Building Resilience Against AI-Powered Cyber Threats Assignment
Document Details
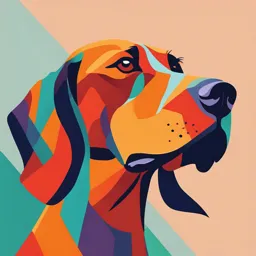
Uploaded by SuperbFarce
Madhavi Shettywarangale
Tags
Summary
This document is an assignment that discusses a cybersecurity strategy for handling AI-driven threats. It covers threat identification, assessment, and proactive measures.
Full Transcript
[Building Resilience Against AI-Powered Cyber Threats] **A cybersecurity team is tasked with developing a strategy to address the growing threat of AI-powered attacks.** 1. **What are the key components of a comprehensive cybersecurity strategy for AI-driven threats, and how can organisations...
[Building Resilience Against AI-Powered Cyber Threats] **A cybersecurity team is tasked with developing a strategy to address the growing threat of AI-powered attacks.** 1. **What are the key components of a comprehensive cybersecurity strategy for AI-driven threats, and how can organisations prioritise their efforts?** In today's digital age, cybersecurity has become a critical priority for organizations across the globe. As cyber threats continue to evolve in sophistication, traditional security measures are often no longer sufficient to keep attackers at bay. Enter Artificial Intelligence (AI) -- a game-changing technology that is reshaping the cybersecurity landscape by enabling organizations to stay one step ahead of malicious actors. GenAI products are evolving at lightning speed, and with them, the security landscape is changing too. New threats are emerging, old ones are shifting, and the risks are becoming harder to ignore While AI offers incredible opportunities, its misuse poses significant risks. The rise of AI-powered phishing highlights the need for a balanced approach---embracing innovation while prioritizing cybersecurity. Businesses and individuals must adapt quickly to this evolving threat landscape by adopting AI-driven defenses and maintaining a culture of cyber-awareness. The fight against AI-enhanced attacks has only begun, and staying informed is the first step toward protection. Cyberattacks are evolving rapidly, but so is the technology to stop them. Orgs are harnessing AI to analyze massive datasets, detect hidden patterns, and enable proactive responses---helping both our systems and organizations around the world stay ahead of even the most sophisticated threats. AI is transforming cybersecurity by shifting it from reactive defense to proactive strategy. With smarter tools and actionable insights, our security teams can predict, detect, and respond to attacks with precision, shaping a safer digital future. ------------------------------------------------------------------------------------------------------------------------------------------------------------------------------------------------------------------------------------------------------------------------------------------------------------------------------------------------------------------------------------------------------------------------------------------------------------------------------------------------------------------------------------------------------------- The Importance of Proactive Cybersecurity Strategies ---------------------------------------------------- Proactive strategies are essential to stay ahead of cyber threats. Organizations must prioritize planning and the implementation of cybersecurity frameworks that evolve alongside emerging threats. This includes adopting a mindset of continuous improvement and vigilance. By staying informed about the latest trends in cybersecurity and threat intelligence, organizations can better anticipate and mitigate risks before they become critical issues. Moreover, integrating cybersecurity into the organizational culture can facilitate an environment in which employees understand the significance of their roles in protecting sensitive information. This cultural shift can be supported by leadership commitment and clear communication about the importance of cybersecurity. Encouraging open discussions around security concerns and recognizing employees who demonstrate exemplary security practices can further enhance this culture, leading to a more resilient organization overall. **1) Identification of Key Components of Cybersecurity Strategy** A comprehensive strategy to combat AI-powered cybersecurity threats includes: **Threat Identification and Assessment:** AI has transformed the world of vulnerability assessments by automating the process of identifying security weaknesses within systems, networks, or applications. AI enhances the assessments by quickly scanning for vulnerabilities and, most importantly, continuously improving its models based on its historical data. This in turn helps reduce the issues often faced by traditional manual methods, which are time-consuming and quite often miss certain attack vectors, especially in a large-scale organization or environment. As for pen testing, we can take for example tools like