z j,k − z ∗j,k for k ζ,... , K (14) In engineering systems, the most accepted and used implementation principle method of employing control variables to achieve resiliency is redundancy (Wei and Ji (2010). Our approach attempts to integrate this concept of redundancy from engi- neering into macroeconomic applications. In response to a negative external incident, the current economic initiatives can be modified and augmented by the supplemental policies that would be redundant when the economy is operating normally. In order to achieve this, our paper places the additional government fiscal assis- tance into the variable called Counter at each of the five frequency ranges. We have integrated this variable into the wavelet decomposed consumption, investment, and unemployment equations. The basket of control variables available to policymakers can thus be separated into policies that primarily affect one of these three variables. This approach is consistent with the U.S. Treasury, which lists its coronavirus relief categories as follows: assistance for families and workers; assistance for small busi- nesses; assistance for state, local, and tribal governments; and assistance for industry.9 For consumption, these policies would include individual economic impact pay- ments, increased child tax credits, and emergency rental assistance payments. Expanding the earned Income Tax Credit would positively impact both consumption and unemployment by increasing the after-tax wages for recipients and increasing the incentives to work (Edelberg & Sheiner, 2020). For investment and unemployment, these policies include: the American Rescue Plan that extends a number of critical tax benefits, particularly the Employee Retention Credit and Paid Leave Credit, to small businesses; the Emergency Capital Investment Programs that support the efforts of low- and moderate-income community financial institutions; and the Paycheck Protection Program (PPP) that provides small businesses with the resources they need to maintain their payroll, hire back employees who may have been laid off, and cover applicable overhead. 9 See https://home.treasury.gov/policy-issues/coronavirus. 123 1416 D. Hudgins, P. M. Crowley Other policies to aid investment would include the Airline and National Secu- rity Relief Programs, the Coronavirus Economic Relief for Transportation Services (CERTS) Program that provides eligible transportation service companies with resources to help to maintain payroll, hire back employees who may have been laid off, and cover applicable overhead and operational expenses. Additional policies that aid both investment and unemployment, are policies such as the Employee Retention Credit, whereby employers of all sizes that face closure orders or suffer economic hardship were incentivized to keep employees on the payroll through a 50% credit on up to $10,000 of wages paid or incurred from March 13, 2020 through December 31, 2020. This is by no means an exhaustive list of the policies used to complement the normal fiscal policies, but provide a sample of those policies that have been utilized by the U.S. government in response to the negative external incident. The Counter variable in the model is an aggregate variable that includes the fiscal outlays that fund all of the programs utilized to aid in economic resilience. Since the model is time-varying, some of the coefficients in the equations will change after the external incident. For this analysis, we assume that the matrices Ak , Bk , and Dk , in Eq. (6) are time-invariant for the pre-incident period, but that some of the elements change to new constant values for the post-incident period. Using U.S. CBO data and other analyses, Edelberg and Sheiner (2020) and Seliski et al. (2020) have examined the short and long term multipliers associated with the U.S. fiscal resilience initiatives that were enacted in 2021. These studies have pro- duced multiplier estimates that have a wide margin of error for the resilience fiscal response measures on personal spending, business investment, and unemployment. For example, the estimated demand multiplier on national output arising from fiscal policy measures ranges from 0.5 to 2.5. The marginal propensity to consume (MPC) from PPP funds was estimated to range from 0.1 to 0.56 depending on the spending channel used for the funds. For the effects on the rebates to households, Edelberg and Sheiner (2020) use a multiplier of 0.7, which is in center of the estimated range of 0.5 to 0.9. Thus, these studies have explored how the coefficient relationships of the post- incident might change after a major negative external incident. These estimates of impacts of fiscal initiatives depend upon the degree of monetary accommodation. If the monetary authorities adopt a tighter monetary policy, then the multipliers would be expected to be on the lower end of the estimated impact values. In our model, monetary policy is determined by optimal control so that the interest rate and money supply adjust according to feedback policy. We emphasize that the primary contribution of this paper is the computational aspects of designing a WBC model that can be used to simulate the resilience curves for macroeconomic variables under various assumptions. Thus, our model can be used to determine the likely impacts of the policy measures under various scenarios for the parameter changes that result from the negative external incident. 