Hypothesis Testing Steps - Lecture 6 PDF
Document Details
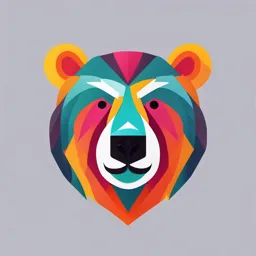
Uploaded by MasterfulNeptunium
Imam Abdulrahman Bin Faisal University
Tags
Summary
This document details the steps involved in hypothesis testing, including defining hypotheses, selecting test statistics, and interpreting results. It also discusses the importance of considering both statistical and economic significance, examining the practical implications of findings.
Full Transcript
I. Hypothesis Testing Steps: The process involves: 1. State the hypothesis: Defining the null hypothesis (H₀, what's being tested, contains =, ≥, or ≤) and the alternative hypothesis (H₁, what the researcher wants to conclude, suggests a difference compared to H₀). The example used is...
I. Hypothesis Testing Steps: The process involves: 1. State the hypothesis: Defining the null hypothesis (H₀, what's being tested, contains =, ≥, or ≤) and the alternative hypothesis (H₁, what the researcher wants to conclude, suggests a difference compared to H₀). The example used is whether reward and incentive schemes increase corporate profits. 2. Select a test statistic: Choosing a metric (e.g., mean, standard deviation) appropriate for the data and hypothesis. 3. Specify the significance level (α): Setting the acceptable probability of rejecting H₀ when it's actually true (Type I error). A common value is 5% (0.05). 4. State the decision rule: Defining the criteria (critical values) for rejecting H₀ based on the test statistic. 5. Collect data and calculate statistics: Gathering a sample and computing the test statistic. 6. Make a decision about the hypothesis: Comparing the test statistic to the critical value(s). If the test statistic exceeds the critical value (or falls outside a range of critical values), H₀ is rejected. Otherwise, H₀ is not rejected (we fail to reject H₀). 7. Make a decision based on test results: Concluding whether there is sufficient evidence to support H₁. II. One-Tailed vs. Two-Tailed Tests: One-tailed test: Used when testing for a specific direction (greater than or less than). The example used was testing if the return on stock options is greater than zero. Two-tailed test: Used when testing for any difference (greater than or less than). The example used was testing if the return on stock options is simply different from zero. Most hypothesis tests in practice are two-tailed due to their greater flexibility in detecting differences in either direction. III. Test Statistic and Critical Values: The test statistic, calculated from sample data, is compared to critical values to determine whether to reject H₀. Critical values are thresholds defining the boundary for rejecting H₀. They are similar to confidence intervals, defining a range where H₀ is accepted. IV. Type I and Type II Errors: Type I error: Rejecting H₀ when it's true (false positive). The probability of making a Type I error is equal to α (the significance level). Type II error: Failing to reject H₀ when it's false (false negative). The probability of making a Type II error is denoted by β. V. Statistical vs. Economic Significance: Statistical significance (p-value < α) only indicates the result is unlikely to have happened by chance; it doesn't guarantee the result is practically meaningful or impactful. Economic significance considers the practical implications of the results, including factors like: Transaction costs Taxes Risk A result can be statistically significant but not economically significant if the magnitude of the effect is small relative to costs, taxes, or risks, making it not worth pursuing. An example illustrates an investment with a statistically significant, yet economically insignificant, return.