Regression Discontinuity - Fuzzy RD, Examples | PDF
Document Details
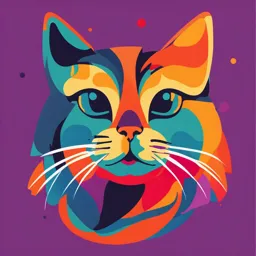
Uploaded by LongLastingGalaxy
Magnus Carlsson
Tags
Related
- Fuzzy Regression Discontinuity Design PDF
- Research Methods: Applied Empirical Economics Lecture 4 PDF
- Lesson 14: Regression Discontinuity (PDF)
- EC338 Microeconometrics Revision Weeks 6-10 PDF
- Regression Discontinuity - Lecture Notes PDF
- Principles of Empirical Analysis: Quasi-Experiments and Instrumental Variables
Summary
This document provides an introduction to regression discontinuity (RD) designs, focusing on fuzzy RD. It covers the key concepts, including the different types of RD, examples in healthcare, financial aid, and class size, and instrumental variables methods. The content is aimed at those with a working knowledge of applied econometrics.
Full Transcript
Regression discontinuity 2 Magnus Carlsson Regression discontinuity (RD) a summary A. Sharp RD (previous lecture) (i) Non-parametric RD (MTE/Difference in means estimator) (ii) Parametric RD B. Fuzzy RD (this lecture) (i) Non-parametric RD (the Wald estimator) (i...
Regression discontinuity 2 Magnus Carlsson Regression discontinuity (RD) a summary A. Sharp RD (previous lecture) (i) Non-parametric RD (MTE/Difference in means estimator) (ii) Parametric RD B. Fuzzy RD (this lecture) (i) Non-parametric RD (the Wald estimator) (ii) Parametric RD Fuzzy Regression discontinuity: intro ln the Fuzzy Regression Discontinuity (FRD) design, the probability of receiving the treatment need not change from zero to one at the threshold. Instead the design allows for a jump in the probability of getting treatment at the threshold Such a situation can arise if incentives to participate in a program change discontinuously at a threshold, without these incentives being powerful enough to move all units from non-treatment to treatment. Fuzzy Regression discontinuity: intro Fuzzy RD comes in two versions: In a “reduced form” version, where actual treatment is unobserved but where we know that the probability of treatment shifts at the threshold ln this version, Fuzzy RD resembles the reduced form equation in the instrumental variables context (remember: where one regressed the outcome, 𝑦, directly on the instrument, 𝑧) ln an instrumental-variables-type version, where (reaching) the discontinuity becomes an instrumental variable for observed treatment status instead of deterministically switching treatment on or off. Example of Fuzzy “reduced form” RD: the marginal efficiency of health care A key policy question is whether the benefits of additional medical expenditures exceed their costs. This is obviously difficult to analyze without randomized trials Almond et al (2010, QJE) propose a research design which, under explicit assumptions, permits direct estimation of the marginal returns to medical care. The design requires an observable, continuous measure of health risk and a diagnostic threshold (based on this risk variable) that generates a discontinuous probability of receiving treatment RD example: the marginal efficiency of health care As an example, they exploit the fact that medical decisions regarding the treatment for “at-risk” newborns is based on a birth weight threshold of 1,500 grams Note, however, that actual treatment is unobserved to the authors; they just know that medical guidelines suggest treatment based on the threshold RD intro: examples As an example, they exploit the fact that medical decisions regarding the treatment for “at-risk” newborns is based on a birth weight threshold of 1,500 grams Note, however, that actual treatment is unobserved to the authors; they just know that