PSCI 2702 Chapter 5 PDF
Document Details
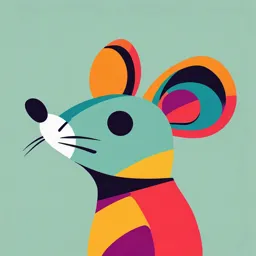
Uploaded by ConscientiousEvergreenForest1127
Tags
Summary
This chapter provides an introduction to inferential statistics, focusing on sampling and sampling distributions. It explains how social science researchers use samples to learn about larger populations and discusses various sampling procedures.
Full Transcript
Book Title: eTextbook: Statistics: A Tool for Social Research and Data Analysis, Fifth Canadian Edition Chapter 5. Introduction to Inferential Statistics: Sampling and the Sampling Distribution 5.1. Introduction 5.1. Introduction One of the goals of social science research is to tes...
Book Title: eTextbook: Statistics: A Tool for Social Research and Data Analysis, Fifth Canadian Edition Chapter 5. Introduction to Inferential Statistics: Sampling and the Sampling Distribution 5.1. Introduction 5.1. Introduction One of the goals of social science research is to test our theories and hypotheses using many different populations of people, groups, societies, and historical eras. Obviously, we can have the greatest confidence in theories that have stood up to testing against the greatest variety of populations and social settings. A major problem we often face in social science research, however, is that the populations we are interested in are too large to test. For example, a theory concerning political party preference among Canadian voters would most suitably be tested using the entire electorate, but it is impossible to interview every member of this group (more than 27 million people in 2021). Indeed, even for theories that could reasonably be tested with smaller populations—such as a local community or the student body at a university— the logistics of gathering data from every single case (entire populations) are staggering to contemplate. If it is too difficult or expensive to do research with entire populations, how can we reasonably test our theories? To deal with this problem, social scientists select samples, or subsets of cases, from the populations of interest. Our goal in inferential statistics is to learn about the characteristics of a population (often called parameters), based on what we can learn from our samples. Two applications of inferential statistics are covered in this textbook. In estimation procedures, covered in Chapter 6, a “guess” of the population parameter is made based on what is known about the sample. In hypothesis testing, covered in Chapters 7, 8, 9, 10, 11, 12, and 13, the validity of a hypothesis about the population is tested against sample outcomes. In this chapter, we look at the theoretical foundations of these inferential statistics. We begin by looking at the techniques used to select cases for a sample and then turn our attention to 153 one of the most important concepts in inferential statistics: the sampling distribution. Book Title: eTextbook: Statistics: A Tool for Social Research and Data Analysis, Fifth Canadian Edition Chapter 5. Introduction to Inferential Statistics: Sampling and the Sampling Distribution 5.2. Probability Sampling 5.2. Probability Sampling In this chapter, we review the basic procedures for selecting probability samples, the only type of sample that fully supports the use of inferential statistical techniques to generalize to populations. These types of samples are often described as “random,” and you may be more familiar with this terminology. Because of its greater familiarity, we will often use the term random sample in the following chapters. The term probability sample is preferred, however, because in everyday language, random is often used to mean “by coincidence” or to give a connotation of unpredictability. As you will see, probability samples are selected by techniques that are careful and methodical and leave no room for haphazardness. Interviewing the people you happen to meet in a mall one afternoon may be “random” in some sense, but this technique will not result in a sample that could support inferential statistics. (In other words, if your sample is not a probability sample, you are limited to descriptive statistical techniques.) Before considering probability sampling, let us point out that social scientists often use non-probability samples. For example, social scientists studying small-group dynamics or the structure of attitudes or personal values might 154 use the students enrolled in their classes as subjects. Such convenience samples are very useful for several purposes (e.g., exploring ideas or pretesting survey forms before embarking on a more ambitious project) and are typically less costly and easier to assemble. The major limitation of these samples is that results cannot be generalized beyond the group being tested. If a theory of ageism (prejudice, discrimination, and stereotyping of older people and old age), for example, has been tested only on the students who happen to be enrolled in a particular section of an Introduction to Gerontology course (i.e., a course on the aging process) at a particular university, we cannot conclude that the theory is true for other types of people. Therefore, even when the evidence is very strong, we cannot place a lot of confidence in theories tested on non- probability samples only. blvdone/Shutterstock.com Probability samples use inferential statistical techniques to generalize to populations. The goal of probability sampling is to select cases so that the final sample is representative of the population from which it was drawn. A sample is representative if it reproduces the important characteristics of the population. For example, if the population consists of 60% females, 38% males, and 2% other genders, the sample should contain essentially the same proportions. In other words, a representative sample is very much like the population—only smaller. It is crucial for inferential statistics that samples be representative; if they are not, generalizing to the population becomes, at best, extremely hazardous. How can we assure ourselves that our samples are representative? Unfortunately, it is not possible to guarantee that our samples will meet this crucial criterion. However, we can maximize the chances of a