Post-Lab Discussion Experiments 1 & 2 PDF
Document Details
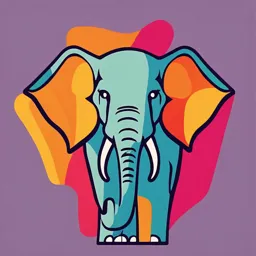
Uploaded by OpulentDrums
Tags
Related
- University of the Philippines Visayas Chem 23.1 Laboratory Techniques PDF
- PIC Practical Manual - Lab Manual of Pharmaceutical Inorganic Chemistry
- Third Stage Inorganic Pharmaceutical Chemistry Lab 5 PDF
- Chem 23.1 Inorganic Analytical Chemistry Laboratory Post-Lab Discussion PDF
- Physical and Inorganic Chemistry Lab 2 PDF
- Inorganic Chemistry Lab Manual (Al-Nahrain University) PDF
Summary
This document is a discussion on lab experiments 1 and 2 for inorganic analytical chemistry laboratory. It outlines learning outcomes, some important terms in chemical analysis, experiments types. It also provides the details on the calculations performed from the experiments.
Full Transcript
INORGANIC ANALYTICAL CHEMISTRY LABORATORY EXPERIMENTS 1 & 2 EXAM SCHEDULE First Long Exam Date: September 26, 2024 Time: 7-10 am Coverage: Experiment 0 – Laboratory Safety and Techniques Experiment 1 – Data Treatment in Analytical Chemistry Experimen...
INORGANIC ANALYTICAL CHEMISTRY LABORATORY EXPERIMENTS 1 & 2 EXAM SCHEDULE First Long Exam Date: September 26, 2024 Time: 7-10 am Coverage: Experiment 0 – Laboratory Safety and Techniques Experiment 1 – Data Treatment in Analytical Chemistry Experiment 2 – Gravimetric Methods of Analyses DATA TREATMENT IN ANALYTICAL CHEMISTRY LEARNING OUTCOMES At the end of this activity, you must be able to: ✓ Define the types of errors that can occur in an experiment ✓ Use statistical parameters to describe a set of data IMPORTANT TERMS IN CHEMICAL ANALYSES ❑ Mean ❑ Median ❑ Precision ❑ Accuracy ❑ Absolute Error ❑ Relative Error IMPORTANT TERMS IN CHEMICAL ANALYSES ❑ Mean - average value of a data set 𝑁 σ𝑖=1 𝑥𝑖 x̄ = 𝑁 Where, x = mean σ𝑁 𝑖=1 𝑥𝑖 = x1 + x2 + x3 +... + xN 𝑁 = number of measurements IMPORTANT TERMS IN CHEMICAL ANALYSES ❑ Median - middle value when replicate data are arranged in increasing or decreasing order If N is odd 𝑥̃ = 𝑋𝑁+1 2 If N is even 𝑋𝑁 + 𝑋𝑁 +1 Where, 2 2 𝑥̃ = 𝑥̃ = median 2 𝑥 = measured data 𝑁 = number of measurements IMPORTANT TERMS IN CHEMICAL ANALYSES ❑ Precision - describes how close your measurements are to the mean value ❑ Accuracy - describes how close your measurements are to the true value (Brown et al., 2019) IMPORTANT TERMS IN CHEMICAL ANALYSES ❑ Absolute Error – difference between the true and calculated value ❑ Relative Error – difference between the true and calculated value divided by the true value ERRORS IN EXPERIMENTAL DATA ❑ Systematic Error – (or determinate) errors vary the experimental mean of a data set from that of an accepted or true value; thus, affecting the accuracy of the experiment. ❑ Random Error – (or indeterminate) errors cause data to be scattered around a mean value. This highly affects the precision of your data. Sources of random errors are usually unknown. ❑ Gross Error – results to outliers in your measurements. Somewhat similar to systematic errors but gross errors usually occur occasionally and often too large. SYSTEMATIC ERRORS ❑ Three types of systematic errors based on their source ✓ Instrumental errors are caused by nonideal instrument behavior, by faulty calibrations, or by use under inappropriate conditions. ✓ Method errors arise from the nonideal chemical or physical behavior of analytical systems. ✓ Personal errors result from the carelessness, inattention, or personal limitations of the experimenter. SYSTEMATIC ERRORS ❑ Classifications ✓ Constant errors will have the same absolute error value whenever the sample size is varied. ✓ Proportional errors will have the same relative error when sample size is changed. SYSTEMATIC ERRORS ❑ Sources can be corrected, detected, and minimized ✓ conduct regular calibrations ✓ analysis using standard reference ✓ performing blank determinations, independent analysis, or varied sample sizes. RANDOM ERRORS ❑ Random errors are ✓ usually hard to quantify due to their very small values ✓ usually unavoidable and caused by uncontrollable variables ✓ usually distributed on what we call a Gaussian curve (or a normal error curve) STATISTICAL TREATMENTS ❑ Some important terms you need to know: ✓ Population ✓ Sample ✓ Parameter ✓ Variables ✓ Statistic ✓ Population (𝜇) and Sample (𝑥̅) Mean ✓ Population (𝜎)and Sample (𝑠) Standard Deviation STATISTICAL TREATMENTS ❑ Some important terms you need to know: ✓ Population - collection of all measurements of interest; can be finite or conceptual ✓ Sample - a subset of the population Sample Population STATISTICAL TREATMENTS ❑ Some important terms you need to know: ✓ Variables – individual data values ✓ Parameter – quantities that define a population or a distribution ✓ Statistic – refers to an estimate of a parameter that is made from a sample of data STATISTICAL TREATMENTS ❑ Some important terms you need to know: ✓ Population (𝜇) and Sample (𝑥̅) Mean 𝑁 𝑁 σ𝑖=1 𝑥𝑖 σ𝑖=1 𝑥𝑖 𝜇= 𝑥ҧ = 𝑁 𝑁 ✓ Population (𝜎) and Sample (𝑠) Standard Deviation 𝑁 σ𝑖=1 𝑥𝑖 − 𝜇 2 σ𝑁 𝑖=1 𝑥𝑖 − 𝑥ҧ 2 𝜎= 𝑠= 𝑁 𝑁−1 EXERCISE Find the mean and standard deviation for 821, 783, 834, and 855. SOLUTION: Mean: Standard deviation: σ𝑁 𝑖=1 𝑥𝑖 σ𝑁 2 𝑥ҧ = 𝑖=1 𝑥𝑖 − 𝑥ҧ 𝑠= 𝑁 𝑁−1 821 + 783 + 834 + 855 (821 − 823.25)2 +(783 − 823.25)2 +(834 − 823.25)2 +(855 − 823.25)2 𝑥ҧ = 𝑠 = 4 4−1 𝑥ҧ = 823.25 𝑠 = 𝟑𝟎. 