Main Manuscript JCAL.docx
Document Details
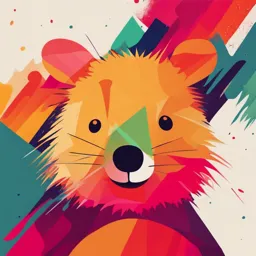
Uploaded by EnviableDiopside
Majmaah University
Tags
Related
- Chapter 8 - Cloud Computing 2023 PDF
- Unit 01 - Introduction to Cloud Computing and Cloud Service Models.pdf
- Chapter 10 - 02 - Understand Cloud Computing Fundamentals - 01_ocred.pdf
- Systems Development 2B (HSYD202-1) Summative Assessment 2 (PDF)
- NCP-CI-AWS Certification Preparation Guide PDF
- Grade 8 Cloud Computing Questions PDF
Full Transcript
**Academics' Use of Cloud Computing in Higher Education:** **Reconceptualising UTAUT via Structural Equation Modelling** ***Dr. Faisal Alshammari*** ***Department of educational sciences, College of education, Majmaah University, Al-Majmaah, 11952, Saudi Arabia.*** ***School of Education, Univer...
**Academics' Use of Cloud Computing in Higher Education:** **Reconceptualising UTAUT via Structural Equation Modelling** ***Dr. Faisal Alshammari*** ***Department of educational sciences, College of education, Majmaah University, Al-Majmaah, 11952, Saudi Arabia.*** ***School of Education, University of Queensland, Brisbane, Queensland, Australia*** [***f.alshammari\@mu.edu.sa***](file:///%5C%5CUXENSVR%5C%7bFD34A37F%7d%5CEXT%5CYR%[email protected]) ***Dr. Vicente Reyes*** ***Faculty of Social Sciences, The University of Nottingham, Nottingham, UK*** [***Vicente.Reyes\@nottingham.ac.uk***](file:///%5C%5CUXENSVR%5C%7bFD34A37F%7d%5CEXT%5CYR%[email protected]) ***Dr Kate McLay*** ***School of Education, University of Queensland, Brisbane, Queensland, Australia*** [***k.mclay1\@uq.edu.au***](file:///%5C%5CUXENSVR%5C%7bFD34A37F%7d%5CEXT%5CYR%[email protected]) Abstract Cloud computing apps have become increasingly widely used in recent years, including in higher education settings. The range of benefits offered by cloud apps, including enhanced communication, collaboration, and engagement between students and universities, has seen many tertiary institutions partner with global technology corporations such as Microsoft and Google, opening up new possibilities for teaching and learning. However, despite the important role of academics in deploying this technology (e.g., Cloud apps) in higher education, the voice of academics is still missing in the educational context. Further, there are few cloud computing acceptance studies that explore faculty members\' voices and perspectives in Saudi universities. This inquiry sought to determine and understand the factors that influence academics; behavioural intention to deploy Cloud Apps in Saudi Arabia. A cross-sectional online survey was undertaken on all Saudi public universities, generating responses from 460 faculty members. The Unified Theory of Acceptance and Use of Technology 2 (UTAUT2) model of Venkatesh was reconceptualised, adding three additional variables to better understand academics\' behaviour and use of cloud technology. Results indicate that performance expectancy, effort expectancy, hedonic motivation and perceived security were all statistically significant predictors of faculty members\' behavioural intentions to use cloud computing apps in higher education. Keywords: Cloud Computing, Higher Education, UTAUT2, Structural Equation Modelling, Technology Adoption Introduction The rapid growth and diffusion of information technology in educational settings has seen simultaneous, widespread development and take-up of e-learning across all sectors. For example, in higher education, wireless and mobile technologies have increasingly afforded collaboration -- students can interact and learn together even when separated by geographical distance (Lee, 2012; El-Masri & Tarhini, 2017). In particular, the collaboration and convenience afforded by cloud computing can support student engagement and contribute to improved educational outcomes (Mousannif et al., 2013) because users no longer need to be "married" to hardware to save and retrieve information and files (Dawson, 2015). According to James and Weber (2016), cloud computing is reasonably priced compared to the costs of hardware, system support, and maintenance in the (tertiary) higher education sector, and potential and efficiency increase when cloud computing is utilised (OECD, 2016). The cloud can combine with any course management system to provide a robust method for sharing information among students (Abrams, 2012). In the United States, the National Institute of Standards and Technology (NIST) defined cloud computing as: a model for enabling ubiquitous, convenient, on-demand network access to a shared pool of configurable computing resources (e.g., networks, servers, storage, applications, and services) that can be rapidly provisioned and released with minimal management effort or service provider interaction (Mell & Grance, 2011, p. 2). This inquiry focuses on cloud apps that operate in Saudi universities, such as Google Cloud and Office 365. Since most of the cloud services are linked to software applications (SaaS) such as Microsoft Office applications and Google Cloud, this inquiry will focus on these forms of technology. This focus involves educational technology research that supports and promotes engagement and provides meaningful learning (Howland et al., 2013). This article focuses on the technology of cloud computing applications and the problematisation of factors that influence behavioural intention for using cloud computing apps. Literature Review Cloud Computing in Education Cloud computing has the potential to offer various benefits to education. From aiding in knowledge acquisition to promoting pedagogical and administrative advancements, this application could unleash tremendous growth and generate encouraging results in a diverse range of fields. It can promote and develop performance in educational subjects such as mobile learning, collaborative learning, 21st-century skills, creativity and solving problems (Edwards & Baker, 2010; Ishihara & Ham, 2012; Rao et al., 2012; Meepian & Wannapiroon, 2013; Belcher, 2014; Kongrugsa et al., 2016). It offers secure data space, advantageous and user-friendly internet services, and a powerful e-learning computing infrastructure (El-Sofany et al., 2019). There are many studies investigating mobile learning and cloud computing. This mode of teaching and learning provides real-time online interaction in a series of short-burst learning activities. For example, Tam et al. (2016) found that students learn more effectively when incorporating cloud technologies in dynamic simulations of computer systems on mobile devices. Jansen et al. (2013) have pointed out that cloud computing is often used to provide solutions for some of the limitations of technical hardware, such as mobile, desktop, or server systems, especially in expanding accessibility and interoperability. Similarly, Wang and Wu (2016) suggest that mobile cloud computing (MCC) can relieve complex computational tasks for students by pairing mobile devices with the cloud. For this reason, contemporary young people have an optimistic attitude towards the use of mobile apps in their daily lives as well as in their learning. These positive attitudes come from countless experiences with communication and interaction tasks, searching and checking for learning and reference materials, and information sharing (Wai et al., 2016). Technology and Education in Saudi Arabia Saudi Arabia is one of the largest markets for communication and technical information in the Middle East. Baldassarre et al. (2018) reviewed articles about cloud computing published from 2014 to 2015 and found that Saudi Arabia ranked fifth in the world in terms of interest in this area (Figure 1). Figure 1: Number of publications about cloud computing by country (Baldassarre et al., 2018) The government of Saudi Arabia has invested up to 217 billion Saudi Riyals (AUD 81.6 billion) into higher (tertiary) education and workforce training. This represents about a quarter (25%) of the approved national budget (GOV.SA, 2020) and reflects the government's concern for improving academic outcomes. This financial investment includes tech tools such as learning management systems (LMSs), cloud computing apps and other digital equipment. Despite the financial investment, the sector of higher education in Saudi Arabia faces a number of challenges. First, education in Saudi Arabia has arguably not focused enough on developing students' 21^st^-century skills, for example, critical thinking, asking questions, and developing knowledge (Rury & Tamura, 2019). Therefore, many students feel that education is uninteresting, and as a result, dissatisfaction rates are increasing (Alshahrani & Ally, 2016). In the context of this article, the availability of various government-supported technologies does not necessarily mean that users will deploy these technologies for teaching and learning. Investing in technology that is underused is not financially viable. Furthermore, as Koehler and Mishra (2009) pointed out, using digital equipment and devices, such as digital projectors, SMART boards, podiums, and other devices, does not guarantee efficient instruction and learning. In the same way, the history of employing technologies has proven to be non-linear, as technologies are influenced by societal contexts and circumstances (Selwyn, 2019). Therefore, there is a need to understand the factors that influence users to deploy technology in higher education contexts. The anticipated outcomes of this article can include strategies for implementing these technologies in higher education in Saudi Arabia. For example, educational technology, educational leadership, educational policy, improvement of curricula, improvement of the capabilities of the beneficiaries of educational services and other contextual matters can be part of possible solutions (Reyes, 2015; Henderson et al., 2015; Hong et al., 2020). Cloud apps in Saudi Arabia There is a lack of research in universities in Saudi