representative sample by following the principle of EPSEM (“Equal Probability of SElection Method”), the fundamental principle of probability sampling. To follow the EPSEM principle, we select the sample so that every element or case in the population has an equal probability of being selected. Our goal is to select a representative sample, and the technique we use to achieve it is to follow the rule of EPSEM. The most basic EPSEM sampling technique produces a simple random sample. There are variations and refinements on this technique, such as the systematic, stratified, and cluster sampling techniques, which are covered in a supplemental chapter which can be found on the student companion website at www.cengage.com/healey5ce. Here we will consider only simple random sampling. To draw a simple random sample, we need a list of all elements or cases in the population and a system for selecting cases from the list that guarantees that every case has an equal chance of being selected for the sample. The selection process could be based on several different kinds of operations, such as drawing cards from a well-shuffled deck, flipping coins, throwing dice, or drawing numbers from a hat; however, cases are often selected using tables of random numbers. These tables are lists of numbers that have no pattern to them (i.e., they are random). An example of such a table is available on the website for this textbook. 155 To use the table of random numbers, first assign each case on the population list a unique identification number. Then, select cases for the sample when their identification number corresponds to the number chosen from the table. This procedure produces an EPSEM sample because the numbers in the table are in random order and any number is just as likely to be chosen as any other number. Stop selecting cases when you have reached your desired sample size, and, if an identification number is selected more than once, ignore the repeats. Remember that the EPSEM selection technique and the representativeness of the final sample are two different things. In other words, the fact that a sample is selected according to EPSEM does not guarantee that the sample will be an exact representation or microcosm of the population. The probability is very high that an EPSEM sample will be representative, but just as a perfectly honest coin sometimes shows 10 heads in a row when flipped, an EPSEM sample occasionally presents an inaccurate picture of the population. One of the great strengths of inferential statistics is that they allow the researcher to estimate the probability of this type of error and interpret results accordingly. To summarize, the purpose of inferential statistics is to acquire knowledge about populations based on information derived from samples of that population. Each of the statistics to be presented in the following chapters requires that samples be selected according to EPSEM. While EPSEM sampling techniques, of which simple random sampling is the most basic form, do not guarantee representativeness, the probability is high that EPSEM samples are representative of the populations from which they are selected. Book Title: eTextbook: Statistics: A Tool for Social Research and Data Analysis, Fifth Canadian Edition 5.3. The Sampling Distribution 5.3. The Sampling Distribution Once we have selected a probability sample, what do we know? On the one hand, we can gather a great deal of information from the cases in the sample. On the other hand, we know nothing about the population. Indeed, if we had information about the population, we probably would not need the sample. Remember that we use inferential statistics to learn more about populations, and information from the sample is important primarily insofar as it allows us to generalize to the population. When we use inferential statistics, we generally measure some variable (e.g., age, political party preference, or opinions about climate change) in the sample and then use the information from the sample to learn more about that 156 variable in the population. In Part 1 of this textbook, you learned that three types of information are generally necessary to adequately characterize a variable: (1) the shape of its distribution, (2) some measure of central tendency, and (3) some measure of dispersion. Clearly, all three kinds of information can be gathered (or computed) on a variable from the cases in the sample. Just as clearly, none of the information is available for the population. Except in rare situations (e.g., IQ and height are thought to be approximately normal in distribution), nothing can be known about the exact shape of the distribution of a variable in the population. The means and standard deviations of variables in the population are also unknown. As a reminder, if we had this information for the population, inferential statistics would be unnecessary. In statistics, we link information from the sample to the population with a device known as the sampling distribution, the theoretical, probabilistic distribution of a statistic for all possible samples of a certain sample size (n). That is, the sampling distribution includes statistics that represent every conceivable combination of cases (i.e., every possible sample) from the population. A crucial point about the sampling distribution is that its characteristics are based on the laws of probability, not on empirical information, and are very well known. In fact, the sampling distribution is the central concept in inferential statistics, and a prolonged examination of its characteristics is certainly in order. As illustrated by Figure 5.1, the general strategy of all applications of inferential statistics is to move between the sample and the population via the sampling distribution. Thus, three separate and distinct distributions are involved in every application of inferential statistics: 1. The sample distribution is empirical (i.e., it exists in reality) and known in the sense that the shape, central tendency, and dispersion of any variable can be ascertained for the sample. Remember that the information from the sample is important primarily insofar as it allows the researcher to learn about the population. 