𝟐𝟔 ഥ = 𝟖𝟐𝟑 𝒙 STATISTICAL TREATMENTS ❑ Pooled standard deviation: 𝑁1 − 1 𝑠1 2 + 𝑁2 − 1 𝑠2 2 + 𝑁3 − 1 𝑠3 2 + ⋯ + 𝑁𝑘 − 1 𝑠𝑘 2 𝑠𝑝𝑜𝑜𝑙𝑒𝑑 = 𝑁1 + 𝑁2 + 𝑁3 + ⋯ + 𝑁𝑘 − 𝑘 OR σ 𝒙𝒊 − 𝒙𝑵 𝟐 𝑠𝑝𝑜𝑜𝑙𝑒𝑑 = 𝑁1 + 𝑁2 + 𝑁3 + ⋯ + 𝑁𝑘 − 𝑘 EXERCISE Calculate a pooled estimate of the standard deviation from the following analysis for nitriloacetic acid, NTA. 𝟐 Sample # N [NTA] ppb Mean, 𝑥 ̅ s 𝒙𝒊 − 𝒙𝑵 1 4 13, 16, 14, 9 13.0 2.94 26.0 2 3 38, 37, 38 37.7 0.577 0.67 3 5 25, 29, 23, 29, 26 26.4 2.61 27.2 SOLUTION: σ 𝒙𝒊 −𝒙𝑵 𝟐 26.0+0.67+27.2 𝑠𝑝𝑜𝑜𝑙𝑒𝑑 = = = 2.45 𝑁1 +𝑁2 +𝑁3 +⋯+𝑁𝑘 −𝑘 4+3+5−3 OR 𝑁1 − 1 𝑠1 2 + 𝑁2 − 1 𝑠2 2 + 𝑁3 − 1 𝑠3 2 + ⋯ + 𝑁𝑘 − 1 𝑠𝑘 2 3 (2.94)2 + 2 (0.577)2 + 4 (2.61)2 𝑠𝑝𝑜𝑜𝑙𝑒𝑑 = = = 2.45 𝑁1 + 𝑁2 + 𝑁3 + ⋯ + 𝑁𝑘 − 𝑘 4+3+5−3 STATISTICAL TREATMENTS ❑ Other measures of precision can be expressed as the variance, the relative standard deviation, the coefficient of variation, and the spread or range. ✓ Variance: ✓ Coefficient of Variation: 𝑁 σ𝑖=1𝑥𝑖 − 𝑥ҧ 2 𝑠 𝑠 2 = 𝐶𝑉 = × 100% 𝑁−1 𝑥ҧ ✓ Relative Standard Deviation: ✓ Spread (or Range): 𝑠 𝑅𝑆𝐷 = 𝜔 = 𝑥𝑚𝑎𝑥 − 𝑥𝑚𝑖𝑛 𝑥ҧ STATISTICAL TREATMENTS ❑ Standard Deviation of Calculated Results Formulas for calculating standard deviations from calculated results (Error Propagation in Arithmetic Calculations) STATISTICAL TREATMENTS ❑ Reporting Computed Data ✓ Significant figures. All digits of a measured quantity, including the uncertain one, are called significant figures. ✓ Rules on the significant figures 1. All nonzero digits are significant. 2. Zeroes between two significant figures are themselves significant. 3. Zeroes at the beginning of a number are never significant. 4. Zeroes at the end of a number are significant if a decimal point is written in the number. PROPAGATION OF ERROR ❑ if the standard deviations of the values making up the final calculation are known, we can apply the propagation of error methods. EXERCISE Estimate the absolute standard deviation and the coefficient of variation for the results of each of the following calculations. Round each result so that it contains only significant figures. (The numbers in brackets are the absolute standard deviations). a) 6.75(±0.03) + 0.843(±0.001) – 7.021(±0.001) SOLUTION: 𝑠 y = 6.75 + 0.843 – 7.021 = 0.572 CV = × 100% 𝑥ҧ sy = (0.03)2 + (0.001)2 + (0.001)2 CV = 0.030 × 100% 0.572 sy = 0.030 CV = 5.244755 ≈ 5% y = 0.57 ± 0.03 EXERCISE 𝑊 𝑚𝑔 𝑥 𝑉 (𝑚𝐿) b) mass of analyte (mg) = 𝑉𝑡 (𝑚𝐿) Where W = 500.0±0.04 mg Vt = 250.0±0.3 mL V = 10.00±0.02 mL SOLUTION: 500.0 𝑥 10.00 y= = 20.00 250.0 𝑠 0.04 2 0.3 2 0.02 2 CV = × 100% 𝑠𝑦 = ( ) +( ) +( ) 𝑥ҧ 𝑦 500.0 250.0 10.00 0.047 𝑠𝑦 CV = × 100% = (0.00233) 20.00 𝑦 CV = 0.235 ≈ 0.2% sy = 20.00 x 0.00233 = 0.047 y = 20.00 ± 0.05 EXERCISE 1.43 ±0.02 𝑥 10−2 −4.76 ±0.06 𝑥 10−3 c) 24.3 ±0.7 + 8.06(±0.08) SOLUTION: Addition and Subtraction 𝑠𝑦 1.43 𝑥 10−2 − 4.76 𝑥 10−3 9.54 𝑥 10−3 = ( 0.00021 2 ) +( 0.7 2 ) y= = 𝑦 9.54 𝑥 10 −3 32.36 24.3 + 8.06 32.36 𝑠𝑦 = 0.0309 (0.0002)2 +(0.00006)2 (0.00021) 𝑦 sy = = 𝑠𝑦 = 2.948 𝑥 10−4 𝑥 0.0309 = 0.0000091 (0.7)2 +(0.08)2 (0.70) 9.54 (±0.21) 𝑥 10−3 y = 2.948 𝑥 10−4 ± (0.0000091) y= 32.36 (±0.70) y = 2.95 ±0.09 𝑥 10−4 Division 9.54 𝑥 10−3 y= = 2.948 𝑥 10−4 CV = 0.091 × 100% 32.36 2.948 CV = 3.0868 ≈ 3% EXERCISE 1 d) 𝑦 = (4.0(±0.4) 𝑥 10−8 ) 2 SOLUTION: 1 −8 −4 0.1 𝑥 10−4 y = (4.0 𝑥 10 ) = 2.0 𝑥 102 CV = × 100% 2.0 𝑥 10−4 𝑠𝑦 1 0.4 𝑥 10−8 CV = 5% = 𝑥 = 0.05 𝑦 2 4.0 𝑥 10−8 −4 −4 sy = 2.0 𝑥 10 𝑥 0.05 = 0.1 𝑥 10 y = 2.0 ±0.1 𝑥 10−4 EXERCISE e) 𝑦 = log[2.00 ±0.02 𝑥 10−4 ] SOLUTION: 𝑦 = log[2.00 𝑥 10−4 ] = -3.6989 CV = 0.0043 × 100% −3.6989 0.02 𝑥 10−4 CV = -0.116250 ≈ -0.1% sy = 0.434 𝑥 = 0.0043 2.00 𝑥 10−4 y = -3.699 (±0.004) EXERCISE e) 𝑦 = antilog[1.200 ±0.003 ] SOLUTION: 1.200 0.109 𝑦 = antilog[1.200] = 10 = 15.849 CV = × 100% 15.849 𝑠𝑦 CV = 0.68774 ≈ 0.7% = 2.303 𝑥 0.003 = 0.0069 𝑦 sy = 15.849 𝑥 0.0069 = 0.109 y = 15.8 (±0.1) DETECTION FOR GROSS ERRORS ❑ Dixon’s Q-test – test for identifying outliers. The Q-test compares the gap between the suspected outlier and its nearest numerical neighbor to the range of the entire data set. The result is compared to a critical value, if Qexp is greater than Q(α, n) then we reject the outlier, if not, we retain. 