123 Resilient Control for Macroeconomic Models 1417 2.4 Rationale for Wavelet Specification Our approach to macroeconomic resilience control can be utilized in an aggregate macroeconomic state-space model set in the time domain, or it can use wavelet analysis where some of the variables are decomposed using wavelets to explore considerations in the time–frequency domain. The first advantage of using a wavelet-based model is that wavelets can be used to capture cyclical effects that are not captured in purely time-domain methods. For example, Aguiar-Conraria et al. (2020) utilizes wavelets to improve the estimates of the Okun’s Law specification by capturing the time–frequency structure. Aguiar-Conraria et al. (2018) used wavelet to estimate the Taylor Rule, and found evidence for a shift in the policy focus between the short, intermediate, and long cycles across different time periods. When estimating large-scale time-series models, Power et al. (2017) found that wavelet decomposition is generally more effective for estimation and forecasting than is adding more factors to the model. An advantage of utilizing a discrete wavelet-based framework is that the parameter estimates can be updated as new data is obtained, as is the case with standard time series methods and adaptive control. The coefficients of the linearized model can therefore be updated during each period as the wavelet filter is applied to new data as it becomes available. The updated coefficients can be utilized in the feedback control rule in (9) – (13). Another rationale for employing a wavelet analysis is that fiscal policy can be improved by utilizing decomposed variables. Crowley and Hudgins (2015) find that fiscal policy is more active within the WBC model than in the aggregate model with- out decomposition. The consumption levels in the aggregate model were overvalued relative to those in the WBC model, whereas investment levels in the aggregate model were undervalued relative to the WBC model. This led to suboptimal fiscal policy from the aggregate model, while the WBC model was also able improve the projections of different fiscal policy priorities on the national debt. This aspect is especially relevant to the resilient control analysis, since the accuracy of the cost projections of utilizing additional fiscal stimulus is critical to the cost–benefit analysis of the mechanisms utilized to help return the system to normal. One further advantage of using a wavelet-based approach is that the policymaker can place more weight on specific cycles, especially the political cycle, which can simulate results based on the pragmatic implementation of the policymakers’ objectives. Thus, the wavelet model enables the policymakers to compute the optimal mix is for the intensity of spending on short, intermediate, and long-term fiscal spending. Crowley and Hudgins (2015) compare the optimal fiscal policy simulations under short-term stabilization targeting, long-run business cycle targeting, and political cycle targeting. This is a crucial consideration, since economic policy choices provided by the model are likely to be irrelevant if their implementation is not politically feasible. The wavelet specification allows for the economic policy to integrate the political cycle preferences, and thus reflects preferences of policymakers. Wavelet models also have the ability to compute the optimal policies when fiscal and monetary policymakers have different preferences for short-term, long-term, and political cycle targeting. Crowley and Hudgins (2017) use a WBC model using euro 123 1418 D. Hudgins, P. M. Crowley area date and find that the optimal monetary strategy is to allow the short-term interest rate to undulate due to the cyclical decomposition, rather than following a steady growth rate or a series of steady discrete jumps. Thus, wavelet decomposition that targets a mix of time–frequency bands provide insights into the optimal timing and thrust of both fiscal and monetary policy that are not possible under standard aggregate models. Lastly, there has been no research that examines the resilience aspects of wavelet models. Thus, our study is a pioneering effort to examine what contributions wavelet analysis can make to resilience control. 