2. The population distribution is empirical but unknown. Amassing information or making inferences about the population is the sole purpose of inferential statistics. 157 3. The sampling distribution is non-empirical, or theoretical. Because of the laws of probability, a great deal is known about this distribution. Specifically, the shape, central tendency, and dispersion of the distribution can be deduced, and, therefore, the distribution can be adequately characterized. Figure 5.1 The Relationship between the Sample, the Sampling Distribution, and the Population The utility of the sampling distribution is implied by its definition. Because it encompasses all possible sample outcomes, the sampling distribution enables us to estimate the probability of any particular sample outcome, a process that will occupy our attention for the next five chapters. The sampling distribution is theoretical, which means it is obtained hypothetically but not in practice. However, to better understand the structure and function of the distribution, let’s consider an example of how one might be constructed. Suppose we want to gather some information about the age of a particular community of 10,000 individuals. We draw an EPSEM sample of 100 residents, ask all 100 respondents their age, and use those individual scores to compute a mean age of 27. This score is noted on the graph in Figure 5.2. Note that this sample is one of a nearly infinite number of possible combinations of 100 people taken from this population of 10,000 and that the mean of 27 is one of millions of possible sample outcomes. Figure 5.2 Constructing a Sampling Distribution Now, replace the 100 respondents in the first sample, draw another sample of the same size (n = 100) , and again compute the average age. Assume that the mean for the second sample is 30 , and note this sample outcome on Figure 5.2. This second sample is another of the possible combinations of 100 people taken from this population of 10,000 , and the sample mean of 30 is another of the possible sample outcomes. Replace the respondents from the second sample and draw still another sample. Calculate and note the mean, replace this third sample, and draw a fourth sample, continuing these operations an infinite number of times, calculating and noting the mean of each sample. Now, try to imagine what Figure 5.2 would look like after tens of thousands of individual samples had been collected and the mean had been computed for each sample. What shape, mean, and standard deviation would this distribution of sample means have after we had collected all possible combinations of 100 respondents from the population of 10,000 ? 158 For one thing, we know that each sample will be at least slightly different from every other sample, because it is very unlikely to sample exactly the same 100 people twice. Because each sample is almost certainly a unique combination of individuals, each sample mean will be at least slightly different in value. We also know that even though the samples are chosen according to EPSEM, they will not be representative of the population in every single case. For example, if we continue taking samples of 100 people long enough, we will eventually choose a sample that includes only the very youngest residents. Such a sample would have a mean much lower than the true population mean. Likewise, some of our samples will include only older adults and will have means that are much higher than the population mean. Common sense suggests, however, that such non-representative samples are rare and that most sample means will cluster around the true population value. To illustrate further, assume that we somehow come to know that the true mean age of the population is 30. As we have seen above, most of the sample means will also be approximately 30 and the sampling distribution of these sample means should peak at 30. Some of the sample means will “miss the mark,” but the frequency of such misses should decline as we get farther away from 30. That is, the distribution should slope to the base as we get farther away from the population value—sample means of 29 or 31 should be common; means of 20 or 40 should be rare. Because the samples are random, the means should miss an equal number of times on either side of the population value, and the distribution itself should therefore be roughly symmetrical. In other words, the sampling distribution of all possible sample means should be approximately normal and resemble the distribution presented in Figure 5.3. Recall from Chapter 4 that, on any normal curve, cases close to the mean (say, within ±1 standard deviation) are common, and cases far from the mean (say, beyond ±3 standard deviations) are rare. Figure 5.3 A Sampling Distribution of Sample Means These common-sense notions about the shape of the sampling distribution and other very important information about central tendency and dispersion are 159 stated in two theorems. The first of these theorems is as follows: If repeated random samples of size n are drawn from a normal population with mean μ and standard deviation σ , then the sampling distribution of sample means will be normal, with mean μ and standard deviation σ/√n. To translate: If we begin with a trait that is normally distributed across a population (IQ, height, or weight, for example) and take an infinite number of equally sized random samples from that population, then the sampling distribution of sample means will be normal. If it is known that the variable is distributed normally in the population, it can be assumed that the sampling distribution will be normal. The theorem tells us more than the shape of the sampling distribution of all possible sample means, however. It also defines its mean and standard deviation. In fact, it says that the mean of the sampling distribution is exactly the same value as the mean of the population. That is, if we know that the mean IQ of the entire population is 100 , then we know that the mean of any sampling distribution of sample mean IQs is also 100. Exactly why this should be so is explained and demonstrated in Section 5.4. Recall for now, however, that most sample means will cluster around the population value over the