𝑜𝑢𝑡𝑙𝑖𝑒𝑟′𝑠 𝑣𝑎𝑙𝑢𝑒 − 𝑛𝑒𝑎𝑟𝑒𝑠𝑡 𝑣𝑎𝑙𝑢𝑒 𝑄𝑒𝑥𝑝 = 𝑙𝑎𝑟𝑔𝑒𝑠𝑡 − 𝑠𝑚𝑎𝑙𝑙𝑒𝑠𝑡 𝑣𝑎𝑙𝑢𝑒 DETECTION FOR GROSS ERRORS ❑ Dixon’s Q-test Critical Values n (number of data) Q (0.05,n) 3 0.970 4 0.829 5 0.710 6 0.625 7 0.568 8 0.526 9 0.493 10 0.466 EXERCISE Apply the Q-test (95% CL) to determine whether or not there is a significant basis for rejection of any outliers in the data set below. 0.0902 0.0884 0.0886 0.1000 SOLUTION: 𝑜𝑢𝑡𝑙𝑖𝑒𝑟′𝑠 𝑣𝑎𝑙𝑢𝑒 − 𝑛𝑒𝑎𝑟𝑒𝑠𝑡 𝑣𝑎𝑙𝑢𝑒 𝑄𝑒𝑥𝑝 = 𝑙𝑎𝑟𝑔𝑒𝑠𝑡 − 𝑠𝑚𝑎𝑙𝑙𝑒𝑠𝑡 𝑣𝑎𝑙𝑢𝑒 0.1000 − 0.0902 𝑄𝑒𝑥𝑝 = 0.1000 − 0.0884 𝑄𝑒𝑥𝑝 = 0.845 𝑄𝑐𝑟𝑖𝑡 for N = 4 is 0.829 𝑄𝑒𝑥𝑝 > 𝑄𝑐𝑟𝑖𝑡 ∴ 𝑹𝒆𝒋𝒆𝒄𝒕 𝟎. 𝟏𝟎𝟎𝟎 DETECTION FOR GROSS ERRORS ❑ Grubb’s test – more reliable test for identifying an outlier as recommended by ISO. The test statistic for Grubb’s test is the distance between the sample’s mean and the potential outlier in terms of the sample’s standard deviation. The value of Gexp is compared to a critical value, G(α,n) where α is the probability that we will reject a valid data point and n is the number of data points in the sample. If Gexp is greater than G, we may reject the data point otherwise, we retain the data. 𝒙𝒐𝒖𝒕 − 𝒙 𝑮𝒆𝒙𝒑 = 𝒔 DETECTION FOR GROSS ERRORS ❑ Grubb’s Test Critical Values n (number of data) G (0.05,n) 3 1.115 4 1.481 5 1.715 6 1.887 7 2.020 8 2.126 9 2.215 10 2.290 EXERCISE Apply the Grubbs test (95% CL) to determine whether or not there is a significant basis for rejection of any outliers in the data set below. 10.8, 11.6, 9.4, 7.8, 10.0, 9.2, 11.3, 9.5, 10.6, 11.6 SOLUTION: 𝑥𝑜𝑢𝑡 − 𝑥 𝐺𝑒𝑥𝑝 = Min = 7.8 𝑠 7.8 − 10.18 Max = 11.6 𝐺𝑒𝑥𝑝 = 1.23 Mean = 10.18 𝐺𝑒𝑥𝑝 = 1.93 𝐺𝑐𝑟𝑖𝑡 for N = 10 is 2.290 SD = 1.23 𝐺𝑒𝑥𝑝 < 𝐺𝑐𝑟𝑖𝑡 ∴ 𝑹𝒆𝒕𝒂𝒊𝒏 𝟕. 𝟖 CONFIDENCE INTERVALS Confidence Intervals - This refers to a range of values by which the population mean (μ) lies with at a specific probability (confidence level). This range is around the experimental mean 𝑥ҧ where the limits are termed as confidence limits. Confidence Intervals = sample mean ± margin of error Ex: We may say that we are 90% probable that the true population mean of K+ measurement lies in the interval 7.25 ± 0.15%. This means that the true mean may fall within the interval 7.10% and 7.40%, 90 out of 100 times. You use CI to estimate the mean value of a population parameter. CONFIDENCE INTERVALS If s is a good estimate for σ for N no. of sample 𝑧 𝜎 Population standard deviation 𝐶𝐼 𝑓𝑜𝑟 𝜇 = 𝑥ҧ ± 𝑁 Sample size When σ is unknown, 𝑡𝑠 𝐶𝐼 𝑓𝑜𝑟 𝜇 = 𝑥ҧ ± 𝑁 where t could be calculated using 𝑥ҧ − 𝜇 𝑡= 𝑠 𝑁 ± CONFIDENCE INTERVALS Exercise: Confidence Interval Determine 95% confidence intervals for the mean of seven measurements (1108 mg/L glucose). Assume that s = 19, is a good estimate of 𝜎. 𝑧𝜎 95% 𝐶𝐼 = 𝑥ҧ ± 𝑁 1.96 × 19 95% 𝐶𝐼 = 1108 ± 7 95% 𝐶𝐼 = 1108 ± 14.1 𝑚𝑔/𝐿 Exercise: Confidence Interval The alcohol content of a sample of blood (% C2H5OH) for N=3 measurement is 0.084%. Calculate the 95% confidence interval for the mean assuming N-1 s = 0.0050 is only indication of the precision of the method. 𝑡𝑠 𝐶𝐼 = 𝑥ҧ ± 𝑁 4.30 × 0.0050 95% 𝐶𝐼 = 0.084 ± 3 95% 𝐶𝐼 = 0.084 ± 0.012 % HYPOTHESIS TESTING Involves testing the validity of a null hypothesis, 𝐻𝑜 , which assumes that the numerical quantities being compared are the same, and an alternate hypothesis 𝐻𝑎 , which states otherwise. You use hypothesis testing if you want to determine if some hypothesis about the parameter is likely true or not (ex. results from experiments supports the models or not) Hypothesis tests often include the comparison of (1) the mean of an experimental data set with what is believed to be the true value, (2) the mean to a predicted or cutoff (threshold) value, (3) the means or the standard deviations from two or more sets of data HYPOTHESIS TESTING We will test the following hypothesis (hence, hypothesis testing): 1. Our null hypothesis, 𝐻𝑜 states that 𝜇 = 𝜇𝑜 2. Our alternative hypothesis, 𝐻𝑎 , could be stated in several ways: a. 𝜇 is different than 𝜇𝑜 (𝜇 ≠ 𝜇𝑜 ) (two-tailed) b. 𝜇 is lesser than 𝜇𝑜 (𝜇 < 𝜇𝑜 ) (one-tailed) c. 