Diagnosis of Genetic Diseases PDF
Document Details
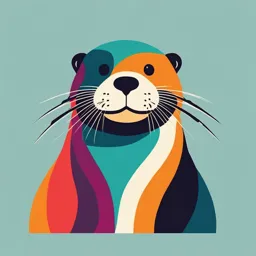
Uploaded by FirmerJaguar4741
Tags
Summary
This document discusses the diagnosis of genetic diseases, covering topics from the fundamentals of genetic testing to the ethical considerations involved. It also highlights different methods and the importance of genetic counseling within the process. Key concepts such as Mendelian disorders and susceptibility genes are also explored.
Full Transcript
Diagnosis of Genetic Diseases Professor Chiara Di Resta [email protected] Exam structure: oral exam Lesson 1 – October 10th The main topic of the course is to delve into the fascinating and complex realm of genetic testing, a pivotal tool in modern medi...
Diagnosis of Genetic Diseases Professor Chiara Di Resta [email protected] Exam structure: oral exam Lesson 1 – October 10th The main topic of the course is to delve into the fascinating and complex realm of genetic testing, a pivotal tool in modern medicine. Genetic testing serves multiple purposes: diagnosing affected patients, stratifying risk for individuals carrying genetic mutations, and even enabling preventive care. These applications span a wide range of conditions, from inherited disorders to oncological susceptibilities, and provide invaluable insights for clinical management, prognosis, and treatment planning. However, genetic testing also presents unique challenges, requiring careful ethical consideration, psychological sensitivity, and legal oversight. The central focus of this course is understanding the characterization of genetic testing, both for diagnosing individuals with pathological conditions and for identifying individuals with familial genetic mutations that increase susceptibility to certain diseases. The goal is to learn how to apply clinical genetic tests effectively while addressing the various challenges that arise during the process. These challenges include ensuring the clinical utility of tests, avoiding unnecessary complications, and considering the ethical and psychological impacts on patients and their families. Genetic testing allows us to address numerous critical objectives. It aids in prevention by identifying individuals at risk, enabling early interventions or lifestyle modifications to reduce the likelihood of disease onset. Testing plays a fundamental role in diagnosis, confirming the presence of mutations responsible for a clinical phenotype and supporting clinical suspicions. It also enhances our understanding of the molecular bases of various disorders, contributing to new research and therapeutic development. Prognosis is another key aspect; specific mutations can predict the severity or progression of a disease, helping clinicians make more informed decisions. Additionally, genetic testing supports risk stratification, identifying individuals who may be at a higher risk of developing certain conditions, thereby enabling targeted monitoring and prevention. Lastly, genetic screening, applied to healthy populations, allows for the early identification of mutations that could affect future generations or lead to disease later in life. A distinction must be made between diagnostic and screening testing. Diagnostic genetic testing is performed on individuals already exhibiting clear pathological signs, with the aim of confirming or clarifying a clinical diagnosis. This approach ensures precise treatment and management tailored to the patient’s condition. In contrast, screening genetic testing is conducted on healthy individuals, often to assess their risk of developing certain conditions or carrying mutations that could be passed on to their offspring. While diagnostic testing focuses on confirming an observed clinical phenotype, screening identifies Bassal 1 potential future risks. This difference underscores the importance of tailoring genetic testing strategies to the specific needs of the target population. Unlike other diagnostic tests, genetic testing carries profound psychological, ethical, and legal implications. Before conducting genetic tests, patients must undergo genetic counseling to fully understand the scope and consequences of the process. Signing an informed consent form is mandatory, emphasizing the gravity of this deeply personal form of testing. Genetic results often reveal information not only about the individual but also about their family, potentially affecting relatives who may be unaware of their risk. The psychological impact of learning about a predisposition to future illness can be immense, especially for young individuals facing a lifetime of uncertainty. The DNA itself is the most private data we possess, containing details about our health, ancestry, and even unexpected revelations, such as unknown paternity. This raises profound ethical and legal questions. For instance, should incidental findings—mutations linked to conditions unrelated to the original purpose of testing—be disclosed to the patient? While such information can be life-saving, it