Arabia regarding the factors that affect the use of cloud computing in education in terms of faculty members' perceptions. No present research investigates academics' opinions about the significant factors that impact their behavioural intentions regarding implementing cloud apps in higher education. This inquiry outlines this gap in the review of the relevant literature and then conducts research using quantitative and qualitative methods to understand what the factors are that influence academics and why. It is hoped that this approach will support the understanding of cloud computing apps used by faculty members and contribute to improved cloud computing apps designed for use in learning and instruction in Saudi education. This greater understanding of academics' use of cloud computing tools, combined with improved cloud apps developed in response to this understanding, may enhance learning outcomes. Studies of the adoption of technology in education are important because they help us understand how to provide technology in an optimal and meaningful way to meet individuals' needs (IRMA, 2019). Understating these higher education environments from faculty members' perspectives may help public university administrations and decision-makers to implement more effective and responsive initiatives designed to harness the advantages of cloud computing in higher education. In recent decades, the subject of the adoption of cloud computing by organisations has been of significant interest to researchers. Although this subject has been widely explored, little research has focused on Saudi Arabia specifically. As illustrated in Table 1, most research has found that the major factors associated with the adoption of cloud technology were usefulness, ease of use, motivation, issues of security and quality of service. It is noteworthy that most of the contributions to research on cloud computing covered the sectors of health, information technology and the economy. In contrast, this study focuses on the higher education context and the voices of academic staff in order to help decision-makers, authorities, and other researchers obtain information to better implement cloud applications. To address the stated problems and achieve the study's research aims, specific research questions were identified. ***Research Question 1: What are the factors that influence faculty members' behavioural intention to use cloud applications in higher education in Saudi Arabia?*** In addressing this overarching research question, this study will interrogate the theoretical components of UTAUT2, which looks at system features (i.e., performance expectancy, effort expectancy, social influence, facilitating conditions and hedonic motivation), speed of the internet) as well as external factors (i.e., security, speed of the internet and awareness). Moreover, this study will examine the extent of moderating variables (i.e., gender, type of university, and experience of higher education) influences between these factors and the behavioural intention to use cloud applications in higher education in Saudi Arabia. ***Research Question 2: What are the dimensions that underpin the significant factors that influence faculty members' behavioural intentions to use cloud applications in education in Saudi Arabia?*** Theoretical Framework and Hypotheses Cloud technology in the context of university teaching in the Saudi higher education environment has not been adequately addressed by current research. Therefore, this study will focus on the acceptance of the adoption of cloud technology in university teaching from the point of view of academics in Saudi Arabia. This research adopts the conceptual framework of the Unified Theory of Acceptance and Use of Technology (second version) (UTAUT2), and incorporates relevant extensions (i.e., factors specific to Saudi culture). As this study is foregrounded in Saudi higher education, this inquiry recognises many longstanding issues among academics in Saudi Arabia. The current study reports measurement models that examine a slight variation in UTAUT2, first via the baseline model (determinants only) and then with the addition of relevant cultural, demographic, education and work-related. As illustrated in Figure 3, the conceptual model added new constructs such as speed of internet, perceived security and awareness as predictors of the behavioural intention outcome. Control variables in this study were gender, type of university and experience of higher education. This exploration, therefore, considers a number of additional factors from previous studies that may potentially have a significant effect on the acceptance of cloud technology in Saudi universities. Figure 3: The conceptual model  ***Hypotheses*** The hypotheses tested in this inquiry have been classified into three: hypotheses of UTAUT2 components, external factors and moderating variables) ***UTAUT2 components*** *Behavioural Intention to Use Technology (BIU)* Behavioural intention is the most commonly measured dependent variable operationalised as an agent for technology use, supporting the strength of UTAUT as an explanatory model of technology tools acceptance and use (Khechine et al., 2016). Almaiah and Al-Khasawneh (2020) have specifically suggested that the potential factors influencing faculty members in the adoption of cloud services in Saudi universities should be carried out using UTAUT or TAM. *Performance Expectancy (PE)* Performance expectancy is the extent to which a person believes the use of technology will help them to realise their desired job performance. Performance expectancy is derived from perceived usefulness, job fit, extrinsic motivation, outcome expectation, and relative advantage (Lai, 2017). According to Venkatesh et al. (2003), it serves as the strongest predictor of intention to use technology. *H01: There is no significant relationship between performance expectancy and faculty members' behavioural intentions to use cloud applications in education.* *Effort Expectancy (EE)* Effort expectancy is the extent of ease of using the system. The construct centres on the notion of perceived complexity and ease of use. *H02: There is no significant relationship between effort expectancy and faculty members' behavioural intentions to use cloud applications in education.* *Social Influence (SocI)* Social influence is the extent to which an individual perceives the importance of others, believing that they should use the system. It is aligned with social status and personal image perception. According to Venkatesh et al. (2003), the social influence construct is not significant in voluntary contexts but critical in mandatory situations. *H03: There is no significant relationship between social influence and faculty members' behavioural intentions to use cloud applications in education.* *Facilitating Conditions (FC)* Facilitating Conditions is defined as the extent to which people believe that the technical infrastructure within their respective organisations can support their use of the system (Venkatesh, Thong, & Xu, 2012). The technological infrastructure in place in institutions is to support the various systems within such organisations. The uptake and use of a system are partly determined by the belief that the infrastructure in place can enhance the ease with which they can interact with the system, i.e., the facilitating conditions. This study defines this factor as the degree to which a faculty member believes that an organisational and technical infrastructure exists to support the use of cloud computing apps. *H04: There is no significant relationship between facilitating conditions and faculty members' behavioural intentions to use cloud applications in education.* *Hedonic Motivation (HM)* Hedonic motivation, therefore, could be a factor in the field of cloud technology. Hering (2018) has pointed out that hedonic motivation is significant because the cloud has significant flexibility of use. Venkatesh et al. (2012) indicate that hedonic motivation is an influencing factor at the beginning of the adoption of new technology but may fade over time. *H05: There is no significant relationship between hedonic motivation and faculty members' behavioural intentions to use cloud applications in education.* ***External Factors*** *Perceived Security (PS)* Perceived security is a factor introduced in this study that is not addressed by UTAUT2. Perceived security can be defined as the extent to which the user of a system believes that their use of a system or a form of technology is secure (Tella et al., 2020). This study defines perceived security as faculty members' perceptions of how they are protected from risks related to cloud computing apps in higher education. Perceived security covers different aspects of the use of a technological system---for example, the security of personal information and finances (Ahson & Ilyas, 2010; James & Weber, 2016; Marinescu, 2017). The present study included this extension based on the recommendation by Uyoma (2020) to add a security component as a significant factor in the consideration of cloud implementation. *H06: There is no significant relationship between perceived security and faculty members' behavioural intentions to use cloud applications in education.* *Speed of Internet (SpI)* Speed of the internet, or bandwidth, refers to the momentum with which data, information, or content moves from the World Wide Web to a technological device, such as a mobile phone, tablet or computer. Internet speed influences the use of different forms of technology (Costello, 2016). Usually, the speed of the internet is measured by kilobits per second (Kbps) or megabits per second (Mbps), while in fibre optic networks, it is measured by gigabytes or terabytes per second (Gbps, Tbps) (McCleary et al., 2020). In this study, this factor refers to whether internet speed affects preferences for using cloud computing apps by teaching staff in higher education environments. This study inserted this extension based on the recommendation for adding speed of internet as an element in cloud adoption by (Kayali & Alaaraj, 2020). *H07: There is no significant relationship between speed of internet and faculty members' behavioural intentions to use cloud applications in education.