long run. Thus, the fact that these two values are equal should have intuitive appeal. As for dispersion, the theorem says that the standard deviation of the sampling distribution, also called the standard error, is equal to the standard deviation of the population divided by the square root of n (symbolically: σ/√n ). If the mean and standard deviation of a normally distributed population are known, the theorem allows us to compute the mean and standard deviation of the sampling distribution. Thus, we know exactly as much about the sampling distribution (shape, central tendency, and dispersion) as we ever knew about any empirical distribution. In the typical research situation, the values of the population mean and standard deviation are, of course, unknown. However, these values can nevertheless be estimated from sample statistics, as we shall see in the chapters that follow. The first theorem requires a normal population distribution. What happens when the distribution of the variable in question is unknown or is known to not be normal in shape (such as income, which typically has a positive skew)? These eventualities (very common, in fact) are covered by a second theorem, called the Central Limit Theorem: If repeated random samples of size n are drawn from any population with mean μ and standard deviation σ , then, as n becomes large, the sampling distribution of sample means will approach normality, with mean μ and standard deviation σ/√n. 160 To translate: For any trait or variable, even those that are not normally distributed in the population, as the sample size grows larger, the sampling distribution of sample means becomes normal in shape. The importance of the Central Limit Theorem is that it removes the constraint of normality in the population. Whenever the sample size is large, we can assume that the sampling distribution is normal, with a mean equal to the population mean and a standard deviation equal to σ/√n , regardless of the shape of the variable in the population. Thus, even if we are working with a variable that is known to have a skewed distribution (like income), we can still assume a normal sampling distribution if the sample size is sufficiently large. Book Title: eTextbook: Statistics: A Tool for Social Research and Data Analysis, Fifth Canadian Edition 5.3. The Sampling Distribution Demonstrating the Central Limit Theorem Demonstrating the Central Limit Theorem What constitutes a “large” sample depends on the shape of the underlying population distribution. The more asymmetric the population distribution, the larger the sample size needed to approximate a normal sampling distribution. While some textbooks state that a sample size of just 25 or 30 is sufficiently large for the sampling distribution to be approximately normal, we use a more conservative threshold of 100. To illustrate the effect of the sample size and shape of the underlying population on the distribution of sample means, three typical population distributions are shown in the first row of Figure 5.4: normal (a), skewed (b), and bimodal with two peaks or modes (c). The second, third, and fourth rows show their respective sampling distributions at three different sample sizes, n = 2 , n = 5 , and n = 100. Figure 5.4 Central Limit Theorem in Action Three key features of the Central Limit Theorem are revealed in this illustration. First, as the sample size increases, the sampling distribution approaches normality, with the population mean (represented by the vertical line) at its centre. Second, the sampling distribution is normal when the population distribution is normal (a) regardless of sample size; however, it becomes taller and narrower (sample means cluster more and more around the population mean or, more technically, the standard error decreases) as the sample size increases from n = 2 through n = 100. This pattern reflects the fact that larger samples are generally more representative of the population. Third, the more asymmetrical the shape of the underlying population, the larger the sample size needed for normality to occur. The bimodal distribution (c) is more irregular than the skewed distribution (b) and thus requires a larger sample to become normal. Overall, a normal sampling distribution can be ensured by using a large sample. As we noted, a good general rule is that if the sample size is 100 or more, you can assume that the sampling distribution of sample means is normal no matter the form of the underlying population distribution. 161 Book Title: eTextbook: Statistics: A Tool for Social Research and Data Analysis, Fifth Canadian Edition Chapter 5. Introduction to Inferential Statistics: Sampling and the Sampling Distribution 5.4. Constructing a Sampling Distribution 5.4. Constructing a Sampling Distribution Developing an understanding of the sampling distribution and the two theorems stated in the previous section—what they are and why they are important—is often one of the more challenging tasks for beginning students of 162 statistics.To help grasp the concepts, we demonstrated (in Figure 5.4) that the sampling distribution of sample means approaches a normal curve as the sample size increases. In this section, we expand on this idea and provide step- by-step calculations for developing a sampling distribution. Let’s start by reviewing the most important points about the sampling distribution: 1. Its definition: The sampling distribution is the distribution of a statistic (like means or proportions) for all possible sample outcomes of a certain size. 2. Its shape: It is normal (see Appendix A). 