can also lead to distress or unintended consequences. The ethical landscape becomes even more complex when genetic testing intersects with societal structures, such as insurance. In countries like the United States, insurers may adjust premiums based on genetic predispositions, even for individuals who are currently healthy. This highlights the tension between the potential benefits of genetic information and the risks of discrimination or privacy breaches. In contrast, European systems prioritize privacy, discouraging such practices and emphasizing the need for strict regulatory oversight. In some cases, genetic testing serves a predictive purpose, enabling preventive measures for individuals at high risk of developing certain conditions. For example, identifying mutations in BRCA1 or BRCA2 can inform strategies to reduce the likelihood of breast or ovarian cancer through monitoring, lifestyle changes, or even prophylactic surgeries. This type of testing, known as predictive or preventive genetic testing, exemplifies how genetic insights can transform healthcare by moving from reactive to proactive care. Molecular diagnostics is the cornerstone of genetic testing, involving the analysis of DNA or RNA to identify pathogenic mutations. This can be applied to diagnosis, classification, prognosis, and treatment. Diagnostically, genetic testing identifies causative mutations underlying clinical symptoms, confirming or refuting initial suspicions. Classification allows for a more refined understanding of disorders, particularly in cases where multiple genetic variants result in similar phenotypes. For example, in Charcot-Marie-Tooth neuropathy, genetic testing helps classify the disorder into its subtypes, each associated with a specific gene. This classification can directly influence treatment strategies. Prognostically, certain mutations predict disease severity or progression, guiding long-term management. In oncology, genetic findings can influence treatment choices, such as selecting targeted therapies for patients with BRCA1 mutations. Bassal 2 The development of molecular techniques has revolutionized genetic diagnostics. PCR (polymerase chain reaction) allows for the amplification of DNA, enabling detailed analysis even from small samples. Sanger sequencing, developed in 1977, remains the gold standard for genetic testing due to its accuracy, despite the advent of next-generation sequencing (NGS). NGS has transformed the field by enabling high- throughput, large-scale analysis of the genome, but results often require confirmation through Sanger sequencing to ensure reliability. Genetic testing is tailored to the type of disorder being investigated. Mendelian disorders, characterized by a one-to-one relationship between a gene and its phenotype, such as cystic fibrosis or Duchenne muscular dystrophy, are well-suited for genetic testing. In these cases, the presence of a mutation directly correlates with the disease. Susceptibility genes, such as those associated with breast and ovarian cancer, present a different challenge. These genes increase the risk of developing a disease but do not guarantee it. Diagnostic strategies for susceptibility genes must account for additional factors, including environmental influences and lifestyle. Genetic testing can be performed at various stages of life. Prenatal testing includes methods such as preimplantation genetic diagnosis during in vitro fertilization, chorionic villus sampling (CVS), amniocentesis, and non-invasive cell-free fetal DNA testing from maternal blood. Postnatal testing spans from newborn screening to adolescent and adult evaluations, addressing clinical suspicions or assessing carrier status. Each approach is carefully tailored to the patient’s life stage and clinical context. The molecular diagnostic workflow is a multidisciplinary process that begins and ends with the patient. It starts with a thorough clinical characterization, ensuring that the genetic test is appropriate and targeted. After obtaining informed consent, biological samples—usually peripheral blood or saliva—are collected and analyzed in the laboratory. Technological advances, particularly in bioinformatics, are critical for managing the vast data generated by modern sequencing techniques. The results are then interpreted by medical geneticists and clinicians, who communicate their findings to the patient in a clear and compassionate manner. Genetic counseling is integral to this process. It involves analyzing the patient’s family history and constructing a pedigree to identify patterns of inheritance and assess familial risk. This step ensures that genetic testing is both relevant and informative, guiding clinical decisions and family planning. The counseling session also addresses the ethical and psychological implications of the test results, helping patients and their families navigate the complexities of genetic information. Biological samples for genetic testing can be obtained from various sources, with