Lesson 14: Regression Discontinuity (PDF)
Document Details
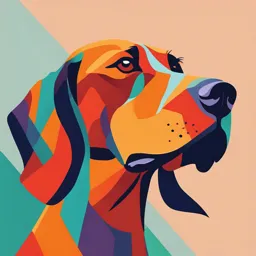
Uploaded by VersatileBoltzmann7628
University of California, Santa Cruz
Tags
Related
- Fuzzy Regression Discontinuity Design PDF
- Research Methods: Applied Empirical Economics Lecture 4 PDF
- EC338 Microeconometrics Revision Weeks 6-10 PDF
- Regression Discontinuity - Lecture Notes PDF
- Regression Discontinuity - Fuzzy RD, Examples | PDF
- Principles of Empirical Analysis: Quasi-Experiments and Instrumental Variables
Summary
This document presents a lesson on regression discontinuity, specifically focusing on balance and instrumental variables. The content covers different aspects of the methodology, suitable for an econometrics course, potentially for undergraduate-level students.
Full Transcript
Lesson 14 Regression Discontinuity: Balance and IV Econ 104 Regression Discontinuity 1 / 33 Today’s Lesson 1 Upcoming assignments: Assignment 3 2 Regression Discontinuity validity: Balance graphs Balance...
Lesson 14 Regression Discontinuity: Balance and IV Econ 104 Regression Discontinuity 1 / 33 Today’s Lesson 1 Upcoming assignments: Assignment 3 2 Regression Discontinuity validity: Balance graphs Balance tables Adding covariates Density test 3 Regression Discontinuity: IV 4 Regression Discontinuity: Overview Econ 104 Regression Discontinuity 2 / 33 Today’s Lesson We have been discussing regression discontinuity (RD) designs. Setup and intuition Making figures (bin width, bandwidth, y-axis) Regression specifications (parametric, non-parametric) A key assumption for the regression discontinuity design to be valid is that the potential outcomes E[Y0i |Xi = x] and E[Y1i |Xi = x] are continuous in x at the threshold. We are going to examine ways to assess the validity of this assumption. Econ 104 Regression Discontinuity 3 / 33 RD: Balance Graphs Econ 104 Regression Discontinuity 4 / 33 RD: Balance Graphs The key to a valid comparison is having groups that are similar on all dimensions other than the treatment of interest. We want the groups to be similar in both their observable and unobservable characteristics (particularly potential outcomes). The groups on either side of the threshold could be different from each other: If the program is something desirable, people might deliberately manipulate the running variable X to get into the program. You could lose some people on one side of the cutoff. For example, with survey data, not everyone responds and you could have differential non response rates at the cutoff. Why might the response rate to a survey about drinking change at 21? Alternatively, groups may (falsely) appear to be different on each side of the cutoff if we do not use an appropriate regression specification (e.g, the polynomial order is too low or high). Econ 104 Regression Discontinuity 5 / 33 RD: Balance Graphs We would like to test if there are sharp changes in the potential outcomes at the threshold. If there are sharp changes in the potential outcome this would violate our continuity assumption. Can we check the that the untreated and treated potential outcomes are the same on both sides of the threshold? What can we check at the cutoff? Econ 104 Regression Discontinuity 6 / 33 RD: Balance Graphs Which covariates should we check to test for evidence of a valid comparison on each side of the cutoff? Check covariates that are determined before the treatment (e.g., fixed characteristics). Do not check variables that are affected by the treatment. For example, in testing the validity of the RD for the case of the minimum legal drinking age: Would we want to check for a discontinuity in how often people go out to clubs at night? Would we want to check for a discontinuity in gender? How should you examine if there is continuity at the cutoff? Make figures with the predetermined variable on the vertical axis. Estimate regressions and check for evidence of statistically significant changes at the cutoff. Econ 104 Regression Discontinuity 7 / 33 RD: Balance Graphs Gender Composition 1.8 Fraction Male.6.4.2 0 18 19 20 21 22 23 24 Age at Time of Survey Do you see evidence of a change? Econ 104 Regression Discontinuity 8 / 33 RD: Balance Graphs Racial Composition 1.8 Fraction White.6.4.2 0 18 19 20 21 22 23 24 Age at Time of Survey Do you see evidence of a change? Econ 104 Regression Discontinuity 9 / 33 RD: Balance Graphs Racial Composition 1.8 Fraction Hispanic.6.4.2 0 18 19 20 21 22 23 24 Age at Time of Survey Do you see evidence of a change? Econ 104 Regression Discontinuity 10 / 33 RD: Balance Graphs Educational Attainment 1 Fraction With HS Diploma.8.6.4.2 0 18 19 20 21 22 23 24 Age at Time of Survey Do you see evidence of a change? What