𝜇 is greater than 𝜇𝑜 𝜇 > 𝜇𝑜 (one-tailed) Z-test and t-test z test let’s us draw conclusions (compare) about the population mean, 𝜇, and the known value, accepted value, a theoretical value, or a threshold value, 𝜇𝑜. applicable for tests concerning one or two means only Only use this if the population variance 𝜎 is known or if sample size is larger than 30 (N>30). Z statistics is used t- test is similar to z-test but is used if 𝜎 is not known, and sample size is small. Procedure is similar to z-test except t statistics is used. When in doubt, use t-test instead of z-test. Z-test Large Sample z test (if s is a good estimate of 𝜎, the z test is appropriate) 1. Our null hypothesis, 𝐻 𝑜 : 𝜇 = 𝜇𝑜 2. Form z statistic: 𝑥ҧ − 𝜇𝑜 𝑧= 𝜎 𝑁 3. State alternative hypothesis Ha and determine rejection region a. Ha : (𝜇 ≠ 𝜇𝑜 ) reject Ho if z ≥ zcrit or if z ≤ −zcrit b. Ha : (𝜇 > 𝜇𝑜 ) reject Ho if z ≥ zcrit c. Ha : (𝜇 < 𝜇𝑜 ) reject Ho if z ≤ −zcrit Exercise: Comparing experimental 𝒙 ഥ with a Known Value A class of 30 students determined the activation energy of a chemical reaction to be 116 kJ mol-1 (mean value) with a standard deviation of 22 kJ mol-1. Are the data in agreement with the literature value of 129 kJ mol–1 at (a) the 95% confidence level and (b) 99% confidence level. Assume s is a good estimate of 𝜎. 𝑘𝐽 𝑘𝐽 Ho : 𝜇 = 𝜇 𝑜 𝑥ҧ − 𝜇𝑜 116 𝑚𝑜𝑙 − 129 𝑚𝑜𝑙 𝑧= 𝜎 = = −3.27 𝑘𝐽 22 Ha : 𝜇 ≠ 𝜇𝑜 𝑁 𝑚𝑜𝑙 30 a. At 95% CI, z = 1.96. Ho is rejected. 𝑥ҧ is significantly different than known value. b. At 99% CI, z = 2.58. Ho is rejected. 𝑥ҧ is significantly different than known value. T-test: Comparing experimental 𝒙 ഥ with a Known Value One-Sample t test ( 𝜎 is not known) for comparison of mean with a known value: 1. Our null hypothesis, 𝐻 𝑜 : 𝜇 = 𝜇𝑜 2. Form t statistic: 𝑥ҧ − 𝜇𝑜 𝑡= 𝑠 𝑁 3. State alternative hypothesis Ha and determine rejection region a. Ha : (𝜇 ≠ 𝜇𝑜 ) reject Ho if t ≥ tcrit or if t ≤ −tcrit b. Ha : (𝜇 > 𝜇𝑜 ) reject Ho if t ≥ tcrit c. Ha : (𝜇 < 𝜇𝑜 ) reject Ho if t ≤ −tcrit T-test: Comparing experimental 𝒙 ഥ with a Known Value Degrees of Freedom for comparing experimental mean with a known value: 𝑁1 − 1 Exercise: Comparing experimental 𝒙 ഥ with a Known Value A new procedure for the rapid determination of sulfur in kerosenes was tested on a sample known from its method of preparation to contain 0.123% S ( 𝜇𝑜 = 0.123% S). The results for % S were 0.112, 0.118, 0.115, and 0.119. Do the data indicate that there is a bias in the method at the 95% confidence level. 𝑥ҧ − 𝜇𝑜 0.116% − 0.123% Ho : 𝜇 = 𝜇 𝑜 𝑡= = = −4.375 𝑠 0.0032% 𝑁 4 Ha : 𝜇 ≠ 𝜇𝑜 a. At 95% CI, t (−4.375) ≤ −3.18. Ho is rejected. 𝑥ҧ is significantly different than known value. Method is bias (bias = 𝜇 − 𝜇𝑜 ). T-test: Comparing two experimental means You can use t-test to know whether a difference in the means of two sets of data. Ex: The results of two chemical analyses are used to determine whether two analytical methods give the same values or whether two analysts using the same methods obtain the same means. You can compare two sets of data by: 1. Independent or Two-sample t-test - for comparing differences in two means. It uses modified z-test to account a comparison of two data sets. 2. Paired t-test - for comparing two methods using the same samples by focusing on differences of each pair. T-test: Comparing two experimental means Two sample t-test for the Differences In Means of Two set of data 1. Our null hypothesis, 𝐻 𝑜 : 𝜇 = 𝜇𝑜 2. Form t statistic: 𝑥ҧ1 − 𝑥ҧ2 𝑡= 𝑁1 + 𝑁2 𝑠𝑝𝑜𝑜𝑙𝑒𝑑 𝑁1 + 𝑁1 − 2 𝑁1 𝑁2 3. State alternative hypothesis Ha and determine rejection region a. Ha : (𝜇 ≠ 𝜇𝑜 ) reject Ho if t ≥ tcrit or if t ≤ −tcrit b. Ha : (𝜇 > 𝜇𝑜 ) reject Ho if t ≥ tcrit c. Ha : (𝜇 < 𝜇𝑜 ) reject Ho if t ≤ −tcrit T-test: Comparing two experimental means Degrees of Freedom for comparing two experimental means: 𝑁1 + 𝑁1 − 2 T-test: Comparing two experimental means Based on six analyses, the average content of a wine A was established to be 12.61% ethanol. Four analyses of the wine B gave a mean of 12.53% alcohol. The 10 analyses yielded a pooled standard deviation spooled = 0.070%. Do the data indicate a difference between the wines at 95% CL? 𝑥ҧ1 − 𝑥ҧ2 12.61 − 12.53 Ho : 𝜇 = 𝜇 𝑜 𝑡= = = 1.771 𝑁1 + 𝑁1 6+4 Ha : 𝜇 ≠ 𝜇𝑜 𝑠𝑝𝑜𝑜𝑙𝑒𝑑 0.070 𝑁1 𝑁2 6×4 Degrees of freedom: 6 + 4 – 2 = 8 At 95% CI, tcrit = 2.31. We do not reject/we failed to reject Ho. There no is significant difference between the two wine sample. Paired t-test Can be used to compare two methods that are used to analyze the same samples. Measurement on the same sample are done in pairs, i.e. sample is analyzed by both method A and method B. Uses the same type of procedure as the normal t-test except that we analyze pairs of data by computing the differences, di. Test statistic value is 𝑑ҧ − ∆𝑜 𝑡= 𝑠 𝑑 𝑁 𝑑ҧ is the average difference = Σ𝑑𝑖 /𝑁 ∆𝑜 specific value of the difference, often 0 Paired t-test Test statistic value is 𝑑ҧ − ∆𝑜 𝑡= 𝑠 𝑑 𝑁 𝑑ҧ is the average difference of values in method 1 and 2 = Σ𝑑𝑖 /𝑁 ∆𝑜 specific value of the difference, often zero (0) Square of the sum of 𝑁 Summation of square of 𝑁 2 ( 𝑖=1 𝑑𝑖 )2 σ differences differences σ𝑖=1 𝑑𝑖 − 𝑠𝑑 = 𝑁 𝑁−1 Paired t-test A new automated procedure for determining glucose in serum (Method A) is to be compared to the established method (Method B). Both methods are performed on serum from the same six patients in order to eliminate patient-to-patient variability. Do the following results confirm a difference in the two methods at the 95% confidence level? P1 P2 P3 P4 P5 P6 Method A 1044 720 845 800 957 650 Method B 1028 711 820 795 935 639 Paired t-test P1 P2 P3 P4 P5 P6 sum Method A 1044 720 845 800 957 650 - Method B 1028 711 820 795 935 639 - Difference, di 16 9 25 5 22 11 88 d i2 256 81 625 25 484 121 1592 Average difference Σ𝑑𝑖 88 𝑑ҧ = = = 14.67 𝑁 6 σ𝑁 2 2 ( 𝑑 𝑖=1 𝑖 ) 882 Standard deviation σ𝑁 𝑖=1 𝑑𝑖 − 1592 − 𝑠= 𝑁 = 6 = 7.76 𝑁−1 6−1 Paired t-test Hypothesis: Ho : 𝜇 = Δ𝑜 = 0 Ha : 𝜇 ≠ Δ𝑜 𝑑ҧ − ∆𝑜 14.46 − 0 t statistic: 𝑡 = 𝑠𝑑 = 7.76 = 4.628 𝑁 6 At 95% CI, tcrit for N=6 is 2.57. We reject Ho. There is significant difference between the two methods. Comparison of Variances: F-test F-test is used to compare the variances (or standard deviations) of two data sets. You can compare whether there is difference in precision. The F test could also be used in comparing more than two means. The test statistic F, which is defined as the ratio of the two sample variances (F = s12 / s22), is calculated and compared with the critical value of F at the desired significance level. The null hypothesis is rejected if the test statistic differs too much from unity. Hypothesis: Ho : 𝜎1 2 = 𝜎2 2 Ha : 𝜎1 2 ≠ 𝜎2 2 The larger variance always appears in the numerator. Comparison of Variances: F-test To find fcrit: Look where DOF (Nx -1) of numerator and denominator intersects. Comparison of Variances: F-test A standard method for the determination of the carbon monoxide (CO) level in gaseous mixtures is known from many hundreds of measurements to have a standard deviation of 0.21 ppm CO. A modification of the method yields a value for s of 0.15 ppm CO for a pooled data set with 12 degrees of freedom. A second modification, also based on 12 degrees of freedom, has a standard deviation of 0.12 ppm CO. Is either modification significantly more precise than the original? Original Method: s= 0.21 ppm (if s is good estimate of 𝜎, DOF is ∞) Modifications: A , s= 0.15 ppm, DOF = 12 B, s= 0.12 ppm, DOF = 12 Comparison of Variances: F-test Original Method: s = 0.21 ppm Modifications: A , s = 0.15 ppm, DOF = 12 B, s = 0.12 ppm, DOF = 12 F statistic Original Method vs. Mod A F1 = s12 / s22 = 0.212 / 0.152 = 1.96 At 95% CL, Fcrit = 2.30 (DOF of numerator is ∞, DOF of denominator is 12). We do not reject Ho. Original method and Mod A has no significant difference in precision. Comparison of Variances: F-test Original Method: s = 0.21 ppm Modifications: A , s = 0.15 ppm, DOF = 12 B, s = 0.12 ppm, DOF = 12 F statistic Original Method vs. Mod B F1 = s12 / s22 = 0.212 / 0.122 = 3.06 At 95% CL, Fcrit = 2.30 (DOF of numerator is ∞, DOF of denominator is 12). We reject Ho. Original method and Mod B has a significant difference in precision. GRAVIMETRIC METHODS OF ANALYSES LEARNING OUTCOMES At the end of this activity, you must be able to: ✓ Perform gravimetric Methods of analyses ✓ Use gravimetric calculations in a given set of data INTRODUCTION TO GRAVIMETRY ❑ Gravimetry pertains to a weight measurement. ❑ Gravimetric analysis is a set of analytical methods for the quantitative determination of an analyte based on the mass of the solid. ❑ Gravimetric methods are quantitative methods that are based on determining the mass of a pure compound to which the analyte is chemically related. USING MASS AS AN ANALYTICAL SIGNAL ❑ Direct analysis – the analyte is the species that is being weighed. Example: determining the total suspended solids in the water released by a sewage-treatment facility. ❑ Indirect analysis – determining the analyte using a signal that is proportional to its disappearance. Example: determining a food’s moisture content by weighing the sample of food before and after we heat it and use the change in its mass to determine the amount of water originally present. TYPES OF GRAVIMETRIC ANALYSES ❑ Precipitation gravimetry – the analyte is separated from a solution of the sample as a precipitate and is converted to a compound of known composition that can be weighed. Example: Weighing the sample Addition of a Filtration Weighing the precipitant dry precipitate TYPES OF GRAVIMETRIC ANALYSES ❑ Volatilization gravimetry – the analyte is separated from other constituents of a sample by converting it to a gas of known chemical composition. The mass of the gas then serves as a measure of the analyte concentration. Example: Initial weighing of the Final weighing of the Mass difference = sample