Synack, which integrates with AI algorithms to reduce the manual effort involved. Tools like these automatically create test scenarios and assess system vulnerabilities which helps the security team focus on remediation. With these AI-enabled tools, organizations can address security gaps faster and much more effectively. No alt text provided for this image - Analyse specific AI-driven risks, such as: - AI-generated phishing schemes that mimic legitimate communication. - Deepfake creation for impersonation attacks. - Adaptive malware that morphs to evade traditional detection tools. - Conduct infrastructure vulnerability assessments to identify exploitable weak points. - Exploitation of AI-powered systems like chatbots to leak sensitive data.. ### 2. Response and mitigation Threats are not usually identified during work hours, and human-mediated threat responses can be slow, error-prone, and unpredictable. However, threat response mediated by AI can result in quick and efficient action. Automated decision-making technologies can respond immediately to identified threats, dramatically lowering reaction time and assisting teams in scaling and accelerating response operations. Furthermore, AI\'s ability to learn from previous incidents improves the accuracy of its responses over time, allowing it to adapt to evolving the technique. ### 3. Vulnerability management Vulnerability management is critical for proactive security. AI-native technologies can enable continuous monitoring and automatic scanning of your system\'s security vulnerabilities. When vulnerabilities are discovered, these tools can provide remediation advice, such as suggestions for altering configurations or patch management, based on AI-powered severity evaluations. AI enables enterprises to deploy always-on vigilance to safeguard their systems from known threats and anticipated future vulnerabilities. ### 4. AI-assisted threat hunting AI enhances human analysts\' threat detection efforts by combining human intuition with AI\'s data processing capabilities. AI can also help with managed detection and response (MDR) by delivering threat intelligence and analytics that human analysts may act on. MDR enables threat prioritisation, detection, research, response, and remediation, all of which are dependent on AI\'s analytics capabilities. ### 5. Streamlined analyst experience By offering natural language queries and streamlining challenging data processing, GenAI is enhancing the workflow of security analysts. By streamlining the process, analysts are able to concentrate on more strategic duties and reach well-informed conclusions quicker than before. **Robust Validation Mechanisms** - Implement regular validation and redundancy mechanisms using multiple AI models to: - Cross-verify outputs for accuracy. - Reduce false positives and detect sophisticated attacks more effectively. **Education and Awareness** - Train teams to recognize AI hallucinations (e.g., false alarms or non-existent threats) and understand their potential impact on decision-making. - Educate stakeholders on emerging AI-driven threats and defensive practices. Invest in Continuous Human Training: Regular training programs that focus on understanding AI-driven threats can help bridge the gap between human capabilities and emerging technologies. Organizations must also prioritize building a culture of cybersecurity awareness. s **Integration with Existing Tools** - Enhance existing SIEM platforms by integrating generative AI to: - Automatically correlate events. - Reduce false positives and identify patterns of sophisticated attacks. - Adopt AI-Enhanced Security Tools: Leveraging AI to combat AI-driven threats is a logical step. AI-powered tools can analyze patterns, detect anomalies, and respond to threats in real-time, offering a dynamic defense against evolving attacks. **Data Governance** - Use diverse, high-quality datasets for AI training to minimize inaccuracies and biases. - Enforce stringent data protection practices to safeguard sensitive information. **Automation and AI in Défense** - Employ generative AI for: - Automated threat detection to identify anomalies in real-time. - Streamlining incident response through automated triage and remediation. - Enhancing vulnerability assessments by generating detailed reports and recommendations. - Implement advanced behavioral detection systems to spot unusual activities.\ Regularly update your security systems to stay ahead of evolving threats.\ Educate your team about identifying and mitigating AI-powered cyberattacks.