Data Visualizations PDF
Document Details
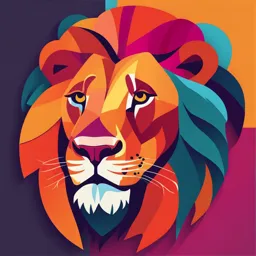
Uploaded by JollyHyperbolic
Tags
Summary
This document explains various data visualization techniques, including bar charts, line graphs, pie charts, scatter plots, and heatmaps. It also describes box-and-whisker plots, their components, and how to use them to understand data distribution and identify outliers. Diagrams are included.
Full Transcript
**Illustrate any five specific data visualizations of your choice in detail. (draw the diagram)** Ans. **DATA VISUALISATION:** - Data visualization is the graphical representation of information and data using visual elements like charts, graphs, maps, and other visuals. - It helps c...
**Illustrate any five specific data visualizations of your choice in detail. (draw the diagram)** Ans. **DATA VISUALISATION:** - Data visualization is the graphical representation of information and data using visual elements like charts, graphs, maps, and other visuals. - It helps communicate data insights, patterns, trends, and relationships clearly and effectively by transforming complex datasets into visual formats that are easier to understand and interpret. **Purpose of Using Data Visualization** The primary purposes of using data visualization include: 1. **Simplifying complex data**: Visuals make large datasets easier to digest by summarizing key insights at a glance. 2. **Spotting trends and patterns**: Visualizations help quickly identify trends, outliers, and patterns that might not be obvious from raw data. 3. **Enhancing decision-making**: Visuals present data in a way that enables faster and more informed decisions by highlighting key performance indicators (KPIs), comparisons, and projections. 4. **Communicating insights effectively**: Data visualizations communicate information clearly to both technical and non-technical audiences, helping teams understand key points without needing to analyze raw data. 5. **Identifying relationships and correlations**: Certain visualizations, like scatter plots, help to explore relationships between variables, showing correlations or causality. 6. **Storytelling with data**: Data visualization aids in narrating a clear and compelling story with data, making it easier for stakeholders to follow and understand the context behind the numbers. **Applications of Data Visualization** Data visualization is used across a wide range of fields and industries to interpret and communicate data. Here are some common applications: 1. **Business Intelligence and Analytics**: - Track company performance (e.g., sales, revenue, profit). - Analyze key performance indicators (KPIs) across various departments (e.g., marketing, sales, finance). - Identify trends in consumer behavior, product performance, or sales cycles. 2. **Healthcare**: - Visualize patient data, treatment outcomes, and healthcare trends. - Track disease outbreaks or healthcare resource allocation. - Analyze medical research findings. 3. **Finance**: - Monitor financial markets and investment performance. - Visualize stock trends, portfolio allocations, and risk analysis. - Track financial metrics like revenue growth, profitability, and costs. 4. **Marketing**: - Analyze customer behavior, campaign performance, and ROI. - Visualize data on social media engagement, website traffic, and conversions. - Segment markets and identify customer demographics and preferences. 5. **Government and Public Policy**: - Track and visualize public data such as crime rates, population growth, and employment statistics. - Visualize the impact of public policies or spending decisions. - Monitor resource allocation in areas like education, transportation, or healthcare. 6. **Education**: - Visualize student performance metrics and trends. - Track attendance, graduation rates, and standardized test results. - Present complex research findings and educational outcomes. Basic visualization techniques help us understand data quickly by representing it in charts, graphs, and plots. Here are some common types: - A bar chart is a graphical representation that uses rectangular bars to show the quantity or frequency of different categories. - The length of each bar is proportional to the value it represents. - Bar charts are commonly used for comparing discrete categories or groups. **Purpose**: - To compare the sizes or values of different categories. - It shows discrete data points clearly, making it easy to see which category has the largest or smallest value. **Usage**: - **Example**: If you're analyzing product sales by category, a bar chart will show you which product category (e.g., electronics, clothing) has the highest and lowest sales. - **Best for**: Simple comparisons across different categories, where you want to highlight differences between them. **Steps**: - **Step 1**: Open Tableau and connect to your dataset (e.g., Sales data). - **Step 2**: Drag the **Category** (e.g., Products) to the **Columns** shelf. - **Step 3**: Drag the **Measure** (e.g., Sales) to the **Rows** shelf. - **Step 4**: Tableau will automatically generate a bar chart. You can adjust the **Marks** to change color or add labels. - To show trends over time or continuous data. - Helps to see how data changes over a period and spot upward or downward trends. - **Example**: Tracking sales performance month by month. A line graph can show whether sales are increasing, decreasing, or fluctuating. - **Best for**: Displaying trends, seasonality, or changes over time, such as sales over years or website traffic growth over months. - **Step 1**: Connect to your dataset (e.g., Time series data). - **Step 2**: Drag the **Date** field to the **Columns** shelf. - **Step 3**: Drag the **Measure** (e.g., Sales or Temperature) to the **Rows** shelf. - **Step 4**: Tableau will generate a line graph. Use the **Marks** section to adjust line thickness or add labels. - **Step 5**: Customize by adding filters to view data for specific time periods. - To show proportions or percentages of a whole. - Pie charts help visualize how different categories contribute to a total. - **Example**: If you want to show how much each department contributes to the total company profit, a pie chart can break it down by department, with each slice representing the proportion of profit. - **Best for**: Visualizing relative proportions in a dataset. However, it\'s most effective when comparing a few categories. Too many slices can make it hard to interpret. - **Step 1**: Connect to your dataset. - **Step 2**: Drag the **Dimension** (e.g., Product Category) to the **Columns** shelf. - **Step 3**: Drag the **Measure** (e.g., Sales or Profit) to the **Rows** shelf. - **Step 4**: Select the **Pie Chart** option from the **Marks** menu. - **Step 5**: Adjust the size of the pie and label each slice by dragging the **Measure Names** to **Labels**. - To show the relationship or correlation between two variables. - Scatter plots help in identifying whether there's a positive, negative, or no correlation between the variables. - **Example**: Comparing sales and profits across different regions. If there\'s a positive relationship, higher sales will typically correspond with higher profits. - **Best for**: Identifying relationships between two quantitative variables, like marketing spend vs. revenue or height vs. weight in a health dataset. - **Step 1**: Connect to your dataset. - **Step 2**: Drag one **Measure** (e.g., Sales) to the **Columns** shelf. - **Step 3**: Drag another **Measure** (e.g., Profit) to the **Rows** shelf. - **Step 4**: Change the **Marks** type to **Circle**. - **Step 5**: Drag a **Dimension** (e.g., Region or Product) to **Color** to differentiate between categories. - **Step 6**: Add labels by dragging the **Measure Names** to **Labels**. **Purpose**: - To show data distribution or relationships between two dimensions using color. - A heatmap helps highlight patterns or correlations between categories, especially when dealing with large amounts of data. **Usage**: - **Example**: Showing sales by product category across different regions. Darker colors can represent higher sales, making it easy to spot which region and product combination performs best. - **Best for**: Visualizing the strength of relationships between two variables and identifying patterns in complex data, such as sales volume across multiple dimensions (e.g., region and product category). **Steps**: - **Step 1**: Connect to your dataset. - **Step 2**: Drag one **Dimension** (e.g., Product Category) to the **Columns** shelf. - **Step 3**: Drag another **Dimension** (e.g., Region) to the **Rows** shelf. - **Step 4**: Drag a **Measure** (e.g., Sales or Profit) to **Color** in the **Marks** card. - **Step 5**: Adjust the color palette to visually represent the data differences (e.g., darker colors for higher values). 