3 Application and Simulations This section applies the resilient and minimax control methods using the large-scale WBC model in Crowley and Hudgins (2021a, 2021b). This discrete WBC model extracts embedded cycles from time series data by decomposing the value of a variable x at time instant k, x k , using Mallat’s pyramid algorithm and multiresolutional analysis, as xk ≈ S J ,k + d J ,k + d J −1,k +... + d1,k (15) where the d j,k terms are wavelet detail “crystals”, j 1,…, J; S J,k is a trend component, called the wavelet “smooth”, and J denotes the frequency bands (number of scales). We apply the asymmetric Daubechies 4-tap (D4) wavelet function, then employ the Maximal Overlap Discrete Wavelet Transform (MODWT) to obtain a time–frequency decomposition over the frequency ranges (j 1,…, 5) shown in Table 1 using U.S. quarterly data from the post-Bretton-Woods period of 1973 quarter 3 to 2018 quarter 2. The wavelet–decomposed output variables include domestic real GDP (Y ), and its components consumption (C), investment (I), government spending, and net exports (NX), foreign GDP (using OECD data). The other decomposed variables are the short- term U.S. interest rate (ir US ), the foreign (G6 G7 minus U.S.) real GDP weighted nominal interest rate (ir f ), the real exchange rate (RER), the unemployment rate (un), Table 1 The time intervals associated with each of the J Time interval in quarters Time interval in years frequency ranges 1 2 to 4 quarters 6 months to 1 year 2 4 – 8 quarters 1 – 2 years 3 8 – 16 quarters 2 – 4 years 4 16 – 32 quarters 4 – 8 years 5 32 – 64 quarters 8 – 16 years 123 Resilient Control for Macroeconomic Models 1419 and resilience aid variable Counter that only enters the equations after a negative external incident.10 C j,k βC, j,0 + f C, j C j,k−1 ,... , C j,k−L C , G ej,k−1 , G j,k−1 ,... , G j,k−L G , R E R j,k−1 ,... , R E R j,k−L R E R , Counter j,k−1 + ωC, j,k−1 (16) I j,k β I j,0 US + f I , j Y j,k−1 ,... , Y j,k−L US C , ir Uj,k−1 S ,..., ir Uj,k−L S irU S , Counter j,k−1 + ω I , j,k−1 (17) N X j,k β N X , j,0 + f N X , j,0 N X j,k−1 ,... , N X j,k−L N X , Y j,k−1 US ,... , Y j,k−L US US , Y f f Y j,k−1 ,... , Y f , R E R j,k−1 ,... , R E R j,k−L R E R + ω N X , j,k−1 (18) j,k−LY f R E R j,k β R E R, j,0 + f R E R, j ir Uj,k−1S ,... , ir Uj,k−L S irU S , ir j,k−1 ,... , f ir j,k−L ir f , R E R j,k−1 ,... , R E R j,k−L R E R + ω R E R, j,k−1 (19) dun, j,k βun,0 + βun,1 d y, j,k + βun,2 Counter j,k + wun, j,k (20) The wavelet-decomposed variables are determined by the linearized difference Eqs. (16) to (20), where the β0 coefficients denote constants. Consumption at each frequency range is a function of a constant, its own lags, as well as government spending, expected government spending, the RER, and Counter. Investment at each frequency range is a function of lagged GDP, the interest rate, and Counter. Net exports at each frequency range depend upon lagged net exports, domestic GDP, foreign GDP, and the RER. The RER at each frequency range is determined based on interest rate parity influences from domestic and foreign interest rates, as well as the lagged RER. The explicit forms and estimates for Eqs. (16)–(19) is provided in appendix Tables A1–A5 in Crowley and Hudgins (2019). The unemployment rate at each frequency range is modeled by Eq. (20). Using the method and estimates from Crowley and Hudgins (2021b), unemployment is deter- mined by Okun’s Law (OL) where the unemployment rate adjusts to the momentum in national output. Then, using Eq. (15), the smooth trend is added back to the sum of the detailed crystals in order to compute the aggregate unemployment rate for each quarter. Unemployment also adjusts to the Counter fiscal resilience policies. in f k βin f ,0 + bin f ,1 in f j,k −1 + bin f ,2 (Yk −1 −Yk∗−1 ) + bin f ,3 R E Rk −1 (21) +βin f ,4 M Sk −1 + βin f ,5 in f k −2 + win f ,k−1 10 G6 interest rates are sourced from the OECD and US rates are sourced from the Federal Reserve. The G6 rates use real GDP in US$ weights sourced from either the IMF or OECD. The U.S. quarterly national income data are chain-weighted, seasonally adjusted and in 2012 prices, sourced from http://www.bea.gov. 123 1420 D. Hudgins, P. M. Crowley M Sk −in f k β M S,0 + b M S,1 (irk−1 US −in f k −1 ) ∗ + β M S,2 (Yk −1 −Yk −1 ) + β M S,3 (M Sk−1 −in f k −1 ) + β M S,4 (M S M Sk−2 −in f k −2 ) + win f , k−1 (22) The aggregate inflation and money supply variables are determined in Eqs. (21) and (22), respectively. Aggregate inflation is an accelerator function of a constant, the lags of inflation, the national output gap, money supply growth (MS), and the RER. When output exceeds its full-employment level, it leads to increases in inflation. Increased money growth also causes upward pressure on inflation. In Eq. (22), real money growth is a function of a constant, lagged real money growth, the real interest rate, and the national output gap. As in Crowley and Hudgins (2019, 2021a), this equation expresses the adjustments of money demand and supply to maintain equilibrium in the asset market. The estimates for Eqs. (21) and (22) are taken from Crowley and Hudgins (2019) appendix Tables A6 