\ The rise of AI-driven cybercrime demands a robust and proactive approach to safeguarding digital environments. Let's work together to outsmart the threats of tomorrow! **Regulatory Compliance** - Align strategies with global frameworks such as GDPR revisions and the AI Risk Management Framework. - Focus on transparency, accountability, and fairness in AI implementation. **2) Prioritization of Efforts** **Risk-Based Prioritization** - Conduct thorough risk assessments focusing on: - High-value targets like financial systems, customer data, and intellectual property. - Key industry-specific vulnerabilities, such as phishing risks in sectors reliant on email communications. - Example: - For healthcare organizations, prioritize defending against deepfakes used for accessing patient records. **Protection of High-Value Targets** - Allocate resources to safeguard assets most critical to business operations and reputation. - Deploy multi-layered defenses around critical systems, including biometric access controls. **Real-World Testing** - Use simulated AI-driven attacks to: - Test and evaluate system defenses. - Identify gaps in incident response processes. - Example: - Design a phishing attack using generative AI to assess the readiness of a financial institution's cybersecurity defenses. **Continuous Monitoring and Feedback** - Implement a feedback loop for: - Regularly updating threat intelligence. - Adapting defenses to the evolving AI-powered threat landscape. - Monitor emerging AI tools that cybercriminals may exploit. - The cybersecurity challenges facing ORGANISATIONSs are becoming more complex. As attackers leverage new technologies like AI and quantum computing to refine their tactics, ORGANISATIONSs must remain agile and forward-thinking in their defense strategies. The increasing sophistication of DDoS attacks, coupled with the rise of state-sponsored threats and unintended data leaks, underscores the need for continuous innovation in cybersecurity practices. - By adopting AI, automation and observability, ORGANISATIONSs can respond to current threats and anticipate and prepare for the evolving risks of the future. As the digital landscape continues to shift, ORGANISATIONSs that prioritize proactive, adaptive security strategies will be best positioned to protect their networks and maintain customer trust. **How AI is Transforming Cybersecurity** 1. For example, AI-powered tools can identify malware signatures and phishing attempts before they penetrate networks. These tools continuously adapt and evolve as they gather new information, enabling organizations to stay resilient against emerging threats. 2. AI-driven systems can detect even subtle deviations from normal activity and flag suspicious behavior. For instance, if an employee suddenly accesses large amounts of sensitive data at an unusual time, AI can trigger alerts and initiate an automated response to mitigate the threat before it escalates. 3. Furthermore, AI enhances fraud detection systems by recognizing suspicious transactions and activities that would typically go unnoticed by traditional security solutions. 4. AI-powered endpoint protection platforms (EPPs) continuously monitor device activity, ensuring that malicious software and unauthorized access are immediately neutralized. 𝗔𝗜 𝗘𝗻𝗵𝗮𝗻𝗰𝗲𝘀 𝗖𝘆𝗯𝗲𝗿𝘀𝗲𝗰𝘂𝗿𝗶𝘁𝘆\ 𝗧𝗵𝗿𝗲𝗮𝘁 𝗗𝗲𝘁𝗲𝗰𝘁𝗶𝗼𝗻: AI analyzes massive datasets to identify unusual behavior patterns, signaling potential breaches or anomalies.\ 𝗥𝗲𝗮𝗹-𝗧𝗶𝗺𝗲 𝗠𝗼𝗻𝗶𝘁𝗼𝗿𝗶𝗻𝗴: AI continuously monitors systems, identifying and neutralizing threats instantly.\ 𝗣𝗵𝗶𝘀𝗵𝗶𝗻𝗴 𝗗𝗲𝘁𝗲𝗰𝘁𝗶𝗼𝗻: AI recognizes fraudulent emails and websites to prevent phishing attacks.\ 𝗠𝗮𝗹𝘄𝗮𝗿𝗲 𝗔𝗻𝗮𝗹𝘆𝘀𝗶𝘀: Machine learning identifies new malware by recognizing behavioral similarities with known threats.\ 𝗜𝗱𝗲𝗻𝘁𝗶𝘁𝘆 𝗣𝗿𝗼𝘁𝗲𝗰𝘁𝗶𝗼𝗻: Biometric AI ensures secure user authentication (e.g., facial recognition).\ 𝗣𝗿𝗲𝗱𝗶𝗰𝘁𝗶𝘃𝗲 𝗔𝗻𝗮𝗹𝘆𝘁𝗶𝗰𝘀: AI forecasts potential attacks and mitigates vulnerabilities in advance.\ 𝗜𝗻𝗰𝗶𝗱𝗲𝗻𝘁 𝗥𝗲𝘀𝗽𝗼𝗻𝘀𝗲: Automates threat response to minimize downtime and damage. Benefits Protecting data across hybrid cloud environments AI tools can identify shadow data, monitor for abnormalities in data access and alert cybersecurity professionals about potential threats by malicious actors accessing the data or sensitive information---saving valuable time in detecting and remediating issues in real time. Generating more accurate and prioritized threats AI-powered risk analysis can produce incident summaries for high-fidelity alerts and automate incident responses, accelerating alert investigations and triage by an average of 55%. The AI technology also helps identify vulnerabilities across threat landscapes and defend against cybercriminals and cyber crime. Balancing user access needs and security AI models can help balance security with user experience by analyzing the risk of each login attempt and verifying users through behavioral data, simplifying access for verified users and reducing the cost of fraud by up to 90%. Also, AI systems help prevent phishing, malware and other malicious activities, ensuring a high security posture within security systems. This post will cover AI threat detection, how it works, and its benefits and challenges. It also includes some real-world use cases of AI threat detection. AI Threat Detection - Featured Image \| SentinelOne**Introduction to AI Threat Detection** ------------------------------------------------------------------------------------------ Artificial intelligence threat detection is the use of machine learning and deep learning (DL) algorithms to help identify cybersecurity threats. In this approach, AI algorithms are trained on a colossal amount of data about common security threats. This makes them capable of recognizing the threats in real-time that may go unnoticed by the manual or conventional approach. Ideally, AI cybersecurity threat detection is used to identify the known types of threats that organizations are identifying with traditional methods. However, with the advancement of AI algorithms, organizations can now continuously track network data, user behavior, and system activity. And if any deviation is found from the regular, these algorithms classify that event as an unknown threat. In contrast to the traditional threat detection approach, the AI-based approach can detect threats earlier in the attack cycle. This helps in minimizing the damage and preventing breaches. One of the most interesting features of AI threat detection is that it can automate the entire process of detecting threats, alerting security teams, and preventing additional threats. **Types of Threats Targeted by AI** ----------------------------------- AI in threat detection has transformed the entire cybersecurity space by providing a robust and wide range of solutions. With the help of various machine learning and deep learning algorithms, AI can detect multiple kinds of threats to enhance surveillance and improve access control. Let's take a look at some of the key threats that AI systems can detect and help mitigate. ### 1. Cyber Threats As organizations are transitioning to the cloud and the amount of data is increasing each day, threats like unauthorized access, data breaches, and network intrusions are becoming common. Traditional security tools usually fail to detect these sophisticated issues, but AI systems excel in identifying and mitigating these cyber threats. AI-driven systems analyze the network traffic in real-time to spot any unusual patterns or potential issues that can harm the network. ### 2. Malware Detection AI-based [malware](https://www.sentinelone.com/cybersecurity-101/cybersecurity/what-is-malware/) detection uses machine learning algorithms to identify malicious and corrupted software by analyzing the file behavior and system changes. While traditional approaches use a database of known malware signatures, AI-based algorithms can spot new and emerging threats by analyzing the way files interact with the system. This approach helps prevent the malware that frequently changes its code to bypass the traditional threat detection methods. ### 3. Phishing and Social engineering [Phishing](https://www.sentinelone.com/cybersecurity-101/threat-intelligence/phishing-scams/) is one of the most common security threats, where the attacker tricks people into stealing their sensitive information. Among all types of threats, AI easily identifies this type of threat. AI algorithms analyze the email metadata, content, and sender patterns to detect and block phishing attempts. Moreover, these AI algorithms are well-versed in detecting [social engineering attacks](https://www.sentinelone.com/cybersecurity-101/threat-intelligence/what-is-social-engineering/) by monitoring communications and interactions. This way, AI helps in safeguarding the information that can otherwise be gathered by manipulating employees or users. ### 4. Physical Security Threats AI systems are now being deployed to monitor the premises and identify potential threats. These AI systems can analyze footage and images in real-time to detect issues like unauthorized access or suspicious behavior. Some deep learning use cases like facial recognition, object detection, etc., also help in preventing unauthorized entry to secure physical environments. ### 5. Access Control Systems AI helps organizations to implement more dynamic security protocols for modern access control. AI algorithms can continuously learn from users' access patterns and can identify any anomalies in behavior. For example, a user or an employee attempting to access restricted areas or logging in from unusual locations can be easily detected and stopped by AI systems. The integration of AI into the [access control](https://www.sentinelone.com/cybersecurity-101/cybersecurity/what-is-access-control/) system can ensure that only authorized people gain access and that any suspicious attempts can be flagged in real-time. ### 6. Behavior Analysis Behavior-based analysis is one of the strengths of AI-based threat detection. While traditional threat detection methods rely on known signatures or patterns, AI systems can learn the usual behavior of an organization's network, applications, and users. And when they observe a deviation from the baseline, they raise alerts in real-time to enable early threat detection. This way, it helps in identifying and preventing both known and unknown threats (zero-day attacks). **How AI Enhances Threat