peripheral blood and saliva being the most common. Proper collection and handling are essential; for instance, blood samples must be collected in EDTA tubes to preserve DNA integrity. In prenatal testing, samples such as amniotic fluid or chorionic villi may be used. Bassal 3 In conclusion, genetic testing is a transformative tool in modern medicine, offering unparalleled insights into disease diagnosis, prevention, and treatment. However, its application requires a multidisciplinary approach, ethical sensitivity, and a clear understanding of its implications. By addressing these challenges, we can harness the full potential of genetic testing to improve patient outcomes and advance healthcare. Lesson 2 – October 17th Utility of Genetic Testing Genetic testing serves multiple purposes, ranging from confirming clinical suspicions to evaluating reproductive risks and assessing the likelihood of developing specific diseases. The application of genetic testing depends on the nature of the disorder and the clinical context. In Mendelian disorders, genetic testing is essential for confirming clinical diagnoses. These disorders exhibit a clear and direct relationship between genotype and phenotype, where a specific genetic mutation causes the pathological condition. Genetic testing helps validate clinical evaluations and identify specific mutations, particularly in conditions with significant clinical heterogeneity. For example, neuropathies often present with diverse clinical manifestations, and genetic testing enables precise classification and diagnosis. For susceptibility genes, genetic testing identifies genetic variations that increase the risk of developing certain diseases, such as cancer. Unlike Mendelian disorders, where a mutation guarantees the development of the disease, susceptibility genes only elevate the likelihood of disease occurrence. Testing in these cases is valuable for confirming the molecular basis of a pathological phenotype and for assessing risks in family members who may share the genetic predisposition. In syndromic and severe conditions involving large-scale chromosomal alterations, cytogenetic approaches are often employed. These conditions are characterized by chromosomal aberrations, such as copy number variations (CNVs), translocations, duplications, and deletions, which can affect multiple organs. Trisomy 21 (Down syndrome) is a well-known example, where cytogenetic testing, particularly karyotyping, is used to identify the chromosomal abnormality. Such approaches are critical in diagnosing syndromic conditions. Carrier testing is performed on healthy individuals to determine whether they carry genetic alterations that could be passed to their offspring. This type of testing is especially important for calculating reproductive risks and guiding family planning decisions. Genetic testing can be broadly categorized into prenatal and postnatal applications. Prenatal testing, conducted during pregnancy, includes invasive techniques such as amniocentesis and chorionic villus sampling, which are considered gold standards. These techniques are used when routine imaging, such as ultrasound, raises suspicions of genetic abnormalities. However, invasive procedures carry a risk of miscarriage. To reduce this risk, non-invasive methods like cell-free fetal DNA testing can be used. While safer, these methods serve primarily as screening tools and require confirmation through invasive techniques if abnormalities are detected. Postnatal testing is performed after birth and is used to confirm clinical diagnoses or investigate suspected genetic abnormalities in newborns, adolescents, or adults. Bassal 4 Genetic counseling is an integral part of the testing process. Patients are informed about the purpose, risks, and expected outcomes of the test, and they must provide informed consent before testing begins. This step is crucial for legal and ethical reasons, as DNA analysis involves sensitive and private information. Sample Collection and Processing Biological samples, such as peripheral blood or saliva, are the primary sources of genetic material for testing. Peripheral blood is commonly collected in tubes containing anticoagulants, specifically those with purple caps, to prevent coagulation and ensure sample integrity. Only a small amount of DNA—about 25 nanograms—is sufficient for most high-throughput sequencing techniques. When samples, clinical documentation, and informed consent are received, the first step in the lab is anonymization. Samples are assigned unique identification codes to ensure that personal information is not directly associated with the biological material during analysis. DNA extraction is performed in the pre-PCR area of the lab, a space specifically designed to minimize contamination risks. This is critical because amplified DNA fragments, known as amplicons, are highly contaminating and can compromise results if proper separation protocols are not followed. Organization of Molecular Diagnostic Labs Molecular