2. **ANALYZE THE NEED FOR BOX-PLOT. WITH A NEAT SKETCH EXPLAIN IN DETAIL ABOUT THE BOX-AND-WHISKER PLOT AND ITS USES.(DRAW THE DIAGRAM)** **NEED FOR A BOX-PLOT** 3. **EXPLAIN THE COMPONENTS AND IMPORTANCE OF BOX PLOT ANALYSIS**. A Box-and-Whisker Plot (or simply Box-Plot) is important because: 1. Summarizes Data Distribution: It provides a visual summary of a dataset's distribution, including the spread and skewness of the data. 2. Identifies Outliers: Box-plots help easily spot outliers (data points that are far away from the rest of the data). 3. Shows Data Spread: It displays how data is spread across different quartiles, helping to understand variability. 4. Compares Multiple Datasets: You can compare the distributions of multiple datasets side by side using box-plots. Explanation of Box-and-Whisker Plot Here's a breakdown of the parts of a box-plot with a diagram: 1\. **Components of a Box-Plot** - Minimum: The smallest value, excluding outliers. - Lower Quartile (Q1): The 25th percentile, meaning 25% of the data falls below this value. - Median (Q2): The middle value of the data, splitting the data into two halves. - Upper Quartile (Q3): The 75th percentile, meaning 75% of the data falls below this value. - Maximum: The largest value, excluding outliers. - Whiskers: Lines that extend from the box to the minimum and maximum values within 1.5 times the interquartile range (IQR). - Outliers: Data points that fall outside the whiskers, represented by individual dots. 2\. **Interquartile Range (IQR)** - IQR: It is the range between the upper quartile (Q3) and lower quartile (Q1), and it shows the middle 50% of the data. 3\. Whiskers - The whiskers extend from the box to the smallest and largest data points within 1.5 times the IQR. Data points outside this range are considered outliers. https://media.labxchange.org/xblocks/lb-LabXchange-d8863c77-html-1/211626365402575-b88c4d0fdacd5abb4c3dc2de3bc004bb.png Box-Plot Diagram Example: The box-plot looks like a rectangular box with lines (whiskers) extending out from both ends, and any outliers are shown as dots beyond the whiskers. **Uses of Box-and-Whisker Plot** 1. Understanding Data Distribution: - The box-plot shows how the data is distributed across the lower quartile, median, and upper quartile. 2. Detecting Outliers: - Easily spot outliers as they appear as individual points outside the whiskers. 3. Comparing Datasets: - By plotting multiple box-plots side by side, you can compare the spread, medians, and presence of outliers across different datasets. 4. Visualizing Skewness: - If the median is closer to one side of the box, it indicates that the data may be skewed. Point-by-Point Summary: - Minimum: The smallest value. - Q1 (Lower Quartile): 25% of the data is below this point. - Median: The middle value of the dataset. - Q3 (Upper Quartile): 75% of the data is below this point. - Maximum: The largest value, excluding outliers. - Whiskers: Extend to the minimum and maximum data points within the range. - Outliers: Points that fall outside of the whiskers**.** 4. **SUMMARIZE THE STEPS IN DETAIL ABOUT HOW TO DESIGN INTERACTIVE DASHBOARDS IN TABLEAU**. (draw the Dashboard diagram) 5. **IDENTIFY AND EXPLAIN THE IMPORTANT STEPS FOR BUILDING EFFECTIVE DASHBOARDS.** **DASHBOARDS** - A dashboard is a visual display that consolidates and presents key information in an easily understandable format. - It serves as a centralized interface for monitoring, analyzing, and visualizing data from various sources, allowing users to make informed decisions based on the insights derived from that data. **Purpose** - **Data Monitoring**: Dashboards provide a quick overview of key metrics and performance indicators, enabling users to monitor progress and performance at a glance. - **Decision Support**: They help in making informed decisions by presenting relevant data insights and trends. **Designing interactive dashboards** - Designing interactive dashboards refers to the process of creating visual data representations that allow users to engage with and explore data dynamically. - These dashboards serve as powerful tools for data analysis, enabling users to derive insights and make informed decisions based on the data presented. - Here are the key elements that define what it means to design interactive dashboards: **1. User Engagement** - **Interactivity**: Users can interact with the dashboard elements (e.g., filters, buttons, and visualizations) to manipulate data views. This engagement helps users explore the data more thoroughly and focus on areas of interest. - **Customizability**: Dashboards often allow users to customize their views based on their preferences, such as selecting different date ranges or metrics. **2. Visual Representation of Data** - **Data Visualization**: Dashboards utilize charts, graphs, maps, and other visual elements to represent data. This visual representation helps users quickly grasp complex data and identify trends, patterns, and outliers. - **Clarity and Readability**: The design should prioritize clear and intuitive layouts, making it easy for users to understand the information presented. **3. Data Integration** - **Multiple Data Sources**: Interactive dashboards often integrate data from various sources, providing a holistic view of information. This can include databases, spreadsheets, APIs, and other data sources. - **Real-Time Updates**: Many dashboards can pull in live data or refresh data periodically, allowing users to work with the most current information available. **4. Dynamic Exploration** - **Filters and Drill-Downs**: Users can apply filters to view specific subsets of data, and they can drill down into detailed views to analyze specific data points or segments. - **Actions and Highlights**: Users can interact with data points to see related information or highlight specific trends, making it easier to analyze complex datasets. **5. Insight Generation** - **KPI Tracking**: Dashboards often focus on key performance indicators (KPIs) relevant to the user's goals, enabling quick assessment of performance. - **Storytelling**: Well-designed dashboards guide users through a narrative using the data, helping them understand the context and implications of the information presented. **6. Usability and Accessibility** - **Responsive Design**: Dashboards should be designed to work across different devices (e.g., desktops, tablets, mobile phones), ensuring accessibility for all users. - **User-Centric Design**: The design process should consider the needs, preferences, and skill levels of the target audience to ensure the dashboard is user-friendly. **7. Maintenance and Adaptability** - **Scalability**: As business needs evolve, dashboards should be easily updatable to accommodate new data, metrics, or visualizations. - **Feedback Mechanism**: Collecting user feedback helps to refine the dashboard over time, enhancing its functionality and effectiveness. 6. **Enlist the different types of Filters in Tableau. Explain any four filters of your choice in detail.** 7. Briefly discuss about the different types of Filters in Tableau. In Tableau, filters are essential tools that allow you to limit and refine the data you analyze and visualize. Each type of filter serves a different purpose and can be applied in various ways to focus on specific data subsets. Here's a detailed explanation of the six main types of filters in Tableau, along with simple examples and their purposes: **1. Context Filter** - **Purpose**: A context filter is used to set a context for other filters. It defines the data subset that subsequent filters will act upon. This can improve performance and simplify complex filtering scenarios. - **Example**: Suppose you have a dataset of sales across various regions and years. You can set a context filter to show data only for the year 2023. Any other filters you apply afterward (like filtering by region) will only consider data from 2023. **2. Extract Filter** - **Purpose**: Extract filters are used when creating a Tableau Data Extract (TDE or Hyper file). They limit the data included in the extract, which can improve performance and reduce file size. - **Example**: If you have a large database containing sales data for multiple years, you can create an extract filter to include only sales data from the last two years. This way, your extract will only contain the most relevant data. **3. Data Source Filter** - **Purpose**: Data source filters are applied at the data source level. They restrict data before it is brought