Detection** ------------------------------------ Due to its effectiveness and accuracy, AI-based threat detection systems are used across digital, physical, and behavioral domains. Let's discuss some of the key ways AI enhances the threat detection process. ### Machine Learning and Pattern Recognition By analyzing the vast amounts of network traffic, user behavior, and system logs, machine learning algorithms can recognize patterns to classify normal and abnormal activities. The more data the model is trained on, the better it becomes in classifying between legitimate activities and possible threats. This results in a faster and more accurate detection of cyberattacks, malware, or insider threats. ### Natural Language Processing Natural language processing (NLP) is gaining a lot of popularity due to the release of various large language models (LLMs). It is the field of ML that enables AI systems to understand and interpret human language. By interpreting human language, these systems can detect threats related to phishing, social engineering, and malicious communications. NLP models are trained on a huge amount of language data such as emails, chats, and documents to identify potentially harmful language, phishing attempts, or insider threats. ### Image and Video Analysis Image and video analysis is the cornerstone of physical security and surveillance. Deep learning algorithms like CNNs (convolutional neural networks) and RNNs (recurrent neural networks) can be trained on images and videos to detect unauthorized access, suspicious behavior, or security breaches in real-time. For example, face recognition models trained on CNNs can help in identifying individuals who are not authorized to access certain areas. Also, object detection models can be trained on images and videos to detect weapons or unrecognized packages for security purposes. ### Anomaly Detection Algorithms Anomaly detection, being one of the core applications of AI threat detection, uses sophisticated algorithms like time-series analysis. These algorithms analyze the system networks and user behaviors over time to establish a baseline. At any point, if a deviation is observed in the system, it indicates a security breach or attack. Some examples of anomaly detection are abnormal login attempts, unusual file access patterns, etc. **How AI Threat Detection Works** --------------------------------- AI-driven threat detection employs machine learning and deep learning algorithms to find suspicious activity or potential security threats. At its core, AI systems gather vast amounts of data from various sources---for example, network traffic, user interactions, system logs, and external threat databases. Then, AI systems analyze this data to identify patterns and establish a baseline for normal activity. Next, AI systems use this baseline and apply anomaly detection techniques to spot deviations that may indicate potential threats and attacks. To further refine this process, organizations can train ML models on historical data to detect both known threats and previously unseen threats. Once the threat is detected, AI systems can alert the security teams for further investigation. Some AI systems are also capable of automatically initiating mitigation actions. This way, AI systems stay one step ahead of attackers and protect the organization's data and information. **Key Technologies in AI Threat Detection** ------------------------------------------- While machine learning plays a key role in AI threat detection, there are some other technologies as well that drive AI-based threat detection: ### \#1. Artificial Neural networks (ANNs) Inspired by the human brain, ANNs are the foundation of many AI systems. These networks can be trained on both labeled (supervised learning) and unlabeled (unsupervised learning) data to spot anomalies that signal potential threats. They are ideal for identifying complex patterns in large datasets, such as user behavior or network activity. ### \#2. Deep Learning Deep learning is a subset of machine learning that can analyze vast amounts of data at multiple levels. Neural networks are the heart of deep learning that can extract higher-level features from raw data. In the cybersecurity space, deep learning models excel in fields like malware detection, phishing prevention, and image/video analysis to detect and prevent threats. ### \#3. Reinforcement Learning Reinforcement learning (RL) is another AI approach where a system learns to make important decisions based on rewards and penalties. For threat detection, RL can optimize response strategies to automatically choose the best course of action when a threat is detected. ### \#4. Big Data Analytics With the help of big data analytics, systems can process and analyze huge amounts of data from different sources, such as network logs, user activity, and threat intelligence feeds. Leveraging this big data, AI threat detection systems can train models that can make the detection process faster and more accurate. **Implementing