and A7. Equations (23) – (26) close the model by specifying aggregate time-domain equa- tions for the national income identity, net taxes (T ), the quarterly budget deficit (DEF), and the national debt stock.11 Yk Ck + Ik + G k + N X k (23) Tk τ Yk (24) D E Fk G k − Tk (25) D E BTk 0.25D E Fk + (1 + i k )D E BTk−1 (26) In this framework, there is a single performance index for jointly determining fiscal and monetary closed-loop feedback policy through optimal tracking control. The numerical values for all of the penalty weights for the tracking errors in the quadratic performance index are given in the appendix.12 Fiscal policymakers determine government spending at each frequency range, where net taxes are passively determined as percentage of national output, and policy- makers are also penalized for both budget deficits and for large changes in spending in adjacent quarters. The central bank optimally determines the short-term interest rate at each frequency range, where both the interest tracking errors and changes in the interest rate in adjacent quarters are penalized. There are penalty weights on the money growth, inflation, and national output tracking errors, so the central bank can choose to focus on monetary, economic growth, or inflation targets by selecting a large value for the penalty weight on any given objective. Crowley and Hudgins (2021b) found that 11 Since the variables are measured as annualized values of quarterly data, Eq. (26) multiples the annualized quarterly deficit by 0.25 to obtain the quarterly deficit value. Then, the new national debt in any quarter is calculated by adding this quarterly deficit to the previous debt plus interest. 12 The dimensions of the matrices and vectors in Eqs. (5) and (6) are as follows: dim Q (n, n) (138, f 138); dim Q k (n, n) (138, 138); dim Rk (m, m) (15, 15); dim A (n, n) (138, 138); dim B (n, m) (138, 15); dim D (n, p) (138, 15); dim x (n, 1) (138, 1); dim u (m, 1) (15, 1); dim w (p, 1) (15, 1). 123 Resilient Control for Macroeconomic Models 1421 fiscal policy was more aggressive when economic growth was relatively prioritized, whereas monetary policy was relatively more aggressive when the inflation rate was emphasized. The simulations set the initial variable values based on the data in 2018 quarter 2. We assume political cycle weighting where the targets at frequency ranges 3 and 4 (from 2 to 8 years) are most heavily prioritized. Government spending and the interest rate are chosen starting in quarter 1 and ending in quarter 16. These choices influence the state variables starting in quarter 2 and ending in quarter 17. Thus, the forecast planning horizon spans a 4-year political cycle. The simulations show the optimal forecasts under a deterministic design where there are no external disturbances, and also for the minimax design with the worst-case disturbances. We then consider the minimax design case where a large negative external incident, or attack, occurs at the end of quarter 7, so that the system parameters and actual variable values are affected in quarter 8 (so that the post-incident time is ζ 8). This roughly coincides with the negative external economic incident associated with the government lockdowns that were attributed to the coronavirus at the end of quarter 1 and during quarter 2 of 2020. This is the first macroeconomic analysis to model such an economic crisis from a resilient control perspective. Since some of the initial projections in April 2020 forecast the unemployment rate to increase to more than 10%, and quarterly national output to decrease by 10%, we have assumed that the external incident affected the unemployment rate and GDP in amounts consistent with these projections.13 It should be emphasized that this analysis is not attempting to model this specific episode; instead, the purpose is show how a WBC resilient control would affect economic policy under a large external incident that is roughly similar in magnitude. Thus, our model framework is more general than any particular incident, but the simulations are specific enough to make some conclusions regarding this type of event. The target unemployment rate is 4.5% and the inflation rate target is 2%. Given the real GDP growth target of 2.5%, this results in a 4.5% annual nominal GDP growth target. Based on the equation of exchange, this suggests a 4.5% money growth target. Given the 2% real interest rate target, the target nominal interest rate is ir US * 4%.14 Since the initial nominal interest rate was only 2%, the unrestricted simulations specify approximate the Fed’s “liftoff” strategy, as in Crowley and Hudgins (2018), where the target annual interest rate steadily increases from 2 to 4% over the horizon.15 After the incident occurs in quarter 7, the interest rate target was reset at 1% for quarter 8, where the target growth rate was maintained.16 Figures 1, 2 show the following 5 cases: Case (1): deterministic forecast, no external incident. 