Raw Score and Hypothesis Testing Review PDF
Document Details
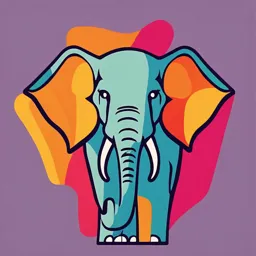
Uploaded by WieldyMandolin2016
Tags
Summary
This document provides a review of raw scores and hypothesis testing, covering concepts like z-scores, probability, and effect size. It explains how raw scores are used as a basis for statistical analysis and details methods for transforming raw data.
Full Transcript
Tab 1 Raw Score - A raw score in psychological statistics refers to the original, unprocessed score obtained from a test or assessment. It reflects an individual's performance without any modifications or transformations. - Raw scores are critical as they serve as the s...
Tab 1 Raw Score - A raw score in psychological statistics refers to the original, unprocessed score obtained from a test or assessment. It reflects an individual's performance without any modifications or transformations. - Raw scores are critical as they serve as the starting point for more complex statistical analyses. - While raw scores provide straightforward numerical values, they often lack context. For example, a raw score of 75 on one test may not be directly comparable to a raw score of 75 on another test if the tests differ in difficulty or scoring methods. Therefore, raw scores are sometimes transformed into standardized scores (like z-scores) to facilitate meaningful comparisons. Z score - a score by itself does not necessarily provide much information about its position within a distribution. - These original, unchanged scores that are the direct result of measurement are called raw scores. - To make raw scores more meaningful, they are often transformed into new values that contain more information. - This transformation is one purpose for z-scores. In particular, we transform X values into 2z-scores so that the resulting z-scores tell exactly where the original scores are located. The process of transforming X values into z-scores serves two useful purposes: 1. Each z-score tells the exact location of the original X value within the distribution. 2. The z-scores form a standardized distribution that can be directly compared to other distributions that also have been transformed into z-scores. - Mean Score: The average IQ score is set at 100. This is the peak of the bell curve, where most individuals' scores cluster. - Standard Deviation: In IQ testing, a standard deviation (SD) is typically 15 points. - Approximately 68% of the population scores between 85 and 115 (within one SD of the mean). - About 95% score between 70 and 130 (within two SDs of the mean). - Only about 2% score below 70 or above 130, representing extreme ends of intelligence. One of the primary purposes of a z-score is to describe the exact location of a score within a distribution. The z-score accomplishes this goal by transforming each X value into a signed number (+ or −). 1. the sign tells whether the score is located above (+) or below (−) the mean, and 2. the number tells the distance between the score and the mean in terms of the number of standard deviations. The new distribution of z-scores has characteristics that make the z-score transformation a very useful tool. Specifically, if every X value is transformed into a z-score, then the distribution of z-scores will have the following properties: Shape - The distribution of z-scores will have exactly the same shape as the original distribution of scores. If the original distribution is negatively skewed, for example, then the z-score distribution will also be negatively skewed. If the original distribution is normal, the distribution of z-scores will also be normal. - Transforming raw scores into z-scores does not change anyone’s position in the distribution. - The z-score distribution will always have a mean of zero. Z score Formula - This part of the formula is called as DEVIATION SCORE. - It measures the distance in points between X and μ and indicates whether X is located above or below the mean. - The deviation score is then divided by σ because we want the z-score to measure distance in terms of standard deviation units. Sample A distribution of scores has a mean of μ = 100 and a standard deviation of σ = 10. What z-score corresponds to a score of X = 130 in this distribution? For a distribution with a mean of μ = 60 and σ = 8, what X value corresponds to a z-score of z = −1.50? Probability - For a situation in which several different outcomes are possible, the probability for any specific outcome is defined as a fraction or a proportion of all the possible outcomes. If the possible outcomes are identified as A, B, C, D, and so on, Types of Probability - Classical Probability: Based on the assumption of equally likely outcomes. For example, when tossing a fair coin, the probability of getting heads is 1/2. - Empirical Probability: Based on observed data rather than theoretical assumptions. It is calculated by conducting experiments and recording outcomes. - Subjective Probability: Based on personal judgment or estimation rather than exact calculations. - Axiomatic Probability: Built on a set of axioms or foundational principles that define how probabilities are assigned to events. Foundation of Probability - Making Predictions: Statistics uses probability to make inferences about populations based on sample data. This involves estimating population parameters, testing hypotheses, and constructing confidence intervals, all of which rely on probability distributions to assess the likelihood of various outcomes. - Analyzing Random Events: Probability focuses on the likelihood of future events occurring, while statistics is concerned with analyzing data collected from past events. Probability helps in predicting outcomes, whereas statistics interprets the data to draw conclusions about those predictions. Random Sampling Our definition of probability is accurate only for random samples. There are two requirements that must be satisfied for a random sample: a. Every individual in the population has an equal chance of being selected. b. When more than one individual is being selected, the probabilities must stay constant. This means there must be sampling with replacement. Probability - Percentiles and percentile ranks measure the relative standing of a score within a distribution. - The percentile rank is the percentage of individuals with scores at or below a particular X value. - A percentile is an X value that is identified by its rank. The percentile rank always corresponds to the proportion to the left of the score in question. Hypothesis testing A hypothesis test is a statistical method that uses sample data to evaluate a hypothesis about a population. - First, we state a hypothesis about a population. Usually the hypothesis concerns the value of a population parameter. - Before we select a sample, we use the hypothesis to predict the characteristics that the sample should have. - Next, we obtain a random sample from the population. - Finally, we compare the obtained sample data with the prediction that was made from the hypothesis. If the sample mean is consistent with the prediction, we conclude that the hypothesis is reasonable. But if there is a big discrepancy between the data and the prediction, we decide that the hypothesis is wrong. - The goal of the hypothesis test is to determine whether the treatment has any effect on the individuals in the population. Steps in Hypothesis Testing: 1. State the Hypotheesis - The first and most important of the two hypotheses is called the null hypothesis. The null hypothesis states that the treatment has no effect. - The null hypothesis is identified by the symbol H0. (The H stands for hypothesis, and the zero subscript indicates that this is the zero-effect hypothesis.) - The alternative hypothesis (H1) states that there is a change, a difference, or a relationship for the general population. In the context of an experiment, H1 predicts that the independent variable (treatment) does have an effect on the dependent variable. 