AI in Threat Detection Systems** ----------------------------------------------- Implementing AI in threat detection requires a thoughtful approach for seamless integration with your organization's existing security infrastructure. Let's check out some of the key aspects that you should consider while implementing AI threat detection. ### Integration with Existing Security Infrastructure You can't simply just go ahead and implement AI in your threat detection system. You should understand that AI systems must integrate smoothly with an organization's existing security tools, such as firewalls, intrusion detection/prevention systems (IDS/IPS), and [security information and event management (SIEM)](https://www.sentinelone.com/cybersecurity-101/data-and-ai/what-is-security-information-and-event-management-siem/) systems. AI systems do not replace these existing systems; rather, they complement these existing systems by enhancing their capabilities with advanced threat detection and predictive analytics. Most of the AI platforms have APIs or connectors for easy integration with the existing infrastructure. ### Real-Time Monitoring and Alerts Real-time monitoring of networks, systems, and user behaviors is one of the key capabilities of AI in threat detection. AI algorithms are capable of continuously analyzing the data for anomalies. This enables early detection of potential threats before they cause significant damage. Moreover, AI-powered threat detection systems can generate real-time alerts. This helps ensure that security teams are notified immediately of any security issue and can respond swiftly to mitigate risks. ### Automation of Responses AI can enhance threat detection systems by automating response actions. For example, once a threat is detected, AI can automatically trigger some predefined security protocols. Moreover, it can block suspicious IP addresses or reset compromised user credentials. This automation significantly reduces the time between detection and response and minimizes any potential damage from cyberattacks. ### Scalability and Flexibility AI-based threat detection systems are highly scalable, which makes them suitable for organizations of all kinds. As cyber threats are evolving and growing in volume, AI-based threat detection systems are becoming essential. These systems can process large amounts of information without sacrificing performance. Moreover, AI systems also provide flexibility so that organizations can customize detection parameters and responses based on their specific needs. ai threat detection - AI-based threat detection \| SentinelOne**AI Threat Detection Benefits** ---------------------------------------------------------------------------------------------- AI threat detection offers a range of benefits to enhance the entire threat detection and defense procedure. Here are some of the benefits of AI threat detection: - **Faster detection**---Due to their ability to correlate and analyze data much faster than humans, AI systems can detect threats more easily and quickly. Moreover, these systems can work in real-time and detect anomalies and suspicious behavior as they occur. This faster approach results in reducing the time difference between threat detection and mitigation. - **Proactive defense against emerging and higher threat volume**---One of the key capabilities of AI-based systems is that they can detect previously unknown or emerging threats such as zero-day vulnerabilities. While traditional threat detection approaches rely on some known signatures, AI systems can detect patterns and signals of new attacks in large volumes. - **Reduced false positives**---Incorrectly identifying normal activities as threats is a major problem in traditional threat detection systems. AI-enabled systems can reduce false positives by learning from patterns of normal behavior and refining their algorithms over time. This results in detecting genuine threats and reducing the time wasted on investigating false cases. - **Improved threat intelligence**---AI systems improve themselves by continuously learning from new data, attacks, and responses. With an integration to both external and internal data feeds, AI systems offer insights into both current and future security risks. **Challenges and Limitations** ------------------------------ While having a lot of advantages, AI systems also come with several challenges and limitations. - **Data privacy and security concerns**---AI systems work by analyzing vast amounts of information, including sensitive information such as logs, personal details, etc. This can result in misuse or unauthorized access to sensitive information. To make sure sensitive data is handled securely, organizations must adhere to security regulations, such as GDPR or [CCPA](https://oag.ca.gov/privacy/ccpa). - **False positives and negatives**---While AI systems can significantly reduce false positives, they cannot completely get rid of them. Also, using AI systems does not guarantee that