into Tableau, affecting all worksheets using that data source. - **Example**: If you have multiple sales regions in your dataset, you might want to set a data source filter to include only data for the \"North America\" region. All visualizations using that data source will only reflect data from North America. **4. Dimension Filter** - **Purpose**: Dimension filters allow you to filter data based on specific categorical values (dimensions). This is useful for filtering data by specific attributes like categories or labels. - **Example**: If you have a dataset of products, you can use a dimension filter to show only \"Electronics\" products. This will exclude all other product categories from your analysis. **5. Measure Filter** - **Purpose**: Measure filters are used to filter data based on quantitative values (measures). This is useful when you want to focus on a specific range of values. - **Example**: If you have a dataset of sales data, you can apply a measure filter to show only those sales where the revenue is greater than \$10,000. This filters out any sales that do not meet this threshold. **6. Table Calculation Filter** - **Purpose**: Table calculation filters allow you to filter data based on the results of a calculation performed on the data in a visualization. This is useful for filtering based on computed metrics rather than raw data. - **Example**: If you have a calculation that computes the running total of sales, you can use a table calculation filter to show only those months where the running total is greater than a specific value (e.g., \$50,000). This helps in focusing on periods of high cumulative sales. - \(i) Gantt Chart - \(ii) Highlight Table i. Gantt Chart : **Gantt Chart:** Typically used in project management, Gantt charts are a bar chart depiction of timelines and tasks. - Gantt charts are primarily used in project management to visualize time duration for events or activities. - As a project management tool, Gantt charts make the interdependencies between tasks visually apparent and illuminate the work flow schedule. - They can also be used to creatively display time being spent doing an activity, whether for a business's product delivery times or maybe how much time you spend watching tv.  **Tips for Formatting Gantt Charts:** - Add horizontal borders between rows to make Gantt charts easier to read. - Use colour to differentiate between tasks or other dimension break-downs. - Format bars to fill the width of the row to make the relationship between tasks easier to view. **Highlight Table:** A form of table that uses colour to categorize similar data, allowing the viewer to read it more easily and intuitively. - Highlight tables and heat maps use colour to help visualize data displayed as a text table (crosstab or tabular view chart). - Highlight tables enhance text tables while keeping their form. - They encode ranges of measure values with the preattentive attribute of colour; from lowest to highest. - These tables can display either continuous colours using sequential or diverging palettes. - They can also use a stepped array of colours. - A diverging colour palette utilizes different colours to highlight crossing a meaningful threshold. - An example would be going from positive to negative values. - A sequential colour palette varies the intensity of a single colour to highlight rank. - Highlight tables display data in a text table. Using colour, they speed up how you identify the most important numbers within a range of values. These tables also have rows and columns to depict different dimensions. - Highlight tables are text tables enhanced through the use of color to show high and low values. **9. Write short notes on the following data visualizations** **(i) Histogram** **(ii) Pie Chart** **Histogram:** A type of bar chart that split a continuous measure into different bins to help analyze the distribution. - A histogram uses bars to visualize the distribution of data for how many things, people, or occurrences happened between a range of values on an axis. - While histograms look like bar charts, they are different in that each bar is an interval of values of a metric. - These bars are called bins or buckets, and together they represent what is called a frequency distribution. - A frequency distribution is the display of how often something occurred in a graph, table, or diagram. - Histograms are graphs and are one way to visualize frequency distributions. - Histograms are useful for analyzing numerical data sets. Analysts and statisticians use them to analyze patterns of frequency, and visualize a numerical breakdown of what is being collected in the data. - Histograms allow you to quickly understand the distribution of data. Depending on the data itself, where the frequency of distribution peaks and drops will tell you valuable information.  **Pie Chart:** A circular chart with triangular segments that shows data as a percentage of a whole. - A pie chart helps organize and show data as a percentage of a whole. - True to the name, this kind of visualization uses a circle to represent the whole, and slices of that circle, or "pie", to represent the specific categories that compose the whole. - This type of chart helps the user compare the relationship between different dimensions (Ex. categories, products, individuals, countries, etc.) within a specific context. - Usually, the chart splits the numerical data (measure) into percentages of the total sum. Each slice represents the proportion of the value, and should be measured accordingly. - Pie charts should be used to show the relationship of different parts to the whole. They work best with dimensions that have a limited number of categories. - To read a pie chart, you must consider the area, arc length, and angle of every slice. Because it can be hard to compare the slices, meaningful organization is key. - Slices in a pie chart should be organized in a coherent way, usually the biggest to smallest, to make it easier for the user to interpret. - Start at the biggest piece and work your way down to the smallest to properly digest the data. - The colors of the slices should match their respective blocks in the legend, so that viewers do not need to consult the legend as much. - If you have a dimension with just a couple of categories to compare, then a pie chart can help display each value of a category within the whole. - The chart should read as a comparison of each group to each other, forming a whole category. - The "whole" could be anything so long as the category can be split into separate slices that are distinguishable from each other. **Use a pie chart if:** - You have a total number that can be split up into 2-5 categories. - One category outweighs the other by a significant margin. **Do not use a pie chart if:** - Your dimension has too many categories. - Similar percentages/numbers exist between different values within the chosen dimension. - Data doesn't represent a uniform "whole", or the percentages don't measure to 100 percent. - There are negative values or complex fractions in your measure value.   UNIT- IV 1. Analyze the role of variables and distribution in univariate analysis in detail. **Univariate Analysis** Univariate analysis involves the examination and analysis of one variable at a time. The primary goal of this type of analysis is to describe the variable\'s central tendency, distribution, dispersion, and overall pattern. Key aspects of univariate analysis include: 1. **Measures of Central Tendency**: - **Mean**: The average value of the data. - **Median**: The middle value when the data is ordered. - **Mode**: The most frequent value in the dataset. 2. **Measures of Dispersion**: - **Range**: The difference between the maximum and minimum values. - **Variance**: The average squared deviation from the mean. - **Standard Deviation**: The square root of variance, indicating the spread of the data. - **Interquartile Range (IQR)**: The range between the first quartile (Q1) and third quartile (Q3), highlighting the middle 50% of the data. 3. **Distribution**: - **Shape**: The distribution can be symmetric, skewed (left or right), or have other characteristics like bimodality. - **Skewness**: Describes the asymmetry of the distribution (positive or negative skew). - **Kurtosis**: Describes the \"tailedness\" of the distribution (leptokurtic, platykurtic). **Types of Data in Univariate Analysis** 1. **Categorical Data**: Variables that represent categories or labels (e.g., gender, city, etc.). - Example: The frequency of students in different academic majors. - Common visualizations: Bar plots, pie charts. 