do we learn? Econ 104 Regression Discontinuity 11 / 33 RD: Balance Graphs Employment Rates 1.8 Fraction Employed.6.4.2 0 18 19 20 21 22 23 24 Age at Time of Survey Is this a variable you should include in your balance table? Econ 104 Regression Discontinuity 12 / 33 RD: Balance Graphs Marital Status 1.8 Fraction Married.6.4.2 0 18 19 20 21 22 23 24 Age at Time of Survey Is this a variable you should include in your balance table? Econ 104 Regression Discontinuity 13 / 33 RD: Balance Tables Econ 104 Regression Discontinuity 14 / 33 RD: Balance Tables We now want to formally check whether the changes at the threshold are not sta- tistically significant. This is analogous to the balance table you made for the RCT. Checking to see if people just above and below the cutoff are similar in their observable characteristics. The assumption is that if they are similar in their observable characteristics then they are similar in their potential outcomes. Estimate regression with the same bandwidth and polynomial order of X used for the first stage and reduced form. For example: malei = φ0 + φ1 Zi + φ2 Agei + φ3 Agei2 + φ4 Agei ∗ Zi + φ5 Agei2 ∗ Zi + ui Make a table of these results with a different characteristic as the outcome in each column. Econ 104 Regression Discontinuity 15 / 33 RD: Balance Tables HS Diploma Married Working LW In School Male Z 7.64 -2.85 1.94 -0.33 1.88 (1.40) (1.24) (1.72) (1.35) (1.78) Age -20.39 6.70 1.07 -2.60 -4.04 (1.71) (1.23) (2.01) (1.72) (2.04) Age*Z 22.83 -2.97 4.30 -1.42 5.40 (2.23) (1.91) (2.68) (2.13) (2.78) Age2 -11.34 0.86 -2.10 1.67 -0.76 (0.58) (0.37) (0.66) (0.59) (0.67) Age2 ∗ Z 10.31 -0.28 1.34 -1.58 0.30 (0.74) (0.62) (0.87) (0.70) (0.91) Constant 74.80 15.73 62.45 17.59 42.16 (1.04) (0.87) (1.26) (1.02) (1.28) Observations 27,756 27,756 27,756 27,756 27,756 R-squared 0.06 0.04 0.03 0.05 0.00 Note: Age is centered at 21 and Z = 1 if age is over 21 and 0 otherwise. Econ 104 Regression Discontinuity 16 / 33 RD: Density Test Econ 104 Regression Discontinuity 17 / 33 RD: Density Test Checking density at the cutoff: 600 500 Count of People Surveyed 400 300 200 100 0 18 19 20 21 22 23 24 Age at Time of Survey Why is it interesting to look for a change in the number of people at the cutoff? Econ 104 Regression Discontinuity 18 / 33 RD: Density Test Another Example: Does a tax deduction for tuition and fees increase college attendance? Study examines a rule under which households with income under 130,000 dollars are eligible for a college tax deduction. Compare households with income just above (untreated) and below (treated) this cutoff. Adjusted gross income is the sum of annual salaries, wages, investment income, etc. Idea is that households earning 129,999 and 130,000 are essentially identical except for eligibility for the tax credit. The hope is that it is essentially random whether a household is just above or below the cutoff. Can you think of anything that might go wrong with this RD design? Econ 104 Regression Discontinuity 19 / 33 RD: Density Test What happened? Are households with incomes just above and below the 130,000 cutoff comparable in terms of the likelihood that their children attend college? Econ 104 Regression Discontinuity 20 / 33 RD: Adding Covariates Econ 104 Regression Discontinuity 21 / 33 RD: Adding Covariates First, examine the first stage and reduced form results with just a polynomial in the running variable: Di = φ0 + φ1 Zi + φ2 Xi + φ3 Xi2 + φ4 Xi ∗ Zi + φ5 Xi2 ∗ Zi + ui and Yi = π0 + π1 Zi + π2 Xi + π3 Xi2 + π4 Xi ∗ Zi + π5 Xi2 ∗ Zi + εi Next, add predetermined characteristics Wi to the regressions: Di = φ0 + φ1 Zi + φ2 Xi + φ3 Xi2 + φ4 Xi ∗ Zi + φ5 Xi2 ∗ Zi + Wi φ6 + ui and Yi = π0 + π1 Zi + π2 Xi + π3 Xi2 + π4 Xi ∗ Zi + π5 Xi2 ∗ Zi + Wi π6 + εi In the MLDA analysis, you can include variables such as age, race, educational attainment in Wi. You should not include drinking or measures of going out at night. For variables such as marital status it is a bit less clear if you should include them. Econ 104 Regression Discontinuity 22 / 33 RD: Adding Covariates What is the value of adding covariates to the regression? It is a way of testing for balance. The coefficients φ1 and π1 should not change much. Often adding covariates will increase the precision of your estimates (reduce the standard errors). You should only add predetermined characteristics. You definitely should not include anything that can be affected by the treatment. These are outcomes! You should include the same variables in the first stage and reduced form. Econ 104 Regression Discontinuity 23 / 33 RD: Adding Covariates The estimates of the change at the threshold φ1 and π1 should not change much as you add