sample Mass of analyte Analytical Sciences Digital Library Volatilization of analyte TYPES OF GRAVIMETRIC ANALYSES ❑ Electrogravimetry – the analyte is separated by deposition on an electrode by an electrical current. The mass of this product then provides a measure of the analyte concentration ❑ Two other types of analytical methods are based on mass: ❑ Gravimetric titrimetry – the mass of a reagent of known concentration required to react completely with the analyte provides the information needed to determine the analyte concentration. ❑ Atomic mass spectrometry - uses a mass spectrometer to separate the gaseous ions formed from the elements making up a sample of matter. The concentration of the resulting ions is then determined by measuring the electrical current produced when they fall on the surface of an ion detector. GRAVIMETRIC ANALYSES CONSIDERATIONS Ideally, a gravimetric precipitating agent should: ❑ react specifically – reacts only with a single chemical species. ❑ Or at least selectively – react with a limited number of species. The ideal precipitating reagent would react with the analyte to give a product that is: ❑ easily filtered and washed free of contaminants; ❑ of sufficiently low solubility that no significant loss of the analyte occurs during filtration and washing; ❑ unreactive with constituents of the atmosphere; ❑ of known chemical composition after it is dried or, if necessary, ignited PARTICLE SIZE AND FILTERABILITY OF PRECIPITATES Factors That Determine the Particle Size of Precipitates ❑ Colloidal suspensions – tiny particles are invisible to the naked eye (10−7 to 10−4 cm in diameter). Colloidal particles show no tendency to settle from solution and are difficult to filter. ❑ Crystalline suspension – temporary dispersion of such particles in the liquid phase. Particles with dimensions on the order of tenths of a millimeter or greater. Solution Colloidal Suspension Crystalline Suspension 10¯⁷ to 10¯⁴ cm > 10¯⁴ cm PARTICLE SIZE AND FILTERABILITY OF PRECIPITATES Particle size of a precipitate is influenced by precipitate solubility, temperature, reactant concentrations, and the rate at which reactants are mixed. The net effect of these variables can be accounted for, at least qualitatively, by assuming that the particle size is related to a single property of the system called Relative Supersaturation. 𝑸−𝑺 Relative supersaturation = 𝑺 Where, Q = concentration of the solute at any instant S = equilibrium solubility ❑ when (Q - S)/S is large, the precipitate tends to be colloidal, and ❑ when (Q - S)/S is small, a crystalline solid is more likely. CONTROLLING PARTICLE SIZE Supersaturation can be minimized through the following experimental variables: ❑ elevating temperature (↑S) ❑ dilute solutions (↓Q) ❑ slow addition of precipitating agent (↓Q) ❑ pH control, provided that solubility depends on pH PRECIPITATE FORMATION MECHANISM ❑ In nucleation, a few ions, atoms, or molecules (perhaps as few as four or five) come together to form a stable solid. Often, these nuclei form on the surface of suspended solid contaminants, such as dust particles. ❑ Crystal/particle growth – enlargement process of the particles. Nucleus grows by deposition of particles precipitate onto the nucleus and forms a crystal of a specific geometric shape. Involves two steps: diffusion of ion to surface and deposition on surface. PURITY OF PRECIPITATES A precipitate must be free from impurities. Because precipitation usually occurs in a solution that is rich in dissolved solids, the initial precipitate often is impure. To avoid a determinate error, we must remove these impurities before we determine the precipitate’s mass. Possible sources of impurities: ❑ Coprecipitation ❑ Post-precipitation COPRECIPITATION AND POST-PRECIPITATION Coprecipitation – occurs when otherwise soluble compounds are removed from the solution during precipitate formation Examples of coprecipitation: ❑ a. inclusion or mixed-crystal formation, ❑ b. occlusion and mechanical entrapment. ❑ c. surface adsorption, COPRECIPITATION AND POST-PRECIPITATION Post-precipitation – inclusion of foreign compound precipitates on top of the desired precipitate. Example: post-precipitation of magnesium oxalate occurs if a precipitate of calcium oxalate is allowed to stand for too long before being filtered. SURFACE ADSORPTION Adsorption is a common source of coprecipitation and is likely to cause significant contamination of precipitates with large specific surface areas, that is, coagulated colloids. Coagulation of a colloid does not significantly decrease the amount of adsorption because the coagulated solid still contains large