they will 100% detect all the genuine threats, which leads to some false negative cases. To make sure false positives and false negatives are reduced, AI systems must be continuously fine-tuned. - **Ethical implications**---When it comes to monitoring user behavior, AI threat detection can result in some ethical concerns. For example, employee surveillance and facial recognition can hurdle individuals' privacy rights, leading to potential misuse or abuse. To make sure things remain ethical, organizations should establish transparent policies on using AI systems. - **Technical limitations**---While AI systems work efficiently, they are a kind of black box. One cannot get a complete understanding of how they are working to draw a conclusion. Also, these AI systems require high-quality data to function effectively. Incomplete or inaccurate threats-related data can lead to problems such as false positive and false negative alerts. Moreover, AI systems can be complex, and they often require significant computational resources and ongoing maintenance to remain effective. **Case Studies and Real-world Applications** -------------------------------------------- Now, let's look at some real-world use cases of AI-based threat detection. ### \#1. AI in Government and Military Governments and military organizations are using AI threat detection systems for national security purposes. This includes detecting cyber intrusions, securing communications, and analyzing massive amounts of intelligence data. For example, the Cybersecurity and Infrastructure Security Agency (CISA) uses SentinelOne, an advanced AI-based cyber threat detection and prevention platform, to enable government-wide cyber defense. ### \#2. AI in Corporate Security Corporations and organizations are adopting AI-based threat detection to protect their sensitive data and critical infrastructure. These enterprises use AI to monitor employee behavior and network traffic for signs of insider threats. For example, [Aston Martin, one of the biggest manufacturers](https://www.sentinelone.com/resources/aston-martin-sentinelone-cybersecurity-partner-vigilance-mdr/) of luxury sports cars, has replaced its legacy security system with SentinelOne to protect a century of motoring heritage. ### \#3. AI in Public Safety Public safety initiatives such as surveillance and anomaly detection increasingly use AI. Public safety agencies or public organizations deploy AI to analyze video feeds from security cameras to identify suspicious activities or unauthorized individuals in real-time. One example of this is one of the [largest K-12 school systems in the U.S.](https://www.sentinelone.com/resources/oneill-secures-their-entire-it-environment-with-sentinelone/), based in Nebraska, using solutions like [SentinelOne](https://www.sentinelone.com/) to prevent its diverse connected devices across MacOS, Windows, Chromebooks, and mobile devices from modern-day threats. ### \#4. Tap Into the Power of AI for Threat Detection After reading this post, you now know about AI-based threat detection. We've covered how AI-based threat detection works, the key technologies involved, and how you can implement AI in your existing threat detection system. Finally, you have seen the benefits, challenges, and some real-world use cases of AI-based threat detection. Since cybercriminals constantly evolve their attacking strategies, you need a solution that can rely on more than just a set of predefined rules and patterns. Using machine learning and deep learning algorithms can help you tackle this issue while providing more accuracy, scalability, and flexibility. [SentinelOne](https://www.sentinelone.com/) is one of the most famous security platforms that can fulfill all your AI-based threat detection needs. AI-driven cybersecurity leverages machine learning algorithms, deep learning techniques, and data analysis to detect, predict, and prevent cyber attacks. From automating threat detection to combating advanced persistent threats, AI is revolutionizing the way security teams safeguard sensitive data and infrastructure. However, the rise of AI has also opened the door for cybercriminals to exploit this technology, creating an arms race between attackers and defenders. **The Future of AI-Driven Cybersecurity** The integration of AI into cybersecurity is still in its early stages, but its potential is vast. In the future, we can expect AI systems to play a more proactive role in threat mitigation, offering autonomous security solutions that can respond to attacks in real time without human intervention. AI will also enable better collaboration between security systems, allowing for seamless sharing of threat intelligence across organizations and industries. As AI algorithms continue to evolve, they will become even more effective at identifying zero-day vulnerabilities and preventing large-scale attacks. However, organizations must also be mindful of ethical considerations, such as data privacy, transparency, and bias in AI