3. Its central tendency and dispersion: The mean of the sampling distribution has the same value as the mean of the population. The standard deviation of the sampling distribution—or the standard error—is equal to the population standard deviation divided by the square root of n. (See the previous theorems.) To illustrate these points, let’s suppose that we have a population of four people and are interested in the amount of money each person has in their possession. (The number of cases in this problem is kept very small to simplify the computations.) We find that the first person in our population has $2 , the second person has $4 , the third person has $6 , and the fourth person has $8. So this population has a mean, μ , of $5 and a standard deviation, σ , of $2.236 , as calculated in Table 5.1. Table 5.1 Calculating the Mean and Standard Deviation of the Population Recall that the sampling distribution of sample means is the theoretical distribution of the mean for all possible samples of a certain sample size (n), with a mean of μ and a standard deviation of σ/√n. So let us derive the sampling distribution of sample means for n = 2 —that is, draw every conceivable combination (every possible sample) of two people from our population of four people. To draw every possible sample, sampling with replacement must be used; we randomly select a person from the population, 163 replace that person back in the population, and then again randomly select a person from the population. Hence, we end up drawing several “odd”-looking samples because the same person can be selected twice into the same sample. In a population of four people, there are 16 theoretical samples of two people (i.e., when n = 2 , there are only 4 × 4 , or 16 , possible samples). With 16 samples, there are 16 sample means. Table 5.2 presents every possible sample of two people from our population of four people. Table 5.2 Calculating the Sampling Distribution of Sample Means (n = 2) Sample Scores Sample Mean 1 ($2, $2) $2 2 ($2, $4) a $3 3 ($2, $6) $4 4 ($2, $8) $5 5 ($4, $2) $3 6 ($4, $4) $4 7 ($4, $6) $5 8 ($4, $8) $6 9 ($6, $2) $4 10 ($6, $4) $5 11 ($6, $6) $6 12 ($6, $8) $7 13 ($8, $2) $5 14 ($8, $4) $6 15 ($8, $6) $7 16 ($8, $8) $8 In the first sample, the person in our population with $2 in their possession was randomly selected twice—this is one of those “odd”-looking samples. The mean for this sample is $2 , or (2 + 2)/2 = 2. In the second theoretical sample, the first person randomly selected from the population was again the person with $2. This person was replaced back in the population. Next, a second person was randomly selected from the population, which was the person with $4. The mean for this sample is $3 , or (2 + 4)/2 = 3. This process continues until every possible sample of two people from our population of four people has been drawn, as shown in Table 5.2. With all possible combinations of samples in hand ( 16 in total), we can build the sampling distribution of sample means. The histogram in Figure 5.5 displays this information. The histogram shows that the sample mean of 5 occurs four times, more than any other mean. We can confirm this by counting the number of times the mean of 5 occurs in Table 5.2. The means of 4 and 6 occur three times each, while the means of 3 and 7 occur twice, and the means of 2 and 8 occur once each. As per the theorems stated in the previous section, Figure 5.5 demonstrates that our sampling distribution of means is symmetrical and approximately normal in shape even though the shape of the underlying population distribution is not normal. 164 Figure 5.5 Sampling Distribution of Sample Means (n = 2) The theorems also tell us that the mean of the sampling distribution has the same value as the mean of the population and that the standard deviation of the sampling distribution (standard error) is equal to the standard deviation of the population divided by the square root of n(σ/√n). This is proved in Table 5.3. 165 Table 5.3 Calculating the Mean and Standard Deviation of the Sampling Distribution of Sample Means (n = 2) Comparing Table 5.3 and Table 5.1, we see that the mean of the sampling distribution, 5 , is the same as the mean of the population, 5. We also see that the standard deviation of the sampling distribution, 1.581 , is equal to the standard deviation of the population divided by the square root of n. That is, the population standard deviation, 2.236 , divided by the square root of 2 , or 2.236/√2 , is 1.581 , which is identical to the standard deviation of the sampling distribution. As further proof, Figure 5.6 shows the sampling distribution of sample means for n = 3. (Note that in a population of four people, when sample size equals three, there are 4 × 4 × 4 , or 64 , possible samples.) We see that the mean of this sampling distribution is also 5 and that the distribution is approximately normal in shape. However, in comparison to Figure 5.5, we see that the sampling distribution for n = 3 is less variable or spread out. That is, the standard deviation of this sampling distribution is equal to 1.291 , or 2.236/√3. Figure 5.6 Sampling Distribution of Sample Means (n = 3) In conclusion, we have confirmed the three fundamental components of the theorems in Section 5.3. First, the sampling distribution is normal if either the sample size is large or the shape of the distribution of the variable in the population is normal. While the sampling distribution can be approximately normal in shape for even small sample sizes, as shown in Figures 5.5 and 5.6, we recommend using a sample size of 100 or more. Second, the sampling distribution has a mean, μ = 5 in our example, that is identical to the mean of the population. Third, the standard deviation of the sampling distribution of 166 sample means is equal to the standard deviation of the population divided by the square root of n. It also decreases as the sample size increases. Book Title: eTextbook: Statistics: A Tool for Social Research and Data Analysis, Fifth Canadian Edition Chapter 5. Introduction to Inferential Statistics: Sampling and the Sampling Distribution 5.5. Normal Approximation of the Sampling Distribution of Proportions 5.5. Normal Approximation of the Sampling Distribution of Proportions While we have focused on the sampling distribution of sample means (for interval-ratio variables), other statistics such as the sample proportion (for nominal and ordinal variables), which the Central Limit Theorem applies to, also have a known sampling distribution. When the sample size is large, it turns out that the sampling distribution of the proportion is approximately normal, with mean (μp) equal to the population value (Pμ) and standard deviation (σp) equal to √Pμ(1 − Pμ)/n. Unlike the sampling distribution of sample means, what is meant by a large sample is more intricate than n ≥ 100. Sample