internal surface areas that remain exposed to the solvent. The coprecipitated contaminant on the coagulated colloid consists of the lattice ion originally adsorbed on the surface before coagulation plus the counter-ion of opposite charge held in the film of the solution immediately adjacent to the particle. The net effect of surface adsorption is, therefore, the carrying down of an otherwise soluble compound as a surface contaminant. MINIMIZING ADSORBED IMPURITIES ON COLLOIDS Digestion – water is expelled from the solid to give a denser mass that has a smaller specific surface area for adsorption. Reprecipitation – A drastic but effective way to minimize the effects of adsorption. In this process, the filtered solid is redissolved and reprecipitated. The first precipitate usually carries down only a fraction of the contaminant present in the original solvent. Thus, the solution containing the redissolved precipitate has a significantly lower contaminant concentration than the original, and even less adsorption occurs during the second precipitation. Reprecipitation adds substantially to the time required for analysis. MIXED-CRYSTAL FORMATION In mixed-crystal formation, one of the ions in the crystal lattice of a solid is replaced by an ion of another element. For this exchange to occur, it is necessary that the two ions have the same charge and that their sizes differ by no more than about 5%. Furthermore, the two salts must belong to the same crystal class. For example, barium sulfate formed by adding barium chloride to a solution containing sulfate, lead, and acetate ions is found to be severely contaminated by lead sulfate. This contamination occurs even though acetate ions normally prevent precipitation of lead sulfate by complexing the lead. In this case, lead ions replace some of the barium ions in the barium sulfate crystals. Other examples of coprecipitation by mixed-crystal formation include MgKPO4 in MgNH4PO4, SrSO4 in BaSO4, and MnS in CdS. OCCLUSION AND MECHANICAL ENTRAPMENT Occlusion is a type of coprecipitation in which a compound is trapped within a pocket formed during rapid crystal growth. Mechanical entrapment occurs when crystals lie close together during growth. Several crystals grow together and in so doing trap a portion of the solution in a tiny pocket A mixed-crystal formation may occur in both colloidal and crystalline precipitates, but occlusion and mechanical entrapment are confined to crystalline precipitates. STEPS IN GRAVIMETRIC ANALYSIS Preparation of Precipitation Digestion Filtration the Solution Drying or Washing Weighing Calculation Ignition PREPARATION OF THE ANALYTE SOLUTION ❑ Steps in preparing analyte solution 1. sampling; bulk representation 2. Preparing analyte solution ❑ May require: ❑ Preliminary separation to separate potential interferences ❑ Adjustment on the conditions of the solutions (pH, temperature, volume, concentration) to maintain low solubility of precipitate and maximize precipitate formation. ❑ Some require treatments with HCl, HNO3, Aqua regia, or fusing with basic flux. But most are readily solute in water. PRECIPITATION PROCESS Analyte + Precipitating agent → Supersaturation → Precipitation Steps involved in Precipitation 1. Nucleation 2. Crystal growth If: Rate of nucleation > Rate of crystal growth; Precipitate containing a large number of small particles Rate of nucleation < Rate of crystal growth; Precipitate containing a smaller number of large crystals Precipitates with large particles are more easily handled during filtration and washing. PRECIPITATE DIGESTION ❑ Precipitate digestion – a process of heating the precipitate (without stirring) for an hour or more in the solution from which it was formed (mother liquor). ❑ The process tends to dissolve small particles and reprecipitate on the surface of the larger particles/crystals. Thus, larger and purer particles are produced. It also reduces surface contamination and reduces crystal imperfection. ❑ In the process, water is expelled from the solid to give a denser mass that has a smaller specific surface area for adsorption. FILTRATION ❑ The choice of medium for filtration depends on the precipitate’s nature, the medium’s cost, and the heating temperature required for drying. ❑ Filtration media used are: ❑ Ashless filter paper – leaves very little ash when it is burnt; usually filters crystalline precipitates. ❑ Sintered glass – can stand up to 500⁰C temperature; usually filters curdy precipitates ❑ Sintered porcelain – can stand up to 1000⁰C temperature WASHING OF PRECIPITATES ❑ Washing – is done to remove adsorbed impurities and the mother liquor. ❑ For