medical guidelines suggest treatment based on the threshold A birth weight-based threshold is attractive for a regression discontinuity design, since it is unlikely to represent breaks in underlying health risk. Since the position of a newborn just above 1500 grams relative to just below 1500 grams is “as good as random”, treatment should also be as good as randomized RD example: the marginal efficiency of health care ln the simplest form, the authors could have estimated: 𝑦𝑖 = 𝛼0 + 𝛼1 𝑉𝐿𝐵𝑊𝑖 + 𝛼2 𝐵𝑊 + 𝜀𝑖 , (1) where 𝑦𝑖 is an outcome such as one-year mortality, 𝑉𝐿𝐵𝑊𝑖 is an indicator that the newborn was classified as very low birth weight ( 𝑥0. Instead, we have the probability of treatment as a function of 𝑥𝑖 , such that: 𝑃[𝐷𝑖 = 1|𝑥𝑖 ] = 𝑓(𝑥𝑖 ) (4) where 𝑓(𝑥𝑖 ) is some function of 𝑥𝑖. For instance, the probability of being treated for low birth weight is some function (linear, square, cube, etc) of birth weight Fuzzy Regression discontinuity as IV Now, we have a jump in the probability of treatment at 𝑥0 , such that: 𝑔0 𝑥𝑖 𝑖𝑓 𝑥𝑖 > 𝑥0 𝑃[𝐷𝑖 = 1|𝑥𝑖 ] = , where 𝑔0 𝑥𝑖 ≠ 𝑔1 𝑥𝑖 (5) 𝑔1 𝑥𝑖 𝑖𝑓 𝑥𝑖 < 𝑥0 where the functions 𝑔0 𝑥𝑖 and 𝑔1 𝑥𝑖 can be anything as long as they differ at 𝑥0 We assume 𝑔1 𝑥𝑖 > 𝑔0 𝑥𝑖 so that 𝑥𝑖 > 𝑥0 makes treatment more likely Fuzzy Regression discontinuity as IV In this specification, the relationship between the probability of treatment and 𝑥𝑖 , the forcing variable, can be written as: 𝑃 𝐷𝑖 = 1 𝑥𝑖 = 𝑔0 𝑥𝑖 + [𝑔𝑖 𝑥𝑖 − 𝑔0 𝑥𝑖 ]𝑇𝑖 , (6) where 𝑇𝑖 = 1(𝑥𝑖 > 𝑥0 ) (7) Thus, 𝑇𝑖 denotes the point where 𝐸 𝐷𝑖 𝑥𝑖 is discontinuous, for instance when reaching the 1,500 grams threshold (note the similarity between 𝑇 and an instrument 𝑍) Fuzzy Regression discontinuity as IV Assume now for instance that 𝑔0 𝑥𝑖 and 𝑔1 𝑥𝑖 can be described by pth-order polynomials: 𝑝 𝑃 𝐷𝑖 = 1 𝑥𝑖 = 𝛾00 + 𝛾01 𝑥𝑖 + 𝛾02 𝑥𝑖2 + ⋯ + 𝛾0𝑝 𝑥𝑖 (8) 𝑝 +[𝛾0∗ + 𝛾1∗ 𝑥𝑖 + 𝛾2∗ 𝑥𝑖 ∗ + ⋯ + 𝛾𝑝 𝑥𝑖 ] 𝑇𝑖 (9) 2 𝑝 = 𝛾00 + 𝛾01 𝑥𝑖 + 𝛾02 𝑥𝑖 + ⋯ + 𝛾0𝑝 𝑥𝑖 (10) ∗ ∗ ∗ 2 ∗ 𝑝 +[𝛾0 𝑇𝑖 + 𝛾1 𝑥𝑖 𝑇𝑖 + 𝛾2 𝑥𝑖 𝑇𝑖 + ⋯ + 𝛾𝑝 𝑥𝑖 𝑇𝑖. (11) Here, the 𝛾 ∗ ´s are the coefficients of the interactions of 𝑥𝑖 with 𝑇𝑖. The fuzzy RD estimator as IV Returning to the simpler case without interaction terms, the “first stage” regression in this fuzzy RD framework can be written: 𝑝 𝐷𝑖 = 𝛾0 + 𝛾1 𝑥𝑖 + 𝛾2 𝑥𝑖2 + ⋯ + 𝛾𝑝 𝑥𝑖 + 𝜋 𝑇𝑖 + 𝜁1𝑖 , (12) where 𝜋 is the “first-stage” effect of 𝑇𝑖 , i.e of reaching the threshold. We can thus use 𝑇𝑖 as an instruments for 𝐷𝑖 in the equation: 𝑝 𝑦𝑖 = 𝛼 + 𝛽1 𝑥𝑖 + 𝛽2 𝑥𝑖2 + ⋯+ 𝛽𝑝 𝑥𝑖 + 𝜌𝐷𝑖 + 𝜂𝑖 (13) Assuming we would have been able to observe treatment for low birth weight, 𝐷𝑖 would indicate the actual treatment, which would be instrumented by 𝑇𝑖 , i.e. the indicator of reaching the threshold of 1,500 grams. Fuzzy RD as IV Note that just like in the sharp RD case, identification in the fuzzy case depends on the ability to distinguish the discontinuity from the effect of the polynomials 2SLS can be used to construct fuzzy RD estimates. The second-stage of the 2SLS is equal to (13), whereas the first-stage is equal to (12) All the usual threats to IV applies also here The assumptions needed are similar and often only a LATE will be identified Fuzzy RD design example: van der Klaauw (2002) van der Klaauw (2002) was one of the first RD studies in applied econometrics. They used a fuzzy design to evaluate the causal effect of university financial aid on college enrollment. ln their study, 𝑥𝑖 is a numerical score assigned to college applicants based on the objective part of the application information (SAT scores, grades) During the initial stages of the admission process, the applicants are divided into L groups based on discretized values of these scores. Fuzzy RD design example: van der Klaauw (2002) 𝐺𝑖 describes the various groups as follows: 1 𝑖𝑓 0 < 𝑥𝑖 < 𝑐1 2 𝑖𝑓𝑐1 < 𝑥𝑖 < 𝑐2. 