2. **Numerical Data**: Variables that represent continuous or discrete numbers (e.g., height, weight, test scores). - Example: The distribution of exam scores in a class. - Common visualizations: Histograms, box plots, density plots. **Univariate Analysis Techniques and Visualizations** 1. **For Categorical Data**: - **Frequency Distribution**: Lists each category and the number of occurrences. - **Bar Charts**: Represent categories on the x-axis and their counts or percentages on the y-axis. - **Pie Charts**: Show proportions of each category as parts of a whole. 2. **For Numerical Data**: - **Histograms**: Display the frequency distribution of a continuous variable by dividing the data into bins. - **Box Plots**: Summarize data by showing the minimum, first quartile, median, third quartile, and maximum, along with potential outliers. - **Density Plots**: Estimate the probability density function of a continuous variable to show the distribution. - **Summary Statistics**: Provide a quantitative summary of the data using mean, median, mode, standard deviation, etc. **Understanding Distributions in Univariate Analysis** In univariate analysis, the distribution of a variable refers to how its values are spread or arranged. Understanding the distribution is crucial for summarizing the data and identifying patterns such as normality, skewness, or the presence of outliers. **Key Components of a Distribution** 1. **Central Tendency**: - **Mean**: The average value of the data. - **Median**: The middle value when the data is ordered. - **Mode**: The value that appears most frequently. 2. **Spread (Dispersion)**: - **Range**: The difference between the highest and lowest values. - **Variance**: The average of the squared deviations from the mean. - **Standard Deviation**: A measure of how spread out the data is from the mean. - **Interquartile Range (IQR)**: The range between the 25th and 75th percentiles, capturing the middle 50% of the data. 3. **Shape of the Distribution**: - **Symmetry**: A distribution is symmetric if the left and right sides are mirror images. - **Skewness**: Describes the direction and degree of asymmetry. - **Positive Skew**: Tail is on the right, meaning a few large values. - **Negative Skew**: Tail is on the left, meaning a few small values. - **Kurtosis**: Describes the \"tailedness\" of the distribution. - **Leptokurtic**: A distribution with heavy tails (more extreme outliers). - **Platykurtic**: A distribution with light tails (fewer extreme values). **Common Types of Distributions** 1. **Normal Distribution** (Bell Curve): - A symmetric distribution where most values cluster around the mean, with fewer values further away. - **Characteristics**: Mean = Median = Mode. - **Example**: Heights of individuals, standardized test scores. 2. **Skewed Distribution**: - **Positively Skewed**: The tail extends to the right, with a few large values pulling the mean up. - **Negatively Skewed**: The tail extends to the left, with a few small values pulling the mean down. - **Example**: Income distribution (typically positively skewed). 3. **Uniform Distribution**: - All values occur with roughly the same frequency, creating a flat distribution. - **Example**: Rolling a fair die. 4. **Bimodal Distribution**: - Contains two distinct peaks, often representing two subgroups in the data. - **Example**: Test scores with two groups of students: one group well-prepared, another less so. **Types of Variables in Univariate Analysis** Understanding the type of variable is critical because it dictates the type of analysis and visualization tools you can use. **1. Categorical Variables** - **Definition**: Variables that represent categories or labels, with no inherent numerical value. - **Subtypes**: - **Nominal**: Categories with no logical order (e.g., colors, gender, types of cars). - **Ordinal**: Categories with a meaningful order but without consistent differences between them (e.g., rankings like "low," "medium," "high"). - **Common Visualizations**: Bar charts, pie charts, frequency tables. - **Example**: Eye color (brown, blue, green). **2. Numerical Variables** - **Definition**: Variables that represent measurable quantities and can take numerical values. - **Subtypes**: - **Discrete**: Countable values, often integers, with distinct, separate points (e.g., number of students in a class). - **Continuous**: Infinite possible values within a range, often involving measurements (e.g., height, weight, temperature). - **Common Visualizations**: Histograms, box plots, density plots. - **Example**: Age, income, test scores. **Summary Table: Types of Variables** **Variable Type** **Subtype** **Definition** **Examples** ------------------- ---------------- ----------------------------------------- ------------------------------------------- **Categorical** **Nominal** Categories without a specific order Colors (red, green, blue) **Ordinal** Categories with a meaningful order Customer satisfaction (low, medium, high) **Numerical** **Discrete** Countable values, often integers Number of cars, number of students **Continuous** Infinite possible values within a range Weight, temperature **Analyzing Different Types of Variables** **1. Categorical Variables:** - **Frequency Distribution**: A simple count of how often each category appears. - **Visualization**: Bar charts or pie charts are typically used to represent the distribution of categorical data. - **Example**: If you have a dataset of students with their preferred programming languages, a bar chart can show how many students prefer Python, Java, or C++. **2. Numerical Variables:** - **Summary Statistics**: Mean, median, mode, standard deviation, variance, and range. - **Visualization**: Histograms, box plots, and density plots are useful for visualizing numerical data. - **Example**: If you have the heights of students, you could use a histogram to show the distribution and calculate summary statistics like the mean height and standard deviation. **Why Variable Types and Distributions is Important** - **Appropriate Analysis**: Each type of variable requires different statistical techniques and visualizations. For example, you cannot calculate a mean for a nominal variable like \"color.\" - **Data Insights**: Understanding the distribution helps to detect outliers, data quality issues, or underlying patterns that could be important for more advanced analysis. - **Model Selection**: Many statistical models require certain types of distributions, like the normal distribution, to perform optimally. 3. EXPLAIN THE 10 ESSENTIAL NUMERICAL SUMMARIES IN STATISTICS WITH EXAMPLE. When analyzing a single variable in univariate analysis, two key aspects to focus on are the **level** (central tendency) and **spread** (dispersion or variability). These measures help summarize the distribution of the variable and offer insights into its overall structure. **1. Measures of Level (Central Tendency)** The **level** of a distribution describes where the data tends to cluster, indicating typical values. Common measures include: **a. Mean** - The **mean** (or average) is the sum of all values divided by the number of observations. - **Use**: Best used for symmetric distributions without outliers. Outliers can distort the mean. **b. Median** - The **median** is the middle value when data is ordered from smallest to largest. - **Example**: For scores \[70, 75, 80\], the median is 75. For an even number of data points, the median is the average of the two middle values. - **Use**: The median is robust to outliers and skewed data, making it a better measure of central tendency in such cases. **c. Mode** - The **mode** is the most frequent value in a dataset. - **Example**: In the dataset \[60, 70, 70, 80\], the mode is 70. - **Use**: The mode is useful for categorical or discrete data, and for detecting the most common value in the dataset. **2. Measures of Spread (Dispersion)** The **spread** of a distribution shows how much the values of a variable differ from each other. Common measures of spread include: **a. Range** - The **range** is the difference between the maximum and minimum values. - **Formula**: Range=Xmax−Xmin - **Example**: For test scores \[60, 70, 75, 90\], the range is: 90−60=3090 - 60 = 3090−60=30 - **Use**: Provides a basic idea of the spread but is sensitive to outliers. **b. Variance** - The **variance** measures how much the data points deviate from the mean. - **Formula (population variance)**: σ2=∑i=1n(Xi−μ)2n\\sigma\^2 = \\frac{\\sum\_{i=1}\^{n} (X\_i - \\mu)\^2}{n}σ2=n∑i=1n​(Xi​−μ)2​ - **Formula (sample variance)**: s2=∑i=1n(Xi−Xˉ)2n−1s\^2 = \\frac{\\sum\_{i=1}\^{n} (X\_i - \\bar{X})\^2}{n-1}s2=n−1∑i=1n​(Xi​−Xˉ)2​ - **Example**: For test scores \[60, 