diagnostic labs are specialized facilities that differ significantly from traditional diagnostic or biochemical labs. These labs require advanced instruments, highly trained personnel, and specific workflows to operate effectively. Due to the high costs of maintaining such facilities, molecular diagnostic labs are often centralized. This means that a single lab serves multiple institutions, reducing redundancy and improving efficiency. Automation is a key feature of molecular diagnostic labs, allowing them to process large volumes of samples efficiently. Automated workstations handle tasks such as DNA extraction and reagent mixing, reducing manual intervention and the risk of human error. In some labs, up to 10,000 samples are processed annually, highlighting the importance of automation in maintaining accuracy and throughput. Despite these advancements, storage remains a significant challenge. DNA samples must be preserved for years, and sequencing data—often amounting to terabytes per patient—requires secure storage. Due to privacy concerns, cloud storage is not used, and physical data clusters are employed instead. These clusters must be expanded continually as data accumulates, adding logistical and financial burdens. Bioinformaticians play a critical role in these labs. They design and manage pipelines to analyze the massive datasets generated during sequencing, ensuring accurate interpretation of results. This role is unique to genomic labs, as the data analysis requirements far exceed those of other diagnostic fields. The lab is divided into distinct areas to prevent contamination: The pre-PCR area handles DNA extraction and reagent preparation. The post-PCR area is dedicated to amplified DNA processing. Bassal 5 Maintaining this separation is crucial to prevent cross-contamination between amplified DNA and other samples, which could lead to erroneous results. Sequencing Approaches in Genetic Testing Genetic sequencing technologies have evolved over four generations, each offering unique features and applications. The first generation, known as Sanger sequencing or direct sequencing, was developed by Frederick Sanger and remains the gold standard due to its high accuracy (approximately 99%). This method involves amplifying DNA using PCR and incorporating modified nucleotides (ddNTPs) that terminate DNA elongation. These nucleotides are labeled with fluorescent dyes, and DNA fragments are separated by size using electrophoresis. A laser excites the dyes, producing chromatograms with colored peaks representing nucleotide sequences. Despite its accuracy and ability to produce long reads (up to 1,000 base pairs), Sanger sequencing is time-consuming and expensive. For instance, analyzing a single gene can take months and cost over €1,000. The second generation, or next-generation sequencing (NGS), introduced massive parallel sequencing, enabling the simultaneous analysis of multiple genes or samples. NGS uses library preparation to enrich target regions instead of traditional PCR. This approach reduces time and cost significantly while increasing efficiency. However, its accuracy depends on factors such as coverage, which refers to the number of times a region is sequenced. The analysis of NGS data requires advanced bioinformatics pipelines to manage and interpret the large datasets generated. The third generation, characterized by single-molecule sequencing, eliminates the need for DNA amplification. By analyzing individual DNA molecules directly, it reduces errors introduced during amplification and provides greater accuracy. However, third-generation sequencing is still under development and has not yet achieved widespread clinical adoption. The fourth generation, or single-cell sequencing, isolates and sequences DNA or RNA from individual cells. Unlike earlier methods that analyze bulk DNA, single-cell sequencing provides unparalleled resolution, making it particularly useful for studying cellular heterogeneity within tissues. This method combines second-generation techniques with single-cell isolation, making it innovative but resource-intensive. The Ion Torrent platform, a second-generation technology, is particularly noteworthy for its unique sequencing approach. Unlike traditional methods that rely on fluorescence, the Ion Torrent uses a label-free process based on changes in pH. During DNA synthesis, the incorporation of a nucleotide releases a hydrogen ion, causing a measurable change in pH. Each well in the platform’s chip contains a sensitive pH meter that detects these changes, allowing the platform to sequence DNA without the need for light or dyes. This method is faster, cost-effective, and maintains good accuracy even in challenging homopolymeric Bassal 6 regions, which posed issues for earlier technologies like the Roche 454 platform. Ion Torrent’s ability to scale effectively makes it a valuable tool in research and diagnostics. Advantages and Limitations Each sequencing generation has specific strengths and weaknesses. Sanger