size is considered large if both nPμ and n(1 − Pμ) are 15 or more. While some textbooks use a lower threshold, such as 5 or 10 , we recommend using a more conservative threshold of 15. (Alternative methods, used when these assumptions are not satisfied, are not considered in this textbook.) The value of Pμ is important, even when the sample size is relatively large, because values closer to the extremes (zero or one) result in a more skewed distribution and therefore a poorer approximation of the normal curve (see Figure 5.7). If both nPμ ≥ 15 and n(1 − Pμ) ≥ 15 , we can safely assume that the sampling distribution of proportions is approximately normal. Figure 5.7 Sampling Distribution of Proportions for Different Values of Pμ and n To illustrate these points, four sampling distributions are shown in Figure 5.7. When Pμ = 0.10 and n = 5 , then nPμ = 5(0.10) = 0.50 and n(1 − Pμ) = 5(1 − 0.10) = 4.50. This scenario is clearly insufficient to approximate a normal distribution, as revealed in the top left corner of Figure 5.7. While a sample of size of 100 provides a better approximation (bottom left corner), the distribution is still skewed. Indeed, nPμ = 10 , which is below the threshold of 15. Finally, when Pμ = 0.50 and n = 100 , both nPμ and n(1 − Pμ) = 50 and the sampling distribution becomes approximately normal. It also becomes smoother and less blocky. 167 Book Title: eTextbook: Statistics: A Tool for Social Research and Data Analysis, Fifth Canadian Edition Chapter 5. Introduction to Inferential Statistics: Sampling and the Sampling Distribution 5.6. Linking the Population, the Sampling Distribution, and the Sample 5.6. Linking the Population, the Sampling Distribution, and the Sample The role of the sampling distribution in inferential statistics is to link the sample with the population. In this section, we look at how the sampling distribution works together with the sample and the population using the General Social Survey (GSS), the database used for SPSS exercises in this textbook. We will start with the “population,” or the group we are actually interested in and want to learn more about. In the case of the GSS, the population consists of all Canadians (aged 15 and older) living in private households in the 10 provinces. This includes about 29 million people. Clearly, we can never interview all these people and learn what they are like or their views on various social issues. What can be done to learn more about this huge population? This brings us to the concept of “sample,” a carefully chosen subset of the population. The GSS is administered to about 27,000 people, each of whom is chosen by a sophisticated technique that is ultimately based on the principle of EPSEM. The respondents are contacted at home and asked for background information (e.g., age, gender, years of education), as well as their behaviours, opinions, or attitudes regarding selected social issues. When all this information is collated, the GSS database includes information on hundreds of variables for the people in the sample. 168 So we have a lot of information about the variables for the sample (the 27,000 or so people who actually respond to the survey), but no information about these variables for the population (the 29 million Canadians aged 15 and older living in private households in the 10 provinces). How do we go from the known characteristics of the sample to the unknown population? This is the central question of inferential statistics, and the answer is “by using the sampling distribution.” Remember that, unlike the sample and the population, the sampling distribution is a theoretical device. However, we can work with the sampling distribution because its shape, central tendency, and dispersion are defined by the theorems presented earlier in this chapter. First, for any variable from the GSS, we know that the sampling distribution is normal in shape because the sample is “large” (n is much greater than 100 ). Second, the theorems tell us that the mean of the sampling distribution has the same value as the mean of the population. If all Canadians, aged 15 and older, living in private households in the 10 provinces met on average 3.29 people in the past month (μ = 3.29) , the mean of the sampling distribution would also be 3.29. Third, the theorems tell us that the standard deviation (or standard error) of the sampling distribution is equal to the population standard deviation (σ) divided by the square root of n. Therefore, the theorems tell us the statistical characteristics of this distribution (shape, central tendency, and dispersion), and this information allows us to link the sample to the population. How does the sampling distribution link the sample to the population? It is crucial to know that the sampling distribution is normal when n is large. This means that more than two thirds (68%) of all samples are within ±1 standard deviation (i.e., standard error) of the mean (which is the same value as the population parameter), that about 95% are within ±2 standard deviations, and so forth. We do not (and cannot) know the actual value of the mean of the sampling distribution because it is impractical to draw every conceivable combination (i.e., every possible sample) of 27,000 Canadians from the population of 29 million Canadians living in private households in the 10 provinces. However, there is no need to draw all possible samples because the theorems give us crucial information about the mean and standard error of the sampling distribution that we can use to link the sample to the population. In practice, as you will see in the following chapters, we draw just one sample and use this information (i.e., that the sampling distribution of all possible sample means is normal, with a mean equal to the population mean and a standard deviation equal to the population standard deviation divided by the square root of n) to link the sample to the population. To summarize, we have focused on the GSS to see the roles played by the population, the sample, and the sampling distribution. Our goal is to infer information about the population (all Canadians aged 15 and older living in 169 private households in the 10 provinces). When populations are too