70, 75, 90\], the variance can be calculated by first finding the mean (73.75), then calculating the squared deviations from the mean. - **Use**: Variance gives a sense of the overall spread, but because it uses squared units, it is less interpretable than standard deviation. **c. Standard Deviation** - The **standard deviation** is the square root of the variance, bringing it back to the original units of the data. **d. Interquartile Range (IQR)** - The **IQR** is the range between the 25th percentile (Q1) and the 75th percentile (Q3). It focuses on the middle 50% of the data and is robust to outliers. - **Use**: The IQR is particularly useful for skewed data and for identifying outliers. Data points outside 1.5 times the IQR are considered outliers. **e. Coefficient of Variation (CV)** - The **coefficient of variation** is the ratio of the standard deviation to the mean, often expressed as a percentage. It shows the relative variability of the data. - **Use**: CV is useful when comparing the spread of different datasets, especially those with different units or means. **Summary Table: Measures of Level and Spread** **Measure** **Type** **Purpose** **Example (Data: 60, 70, 75, 90)** ------------------------------- ------------------ --------------------------------------------- -------------------------------------- **Mean** Central Tendency Average value of the data Mean = 73.75 **Median** Central Tendency Middle value in ordered data Median = 72.5 **Mode** Central Tendency Most frequent value Mode = None (each value occurs once) **Range** Dispersion Difference between max and min Range = 90 - 60 = 30 **Variance** Dispersion Average of squared deviations from the mean Variance = 120.25 **Standard Deviation** Dispersion Spread in original units Standard deviation = 10.97 **IQR (Interquartile Range)** Dispersion Spread of middle 50% IQR = Q3 - Q1 = 85 - 65 = 20 **Coefficient of Variation** Dispersion Relative variability CV = (10.97 / 73.75) × 100 ≈ 14.87% **When to Use These Measures** - **Symmetric Data (Normal Distribution)**: Use **mean** and **standard deviation** to summarize the data, as they are more informative for normal data. - **Skewed Data**: Use **median** and **IQR**, as these are more robust to the influence of outliers. - **Presence of Outliers**: Avoid using **mean** and **range**, which can be highly affected by extreme values. Use **median**, **IQR**, and **standard deviation** instead. 4. Discuss various types of bivariate analysis.(diagram refer notes) 5. Discuss the common ways to perform bivariate analysis in detail. Bivariate analysis is a statistical method that explores the relationship between two variables. It helps us understand how changes in one variable may affect another. Here's a detailed look at the various types of bivariate analysis, explained in simple terms: **1. Scatter Plots** - Definition: A scatter plot is a type of graph that displays values for two variables as points in a Cartesian coordinate system. - Purpose: It visually shows the relationship between the two variables. By looking at the pattern of points, you can determine if there is a positive, negative, or no correlation. - Example: If you plot hours studied (x-axis) against test scores (y-axis), you can see if more studying correlates with higher scores. If the points trend upwards, it suggests that studying more is associated with better test performance. **2. Correlation Analysis** - Definition: Correlation analysis measures the strength and direction of the relationship between two variables. - Purpose: It quantifies how closely the two variables are related, producing a correlation coefficient (r) that ranges from -1 to 1. - Positive Correlation (0 to 1): As one variable increases, the other also increases. - Negative Correlation (-1 to 0): As one variable increases, the other decreases. - No Correlation (0): No relationship between the variables. - Example: A correlation coefficient of 0.8 between hours studied and test scores would indicate a strong positive relationship, suggesting that increased studying leads to higher scores. **3. Regression Analysis** - Definition: Regression analysis is a statistical method used to model the relationship between two variables by fitting a line (or curve) to the data points. - Purpose: It allows you to predict the value of one variable based on the value of another. It can also assess how much of the variation in one variable can be explained by changes in the other variable. - Example: Using regression analysis, you might find that for every additional hour studied, a student's test score increases by an average of 5 points. This provides a predictive equation for estimating scores based on study time. **4. Chi-Square Test** - Definition: The chi-square test is a statistical test used to determine if there is a significant association between two categorical variables. - Purpose: It tests whether the frequency distribution of sample data matches an expected distribution, helping to identify if the two variables are independent or related. - Example: If you want to see if there is a relationship between gender (male/female) and preference for a type of study method (group/individual), you can use a chi-square test to assess if preferences differ significantly between genders. **5. T-tests and ANOVA** - T-tests: - Definition: A t-test compares the means of two groups to see if they are significantly different from each other. - Purpose: It determines if the difference in means is due to random chance or if it reflects a true difference. - Example: You might compare the average test scores of students who study alone versus those who study in groups to see if there's a significant difference. - ANOVA (Analysis of Variance): - Definition: ANOVA is an extension of the t-test that compares the means of three or more groups. - Purpose: It assesses whether at least one group mean is different from the others. - Example: If you want to compare test scores among students using different study methods (group, individual, and online), ANOVA will help you determine if there's a significant difference in scores across these methods. Applications of Bivariate Analysis Bivariate analysis finds applications in various fields, including: - It helps researchers understand relationships between variables like income and education level, crime rates and unemployment, or happiness and marital status. - Bivariate analysis is used to study the relationship between factors like supply and demand, interest rates and inflation, or GDP and unemployment. - It helps in analyzing the correlation between factors such as diet and health outcomes, exercise and disease risk, or medication adherence and treatment effectiveness. - Bivariate analysis assists marketers in understanding relationships between variables like advertising expenditure and sales revenue, customer demographics and purchasing behavior, or product features and consumer satisfaction. - It helps in studying correlations between factors such as pollution levels and respiratory illnesses, climate variables and agricultural productivity, or habitat loss and species diversity. - Bivariate analysis is used to explore relationships between factors like study habits and academic performance, class size and student engagement, or teacher qualifications and student achievement. - It helps in analyzing relationships between variables like stock prices and company earnings, interest rates and bond yields, or asset allocation and investment returns. Bivariate analysis helps psychologists understand correlations between factors such as stress levels and mental health, personality traits and behavior patterns, or therapy outcomes and treatment adherence. +-----------------------------------------------------------------------+ | 1. Find the Z-Score Normalization Values for the following Data Set | | = {-5, 0, 23, 17.6, 9.2, 3.1, 11} (13) | +=======================================================================+ | i. Find the Decimal Scaling Normalization Values for the following | | Data Set = {-5, 0, 23, 17.6, 9.2, 3.1, 11} | | | | \(ii) Find the Min Max Normalized Values for the following Data S | | et | | = {1000, 2000, 3000, 5000, 8000, 9000} (check the pdf in group) | +-----------------------------------------------------------------------+ +-----------------------------------------------------------------------+ | 3. Discuss in detail about scatter plot and resistant lines in | | bivariate | | | | analysis. | +=======================================================================+ | 2. Analyze the role of resistant lines in scatterplots for | | understanding the relationship between two numerical variables. | | Use examples to illustrate how resistant lines can help identify | | trends and outliers. | +-----------------------------------------------------------------------+ **ANALYZING SCATTERPLOTS AND USING RESISTANT LINES** **Use scatter plots to visualize relationships between numerical variables.