sequencing is highly accurate and capable of producing long reads, but it is slow and expensive, making it unsuitable for large-scale studies. Second-generation sequencing offers high-throughput capabilities and reduced costs but requires complex bioinformatics tools and struggles with repetitive sequences. Third-generation sequencing eliminates amplification errors but is still developing and less widely used. Fourth-generation sequencing provides unmatched resolution at the single-cell level but is technically demanding and costly. Understanding these advantages and limitations is crucial for selecting the appropriate sequencing approach. The choice depends on the clinical scenario, the type of genetic alteration being investigated, and the resources available. Lesson 3 – October 18th Introduction to Sequencing Generations The evolution of DNA sequencing technologies has revolutionized the field of genetics, enabling unprecedented insights into genetic disorders and molecular biology. These technologies are categorized into four generations, each addressing the limitations of its predecessors while introducing significant advancements. The progression from Sanger sequencing to modern high-throughput platforms exemplifies the rapid innovation in this domain. First-Generation Sequencing: The Sanger Method The foundation of DNA sequencing lies in the Sanger method, renowned for its exceptional accuracy of 99.9%. This method involves amplifying DNA through polymerase chain reaction (PCR) and incorporating dideoxynucleotide triphosphates (ddNTPs). These ddNTPs lack a hydroxyl group, terminating DNA synthesis at specific points. Tagged with fluorescent dyes corresponding to individual nucleotides (A, T, C, or G), the ddNTPs enable the generation of fragments of varying lengths. Capillary electrophoresis separates these fragments by size, and their sequence is determined based on the emitted fluorescence. While Sanger sequencing remains a gold standard in diagnostic accuracy, it has critical limitations. It is time-intensive, often requiring months to sequence a single gene, and its cost, exceeding €1,000 per gene, renders it impractical for large-scale projects. These constraints have driven the development of more advanced sequencing technologies. Second-Generation Sequencing: High-Throughput Revolution Second-generation sequencing introduced high-throughput capabilities, transforming DNA analysis by enabling the simultaneous sequencing of millions of fragments. One of the earliest platforms in this generation, Roche 454, employed sequencing by synthesis, recording nucleotide incorporation during DNA Bassal 7 replication. However, Roche 454 faced challenges, particularly in homopolymeric regions, where repeated bases caused frequent errors. Despite its eventual obsolescence, Roche 454 laid the groundwork for subsequent technologies like Illumina sequencing. Illumina’s dominance stems from its scalability, affordability, and precision. This platform introduced the concept of library preparation, where DNA fragments are enriched and labeled with unique molecular barcodes (indices). Pooling these samples for simultaneous analysis allowed for greater efficiency, making Illumina sequencing an indispensable tool for research and diagnostics. Third-Generation Sequencing: Single-Molecule Accuracy Third-generation sequencing eliminated the need for DNA amplification, addressing a significant source of errors in earlier methods. Technologies such as PacBio rely on single-molecule sequencing, which sequences DNA directly from extracted samples. By avoiding amplification, these platforms reduce the risk of introducing artifacts and provide long reads. This capability is particularly valuable for analyzing structural variants, repetitive regions, and large insertions or deletions, offering a clearer view of complex genomic structures. Fourth-Generation Sequencing: Single-Cell Precision Building on the advancements of second-generation sequencing, the fourth generation focuses on single- cell genomics. This method isolates DNA or RNA from individual cells, allowing researchers to study cellular heterogeneity within tissues. Amplification and sequencing techniques similar to those in the second generation are employed, but the focus on single-cell data provides unparalleled insight into cellular diversity. This technology is invaluable in cancer research and developmental biology, where understanding variations at the single-cell level can illuminate critical biological processes. Illumina Sequencing: Platforms and Workflow Illumina sequencing is a dominant technology in genomics, known for its versatility and efficiency. Its platforms—such as NextSeq and NovaSeq—are tailored to meet different needs, from diagnostic laboratories to high-throughput research facilities. These platforms rely on the sequencing by synthesis (SBS) method, which involves library preparation, clustering, sequencing, and data analysis. Illumina Sequencing: Platforms and Workflow Illumina offers