* *Technology Awareness (TA)* Awareness is a vital component of a human factor that can aid or hinder the implementation of technology adoption (Raed Alotaibi et al., 2016; Khosrow-Pour, 2005). A. Khan and Qudrat-Ullah (2021) considered user knowledge and awareness in the definition of innovation in the diffusion of innovation theory (DOI) of technology acceptance. Awareness of technology means context awareness and refers to environmental and situational information about things, people, and places in predicting their immediate needs. Awareness is critical in technology, as it leads to the provision of services that reflect real-life circumstances. Recent work on challenges in digital transformation in Saudi Arabia has established that awareness is one of the primary factors for implementing digital transformation (Alharbi, 2019). In the context of Saudi universities, Aljaber (2018) notes that there is a lack of awareness of e-learning services that must be addressed to take advantage of these technologies. This study defines awareness as the extent to which faculty members are conscious of implementing cloud computing apps in education. *H08: There is no significant relationship between technological awareness and faculty members' behavioural intentions to use cloud applications in education.* ***Moderator Variables*** *Gender, Type of University and Experience* The current study reports measurement models that examine a slight variation in UTAUT2, first via the baseline model (determinants only) and then with the addition of relevant cultural, demographic, education and work-related. The conceptual model added new constructs such as speed of internet, perceived security and awareness as predictors of the behavioural intention outcome, given that user behaviour was not available. This study also introduces moderator variables, namely Gender, Type of University and Experience of Higher Education. *H09:* *There is no significant relationship between these factors (performance expectancy, effort expectancy, social influence, facilitating conditions, hedonic motivation, speed of internet, perceived security and awareness) and behavioural intentions (outcomes) moderated by demographic information (gender, type of university and experience).* Research Methodology Population and Sampling The target population of this study was all faculty members (male and female) employed in any of the public universities in the Kingdom of Saudi Arabia. According to current statistical information about the population of academia from the Ministry of Education in the Kingdom of Saudi Arabia, there are 65,404 faculty members in Saudi public universities (MOE.SA, 2019). The minimum sample size required for this study is 382 participants. This means that 382 participants were needed to achieve 95% confidence in the results (E = 0.5 and z = 1.96), with the degree of accuracy D = 0.05. This research collected surveys from 888 faculty members. However, only 460 responses who willingly participated in the study were valid. This large sample size indicates a reliable result (Okes, 2009; May, 2011). Most of the respondents were native Arabic speakers; they received an Arabic copy of the questionnaire, which had been sent to expert translators to ensure clarity and avoid errors. The questionnaire was divided into two main sections. The first section collected data about the demographic characteristics of the target respondents. Demographic questions can be used to disaggregate data to look at congruence within divisions or units or across job titles. The second section was a Likert-based questionnaire measuring the respondents' views. Ethical approval was granted for this study by the University of Queensland for the Social Sciences. Moreover, ethical approval was granted by Saudi authorities. The majority of participants were male academics (61.5%), and the majority of participants in the study (50.2%) were 31--40 years of age. In aggregate, this research study collected data from all public universities, and these were categorised as either established or emerging. Of the 28 universities, 29.5% were established, and 70.5% were emerging universities, consistent with previous literature. In Saudi higher education, there are two kinds of faculty: Science colleges and Humanities colleges. Both types were involved in this research study, with the majority (62%) of participants from Humanities colleges. The majority of the participants (62%) had experience of working in higher education for five to ten years. The largest group of participants were lecturers (44%) and the second