** **In Tableau, you create a scatter plot by placing at least one measure on the Columns shelf and at least one measure on the Rows shelf. If these shelves contain both dimensions and measures, Tableau places the measures as the innermost fields, which means that measures are always to the right of any dimensions that you have also placed on these shelves. The word \"innermost\" in this case refers to the table structure.** **Creates Simple Scatter Plot** **Creates Matrix of Scatter Plots** --------------------------------- -------------------------------------  **A scatter plot can use several mark types. By default, Tableau uses the shape mark type. Depending on your data, you might want to use another mark type, such as a circle or a square. For more information, see [Change the Type of Mark in the View](https://help.tableau.com/current/pro/desktop/en-us/viewparts_marks_marktypes.htm).** **To use scatter plots and trend lines to compare sales to profit, follow these steps:** 1. **Open the Sample - Superstore data source.** 1. **Drag the Profit measure to Columns.** **Tableau aggregates the measure as a sum and creates a horizontal axis.** 1. **Drag the Sales measure to Rows.** **Tableau aggregates the measure as a sum and creates a vertical axis.** **Measures can consist of continuous numerical data. When you plot one number against another, you are comparing two numbers; the resulting chart is analogous to a Cartesian chart, with x and y coordinates.** **Now you have a one-mark scatter plot:** 1. **Drag the Category dimension to Color on the Marks card.** **This separates the data into three marks---one for each dimension member---and encodes the marks using color.**  1. **Drag the Region dimension to Detail on the Marks card.** **Now there are many more marks in the view. The number of marks is equal to the number of distinct regions in the data source multiplied by the number of departments. (If you\'re curious, use the Undo button on the toolbar to see what would have happened if you\'d dropped the Region dimension on Shape instead of Detail.)** 1. **To add trend lines, from the Analytics pane, drag the Trend Line model to the view, and then drop it on the model type.**  **A trend line can provide a statistical definition of the relationship between two numerical values. To add trend lines to a view, both axes must contain a field that can be interpreted as a number---by definition, that is always the case with a scatter plot.** **Tableau adds three linear trend lines---one for each color that you are using to distinguish the three categories.** 1. **Hover the cursor over the trend lines to see statistical information about the model that was used to create the line:**  **For more information, see [Assess Trend Line Significance](https://help.tableau.com/current/pro/desktop/en-us/trendlines_add.htm#significance). You can also customize the trend line to use a different model type or to include confidence bands. For more information, see [Add Trend Lines to a Visualization](https://help.tableau.com/current/pro/desktop/en-us/trendlines_add.htm).** **Check your work! See steps 1-7 below:** +-----------------------------------------------------------------------+ | 2. Explain the use of contingency tables in bivariate analysis with | | | | example. | +=======================================================================+ | 1. Explain in detail about relationship between two variables in the | | | | percentage tables. | +-----------------------------------------------------------------------+ **CREATING AND INTERPRETING PERCENTAGE TABLES AND CONTINGENCY TABLES** **Creating and interpreting percentage tables and contingency tables in Tableau is a powerful way to conduct bivariate analysis. Bivariate analysis involves examining the relationship between two variables, often to understand the association or correlation between them. Here's a guide on how to create and interpret these tables using Tableau.** **There are two factors that contribute to the percentage calculation:** **1. The data to which you compare all percentage calculations** **Percentages are a ratio of numbers. The numerator is the value of a given mark. The denominator depends on the type of percentage you want, and is the number to which you compare all your calculations. The comparison can be based on the entire table, a row, a pane, and so on. By default, Tableau uses the entire table. Other percentage calculations are available via the Percentage of menu item. See [Percentage options](https://help.tableau.com/current/pro/desktop/en-us/calculations_percentages_options.htm#Options).** **The figure below is an example of a text table with percentages. The percentages are calculated with the Sales measure aggregated as a summation, and are based on the entire table.**  **2. The aggregation** **Percentages are computed on the basis of the aggregation for each measure. Standard aggregations include summation, average, and several others. See [Data Aggregation in Tableau](https://help.tableau.com/current/pro/desktop/en-us/calculations_aggregation.htm) for more information.** **For example, if the aggregation applied to the Sales measure is a summation, then the default percentage calculation (percent of table) means that each number displayed is the SUM(Sales) for that mark divided by the SUM(Sales) for the entire table.** **In addition to using predefined aggregations, you can use custom aggregations when calculating percentages. You define your own aggregations by creating a calculated field. Once the new field is created, you can use percentages on the field as you would any other field. See [Aggregate Functions in Tableau(Link opens in a new window)](https://help.tableau.com/current/pro/desktop/en-us/calculations_calculatedfields_aggregate_create.htm) for more information.** **Percent calculations can also be applied to disaggregated data. In this case, all values are expressed as the percentage of a summation. You cannot choose any other aggregation.** **Example** **The view below shows a nested bar chart created using two dimensions and a measure that is aggregated as a maximum. Additionally, the data are color-encoded by a dimension and the default percentage calculation has been applied. Notice that the axis labels are modified to reflect the percent calculation.** **The tooltip reveals that the maximum sales for furniture in the east in 2011 is 17.70% of the maximum for the entire table. What is the maximum for the table? If you recreate the view you\'ll see that the maximum occurs in the South, in the Technology category, in the year 2011. The tooltip for this bar segment would reveal a maximum sales of 100%.** A graphic depicting a bar chart with percentages turned on. The tooltips display percentage information too. **The next view displays two disaggregated measures as a scatter plot. Again, the default percentage calculation has been applied as reflected by the modified axis labels.** **The tooltip shows that the selected data point constitutes -0.475 percent of total profit and a 0.3552 percent of total sales. Percentage calculations are based on the entire data source.**  **How to calculate percentages** **To calculate percentages in your visualization:** - **Select Analysis \> Percentages Of, and then select a percentage option.** **Percentage options** **Computing a percentage involves specifying a total on which the percentage is based. The default percentage calculation is based on the entire table. You can also choose a different option.** **The option you choose is applied uniformly to all measures that appear on a worksheet. You cannot choose Percent of Column for one measure and Percent of Row for another.** **The percentage options on the Analysis menu correspond to the percentage table calculations. When you select a percentage option, you are actually adding a Percent of Total table calculation. See [Transform Values with Table Calculations](https://help.tableau.com/current/pro/desktop/en-us/calculations_tablecalculations.htm) for more information.** **If you are unsure what the current percentage calculation means, display the grand totals. This provides more information about each row and column. For example, if you select Percent of Row while displaying grand totals, you will see that the total for each row is exactly 100%. See [Show Totals in a Visualization](https://help.tableau.com/current/pro/desktop/en-us/calculations_totals_grandtotal_turnon.htm) for more information on grand totals.