Exploratory Data Analysis (EDA) in Finance PDF
Document Details
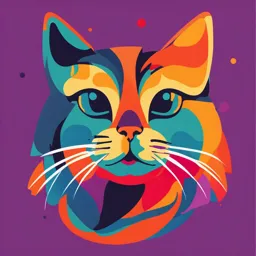
Uploaded by HardWorkingPalladium
Tags
Summary
This presentation provides an overview of exploratory data analysis (EDA) techniques in finance. It covers visualizing data through graphs and charts, using descriptive statistics like mean, median, standard deviation, and correlation, and handling missing data and outliers. The presentation concludes by emphasizing the importance of EDA in understanding complex relationships between variables within time series data.
Full Transcript
Exploratory Data Analysis (EDA) in Finance Agenda Introduction What EDA involves in finance Exploratory Data Analysis (EDA) Exploratory Data Analysis (EDA) is like getting to know your data before you do any complex calculations or build models....
Exploratory Data Analysis (EDA) in Finance Agenda Introduction What EDA involves in finance Exploratory Data Analysis (EDA) Exploratory Data Analysis (EDA) is like getting to know your data before you do any complex calculations or build models. In finance, EDA helps you understand the patterns, trends, and key characteristics of financial data, such as stock prices, interest rates, or company revenues. It’s the first step in analyzing data to find insights, and it helps you make decisions about how to proceed with further analysis. What EDA involves in finance 1. Visualizing the Data Graphs and charts: You create simple visual representations like line charts, histograms, or scatter plots to see how things change over time. For example, you could plot stock prices over the last 10 years to identify patterns like uptrends, downtrends, or seasons of volatility. Boxplots and histograms: These help you see how data is spread out. For instance, you might check how often stock prices are high or low, and whether there are any unusual jumps or outliers. These are basic calculations that help you understand the main features of the data, like: Mean: The average value, such as the average price of a stock over time. Median: The middle value, which is helpful when data has outliers (extremely high or low values). Standard Deviation: This tells you how spread out the data is. In finance, it can help measure risk or volatility. Correlation: This shows how different financial variables move together. For example, you might see if two stocks tend to rise or fall at the same time. 2. Descriptive Statistics Mean Sometimes, you might have missing information in your dataset. EDA helps you identify these gaps and decide whether to remove those data points, replace them, or fill them in with an 3. Handling estimate. Missing Data An outlier is a value that is significantly different from the rest. For instance, if a stock price suddenly jumps by 50% in one day, it might be an outlier. EDA helps you spot these, which can affect your analysis or predictions. 4. Identifying Outliers In finance, different factors like interest rates, inflation, or company earnings can affect stock prices. EDA lets you see how these different variables relate to each other, for 5. example by creating scatter plots that show how two variables move in Understanding relation to each other. Relationships Between Variables Since financial data often involves time (like daily stock prices or monthly sales), EDA includes looking at trends over time. T his helps you see long-term growth or patterns that repeat (like seasonality). 6. Time Series Analysis In short, EDA is like a detective’s toolkit. It helps you explore, understand, and clean your data so that you can start making meaningful financial decisions or predictions. Thank you