𝐺𝑖 = , (14).. 𝐿 𝑖𝑓𝑐𝐿−1 < 𝑥𝑖 Fuzzy RD design example: van der Klaauw (2002) For simplicity, let us focus on the case of group 2 and a cutoff point 𝑐. Having a score of just over 𝑐 will put an applicant in a higher category This will increase the chances of financial aid discontinuously compared to having a score just below 𝑐 Fuzzy RD design example: van der Klaauw (2002) Other components of the application that are not incorporated in the numerical score (such as the essay and recommendation letters) undoubtedly play an important role. ln van der Klaauw (2002), college aid is thus not a deterministic function of the financial aid categories, making this a fuzzy RD design. Nevertheless, there is a clear discontinuity in the probability of receiving an offer of a larger financial aid package at the cutoff point, which is exploited in the RD design. Fuzzy RD as IV example: Angrist and Lavy (1999) Angrist and Lavy use a fuzzy RD design to estimate the effect of class size on children’s test scores They extend the approach in two important ways: First, their causal variable of interest, class size, takes on many values. The first-stage thus exploits jumps in average class size rather than probabilities Second, there is not one discontinuity but many It is a fuzzy design, since the class size rules are not followed perfectly Fuzzy RD as IV example: Angrist and Lavy (1999) The starting point in the study is the observation that class size in Israeli schools is capped at 40. While students in grades with up to 40 students can expect to be in classes as large as 40, grades with 41 students are split into two classes There are multiple thresholds however and grades with 81 students are split into three classes etc Angrist and Lavy call this “Maimonides’ rule” Fuzzy RD as IV example: Angrist and Lavy (1999) The rule can be formally expressed as: 𝑒𝑠 𝑚𝑠𝑐 = (𝑒𝑠 −1) (15) 𝑖𝑛𝑡 +1 40 ln this expression, 𝑚𝑠𝑐 denotes predicted class size in class 𝑐 in school 𝑠, 𝑒𝑠 is (𝑒𝑠 −1) enrollment in the grade, and 𝑖𝑛𝑡 is the integer part of a real number. 40 With enrollment equal to 41 (𝑒𝑠 = 41) predicted class size becomes 20.5. One can plot this function, giving a sawtooth pattern, with discontinuities at integer multiples of 40, see figures on next slide Fuzzy RD as IV example: Angrist and Lavy (1999) The discontinuities in Maimonides’ rule are exploited to construct 2SLS, with the first-stage being written as: 𝑝 𝐶𝑖𝑠𝑐 = 𝛼0 + 𝛼𝑇𝑠 + 𝛽1 𝑒𝑠 + 𝛽2 𝑒𝑠2 + ⋯ + 𝛽𝑝 𝑒𝑠 + 𝜂𝑖𝑠𝑐 , (16) where 𝐶𝑖𝑠𝑐 is 𝑖 ′ s class size in school 𝑠 and class 𝑐, 𝑇𝑠 is whether or not reaching a class size threshold, and 𝑒𝑠 is enrollment in the grade (the forcing variable). ln the second stage, we have: 𝑝 𝑦𝑖𝑠𝑐 = 𝛿0 + 𝛿1 𝐶𝑖𝑠𝑐 + 𝛿2 𝑒𝑠 + 𝛿3 𝑒𝑠2 + ⋯ + 𝛿𝑝 𝑒𝑠 + 𝛾𝑖𝑠𝑐 , (17) where 𝑦𝑖𝑠𝑐 is 𝑖 ′ s test score in school 𝑠 Fuzzy RD as IV example: Angrist and Lavy (1999) The class size thresholds can here be used as an instrument for 𝐶𝑖𝑠𝑐 in the first stage, since: the thresholds affect actual class size, but not perfectly conditional on 𝑒𝑠 (total enrollment), it should not enter in the main equation, i.e the exclusion restriction holds. Fuzzy RD as IV example: Angrist and Lavy (1999) What could go wrong in their study? Selective manipulation could occur if more-educated parents successfully place children in schools with grade enrollments of 41—45, knowing that this will lead to smaller classes in a particular grade. There is no way to know, however, whether a predicted (by the parents) enrollment of 41 will not decline to 38 by the time school starts, obviating the need for two small classes in the relevant grade. What