** **The percent calculation options are described in the following sections. In each case, the grand totals are displayed as well.** **Percent of Table** **When you select Percentage Of \> Table from the Analysis menu, each measure on the worksheet is expressed as a percentage of the total for the entire worksheet (table). For example, Technology in the East region accounts for 3.79% of total sales in 2014. The grand totals for rows show that 2014 accounts for 31.95% of the total sales. Summing the grand totals for rows or for columns yields 100% of the total.** A graphic depicting a text table with the percentage of the table turned on. **Percent of Column** **When you select Percentage of \> Column from the Analysis menu, each measure on the worksheet is expressed as a percentage of the total for the column. The values within the red box add up to 100%.**  **Percent of Row** **When you select Percentage of Row, each measure on the worksheet is expressed as a percentage of the total for the row. The values within the red box add up to 100%.** A graphic depicting a text table with the percentages of rows turned on. **Percent of Pane** **When you select Percentage of \> Pane from the Analysis menu, each measure on the worksheet is expressed as a percentage of the total for the panes in the view. This option is equivalent to Percent of Table when the table consists of only a single pane.** **In the following view, the red box constitutes a single pane; the values within the red box add up to 100%.**  **Percent of Row in Pane** **When you select Percentage of \> Row in Pane from the Analysis menu, each measure on the worksheet is expressed as a percentage of the total for a row within a pane. This option is equivalent to as Percent of Row when the table is only a single pane wide.** **In the following view, the red box constitutes a row within a pane; the values within the red box add up to 100%.** A graphic depicting a text table with the East row highlighted in the Technology pane. Grand totals are turned on to show the percentages of a single row within a pane. **Note: If you place Measure Names as the inner dimension on the Columns shelf (that is, the dimension farthest to the right), Tableau will return 100% for each mark because you cannot total up the values for multiple measure names. For example, you can't total up the values for SUM(Sales) and SUM(Profit).** **Percent of Column in Pane** **When you select Percentage of \> Column in Pane from the Analysis menu, each measure in the worksheet is expressed as a percentage of the total for a column within a pane. This option is equivalent to as Percent of Column when the table is only a single pane high.** **In the following view, the red box constitutes a column within a pane; the values within the red box add up to 100%.**  **If you place Measure Names as the inner dimension on the Rows shelf (that is, the dimension farthest to the right on the shelf), Tableau will return 100% for each mark because you cannot total up the values for multiple measure names. For example, you can't total up the values for SUM(Sales) and SUM(Profit).** **Percent of Cell** **When you select Percentage Of \> Cell from the Analysis menu, each measure on the worksheet is expressed as a percentage of the total for each individual cell in the view. Most views show only one value per cell, in which case all cells show a percentage of 100%. But in some cases, as, for example, when you disaggregate data, a single cell can contain multiple values:** 1. Compare and Contrast Bar chart and Bullet graph. Explain the need of both these charts with diagrams **Bullet Graph:** A bar marked against a background to show progress or performance against a goal, denoted by a line on the graph. - A bullet graph is a bar marked with extra encodings to show progress towards a goal or performance against a reference line. - Each bar focuses the user on one measure, bringing in more visual elements to provide additional detail. - The bullet graph, designed by Stephen Few, replaces meters and gauges that dominated early dashboards and reports. - It provides more information in a smaller space; making it ideal for a compact dashboard. **Bar Chart:** Bar charts represent numerical values compared to each other. The length of the bar represents the value of each variable. - Bar charts enable us to compare numerical values like integers and percentages. They use the length of each bar to represent the value of each variable. - For example, bar charts show variations in categories or subcategories scaling width or height across simple, spaced bars, or rectangles. - Bar charts can represent quantitative measures vertically, on the y-axis, or horizontally, on the x-axis. The style depends on the data and on the questions the visualization addresses. - The qualitative dimension will go along the opposite axis of the quantitative measure. - Bar charts typically have a baseline of zero. If another starting point is used, the axis should be clearly labelled to avoid misleading the Viewer. - Many other variations of bar charts exist. Stacked bar charts, side-by-side bar charts, clustered bar charts, and diverging bar charts are representative examples. - Labels and legends help the viewer determine the details included in these charts. - Bar charts are versatile and can answer many questions in visual analysis. They can highlight the largest or smallest number in a set of data or to show relationships between values. **A good bar chart will follow these rules:** - The base starts at zero - The axes are labelled clearly - Colours are consistent and defined - The bar chart does not display too many bars  **Comparison:** **Feature** **Bar Chart** **Bullet Graph** ---------------------------- ------------------------------------------------- ------------------------------------------------ **Purpose** **Compare multiple categories** **Show performance against a target** **Data Representation** **Single measure per bar** **Multi-dimensional (actual, target, ranges)** **Space Efficiency** **Requires more space for multiple categories** **More compact representation** **Ease of Interpretation** **Simple to read** **May require familiarity** **Context** **No inherent context** **Provides context for performance** **Need for Both Charts:** 1. **Bar Chart:** - **Simplicity: Ideal for presenting clear and straightforward comparisons across categories.** - **Versatility: Useful for various types of data visualization where multiple items need comparison.** 2. **Bullet Graph:** - **Compactness: Saves space while conveying more information, making it suitable for dashboards.** - **Performance Context: Enables quick assessment of how well a measure is doing against a target or standard, which is crucial for decision-making.** 2. WHAT IS A TIME SERIES? EXPLAIN TYPES, PROPERTIES, AND DECOMPOSITION OF TIME SERIES. **A time series is a sequence of data points collected or recorded at successive time intervals. Time series data is used to analyze trends, patterns, and seasonal variations over time.** **Definition of Time Series** - **Time Series: A time series is a collection of observations recorded at regular intervals over time, often used to identify trends, cycles, and seasonal patterns.** **Types of Time Series** 1. **Univariate Time Series:** - **Definition: Involves a single variable measured over time.** - **Example: Monthly sales figures for a retail store.** 2. **Multivariate Time Series:** - **Definition: Involves multiple variables measured over time, allowing analysis of relationships between them.** - **Example: Monthly sales figures, advertising spend, and economic indicators.** 3. **Seasonal Time Series:** - **Definition: Exhibits regular patterns or fluctuations at specific intervals due to seasonal factors.** - **Example: Ice cream sales that peak during summer months.** 4. **Non-seasonal Time Series:** - **Definition: Lacks regular seasonal patterns, often showing trends and irregular variations.** - **Example: Yearly unemployment rates that do not follow a predictable seasonal pattern.** **Properties of Time Series** 1. **Trend:** - **Definition: The long-term movement or direction in the data over time, indicating an increase, decrease, or stability.** - **Example: An increasing trend in annual global temperatures over several decades.** 2. **Seasonality:** - **Definition: Regular fluctuations that occur at specific intervals, such as daily, monthly, or yearly.** - **Example: Higher retail sales during the holiday season each year.