large to test (and contacting 29 million Canadians is far beyond the capacity of even the most energetic pollster), we use information from carefully drawn probability samples to estimate the characteristics of the population—the full sample of the GSS consists of about 27,000 Canadians aged 15 and older living in private households in the 10 provinces. The sampling distribution, the theoretical distribution whose characteristics are defined by the theorems, links the known sample to the unknown population. Book Title: eTextbook: Statistics: A Tool for Social Research and Data Analysis, Fifth Canadian Edition Chapter 5. Introduction to Inferential Statistics: Sampling and the Sampling Distribution 5.7. Symbols and Terminology 5.7. Symbols and Terminology In the following chapters, we will work with three entirely different distributions (i.e., the sample distribution, the population distribution, and the sampling distribution). The purpose of inferential statistics is to acquire knowledge of the population from information gathered from a sample by means of the sampling distribution. To distinguish clearly among these various distributions, we will often use symbols. The symbols used for the means and standard deviations of samples and populations were already introduced in Chapter 3. In this chapter, we have also used these population symbols for the sampling distribution for convenience. However, Table 5.4 provides new symbols for this distribution, denoted with Greek-letter symbols that are subscripted according to the sample statistic of interest. Table 5.4 Symbols for Means and Standard Deviations of Three Distributions Mean Standard Proportion Deviation 1. Samples ¯ X s Ps 2. Populations μ σ Pμ 3. Sampling distributions ¯ ¯ μx σx of means μp σp of proportions To read this table, note that the mean and standard deviation of a sample are denoted with Roman letters ( X and s), while the mean and standard ¯ deviation of a population are denoted with the Greek-letter equivalents ( μ and σ ). Proportions calculated on samples are symbolized as P-sub-s (s for sample), while population proportions are denoted as P-sub- μ ( μ for the population). The symbols for the sampling distribution are Greek letters with Roman letter subscripts. The mean and standard deviation of a sampling distribution of sample means are “mu-sub-x-bar” and “sigma-sub-x-bar.” The mean and standard deviation of a sampling distribution of sample proportions are “mu-sub-p” and “sigma-sub-p.” 170 While only the mean and proportion have been mentioned here, the list of statistics with a sampling distribution that the Central Limit Theorem applies to can be extended even further. Altogether, this textbook will examine four sampling distributions: the Z distribution (also called the standard normal distribution), the Student’s t distribution, the chi-square distribution, and the F- ratio distribution. Book Title: eTextbook: Statistics: A Tool for Social Research and Data Analysis, Fifth Canadian Edition Chapter 5. Introduction to Inferential Statistics: Sampling and the Sampling Distribution Summary Summary 1. Because populations are almost always too large to test, a fundamental strategy of social science research is to select a sample from the defined population and then use information from the sample to generalize to the population. This is done either by estimation or by hypothesis testing. 2. Researchers choose simple random samples by selecting cases from a list of the population following the rule of EPSEM (each case has an equal probability of being selected). Samples selected by the rule of EPSEM have a very high probability of being representative. 3. The sampling distribution, the central concept in inferential statistics, is a theoretical distribution of all possible sample outcomes. Because its overall shape, mean, and standard deviation are known (under the conditions specified in the two theorems), the sampling distribution can be adequately characterized and utilized by researchers. 4. The two theorems that were introduced in this chapter state that when the variable of interest is normally distributed in the population or when the sample size is large (n ≥ 100) , the sampling distribution is normal in shape, with its mean equal to the population mean and its standard deviation (or standard error) equal to the population standard deviation divided by the square root of n. 5. The theorems can be extended to proportions. As sample size increases, the distribution of the sample proportion tends to become normal. 6. All applications of inferential statistics involve generalizing from the sample to the population by means of the sampling distribution. Both estimation procedures and hypothesis testing incorporate the three distributions, and it is crucial that you develop a clear understanding of each distribution and its role in inferential statistics. Glossary Central Limit Theorem EPSEM Parameters Representative Sampling distribution Simple random sample Standard error Multimedia Resources 171 Visit the companion website for the fifth Canadian edition of Statistics: A Tool for Social Research and Data Analysis to access a wide range of student resources: www.cengage.com/healey5ce. Problem 5.1 This exercise is extremely tedious and hardly ever works out the way it ought to (mostly because not many people have the patience to draw an “infinite” number of even very small samples). However, if you want a more concrete and tangible understanding of sampling distributions and the two theorems presented in this chapter, then this exercise may have a significant payoff. Below are listed the ages of a population of students at a very small community college (N = 50). By a random method (such as a table of random numbers), draw at least 50 samples of size 2 (i.e., 50 pairs of cases), compute a mean for each sample, and plot the means on a histogram. (Incidentally, this exercise will work better if you draw 100 or 200 samples and/or use samples larger than n = 2.) a. The curve you have just produced is a sampling distribution. Observe its shape: after 50 samples, it should be approaching normality. What is your estimate of the population mean (μ) based on the shape of the curve? b. Calculate the mean of the sampling distribution (μx). Be ¯ careful to do this by summing the sample means (not the scores) and dividing by the number of samples you have drawn. Now compute the population mean ( μ ). These two means should be very close in value because μx = μ by the Central Limit ¯ Theorem. c. Calculate the standard deviation of the sampling distribution (use the means as scores) and the standard deviation of the population. Compare these two values. You should find that the two standard deviations are very close in value because σx = σ/√n. ¯ d. If none of the above exercises turned out as they should have, it is for one or more of the following reasons: 1. You did not take enough samples. You may need as many as 100 or 200 (or more) samples to see the curve begin to look “normal.” 