crystalline precipitates: Impurities can be removed by several rinsings with a small amount of pure water. ❑ For coagulated colloids: Commonly washed with an electrolyte solution. Washing coagulated colloids with pure water may lead to the dilution and removal of foreign ions and the counter ions will occupy a larger volume. The charge of the primary ions repels each other and will revert back to the colloidal form. Such process is called peptization and results in the loss of colloidal precipitate through the filter. DRYING OR IGNITION OF PRECIPITATES ❑ Drying or ignition – removes the solvent(s) and any other electrolytes or any volatile species carried down with the filtered precipitate. Done by heating at 110 to 120⁰C for one to two hours. ❑ After the drying and ignition process, the precipitates are cooled down to room temperature in a desiccator before weighing. WEIGHING ❑ Weighing of precipitates – after the precipitates are cooled in a desiccator, it is weighed with the crucible. The process of heating, cooling, and weighing is repeatedly done until a constant weight is obtained. Whereas, the difference between the last two consecutive weighings is below 0.001 g. SAMPLE PROBLEM The calcium in a 200.0-mL of a natural water was determined by precipitating the cation as CaC2O4. The precipitate was filtered, washed, and ignited in a crucible with an empty mass of 26.6002 g. The mass of the crucible plus CaO (56.077 g/mol) was 26.7134 g. Calculate the concentration of Ca (40.078 g/mol) in water in units of grams per 100 mL of the water. SAMPLE PROBLEM An iron ore was analyzed by dissolving a 1.1324-g sample in a concentrated HCl. The resulting solution was diluted with water, and the Iron (III) was precipitated as the hydrous oxide Fe2O3 xH2O by the addition of NH3. After filtration and washing, the residue was ignited at a high temperature to give a 0.5394 g of pure Fe2O3 (159.69 g/mol). Calculate (a) the %Fe (55.847 g/mol) and (b) the %Fe3O4 (231.54 g/mol) in the sample. SAMPLE PROBLEM The combined constant factors in a gravimetric calculation are referred to as the gravimetric factor. When the gravimetric factor is multiplied by the mass of the weighed substance, the result is the mass of the sought for substance GRAVIMETRIC DETERMINATION OF SO 3 IN A SOLUBLE SULFATE GENERAL PROCEDURE Filtration and Ignition, Drying, Preparation of Precipitation washing of and Weighing of Sample precipitate sample Drying of sample Heating of Filtration of Oven drying of sample near precipitate using precipitate Dissolution of boiling. ashless filter sample in water paper. Perform and addition of Addition of constant conc. HCl excess BaCl2 to Washing with weighing allow solvent to procedure. precipitation. remove impurities. EXPERIMENTAL SO3 CONTENT OF SAMPLE STANDARDIZATION OF NaOH Calculated %SO3 content of sulfate sample. Wt. of Sample wt. precipitate Mass SO3 %SO3 1 0.6290 g 0.8252 g 0.2831 g 45.00% 2 0.7936 g 1.0454 g 0.3586 g 45.19% 3 0.7415 g 0.9128 g 0.3131 g 42.23% Average 44.14% SD 1.66 RSD (ppt) 37.56 CALCULATION FOR MASS OF SO3 STANDARDIZATION OF NaOH Dimensional analysis: 1 𝑚𝑜𝑙 𝐵𝑎𝑆𝑂4 80.06 𝑔 𝑤𝑡. 𝐵𝑎𝑆𝑂4 𝑔 × × 233.38 𝑔 1. 𝑚𝑜𝑙 𝑆𝑂3 % SO3 w/w = × 100% 𝑤𝑡. 𝑠𝑎𝑚𝑝𝑙𝑒 (𝑔) Sample calculation: Trial 1 1 𝑚𝑜𝑙 𝐵𝑎𝑆𝑂4 80.06 𝑔 0.8252 𝑔 × × 233.38 𝑔 1. 𝑚𝑜𝑙 𝑆𝑂3 % SO3 w/w = × 100% = 45.00% 0.6290 𝑔 CALCULATION OF %SO3 (w/w) OF SAMPLE Identity of Sulfate Sample: Na2SO4 Theoretical %SO3 content of sulfate sample. Wt. of Sample Mass SO3 %SO3 1 0.6290 g 0.3546 g 56.37% 2 0.7936 g 0.4474 g 56.37% 3 0.7415 g 0.4180 g 56.37% Average 56.37% THEORETICAL SO3 CONTENT OF SAMPLE Dimensional analysis: 1 𝑚𝑜𝑙 𝑁𝑎2 𝑆𝑂4 1 𝑚𝑜𝑙 𝐵𝑎𝑆𝑂4 1 𝑚𝑜𝑙 𝑆𝑂3 80.06 𝑔 𝑤𝑡. 𝑁𝑎2 𝑆𝑂4 𝑔 × × × × 142.04 𝑔 1 𝑚𝑜𝑙𝑁𝑎2 𝑆𝑂4 1 𝑚𝑜𝑙 𝐵𝑎𝑆𝑂4 1. 𝑚𝑜𝑙 𝑆𝑂3 % SO3 w/w = × 100% 𝑤𝑡. 𝑠𝑎𝑚𝑝𝑙𝑒 (𝑔) Sample calculation: Trial 1 1 𝑚𝑜𝑙 𝑁𝑎2 𝑆𝑂4 1 𝑚𝑜𝑙 𝐵𝑎𝑆𝑂4 1 𝑚𝑜𝑙 𝑆𝑂3 80.06 𝑔 0.6290 𝑔 × × × × 142.04 𝑔 1 𝑚𝑜𝑙𝑁𝑎2 𝑆𝑂4 1 𝑚𝑜𝑙 𝐵𝑎𝑆𝑂4 1. 𝑚𝑜𝑙 𝑆𝑂3 % SO3 w/w = × 100% = 56.37% 0.6290 𝑔 Exercise Calculate the mass SO3 and %SO3 in the sample given the data in the table below. REFERENCES Brown et. al (2019) Chemistry – The Central Science. Retrieved from https://chem.libretexts.org/Bookshelves/General_Chemistry/Map%3A_Chemistry_- _The_Cen tral_Science_(Brown_et_al.). Harvey, D. (2016). Analytical Chemistry 2.1. DePauw University. Retrieved from https://chem.libretexts.org/Bookshelves/Analytical_Chemistry/Book%3A_Analytical_C hemistr y_2.1_(Harvey) Alternative site: https://open.umn.edu/opentextbooks/text books/analytical- chemistry-2-1. Skoog, Douglas A., West, Donald M., Holler, F. James, Crouch, Stanley R.. (2014). Fundamentals of Analytical Chemistry (Ed. 9th). Singapore: Cengage Learning.