Covariance Research Design PDF
Document Details
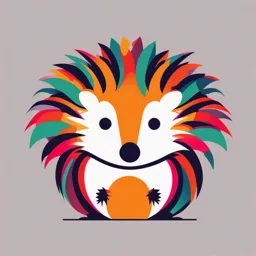
Uploaded by WellInformedSunstone
MSCP-1
Tags
Related
Summary
This document provides a comprehensive overview of covariance research design. It explores different types of covariance analysis, including ANCOVA, MANCOVA, and regression analysis with covariates. The document also discusses the importance of controlling for covariates in various research designs. This includes quasi-experimental, randomized controlled trials, longitudinal, and cross-sectional designs, providing theoretical and real-world examples.
Full Transcript
Covariance Research Design MSCP I Submitted to: Dr. Mussarat Group Members Ayesha Tahir Arshia Kamal Kousar Khan Mahnoor Shakil Arfa Sattar Covariance Research Design Covariance research design is a type of research methodology that focuses on understanding the relat...
Covariance Research Design MSCP I Submitted to: Dr. Mussarat Group Members Ayesha Tahir Arshia Kamal Kousar Khan Mahnoor Shakil Arfa Sattar Covariance Research Design Covariance research design is a type of research methodology that focuses on understanding the relationship between variables while controlling for other variables that might influence that relationship. This helps to isolate the true effect of the independent variable on the dependent variable. Direction of Relationship Positive Covariance: This occurs when an increase in one variable is associated with an increase in another variable. Negative Covariance: This happens when an increase in one variable is associated with a decrease in another variable. Zero Covariance: This indicates that there is no relationship between the two variables; changes in one variable do not predict changes in the other. Importance of Covariance Research Designs in Psychology 1. Understanding Complex Behaviors 2. Controlling for Individual Differences 3. Enhancing Validity of Findings 4. Application in Interventions 5. Statistical Analysis Covariance Research Design vs. Correlational Research Design 1. Controlling for the effects of other variables 2. Covariance research design typically uses analysis of covariance (ANCOVA) or multivariate analysis of covariance (MANCOVA), whereas correlational research design typically uses Pearson's correlation coefficient or Spearman's rank correlation coefficient. Covariance Research Design vs. Experimental Research Design 1. Corelation vs Causation 2. Manipulation 3. Experimental research design involves controlling for extraneous variables through randomization, matching, or other techniques, whereas covariance research design controls for covariates statistically. 4. Experimental research design typically has higher internal validity than covariance research design due to the manipulation of variables and control for extraneous variables. Types of Covariance Research Designs and Differences Arshia Kamal ANCOVA Purpose: Tests the effect of one or more independent variables (IVs) on a single dependent variable (DV) while controlling for one or more covariates. Example: Investigating how a teaching method (IV) affects test scores (DV), while controlling for students' prior knowledge (covariate). MANOVA Purpose: Similar to ANCOVA but involves multiple dependent variables (DVs). It tests the effects of IVs on multiple DVs while controlling for covariates. Example: Examining how a teaching method (IV) affects both math and reading scores (DVs), while controlling for students' prior knowledge (covariate). Regression Analysis with Covariates Purpose: Examines the relationship between one or more independent variables (including covariates) and a continuous dependent variable (DV). Example: Investigating how time spent studying (IV) and socioeconomic status (covariate) predict exam scores (DV). Covariance Structure Models (SEM): Purpose: SEM is a broader technique that models complex relationships between multiple observed and latent variables. It can incorporate both direct and indirect effects, mediating variables, and covariates. Example: Testing how self-esteem (latent variable) influences mental health (DV), while controlling for age and gender (covariates), and examining if the effect of self-esteem is mediated by social support. Quasi-Experimental Designs with Covariates: Purpose: Used when random assignment is not possible. Researchers use covariates to control for pre-existing differences between groups in non-randomized studies. Example: Evaluating the impact of a new teaching method on test scores in two different schools (non- random assignment), adjusting for baseline test scores and other factors. Randomized Controlled Trials (RCTs) with Covariates Purpose: The gold standard for testing causal relationships. RCTs involve random assignment, but covariates can still be included to increase precision or account for variables that may influence the outcome. Example: Testing a new drug's effect on blood pressure in a randomly assigned group of participants, adjusting for baseline health variables. Longitudinal Designs with Covariates Purpose: Measures variables over time to observe changes and causal relationships while controlling for covariates. Example: Tracking students' academic performance over several years and analyzing how early life experiences (covariates) influence their achievement over time. Cross-Sectional Designs with Covariates Purpose: Collects data at one point in time and examines relationships between variables while controlling for covariates. Example: Investigating the relationship between income and health at a single point in time, controlling for age, gender, and education (covariates). Key Differences Research Design Primary Focus Number of Dependent Data Structure Variables ANCOVA Comparing group means while controlling Single dependent variable Cross-sectional or for covariates pre/post data MANCOVA Comparing group means with multiple Multiple dependent variables Cross-sectional or dependent variables pre/post data Regression with Relationship between IVs and a Covariates continuous DV Single dependent variable Continuous data SEM Complex relationships among variables Multiple dependent variables Both cross-sectional or (including latent) longitudinal data Quasi-Experimental Non-randomized group comparisons with Single or multiple dependent Non-randomized, cross- with Covariates covariates variables sectional or longitudinal Randomized group comparisons with Single or multiple dependent Randomized, can be RCTs with Covariates covariates variables cross-sectional or longitudinal Longitudinal with Single or multiple dependent Longitudinal, repeated Covariates Tracking change over time with covariates variables measures Cross-Sectional with Single or multiple dependent Cross-sectional, single Covariates Snapshot of relationships with covariates variables time point Analysis of Covariance Kousar Khan 007959-MSPSY/F24 ANCOVA A statistical technique that allows us to examine the effects of an independent variable on a dependent variable while controlling for the influence of one or more covariates. Covariates are variables that are not of primary interest but can influence the dependent variabl. Adjusted Mean Predicted mean value of dependent variable for a specific group, controlling for covariates. Example: Comparing Teaching Methods Research Question: Is there a significant difference in student achievement between Method A and Method B? Covariate: Prior knowledge ANCOVA Analysis: Calculate adjusted means for each teaching method Conclusion: If adjusted means differ significantly, teaching method has a significant effect on student achievement, independent of prior knowledge. Types of ANCOVA One-Way ANCOVA 1 categorical independent variable (factor) 1 continuous covariate Example: Comparing the mean test scores of three different teaching methods (factor), while controlling for the students’ prior knowledge (covariate). Two-Way ANCOVA 2 categorical independent variables (factors) 1 continuous covariate Example: Investigating the effect of gender (factor 1) and type of therapy (factor 2) on depression scores (dependent variable), while controlling for initial depression levels (covariate) Basic Requirements For ANCOVA Independent variable (Discrete, nominal or ordinal) Dependent Variable (Continuous, interval or ratio) Covariate (Continuous, interval or ratio) Real World Example Real-World Example: MBSR and Chronic Pain Study: Creswell & Kabat-Zinn (1994) Research Question: Does Mindfulness-Based Stress Reduction (MBSR) reduce psychological distress in chronic pain patients? Variables: Independent Variable: MBSR Program (Nominal: MBSR vs. Control) Dependent Variable: Psychological Distress (Continuous: Measured by a standardized scale) Covariate: Baseline Psychological Distress (Continuous: Measured by the same scale) Analysis: ANCOVA to control for baseline distress levels. Findings: MBSR significantly reduced psychological distress compared to the control group, even after accounting for initial distress levels Analysis of covariance in Example Measured baseline distress Included covariate in ANCOVA model Adjusted mean Compared adjusted mean Assumptions of ANCOVA Normality Homogeneity of Variance Independence of Observations Linearity Independence of Covariate and Treatment Effect Advantages of ANCOVA Increased Power Reduced Error Variance Improved Precision