EC338 Microeconometrics Revision Weeks 6-10 PDF
Document Details
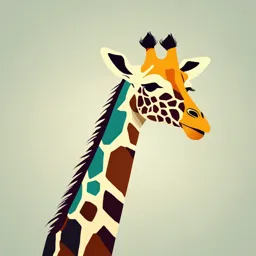
Uploaded by UsefulEmerald368
University of Warwick
Dita Eckardt
Tags
Summary
This document provides a revision summary of topics covered in a microeconometrics course, focusing on instrumental variables, regression discontinuity design, binary outcomes, and multinomial and ordered models. These topics are key concepts in economics.
Full Transcript
EC338 - Microeconometrics Weeks 6-10 Brief Revision Dita Eckardt Department of Economics University of Warwick Weeks 6-10 Revision Topics I Instrumental Variables I Regression Discontinuity Design I Binary Outcomes I Multinomial and Ordered Models...
EC338 - Microeconometrics Weeks 6-10 Brief Revision Dita Eckardt Department of Economics University of Warwick Weeks 6-10 Revision Topics I Instrumental Variables I Regression Discontinuity Design I Binary Outcomes I Multinomial and Ordered Models I Selection Models Weeks 6-10 Revision Instrumental Variables IV Revision The IV setup I The instrument Zi : dummy variable equal to one if i is offered a seat at KIPP I The treatment variable Di : dummy variable equal to one if i attends KIPP I The outcome variable Yi : fifth-grade math scores for student i I Causal chain reaction: Zi (instrument) =⇒ |{z} Di (treatment) =⇒ |{z} Yi (outcome) first stage effect:φ effect of interest:λ | {z } reduced form effect:ρ IV uses first stage and reduced form effect → effect of interest IV Revision IV assumptions I For IV to work, three assumptions need to be satisfied 1) First stage I The instrument Zi has a causal effect on the treatment Di I Equivalent to φ being non-zero: can check this in the data 2) Random assignment I The instrument Zi is as good as randomly assigned I Cannot be tested, but balance checks can help support this assumptions 3) Exclusion restriction I The instrument Zi affects Yi only through Di I Cannot be tested if only have one instrument Zi IV Revision Local Average Treatment Effect (LATE) I This applies to case with heterogeneous treatment effects I The effect of interest is the Local Average Treatment Effect (LATE) I Measures the treatment effect for the subsample of compliers: individuals who change treatment status because of the instrument I Subsample of compliers depends on the instrument and setting I LATE for binary instrument and treatment: E [Yi |Zi = 1] − E [Yi |Zi = 0] ρ λ= = E [Di |Zi = 1] − E [Di |Zi = 0] φ Weeks 6-10 Revision Regression Discontinuity Designs RDD Revision The RDD setup I Running variable a: variable determining treatment according to threshold: age I Treatment variable Da : dummy variable equal to one if can drink legally ( 1 if a ≥ 21 Da = 0 if a < 21 Sharp RDD has treatment switch on cleanly with cutoff I Outcome variable M̄a : average mortality rates at age a I We are interested in estimating the following equation: M̄a = α + ρDa + γa + ea RDD Revision Fuzzy RDD I Fuzzy RDD exploits discontinuities in the prob. of treatment at a cutoff I Define Ti as a dummy variable for making the cutoff ( 1 if xi ≥ x0 Ti = 0 if xi < x0 I Recall our outcome equation: Yi = α1 + λDi + γ1 xi + where - Yi is student i’s earnings - Di = 1 if attend Harvard - xi is student i’s GRE I A fuzzy RDD setup allows us to instrument for Di (attending Harvard) using Ti (making the GRE cutoff) RDD Revision Difference between sharp and fuzzy RDD I Sharp RDD - Treatment is a deterministic function of the running variable: everyone above 21 is allowed to drink legally, everyone below not - Treatment goes from 0 to 1 at the cutoff - Examples include elections, country boarders, age policies I Fuzzy RDD - Treatment is not deterministic function of the running variable: some below cutoff still go to Harvard, some above don’t - Treatment probability or intensity changes at the cutoff - Conditional on running variable, treatment is endogenous RDD Revision Fuzzy RDD is IV I Intuition is that treatment becomes more likely to the right of the cutoff, just like the offer of a KIPP seat made it more likely to attend KIPP I Unlike sharp RDD, where treatment switches on at cutoff, we need to scale the fuzzy RDD estimate by fraction getting treated due to cutoff I Outcome equation: Yi = α1 + λDi + γ1 xi + i I First stage equation: Di = α2 + φTi + γ2 xi + ξi I 2SLS second stage: Yi = α1 + λ2SLS D̂i + γ1 xi + i Weeks 6-10 Revision