about parents transferring their kids to other schools or opting out from the public school system? Non-parametric fuzzy RD The non-parametric version of fuzzy RD consists of IV estimation in the small neighborhood around the discontinuity Recall the Wald estimator, where we got the IV by dividing the reduced form by the first stage. lt translates to the fuzzy RD setting: 𝑙𝑖𝑚 𝐸 𝑦𝑖 𝑥0 < 𝑥𝑖 < 𝑥0 + 𝛿 −𝐸 𝑦𝑖 𝑥0 − 𝛿 < 𝑥𝑖 < 𝑥0 𝛿→0 𝐸 𝐷𝑖 = 𝜌 (18) 𝑥0 < 𝑥𝑖 < 𝑥0 + 𝛿 −𝐸 𝐷𝑖 𝑥0 − 𝛿 < 𝑥𝑖 < 𝑥0 This gives a LATE estimate of the causal effect among the compliers. Note that this LATE is even more “local” since the estimate is of those close to the threshold Graphical analysis in RD designs (see the recommended article of Imbens & Lemiaux on the reading list) A graphical analysis should be an integral part of any RD study. You should show the following graphs: 1. Outcome by forcing variable (𝑋𝑖 ): This is the standard graph showing the discontinuity in the outcome variable. Construct bins (intervals) and average the outcome within bins on both sides of the cutoff Plot the forcing variable 𝑋𝑖 on the horizontal axis and the average of 𝑌𝑖 for each bin on the vertical axis. Graphical analysis in RD designs You should look at different bin sizes when constructing these graphs In addition, you may also want to plot a relatively flexible regression line on top of the bin means. Inspect whether there is a discontinuity at x0. Inspect whether there are other unexpected discontinuities Example: Outcomes by Forcing Variable From Lee and Lemieux (2010) based on Lee (2008) Example: Outcomes by Forcing Variable – smaller bins Graphical analysis in RD designs 2. Probability of treatment by forcing variable if fuzzy RD. ln a fuzzy RD design, you also want to see that the treatment variable jumps at x0. This tells you that there is a first-stage 3. Covariates by forcing variable. Construct a similar graph to the one before but using a covariate as the “outcome”. There should be no jump in the mean of the covariate at the discontinuity. Example Covariates by Forcing Variable. From Lee and Lemieux (2010) Graphical analysis in RD designs 4. The density of the forcing variable. One should plot the number of observations in each bin. This plot allows to investigate whether there is a discontinuity in the distribution of the forcing variable at the threshold. This would suggest that people can manipulate the forcing variable around the threshold. This is an indirect test of the identifying assumption that each individual has imprecise control over the assignment variable. Example: Density of the forcing variable. From Lee & Lemieux (2010) Testing the continuity of the density of the forcing variable Besides graphically, can we test the continuity of the density of the forcing variable at the discontinuity point? McCrary (2008) suggests a procedure where you in the first step partition the assignment variable into bins and calculate frequencies (number of observations) in the bins. ln the second step you treat those frequency counts as dependent variable in a local linear regression, or polynomial regression, as described above Summary of RD The RD design comes in two forms, sharp and fuzzy The fuzzy RD has an IV interpretation and can be estimated using 2SLS ln both the sharp and fuzzy design, it is important that the threshold cannot be manipulated and that people do not act strategically around the threshold With RD it is always a good idea to report the results graphically, which makes them more compelling Fuzzy RD gives a local average treatment effect The RD design has high internal validity but low external validity. But; we mostly care about internal validity