multiple sequencing platforms, each designed for specific scales of operation: NextSeq: Commonly found in hospital diagnostic labs, NextSeq is a compact and efficient platform capable of generating hundreds of millions of reads per run. It is particularly suitable for medium- scale applications, such as targeted panels or smaller whole-genome sequencing projects. NovaSeq: A high-capacity platform typically used in research institutions, NovaSeq generates billions of reads per run and supports large-scale projects such as population genomics or extensive Bassal 8 transcriptome studies. Its advanced clustering capabilities and massive parallel sequencing make it ideal for high-throughput applications. While these platforms differ in scale, they share the fundamental principles of library preparation and sequencing by synthesis. The choice of platform depends on the specific experimental or diagnostic requirements, such as the number of samples, size of the target region, and desired depth of coverage. Library Preparation: The Foundation Library preparation is the first step in the Illumina workflow, transforming DNA into a format suitable for sequencing. The process involves: Fragmentation: DNA is broken into fragments of approximately 300 base pairs. Addition of Molecular Elements: These include: Primers (Read 1 and Read 2): Starting points for sequencing, analogous to forward and reverse primers in PCR. Indices (Molecular Barcodes): Unique short sequences assigned to each sample, enabling pooling of multiple samples in a single run. These indices are crucial for identifying the source of each sequence during data analysis. Adapters (P5 and P7): Sequences that attach DNA fragments to the flow cell, a solid support on which the sequencing occurs. The structured library ensures the success of downstream steps, as missing components can lead to failed sequencing or unusable data. Clustering and Bridge Amplification After library preparation, the DNA fragments are loaded onto the flow cell, where they hybridize to oligos complementary to the adapters. During bridge amplification, fragments bend to form bridges, which are then copied multiple times. This process creates clusters of identical DNA sequences, amplifying the signal for accurate base calling. Sequencing by Synthesis: Core Technology Sequencing by synthesis (SBS) is the hallmark of Illumina technology. It involves the stepwise addition of fluorescently tagged nucleotides to DNA fragments. When a nucleotide is incorporated, its fluorescence is emitted and captured by a high-resolution camera. The process repeats base by base, generating billions of reads. A key feature of Illumina SBS is the use of modified nucleotides. Unlike the terminators used in Sanger sequencing, these nucleotides allow continuous synthesis without interrupting the process. The emitted fluorescence not only identifies the base but also links the sequence to its specific sample via the barcode index. Data Quality and Metrics Bassal 9 Illumina employs rigorous quality control measures to ensure reliable results, monitored through key metrics: Q Score: Predicting Sequencing Accuracy The Q score quantifies the likelihood of sequencing errors. A Q30 score represents 99.9% accuracy, meaning only one in 1,000 bases is expected to be incorrect. Illumina platforms consistently achieve high Q scores, and reads failing to meet the minimum threshold are filtered out during primary data analysis. Read Depth and Coverage Read Depth: This measures how many times a specific DNA region is sequenced. Higher depths increase confidence in variant detection and reduce the impact of random errors. Coverage: Coverage reflects the average number of reads spanning the target region, reported as “nX” (e.g., 100X coverage). High coverage ensures robust data and is critical for detecting rare variants or providing diagnostic reliability. NextSeq and NovaSeq in Data Generation Platforms like NextSeq generate 400–500 million reads per run, making them suitable for focused diagnostic applications. In contrast, NovaSeq produces up to 10 billion reads, supporting large-scale research projects. Depending on the experimental setup, NovaSeq can generate 6,000 gigabytes of data per run, while NextSeq runs typically produce smaller datasets. Storage and Bioinformatics Challenges The high output of Illumina platforms poses challenges in data storage and analysis. NextSeq and NovaSeq generate vast amounts of sequencing data, requiring specialized infrastructure to manage storage and computational pipelines. Data Storage Storage limitations arise due to the volume of sequencing data. Clusters of storage systems are necessary to house the data, as privacy concerns restrict the use of cloud-based solutions in clinical settings. For instance, in diagnostic laboratories, DNA samples and their associated sequencing data must be retained for years, further compounding storage demands. Bioinformatics Pipelines Data analysis in Illumina sequencing occurs in three stages: 1. Primary Analysis: Converts raw data into usable file formats like FASTQ, discarding low-quality reads. 