2. Setting Criteria for Decision - Eventually the researcher will use the data from the sample to evaluate the credibility of the null hypothesis. The data will either provide support for the null hypothesis or tend to refute the null hypothesis. - In particular, if there is a big discrepancy between the data and the hypothesis, we will conclude that the hypothesis is wrong. EXAMPLE For our example, the null hypothesis states that the red shirts have no effect and the population mean is still μ = 15.8 percent, the same as the population mean for waitresses wearing white shirts. If this is true, then the sample mean should have a value of around 15.8. Therefore, a sample mean near 15.8 is consistent with the null hypothesis. On the other hand, a sample mean that is very different from 15.8 is not consistent with the null hypothesis. 3. The Alpha Level - The alpha level (or significance level) is a crucial concept in hypothesis testing, representing the probability of making a Type I error, which occurs when the null hypothesis is incorrectly rejected while it is actually true. This probability is denoted by α. - Set by common values such as 0.05 (5%), 0.01 (1%), or 0.10 (10%) depending on the context of the test and the acceptable risk of error - An alpha level of 0.05 indicates a 5% risk of committing a Type I error, meaning there is a 5% chance of rejecting the null hypothesis when it is actually true. - This level is often considered acceptable in many research contexts, providing a reasonable trade-off between sensitivity (detecting true effects) and specificity (avoiding false positives) - The choice of 0.05 corresponds to a confidence interval of approximately 95%. This means researchers can be confident that if they were to repeat the study multiple times, 95% of the calculated confidence intervals would contain the true population parameter. This level of confidence is generally deemed sufficient for many scientific inquiries. 4. Critical Region - The critical region (also known as the rejection region) is the set of values for the test statistic that would lead to rejecting the null hypothesis. - If the calculated test statistic falls within this region, it indicates that the observed data is sufficiently extreme under the null hypothesis, prompting rejection of the null hypothesis - For example, if you set an alpha level of 0.05, this means that 5% of the distribution will be in the critical region. This corresponds to extreme values in either the tail of the distribution for two-tailed tests or one tail for one-tailed tests. Type of Errors - A Type I error occurs when a researcher rejects a null hypothesis that is actually true. In a typical research situation, a Type I error means the researcher vconcludes that a treatment does have an effect when in fact it has no effect. - A Type II error occurs when a researcher fails to reject a null hypothesis that is really false. In a typical research situation, a Type II error means that the hypothesis test has failed to detect a real treatment effect. Hypothesis for Directional Test - A directional test, also known as a one-tailed test, is a type of hypothesis test where the researcher specifies the expected direction of the effect or relationship between variables. - For example, it may state that one variable is greater than or less than another variable, indicating a clear expected outcome (e.g.,"As sleep deprivation increases, cognitive performance decreases"). - Directional tests are referred to as one-tailed tests because they focus on one tail of the distribution. This means that the critical region for rejecting the null hypothesis is located entirely in one direction (either positive or negative). EXAMPLE In psychological research, a directional hypothesis might state: "Participants who receive cognitive training will perform better on memory tasks than those who do not receive training. " This clearly indicates that the expectation is for improvement in performance due to training”. Type of Directional Test One-Tailed Test: This test evaluates whether a parameter is either greater than or less than a certain value, focusing on one direction. It can be classified as: - Left-Tailed Test: Tests if the parameter is less than a specified value. - Right-Tailed Test: Tests if the parameter is greater than a specified value Two-Tailed Test: This test assesses whether a parameter is significantly different from a specified value, without specifying a direction. It checks for both increases and decreases, making it more general. - Critical ERegion. - One-Tailed Test: Has one critical region where the entire alpha level (e.g., 0.05) is allocated. This means that all statistical power is focused in one tail of the distribution, making it easier to detect an effect in that direction. - Two-Tailed Test: Contains two critical regions, each receiving half of the alpha level (e.g., 0.025 in each tail for an alpha of 0.05). This requires more extreme results to achieve significance, as the critical values are split between both tails. - Proper Usage ' - One-Tailed Test: Appropriate when there is a strong theoretical basis or prior evidence suggesting that an effect will occur in one direction. For example, if a new drug is expected to improve recovery times compared to a placebo, a right-tailed test would be suitable. - Two-Tailed Test: Suitable when researchers want to detect any significant difference, regardless of direction. This is common in exploratory research where any change (increase or decrease) is of interest, such as testing whether a new teaching method affects student performance compared to traditional methods. Effect Size - Effect Size: Effect size is a numerical value that expresses the strength of the relationship between two variables or the size of the difference between groups. - A large effect size means that a research finding has practical significance, while a small effect size indicates limited practical applications - Cohen's d: Measures the standardized difference between two means. - Where X1 and Xˉ2 are the sample means, and ss is the pooled standard deviation. Cohen's d values are interpreted as small (0.2), medium (0.5), or large (0.8) effects.