Experimental Design I: Single Factor Designs Chapter 7 PDF
Document Details
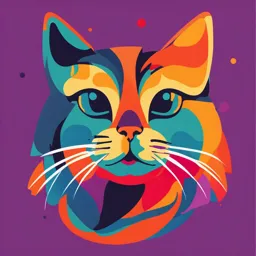
Uploaded by AccomplishedSodium
York University
Goodwin & Goodwin
Tags
Summary
This document details experimental design concepts, including single-factor designs (two-level and multi-level) and types of control groups. It also explores inferential statistics like t-tests and ANOVA for analyzing data related to these designs. Different graph types to present data results are also discussed.
Full Transcript
EXPERIMENTAL DESIGN I: SINGLE FACTOR DESIGNS – CHAPTER 7 SINGLE-FACTOR TWO-LEVEL DESIGN The term “factor” is synonymous with independent variable (IV). Know the names of the following designs: Goodwin & Goodwin, 2017 a) Independent groups – the IV is a manipulated variable and random assignment to...
EXPERIMENTAL DESIGN I: SINGLE FACTOR DESIGNS – CHAPTER 7 SINGLE-FACTOR TWO-LEVEL DESIGN The term “factor” is synonymous with independent variable (IV). Know the names of the following designs: Goodwin & Goodwin, 2017 a) Independent groups – the IV is a manipulated variable and random assignment to groups is used b) Matched groups – Ss are matched on a variable then randomly assigned to conditions c) Ex post facto – the IV is a subject variable d) Repeated-measures design – different term for within-S design Inferential Statistics for Single-Factor, Two-Level Designs A t-test is an inferential statistic used to determine whether the difference between both groups is statistically significant (as opposed to be just due to chance). Use an independent samples t-test for independent groups and ex post facto designs. Use a dependent samples t-test for matched groups and repeated-measures designs. (the formulas for the above t-tests are slightly different). 1 SINGLE-FACTOR – MULTI-LEVEL DESIGNS -more than 2 levels are involved; these are more common than 2-level designs ADVANTAGE – it allows you to discover non-linear effects (any outcome that doesn’t form a straight line when graphed). (With 2 levels, when you connect the points, you always get a straight line and that can be misleading). Goodwin and Goodwin, 2017 2 ANALYZING SINGLE-FACTOR MULTILEVEL DESIGNS Suppose you have Alcohol (IV) with 4 levels: 0, 1, 2, and 3 ozs. If you did t-tests for every possible comparison, there would be a total of 6 t-tests. Do NOT do multiple t-tests because this could increase the chance of a Type I Error (where you say groups differ but they really don’t; i.e., that difference is really only due to chance). The chances of making a Type I error when doing multiple t-tests can be determined by this formula: 1-(1-alpha)c, where c = the number of comparisons made and alpha is usually =.05 (if you had 10 comparisons there would be a 40% chance of making a Type I error). Inferential Statistics for Single-Factor, Multilevel Designs: One-Way Analysis of Variance (ANOVA) The proper inferential statistic to use with multilevel designs is a one-way analysis-of-variance (ANOVA), which tests for some overall level of significance but it doesn’t tell you exactly which conditions are significantly different from the other. In order to establish that, you need to perform post-hoc tests but you should do this only if the one-way ANOVA is significant. Summary: -Conduct a one-way ANOVA (inferential statistic) -If significant, then perform post-hoc tests to see exactly which conditions differ -If the 1-way ANOVA is not significant, stop there – do not do post-hoc tests PRESENTING DATA Data can be presented in sentence form (if it’s a very simple design), in the form of a table or graph. Types of Graphs (disregard this section in the text and use my straightforward lecture notes instead) A) Line Graphs – the IV always goes on the x-axis and the DV on the y-axis. The mean for each condition is represented by a point on a graph and then joined by a line. B) Bar Graphs – the mean for each condition is represented by bars that are separated 3 C) Histograms (text doesn’t discuss this) – same as a bar graph except that the bars touch one another. Which type of graph should be used? It depends on whether the IV is continuous or discrete. Continuous variable – a variable for which an infinite # of values potentially exists (ex. alcohol dose, age, time spent exercising, etc) Discrete variable – each level of the IV represents a distinct category that is qualitatively (as opposed to quantitatively) different from another. No intermediate points can occur. (ex. #kids in a family, types of exercise activities, eye color etc). General rule (applies for experiments that have only one IV): If the IV is continuous, a line graph or histogram is appropriate. If the IV is discrete, use a bar graph. SPECIAL TYPES of CONTROL GROUP DESIGNS A) Placebo Control Group Design (A placebo is something that may seem to have a particular effect but the effect is actually just due to expectation). A placebo control group is usually used in drug-related studies where people have strong expectations about drug effects. In alcohol studies, the placebo control group is given something to drink but there’s no alcohol in it. (In contrast, a regular control group wouldn’t be given nothing at all to drink). -It controls for subjects’ expectations that could affect results. -If the control group is given a placebo, then it’s assumed that all groups (control and 4 experimental) are equal in terms of expectation, and so, any differences in behavior can be attributed to the actual alcohol dose and not to expectation. B) Wait List Control Group -Ss who were randomly assigned to the control group aren’t receiving treatments but eventually will after the study is over (makes things more ethical). An alternative would be to use a pre- post design. C) Yoked Control Group Design - This type of control group is used when the procedures applied to each experimental group member vary because they depend on the behavior of that specific member. (Typically each member within a group receives the exact same treatment/procedures but in some cases that can’t happen). Each control group member is procedurally linked (yoked) to a specific experimental group member, whose behavior will determine how both of them are treated. Example – Does REM deprivation increase sleepiness? Experimental group: in sleep lab; whenever a subject enters REM they are awoken and kept awake for 15 mins Control group: sleeps throughout the night and gets normal REM Problem: maybe sleepiness isn’t due to REM deprivation per se, but rather, due to sleep interruptions. Because the # of awakenings and the time of night at which they occurred, could not be held constant across all Ss in the experimental group, a yoked control group was needed. So, Bob is in the control group and he’s yoked to you. Every time you enter REM and are awoken, Bob is also awoken. So by morning both you and Bob will have been awoken the same # of times, at the same time of night, and kept awake for the same length of time. REM deprived normal REM Experimental group Control group (each S is yoked to a S in the experimental group) S1 - 9 awakenings same 9 awakenings S2 - 4 awakenings same 4 awakenings S3 - 6 awakenings same 6 awakenings The time of the awakenings and the number of awakenings in both groups has now been controlled for through yoking, so any differences in sleepiness can be attributed to REM deprivation and not sleep interruptions. 5