systems. Building trustworthy AI solutions will be essential to ensure their effectiveness and adoption on a global scale. The rise of AI-driven cybersecurity marks a significant milestone in the ongoing battle against cyber threats. By automating threat detection, predicting risks, and enhancing response times, AI is helping organizations fortify their defenses in an increasingly hostile digital environment. However, as cybercriminals begin to exploit AI for malicious purposes, the need for constant innovation and vigilance becomes paramount. In this dynamic landscape, AI is not just an option but a necessity for organizations seeking to stay ahead of cyber adversaries. By embracing AI-driven cybersecurity, businesses can safeguard their future and ensure resilience in an ever-evolving threat landscape. **Collaboration and Information Sharing** Essential Cybersecurity Measures for 2025 ----------------------------------------- Preparation is pivotal in the ever-evolving world of cybersecurity. Organizations must take proactive measures to safeguard themselves against future threats. This involves investing in cyber defenses and fostering a culture of security within the organization. As cyber threats become increasingly sophisticated, the need for a multi-layered approach to security has never been more critical. This means not only relying on technology but also emphasizing human factors and organizational processes that contribute to a robust security posture. - - - **Industry Forums and Partnerships** - Join platforms such as the Information Sharing and Analysis Centers (ISACs) to exchange threat intelligence and develop industry-specific best practices. - Example: - Banks collaborating through Financial Services ISAC (FS-ISAC) to mitigate AI-enabled fraud. **Vendor and Technology Partnerships** - Partner with technology vendors to: - Stay updated on AI advancements. - Integrate cutting-edge tools for real-time threat detection and response. **Government and Regulatory Agencies** -------------------------------------- **G**overnment Policies and Regulations for Cybersecurity --------------------------------------------------------- Governments around the world are putting policies in place to address cybersecurity concerns proactively. Regulations such as the General Data Protection Regulation (GDPR) and various national cybersecurity strategies highlight the importance of establishing a regulatory framework that promotes robust cybersecurity practices. By creating guidelines that promote best practices, governments not only protect citizens but also bolster the overall security of the digital ecosystem. Furthermore, these regulations often mandate regular audits and compliance checks, ensuring that organizations remain vigilant and accountable in their cybersecurity efforts. This not only helps in identifying vulnerabilities but also in fostering a culture of continuous improvement within organizations, encouraging them to adopt the latest technologies and methodologies to safeguard sensitive information. - Engage with national and international bodies to: - Align cybersecurity strategies with evolving regulatory standards. - Advocate for ethical AI practices and collaborative incident response mechanisms. - Example: - US-EU collaboration on updating GDPR to address generative AI challenges. Industry's Response to Evolving Cybersecurity Threats ----------------------------------------------------- Industries must take a proactive stance toward evolving threats as well. Collaboration among sectors can lead to sharing resources, threat intelligence, and best practices. By engaging in partnerships with governmental bodies, businesses can contribute to more comprehensive and effective cybersecurity strategies. Additionally, organizations should engage in forums and consortia focused on cybersecurity to foster innovation and resilience against cyber threats. These collaborative platforms allow for the exchange of insights and experiences, enabling companies to learn from one another's successes and failures. Moreover, industry leaders are increasingly investing in research and development to create advanced security solutions, such as artificial intelligence-driven threat detection systems, which can significantly enhance their ability to respond to potential breaches. As cyber threats become more sophisticated, the importance of such innovations cannot be overstated, as they play a crucial role in staying one step ahead of malicious actors. **Specific Collaboration Initiatives** - Financial institutions can develop joint training programs on detecting generative phishing schemes. - Governments can simulate AI-driven cyberattacks on critical infrastructure to improve resilience. - Industry and academia partnerships for research into advanced AI defense mechanisms. This comprehensive strategy ensures a proactive, multi-faceted approach to mitigating the growing threats posed by AI-driven cyberattacks while fostering collaboration across sectors.