2. The sample size (2) is too small. An n of 5 or 10 would work much better. 3. Your sampling method is not truly random and/or the population is not arranged in random fashion. 17 20 20 19 20 18 21 19 20 19 19 22 19 23 19 20 23 18 20 20 22 19 19 20 20 23 17 18 21 20 20 18 20 19 20 22 17 21 21 21 21 20 20 20 22 18 21 20 22 21 You Are the Researcher Using SPSS to Draw Random Samples with the 2018 CCHS The demonstration and the exercise below use the shortened version of the 2018 CCHS data. Start SPSS, and open the CCHS_2018_Shortened.sav file. 172 SPSS DEMONSTRATION 5.1 Estimating Average BMI SPSS includes a procedure for drawing random samples from a database. We can use this procedure to illustrate some points about sampling and to convince the skeptics in the crowd that properly selected samples produce statistics that are close approximations of the corresponding population values or parameters. For the purposes of this demonstration, the CCHS sample is treated as a population and its characteristics are treated as parameters. The instructions below calculate a mean for hwtdgcor (BMI, or body mass index) for three random samples of different sizes drawn from the CCHS sample. The actual average BMI score of the sample (which is the parameter or μ ) is 27.26 (see Demonstration 3.2). The samples are roughly 10% , 25% , and 50% of the population size, and the program selects them by a process that is quite similar to a table of random numbers. Therefore, these samples may be considered simple random samples. As a part of this procedure, we also request the “standard error of the mean” or S.E. mean. This is the standard deviation of the sampling distribution of sample means (σx) for a sample of this size. This statistic is of interest because we ¯ can expect our sample means to be within this distance of the population value or parameter. With the CCHS_2018_Shortened.sav file loaded, click Data from the menu bar of the Data Editor window and then click Select Cases. The Select Cases window appears and presents several different options. To select random samples, check the circle next to “Random sample of cases” and then click on the Sample button. The Select Cases: Random Sample dialog box will open. We can specify the size of the sample in two different ways. If we use the first option, we can specify that the sample will include a certain percentage of cases in the database. The second option allows us to specify the exact number of cases in the sample. Let’s use the first option and request a 10% sample by typing 10 in the box on the first line. Click Continue, and then click OK in the Select Cases window. The sample will be selected and can now be processed. To find the mean income for the 10% sample, click Analyze, Descriptive Statistics, and then Descriptives. The Descriptives dialog box will open. Find hwtdgcor in the variable list, and transfer it to the Variable(s) box. In the Descriptives dialog box, click the Options button and select S.E. mean in addition to the usual statistics. Click Continue and then OK, and the requested statistics will appear in the output window. Now, to produce a 25% sample, return to the Select Cases window by clicking Data and Select Cases. Click the Reset button at the bottom of the window, and then click OK, and the full data set (N = 1,500) will be restored. Repeat the procedure we followed for selecting the 10% sample. Click the button next to “Random sample of cases,” and then click on the Sample button. The Select Cases: Random Sample window will open. Request a 25% sample by typing 25 in the box, click Continue and OK, and the new sample will be selected. 173 Run the Descriptives procedure for the 25% sample (do not forget S.E. 174 mean), and note the results. Finally, repeat these steps for a 50% sample. The results are summarized below: Sample % Sample Sample Standard Sample Mean Size Mean Error ± Standard Error 10 154 28.75 0.45 28.30 − 29.20 25 385 26.80 0.25 26.55 − 27.05 50 811 27.28 0.18 27.10 − 27.46 Notice that the standard error of the mean (i.e., the standard deviation of the sampling distribution of sample means) decreases as the sample size increases. This should reinforce the common-sense notion that larger samples provide more accurate estimates of population values. All three samples produced estimates (sample means) that are quite close in value to the population value of 27.26. However, the largest sample is the most accurate or closest to the true population value of 27.26 ; it is only 0.02 higher. This demonstration should reinforce one of the main points of this chapter: Statistics calculated on samples that have been selected according to the principle of EPSEM are (almost always) reasonable approximations of their population counterparts. Exercise (using CCHS_2018_Shortened.sav) 3.1 Following the procedures in Demonstration 5.1, select three samples from the 2018 CCHS database (CCHS_2018_Shortened.sav): 15% , 30% , and 60%. Get descriptive statistics for hwtdgcor (don’t forget to get the standard error), and use the results to complete the following table: Sample Sample Size Sample Mean Sta % 15 30 60 Summarize these results. What happens to the standard error as the sample size increases? Why? How accurate are the estimates (sample means)? Are all sample means within a standard error of 27.26 ? How does the accuracy of the estimates change as the sample size changes?