largest participants with a doctoral degree (40%). A majority of the participants (59%) reported having additional duties. Almost 26% lived outside the city in which they worked. Most participants (at least 80%) checked emails and social media (i.e., both types of internet usage) multiple times a day. Almost 60% of the participants confirmed that in their universities, Microsoft provided cloud computing services. About 50% of participants had used cloud applications for either 4--6 years (22.65%) or more than six years (31.84%). In contrast, about 23% had either never used cloud computing applications or had done so for less than a year. Table 1 provides a snapshot of the key demographic traits of the respondents. *Table 1. Respondents selected demographic profile* **Category** **Frequency** **Percentage** -------------------------------------- --------------- ---------------- ***Gender*** Male 283 61.5 Female 177 50.2 ***Age*** Less than 25 6 1.3 25-30 105 22.4 31-40 235 50.2 41-50 87 18.6 51-60 29 6.3 60 and above 6 1.3 ***Type of Universities*** Established 8 29.5 Emerging 20 70.5 ***Experience in Higher Education*** Less than 1 year 33 7.1 1-4 years 141 30.1 5-10 years 174 37.2 More than 10 years 120 25.6 Measurement As explained earlier, this study adopted most of the UTAUT2 items in the model developed by Venkatesh et al. (2012). The UTAUT2 model has been used to examine technology acceptance and utilisation in various areas. The validity and reliability of this model have been verified by studies in several disciplines (Khan, 2018; Arain et al., 2019; Nikolopoulou et al., 2020). Cloud computing apps are one of the new technologies implemented by academic staff in Saudi universities, and the UTAUT2 model was relevant in relation to exploring the acceptance and usage of cloud computing apps by academic staff. Since this study's conceptual model incorporates factors associated with Saudi culture and societal and institutional mores, it seems appropriate to test its validity and reliability in this setting. This view is supported by Creswell and Creswell (2017), who have stated that if a model has been restructured or merged with another model in any study, the validity and reliability of the new instrument cannot be assured and it becomes necessary during data analysis to re-examine validity and reliability. The conceptual model of this study includes five performance expectancy items, five effort expectancy items, five social influence items, five facilitating conditions items, four hedonic motivation items and five behavioural intention items. It also includes five-speed of internet items, five perceived security items and four awareness items. These were all measured at seven levels on a Likert-type scale. Participants were asked to choose from 7 = Strongly Agree, 6 = Moderately Agree, 5 = Slightly Agree, 4 = Neutral, 3 = Slightly Disagree, 2 = Moderately Disagree and 1 = Strongly Disagree. The results of the analysis showed that Cronbach's alpha ranged from.767 to.954, with all these values somewhat better than the minimum acceptable value of.600. Analysis and Findings Descriptive Analysis The descriptive statistics for each factor in the proposed research model are presented in Table 2. All means were greater than 4.65, which indicate that the majority of the participants express positive responses to the constructs that are measured in the study. Additionally, Cronbach's alpha scores indicated that all factors exhibited strong internal reliability. *Table 2. Descriptive Statistics of the Constructs* **Construct** **Mean** **SD** **Cronbach's ** --------------------------------- ---------- -------- ------------------ Performance Expectancy (PE) 6.25 1.00 0.91 Effort Expectancy (EE) 6.05 1.04 0.86 Social Influence (SocI) 5.09 1.52 0,77 Facilitating Conditions (FC) 5.73 1.25 0.77 Hedonic Motivation (HM) 5.61 1.30 0.94 Speed of Internet (SpI) 4.83 1.86 0.94 Perceived Security (PS) 5.22 1.46 0.92 Technology Awareness (TA) 4.70 1.71 0.93 Behavioural Intention Use (BIU) 6.15 0.99 0.95 **Measurement Model** As stated by Watters and Boslaugh (2008), the use of split-half methods to measure internal consistency requires that the dataset be split into two parts, with ideally two halves with equal numbers of cases. The sample dataset was split for more validation into two randomly assigned samples using SPSS. One sample (n = 234) was used to conduct exploratory factor analysis (EFA) with ProMax rotation. An exploratory factor analysis with Principal Axis Factoring (PAF) and a Promax rotation was conducted with one of the two split-half-sample (*N* = 324) datasets (see Costello & Osborne, 2005 for an explanation of PAF). Oblique rotation (Promax) was used because the factors were expected to be correlated. There were a number of indications that the data were suitable for factor analysis. The KMO measure of sampling adequacy was high (.92), and Bartlett's test of Sphericity was significant (*χ*^2^= 17383, *p*\