covariates to the regression. Why should adding the covariates not affect the estimate of the change at the threshold? Recall omitted variables bias. It depends on whether some third variable (or characteristic) is correlated with treatment and the outcome. In this case the variables we are adding should be very similar across the two groups and thus are uncorrelated with treatment. Econ 104 Regression Discontinuity 24 / 33 RD: Adding Covariates Drank in the last month. Effect of adding covariates: Z 9.25 8.77 8.53 8.44 8.15 (2.15) (2.13) (2.12) (2.12) (2.09) Age -3.00 -2.14 -2.10 -1.61 -0.73 (3.65) (3.64) (3.63) (3.63) (3.59) Age ∗ Z 10.29 9.19 8.81 8.36 7.12 (4.92) (4.89) (4.87) (4.87) (4.80) Age2 -3.80 -3.21 -2.94 -2.86 -2.56 (1.77) (1.77) (1.76) (1.76) (1.75) Age2 ∗ Z 1.15 0.64 0.39 0.40 0.23 (2.37) (2.35) (2.34) (2.34) (2.31) HS Diploma 0.14 0.13 0.11 0.12 (0.01) (0.01) (0.01) (0.01) Work LW 0.09 0.14 0.11 (0.01) (0.01) (0.01) In School 0.09 0.06 (0.01) (0.01) Male 0.15 (0.01) Constant 53.47 42.14 37.41 34.56 29.92 (1.57) (1.73) (1.76) (1.80) (1.78) Econ 104 Regression Discontinuity 25 / 33 RD: IV Estimate Econ 104 Regression Discontinuity 26 / 33 RD: IV Estimate The Regression discontinuity design can in some cases be viewed as an instru- mental variables approach. Suppose want to know the effect of drinking on arrests. We use the MLDA as a source of variation in drinking that is not due to individual’s choices. First Stage: Effect of being able to drink legally on alcohol consumption Drinkeri = φ0 + φ1 Zi + φ2 Agei + φ3 Agei2 + φ4 Agei ∗ Zi + φ5 Agei2 ∗ Zi + ui Reduced Form: Effect of being able to drink legally on arrest rates Arresta = π0 + π1 Za + π2 Agea + π3 Agea2 + π4 Agea ∗ Zi + π5 Agea2 ∗ Za + ua What does the reduced form tell us? Econ 104 Regression Discontinuity 27 / 33 RD: IV Estimate We can then combine the estimates from the two equations to get the increase in crime rates on a per drinker basis. RF π1 Effect of drinking = FS = φ1 This is an estimate of the increase in the arrest rate if we went from no one drinking to everyone drinking. Remember that for valid IV we need two assumptions: 1 The instrument Z needs to affect the explanatory variable D. 2 The instrument Z needs to affect the outcome Y only through the variable we are interested in (i.e. uncorrelated with omitted variables). Are these two assumptions testable? Econ 104 Regression Discontinuity 28 / 33 RD: IV Estimate Instrumental Variables Assumptions: 1 We need the instrument to actually affect the explanatory variable (drinking). Does this hold? 2 We need the instrument to affect the outcome only through the explanatory variable of interest. That is, the MLDA affects crime only through the 9 percent of the population who start drinking at age 21. Do you think this is true or are there other changes? Econ 104 Regression Discontinuity 29 / 33 Regression Discontinuity: Overview Econ 104 Regression Discontinuity 30 / 33 Regression Discontinuity: Overview Let’s review what we have discussed on the steps to implementing an RD design. 1 Make a good figure for the first stage and each outcome. This involves choosing appropriate ranges for Y and X and the right bin size over which to average the outcome Y. The goal is to make the jump at the threshold (if there is one) apparent in the figures. You do this by visually evaluating different choices of bin width and bandwidth for the histogram. The final figure should have the fitted regression superimposed on it. Econ 104 Regression Discontinuity 31 / 33 Regression Discontinuity: Overview 2 Estimate regressions. Parametric Approach: experiment with polynomial order, superimposing the fitted regression over the figure. Select the lowest order polynomial that fits well and include it superimposed on the figure. Non-parametric Approach: use a linear model and experiment with different bandwidths, superimposing the fitted regression over the figure. 3 Present regression results in a clearly laid out table. 4 Document that the choices you made for the regression are not important. Show results for different polynomial orders and bandwidths. Econ 104 Regression Discontinuity 32 / 33 Regression Discontinuity: Overview Typical figures and tables for an RD paper: 1 Figures showing balance in covariates. 2 Figure for first stage. 3 Figures of reduced form outcomes. 4 Regression estimates showing balance. 5 Regression estimates for first stage. 6 Regression estimates for reduced form outcomes. 7 IV estimates. 8 Often an appendix will include density figures, regression results with alternative polynomials and bandwidths that show that the results are not highly sensitive to these choices. Econ 104 Regression Discontinuity 33 / 33