Efficiency Disadvantages of ANCOVA Assumption Sensitivity Complexity Measurement Error Limited Generalizability MANCOVA Mahnoor Shakil Introduction MANCOVA (Multivariate Analysis of Covariance) is a statistical technique used to analyze the relationship between multiple dependent variables and one or more independent variables, while controlling for the effects of one or more covariates. Types There are several types of MANCOVA, including one-way and two-way MANCOVA: One-way MANCOVA :Similar to a one-way ANOVA, this type of MANCOVA uses one multi-level nominal independent variable and multiple dependent variables. For example, you could use a one-way MANCOVA to compare exam performance based on test anxiety levels while controlling for revision time. Two-way MANCOVA :Often considered an extension of the two-way ANOVA, this type of MANCOVA is used when there are two or more dependent variables. Factorial MANCOVA: Analyzes the effects of multiple independent variables and their interactions on multiple dependent variables with covariates Assumptions: Independent Random Sampling: MANCOVA assumes that the observations are independent of one another, there is not any pattern for the selection of the sample, and that the sample is completely random. Level and Measurement of the Variables: MANCOVA assumes that the independent variables are categorical and the dependent variables are continuous or scale variables. Covariates can be either continuous, ordinal, or dichotomous. Absence of multicollinearity: The dependent variables cannot be too correlated to each other. Tabachnick & Fidell (2012) suggest that no correlation should be above r =.90.. Normality: Multivariate normality is present in the data. Homogeneity of Variance: Variance between groups is equal. Relationship between covariate(s) and dependent variables: in choosing what covariates to use, it is common practice to assess if a statistical relationship exists between the covariate(s) and the dependent variables; this can be done through correlation analyses. Steps 1. Initial Setup:Identify dependent variables, independent variables, and covariates.Check sample size requirements ,code and organize data properly 2. Preliminary Analysis:Screen for outliers, check for missing data ,verify assumptions 3. Main Analysis: Adjust independent variables for covariate effects ,calculate the multivariate test statistics (Wilks' Lambda, Pillai's Trace, etc.),determine significance of main effects and interactions 4. Post-hoc Analysis :Conduct follow-up univariate ANCOVAs if multivariate tests are significant ,perform pairwise comparisons with appropriate adjustments ,calculate effect sizes ADVANTAGES OF MANCOVA: Statistical Power Control for Confounding Variables Relationship Analysis Efficiency Disadvantages Complexity Assumptions Sample size requirements Sensitivity to outliers Power considerations Real life applications 1. Program evaluation 2. Psychological research Key difference *Covariates*: MANCOVA includes covariates to control for their effects, while MANOVA does not. Model complexity*: MANCOVA is a more complex model than MANOVA, as it includes additional variables (covariates). Assumptions*: MANCOVA has an additional assumption (homogeneity of regression slopes) compared to MANOVA. Advantages, Diadvantages, Threats to Validity Arfa Sattar Covariance Research Design Advantages Disadvantages 1. Identification of 1. Causation. Relationships. 2. Limited Control. 2. Flexibility. 3. Data Quality. 3. Cost-Effectiveness. 4. Complexity of 4. Ethical Considerations Interpretation. 5. Foundation for Further Research. Threats to Validity Internal Validity External Validity 1. Confounding variables. 1. Sampling bias. 2. Selection bias. 2. Setting. 3. Measurement error. 3. Participant reactivity. 4. Regression to the mean. 4. Experimenter bias. 5. History. How to Address Threats a)Controlling for covariates. Including relevant covariates in the analysis to control for their effects. b)Validating measures. Ensuring that measures are valid and reliable. c)Using diverse samples. Recruiting diverse samples to increase generalizability. d)Replicating findings. Replicating findings to ensure reliability and validity. References Bock, R. D., & Bargmann, R. E. (1966). Analysis of covariance structures. Psychometrika, 31(4), 507– 534. https://doi.org/10.1007/bf02289521 Elliott, A. C., & Woodward, W. A. (2006). Statistical Analysis Quick Reference Guidebook: with SPSS examples. In SAGE Publications Pvt. Ltd eBooks (Issue 1). http://ci.nii.ac.jp/ncid/BA87454441 Van Breukelen, G. (2010). Encyclopedia of Research Design. In SAGE Publications, Inc. eBooks. https://doi.org/10.4135/9781412961288