** 3. **Cyclic Patterns:** - **Definition: Long-term fluctuations that are not of fixed periodicity, often related to economic or business cycles.** - **Example: Economic growth and recession cycles occurring over several years.** 4. **Irregular Variations:** - **Definition: Unpredictable variations that cannot be attributed to trend, seasonality, or cyclic patterns.** - **Example: Sudden spikes in sales due to an unexpected event, like a natural disaster.** **Decomposition of Time Series** **Time series decomposition involves breaking down a time series into its individual components to better analyze and understand the underlying patterns. The main components are:** 1. **Trend Component (T):** - **Definition: Represents the long-term movement in the data, showing the overall direction over time.** - **Example: A steady increase in company profits over several years.** 2. **Seasonal Component (S):** - **Definition: Represents the repeating fluctuations due to seasonality, reflecting patterns at regular intervals.** - **Example: Increased electricity usage during summer months due to air conditioning.** 3. **Cyclic Component (C):** - **Definition: Reflects long-term cyclical fluctuations that are related to economic or business cycles, which may not have a fixed period.** - **Example: Economic expansions and contractions that affect consumer spending.** 4. **Irregular Component (I):** - **Definition: Consists of random, unpredictable variations in the data that cannot be attributed to trend, seasonality, or cycles.** - **Example: An unexpected event, such as a global pandemic, causing sudden changes in sales patterns.** **Example of Decomposition** **Suppose you have monthly sales data for a retail store. By decomposing this data, you may find:** - **Trend: Sales have generally increased over the last three years.** - **Seasonality: Sales peak in December due to holiday shopping.** - **Cyclic Patterns: A noticeable decline in sales during economic recessions.** - **Irregular Variations: A significant drop in sales in March due to a sudden supply chain disruption.** **Top of Form** **APPLY TIME SERIES ANALYSIS TECHNIQUE TO DEMONSTRATE THEIR USEFULNESS IN DATA ANALYSIS. PROVIDE EXAMPLES FROM VARIOUS DOMAINS SUCH AS FINANCE, WEATHER FORECASTING, OR SALES FORECASTING** **Time Series Forecasting** Definition: Time series forecasting is the process of using historical data to predict future values based on identified patterns, trends, and seasonal variations. It employs various statistical methods and machine learning algorithms to analyze the time-dependent data and generate forecasts. Applications of Time Series Forecasting Time series forecasting is widely used across different fields and industries. Here are some common applications: 1. Finance and Economics: - Stock Price Prediction: Analysts forecast stock prices using historical price data and trading volumes. - Economic Indicators: Forecasting GDP, inflation rates, and unemployment figures helps policymakers make informed decisions. 2. Retail and Sales: - Sales Forecasting: Retailers use historical sales data to predict future sales trends, helping with inventory management and supply chain optimization. - Demand Planning: Businesses forecast product demand to ensure optimal stock levels during peak seasons. 3. Supply Chain Management: - Inventory Management: Companies forecast future inventory needs to avoid overstocking or stockouts. - Logistics Planning: Predicting delivery times and optimizing routes based on historical shipping data. 4. Weather Forecasting: - Meteorologists use time series data from weather stations to predict future weather conditions, such as temperature and precipitation. 5. Healthcare: - Patient Admissions: Hospitals forecast patient admissions to manage resources and staffing levels effectively. - Epidemiology: Forecasting the spread of diseases helps in planning public health interventions. 6. Energy Consumption: - Utility companies forecast energy demand to ensure that supply meets consumption needs during peak hours or seasons. Examples of Time Series Forecasting 1. Monthly Sales Forecasting: - A retail store analyzes its sales data for the past three years to identify trends and seasonal patterns. Using methods like ARIMA (AutoRegressive Integrated Moving Average) or exponential smoothing, the store can predict future sales for the upcoming months. 2. Stock Price Prediction: - A financial analyst uses historical stock prices and volumes to create a forecasting model. By applying machine learning techniques such as recurrent neural networks (RNNs) or LSTM (Long Short-Term Memory) networks, the analyst predicts future stock prices. 3. Weather Prediction: - Meteorological agencies collect temperature and precipitation data over several years. They use time series forecasting models to predict future weather patterns, aiding in agriculture planning and disaster preparedness. 4. Energy Demand Forecasting: - An electricity provider analyzes hourly energy consumption data to forecast future demand. This helps in resource allocation and ensures that the supply of electricity meets consumer demand, especially during peak usage times. 5. Economic Forecasting: - Economists analyze historical data on inflation, employment, and GDP growth rates to predict future economic conditions. This information assists policymakers in making decisions related to fiscal and monetary policies. **When to Use Time Series Forecasting** 1. **Clear Business Question:** - **Definition: Before starting, you need to know what specific question you want to answer.** - **Example: If you want to predict next quarter's sales based on past sales data, this is a clear question that can be addressed with time series forecasting.** 2. **Appropriate Data Availability:** - **Definition: You need to have sufficient historical data collected over regular time intervals (e.g., daily, monthly, yearly).** - **Example: A retail store might have three years of monthly sales data, which is enough to identify trends and patterns.** 3. **Clean, Time-Stamped Data:** - **Definition: The data should be well-organized and free of errors, with clear time stamps to indicate when each observation was recorded.** - **Example: Sales data that has missing values or inconsistent recording times can lead to inaccurate forecasts.** 4. **Identification of Trends and Patterns:** - **Definition: The ability to identify genuine trends and patterns in historical data is essential for effective forecasting.** - **Example: If the data shows a consistent increase in sales every holiday season, this pattern can be used for future predictions.** 5. **Separation of Random Fluctuations:** - **Definition: Analysts must distinguish between normal variations and genuine insights in the data.** - **Example: If there is a sudden spike in sales due to a one-time event, it should not be confused with a long-term trend.** 6. **Understanding Seasonal Variations:** - **Definition: Recognizing seasonal factors that affect the data helps improve forecasting accuracy.** - **Example: A toy store might see increased sales every December, which should be considered in predictions for that period.** 7. **Modeling Capabilities:** - **Definition: You need to have the appropriate forecasting models and tools available to analyze the data.** - **Example: Utilizing methods like ARIMA or exponential smoothing requires familiarity with statistical software and modeling techniques.** **Limitations of Time Series Forecasting** 1. **Unpredictable Events:** - **Definition: Time series forecasting may not effectively predict sudden, unexpected events (e.g., natural disasters, economic crises).** - **Impact: These unpredictable events can significantly skew results and make forecasts less reliable.** 2. **Not Suitable for All Data Types:** - **Definition: Time series forecasting is most effective for data that shows temporal patterns. Data without time-related trends may not be suitable.** - **Example: A one-time survey result or data without a clear time component may not benefit from time series analysis.** 3. **Overfitting:** - **Definition: Using overly complex models may fit the historical data perfectly but perform poorly on new data.** - **Impact: This can lead to misleading forecasts if the model captures noise rather than the actual trend.** 4. **Dependence on Historical Data:** - **Definition: Time series forecasting relies heavily on past data. If past trends change dramatically, forecasts may become inaccurate.** - **Example: A sudden market shift (like a new competitor) can render previous data less relevant for future predictions.** **Bottom of Form** 3.