13 See the U.S. CBO macroeconomic variable projections in the documents for April, 2020, available at https://www.cbo.gov/publication/56314 and https://www.cbo.gov/publication/56335. 14 This balances a real interest rate of 2% with a productivity growth of 2%. For an annual population growth of 0.5%, this is consistent with an annual real GDP target growth of 2.5%. 15 The target interest rate thus grows at a quarterly compounded growth rate of 0.04729. This approximates an interest rate response in the short-term bond market to series of eight semi-annual Fed discount rate increases by 25 basis points over the four-year horizon. 16 This 1% target reflects the average of the 3-month T-Bill rates for February and March 2020. During the 2-year period from March 2016 to early March 2018, this interest rate had been steadily increasing at 123 1422 D. Hudgins, P. M. Crowley (a) Case (1): deterministic forecast, no external attack (c) Case (3): minimax worst-case forecast with fiscal restrictions, no external attack irUS* Money Growth k inflation Nominal irUS Real irUS irUS* Money Growth k inflation Nominal irUS Real irUS 6.00 7.00 Interest Rates, Inflation, Money Growth, in percent Interest Rates, Inflation, Money Growth, in percent 6.00 5.00 5.00 4.00 4.00 3.00 3.00 2.00 2.00 1.00 0.00 1.00 -1.00 0.00 -2.00 0 1 2 3 4 5 6 7 8 9 10 11 12 13 14 15 16 17 0 1 2 3 4 5 6 7 8 9 10 11 12 13 14 15 16 17 k (quarter) k (quarter) (b) Case (2): minimax worst-case forecast without fiscal restrictions, no external attack (d) Case (4): minimax worst-case forecast without fiscal restrictions, external attack with nocounter response irUS* Money Growth k inflation Nominal irUS Real irUS irUS* Money Growth k inflation Nominal irUS Real irUS 7.00 Interest Rates, Inflation, Money Growth, in percent 8.00 6.00 Interest Rates, Inflation, Money Growth, in percent 7.00 5.00 6.00 4.00 5.00 3.00 4.00 3.00 2.00 2.00 1.00 1.00 0.00 0.00 -1.00 -1.00 -2.00 -2.00 -3.00 0 1 2 3 4 5 6 7 8 9 10 11 12 13 14 15 16 17 0 1 2 3 4 5 6 7 8 9 10 11 12 13 14 15 16 17 k (quarter) k (quarter) (e) Case (5): minimax worst-case forecast without fiscal restrictions, external attack with counter response irUS* Money Growth k inflation Nominal irUS Real irUS 8.00 Interest Rates, Inflation, Money Growth, in percent 7.00 6.00 5.00 4.00 3.00 2.00 1.00 0.00 -1.00 -2.00 -3.00 0 1 2 3 4 5 6 7 8 9 10 11 12 13 14 15 16 17 k (quarter) Fig. 1 Short-term U.S. Nominal Interest Rate Target (irUS *), U.S. Nominal Market Interest Rate (irUS ), Real Interest Rate, Inflation (inf ), Money Growth (MS) a Case (1): deterministic forecast, no external attack b Case (2): minimax worst-case forecast without fiscal restrictions, no external attack c Case (3): minimax worst-case forecast with fiscal restrictions, no external attack d Case (4): minimax worst-case forecast without fiscal restrictions, external attack with no counter response e Case (5): minimax worst-case forecast without fiscal restrictions, external attack with counter response Case (2): minimax worst-case forecast with small fiscal deficit restrictions, no external incident. The small penalty weight on the quadratic budget deficit tracking error (DEF) 2 is only 0.2. Case (3): minimax worst-case forecast with fiscal deficit restrictions, no external inci- dent. The large penalty weight on the quadratic budget deficit tracking error (DEF) 2 is 2,000, which is 10,000 times larger than in Case (2). Case (4): minimax worst-case forecast without fiscal restrictions, external incident with no countering policy actions Case (5): minimax worst-case forecast without fiscal restrictions, external incident with countering policy actions In case (1), the disturbances are set to 0. This scenario reflects the optimal policy trajectories when policymakers focus on the historically low unemployment rate, and assume that the economy is strong and could experience inflation. Thus, monetary policy is relatively tight in Fig. 1a, where the nominal interest rate initially increases Footnote 16 continued rate that had fallen short of the liftoff target, as was forecast by the WBC model in Crowley and Hudgins (2018). For the U.S. interest rate data, see https://fred.stlouisfed.org/series/TB3MS. 123 Resilient Control for Macroeconomic Models 1423 13 (a) Unemployment Rate (un) Optimal Forecasts 10000 (c) Government Spending (G) Optimal Forecasts G (Government Spending: billions of constant $) 12 9000 11 un (unemployment rate in %) 10 8000 9 7000 8 un* G* 6000 7 un (Case 1) G (Case 1) 6 un (Case 2) 5000 G (Case 2) 5 un (Case 3) 4000 G (Case 3) 4 un (Case 4) 3000 G (Case 4) 3 2 un (Case 5) 2000 G (Case 5) 1 1000 0 0 1 2 3 4 5 6 7 8 9 10 11 12 13 14 15 16 17 0 1 2 3 4 5 6 7 8 9 10 11 12 13 14 15 16 k (quarter) k (quarter) 5000 (b) Investment (I) Optimal Forecasts (d) Consumption (C) Optimal Forecasts 18000 I (Investment in billions of constant $) 4500 C (Consumption, in billions of constant $) 17000 4000 16000 3500 I* 15000 3000