Lesson 4 Part 1.pdf
Document Details
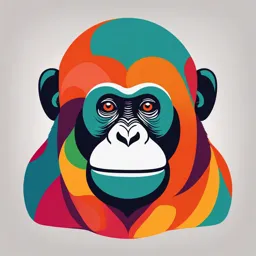
Uploaded by CooperativeJacksonville
Nanyang Technological University
Tags
Full Transcript
Lesson 4 Part 1 Welcome to lesson four. This lesson will be on anomaly detection. By the end of this lesson, you'll be able to understand behaviour drivers and develop threat profiles, develop models for directing abnormal sequences of activities and evaluate the effectiveness of different sequence...
Lesson 4 Part 1 Welcome to lesson four. This lesson will be on anomaly detection. By the end of this lesson, you'll be able to understand behaviour drivers and develop threat profiles, develop models for directing abnormal sequences of activities and evaluate the effectiveness of different sequence and model development approaches. And finally, you will be able to understand anomaly detection and use tools and techniques for identifying anomalies in user behaviour to develop threat profiles. So let's start by talking about what is anomaly detection. Well, it's a great way to detect unusual activities by users outside what their normal activity is. So every user within an organisation will have variations in their when they conduct their activities on organisational networks or systems. No single user's activities will be identical to each other. So for example, Bob, who works in the marketing department, sends many emails to external vendors every day. But Anna, who works in product development, does not send any emails to external vendors. So to find anomalies in activities conducted by users, we need to look at the activity and insider threat indicators of each individual using standard deviation to detect anomalies. And anomaly detection uses things like number one user activity. We can use user activity to detect these anomalies for certain virtual insider threat indicators, such as unusual logins or excessive downloads. And then we can use contextual and non-virtual indicators to apply risk score modifiers to the anomaly detection to help prioritise alerts. Okay, so here you're going to learn about how to use standard deviation to detect an anomaly from this example. So for the purposes of this example, we'll look at the user Bob and his job searching activity he conducted on indeed.com over the last seven months. Job searches conducted by users can give us insight into whether a user may intend to leave the organisation and therefore pose a greater risk to potentially taking data with them. Job searches using company internet can also be tied back to non-virtual indicators, such as performance review periods and other factors that could increase the likelihood of a user wanting to leave the organisation. So for this example, we're using a small data set. So however, the larger data set, the better the accuracy for detecting anomalies. And this can also be applied to other activities such as downloading sensitive files, accessing systems, or login times as well. Firstly, we need to calculate the average job searches that Bob has carried out for the last seven months, or the mean, in order to work out the standard deviation.