Binary Outcomes Binary Outcomes Revision Linear Probability Model (LPM) I In a LPM, we have X P(Yi = 1|xi ) = E (Yi |xi ) = β0 + βj xij j I This naturally leads to a linear regression model X Yi = β0 + βj xij + i j I The regression fitted values will give us an estimate of the probability of completing high school, conditional on all covariates X P̂(Yi = 1|xi ) = Ŷi = βˆ0 + β̂j xij j I The problem with the LPM is that these estimated probabilities could be negative or greater than 100%, which is hard to interpret Binary Outcomes Revision Probit and logit models I If non-linear function G (xi β) ∈ [0, 1] ⇒ P̂(Yi = 1|xi ) ∈ [0, 1] I Two non-linear functions G (xi β) are of particular interest - Probit: R xi β 1 G (xi β) = Φ(xi β) = −∞ = φ(v )dv , φ(v ) = (2π)− 2 exp(−v 2 /2) (the cdf of the normal distribution) - Logit: exp(xi β) G (xi β) = Λ(xi β) = 1+exp(xi β) (the cdf of the logistic distribution) I These are the most common for binary choice in applied research Binary Outcomes Revision Probit and logit models I With non-linear functions, marginal effects are no longer βj I Recall that the probability of event Yi = 1 is P(Yi = 1|xi ) = G (xi β) I This means that marginal effects are given by ∂P(Yi = 1|xi ) = G 0 (xi β)βj = g (xi β)βj ∂xij where g (xi β) = G 0 (xi β) I Note that the marginal effects depend on xi Binary Outcomes Revision Maximum Likelihood Estimation I In our binary discrete choice model I In that case, P(Yi = 1|xi ) = G (xi β) and we have n Y L(β) = G (xi β)Yi [1 − G (xi β)]1−Yi i=1 I The log-likelihood is given by n X n X ln L(β) = Yi ln[G (xi β)] + (1 − Yi ) ln[1 − G (xi β)] i=1 i=1 n n = 1[Yi = 1] ln[G (xi β)] + 1[Yi = 0] X X ln[1 − G (xi β)] i=1 i=1 I You will not need to do this manually in your applied work - econometric packages can implement this for you! Weeks 6-10 Revision Multinomial and Ordered Models Multinomial Models Revision Multinomial logit I Recall that in the binary logit model exp(xi β) P(Yi = 1|xi ) = 1 + exp(xi β) I In the multinomial logit model, we have exp(xi βk ) P(Yi = k|xi ) = PK for k 6= 0 1+ h=1 exp(xi βh ) K K X X exp(xi βk ) P(Yi = 0|xi ) = 1 − P(Yi = k|xi ) = 1 − PK k=1 k=1 1+ h=1 exp(xi βh ) PK k=1 exp(xi βk ) 1 =1− P K = PK 1 + h=1 exp(xi βh ) 1 + h=1 exp(xi βh ) I Second inequality follows since probabilities need to sum to one (equivalent to normalising xi β0 = 0) I Note that the βk coefficients depend on k Multinomial Models Revision Multinomial logit I Marginal effect of alternative k wrt covariate j is given by PK ∂P(Yi = k|xi ) h=1 exp(xi βh )βjh h i = P(Yi = k|xi ) βjk − PK ∂xij 1 + h=1 exp(xi βh ) I For example, how does the probability of choosing premium plan (alternative k) change when income (covariate j) increases? I No longer necessarily has the same sign as βjk ! Multinomial Models Revision Multinomial vs. conditional logit I Multinomial logit: - xi variables specific to individuals, but don’t vary with alternatives - βk varies with alternatives - For example, occupational choice where xi includes education, experience, etc. and effect of education differs across occupations I Conditional logit: - xik variables vary with alternatives - β does not vary with alternatives - For example, mode of transport choice where cost, time, comfort, etc. differs across alternatives, but effect on utility same Ordered Models Revision I Ordered models: order of options is informative about outcomes - Survey data: how would you rate our service today? - very poor=1, poor=2, good=3, very good=4 - relabelling would break up the natural ordering of the outcomes I Back to our latent variable model (e.g. measure of satisfaction) Yi∗ = xi β + ei , ei ∼ N(0, 1) where, for a set of cut-off parameters α1 ,..., αK ∗ 0 if Yi ≤ α1 1 if α1 < Yi∗ ≤ α2 Yi =. .. K if Yi∗ > αK I Note that there are no alternative-specific xi ’s or β’s Ordered Models Revision Ordered probit I The choice probabilities are given by P(Yi = 0|xi ) = P(Yi∗ ≤ α1 ) = P(xi β + ei ≤ α1 |xi ) = Φ(α1 − xi β) P(Yi = 1|xi ) = P(α1 < Yi∗ ≤ α2 ) = Φ(α2 − xi β) − Φ(α1 − xi β)... P(Yi = K |xi ) = P(Yi∗ > αK ) = 1 − Φ(αK − xi β) where Φ(·) is the cdf of the standard normal distribution I Marginal effects are given by ∂P(Yi = 0|xi ) = −βj φ(α1 − xi β) ∂xij ∂P(Yi = k|xi ) = −βj [φ(αk+1 − xi β) − φ(αk − xi β)], k = 1,..., K − 1 ∂xij ∂P(Yi = K |xi ) = βj φ(αK − xi β) ∂xij so sign of βj only informative for alternatives 0 and K