Lecture 4- Interval Estimation PDF
Document Details
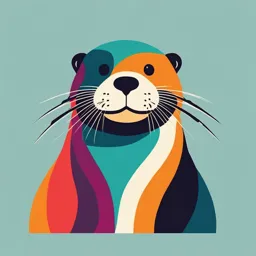
Uploaded by ReasonableDerivative
University of Southampton
2024
Tags
Summary
This document discusses interval estimation, focusing on the normal distribution and t-distribution. Hypothesis testing and confidence intervals are also covered.
Full Transcript
Lecture 4- interval estimation Friday, 25 October 2024 2:34 PM The normal distribution Confidence intervals An interval that (with a specific probability) contains the true prameter value b2 95% confidence e.g - ○ The t-distribution The t-d...
Lecture 4- interval estimation Friday, 25 October 2024 2:34 PM The normal distribution Confidence intervals An interval that (with a specific probability) contains the true prameter value b2 95% confidence e.g - ○ The t-distribution The t-distribution is a bell shaped curve centred at zero Looks like the standard normal distribution, except it's more spread out, with a larger variance and thicker tails The shape of the t-distribution is controlled by a parameter - the degrees of freedom As df tend to infinity, t,df tends to a standard normal distribution Obtaining interval estimates Hypothesis test Components of hypothesis testing - A null hypothesis H0 ○ Specifies a value for a regression parameter ○ Stated - H0: bk=c, where c is a constant and is a certain value of interest in the context of a specific regression model - An alternate hypothesis H1 ○ Accept if the null hypothesis is rejected ○ H1: bk < or > or not equal to c - A test statistic ○ Accept if the null hypothesis is rejected ○ H1: bk < or > or not equal to c - A test statistic ○ - A rejection region ○ Set of values that low probability of being observes under the null, so if they happen we are led to conclude that the null hypothesis, H0 must have been false ○ The rejection region depends on the form of the alternative ○ It is possible to construct a rejection region once we have ▪ A test statistic with a known distribution under the null ▪ An alternate hypothesis ▪ A level of significance - A conclusion ○ We decide to reject or not to reject the null hypothesis ○ The decision is correct if ▪ The null hypothesis is false and we decide to reject it ▪ The null hypothesis is true and we decide not to reject it ○ The decision is incorrect if ▪ The null hypothesis us true and we decide to reject it (type 1 error) ▪ The null hypothesis is false and we decide not to reject it (type 2 error) ○ Type 1 and type 2 errors We would like both a and b to be as small as possible but there is a trade-off between them The probability of b - type 2 error- varies inversely with the level of significance of the test a, the probability of a type 1 error. Choosing to make a Type 1 and type 2 errors We would like both a and b to be as small as possible but there is a trade-off between them The probability of b - type 2 error- varies inversely with the level of significance of the test a, the probability of a type 1 error. Choosing to make a smaller, increases the probabilityof b The power of the test depends on two main aspects - If the null hypothesis is bk=c, and if the true value of b I snot c, but close, then the probability of a type 2 error is high - The smaller the variance of the estimator, the lower the probability of a type 2 error, given the probability of a type 1 error ○ If the estimator is very precise and I fail to reject it, it must be that the null is true or the alternate is very close to the null. ○ Why we 'fail to reject' rather than just 'accept' A statistical test procedure cannot prove the truth of a null hypothesis. When we fail to reject a null hypothesis, all the hypothesis test can establish is that the information in a sample of data is compatible with the null hypothesis. On the other hand, a statistical test can lead us to reject the null hypothesis, with only a small probability α of rejecting the null hypothesis when it is actually true. Thus rejecting a null hypothesis is a stronger conclusion than failing to reject it. The p value Probability of getting a tests statistic, as large as the value we got from the sample, ror even more extreme, given that H0 is true. - pv = P(t > t∗|H0) The p-value rule: reject the null hypothesis when the p-value is less than, or equal to, the level of significance α. That is, if pv ≤ α then reject H0. If pv > α then do not reject H0. If t is the calculated value of the test-statistic, then: - If H1 :βk >c,pv =probability to the right of t - If H1 :βk