2. Secondary Analysis: Aligns reads to a reference genome, identifying variants such as single nucleotide polymorphisms (SNPs) or structural variations. Bassal 10 3. Tertiary Analysis: Interprets and prioritizes detected variants, linking them to phenotypes or diseases. This step integrates clinical data, requiring collaboration between bioinformaticians, geneticists, and clinicians. Lesson 4 – October 24th Introduction to NGS Data Analysis Next-generation sequencing (NGS) data analysis is a complex process involving three distinct stages: primary analysis, secondary analysis, and tertiary analysis. Each stage serves a critical role in transforming raw sequencing data into meaningful insights that can inform diagnostic and research outcomes. Primary Analysis: From Raw Data to Usable Files Primary analysis begins with the raw data generated during sequencing, typically in the form of image files (TIF files) from the flow cell. These images capture fluorescence signals emitted at each step of the sequencing-by-synthesis process, providing information about individual clusters on the flow cell. The raw data is converted into binary files, which consolidate all sequencing information into a single file. However, for downstream analysis, it is necessary to demultiplex the data, associating each read with its specific sample. This process generates the FASTQ file, a vital output of primary analysis. The FASTQ file contains comprehensive details about the sequencing run, including the instrument used, the date of sequencing, and the specific nucleotide sequences detected in each sample. Each sample has its own FASTQ file, which is essential for all subsequent analyses. This computational process, often performed by bioinformaticians, can take several hours, and the primary analysis phase frequently extends beyond the time required for sequencing itself. Secondary Analysis: Alignment and Variant Calling In the secondary analysis phase, the reads from the FASTQ files are aligned to a reference genome. This alignment process is performed using bioinformatic algorithms and software such as BWA or Bowtie. However, this step is particularly tricky, as errors can be introduced depending on the algorithms and pipelines used. Moreover, there are no universal guidelines dictating which tools are best for robust and accurate data processing. A notable example of challenges during alignment involves genes like SHOX, located in pseudo-autosomal regions of both the X and Y chromosomes. Bioinformatic pipelines may mistakenly align reads exclusively to one chromosome, introducing errors. To mitigate such risks, it is common practice to use multiple algorithms and pipelines to ensure accuracy. Errors can also arise from inaccuracies in the reference genome itself, such as when it records minority alleles instead of the more prevalent majority alleles, leading to misleading variant interpretations. Following alignment, the BAM file is generated. This file maps the reads to the reference genome and is crucial for the subsequent variant calling process. Variant calling identifies mismatches between the sample’s DNA and the reference genome, Bassal 11 producing a list of variations. These variations are stored in the Variant Call Format (VCF) file, which is more accessible and can be analyzed using personal computers or specialized software. Tertiary Analysis: Interpretation and Prioritization of Variants Tertiary analysis is the most interpretive and time-intensive stage, requiring operators to prioritize, classify, and interpret the variants identified in the secondary analysis. The goal is to identify the mutations responsible for the patient’s clinical phenotype or underlying pathological disorder. This step often involves filtering out variations unrelated to the clinical phenotype, as not all genetic variations are causative of disease. A significant challenge is that many detected variations are novel and lack prior documentation in scientific literature or experimental studies. In such cases, predictive tools are used to estimate the potential impact of these variations. Additionally, filtering criteria such as inheritance patterns (e.g., autosomal recessive or dominant) and variant localization (e.g., exonic versus intronic regions) are applied to refine the list of candidate mutations. The tertiary analysis culminates in the generation of a clinical report. This report is reviewed independently by multiple operators to ensure accuracy and validity. Before reporting a pathogenic or likely pathogenic mutation, the variation must be confirmed using the gold standard Sanger sequencing method, which provides an additional layer of verification. Transition from Sanger to NGS Sequencing The shift from Sanger sequencing to NGS has revolutionized the diagnostic workflow, dramatically increasing efficiency and scalability. In the Sanger era, sequencing was performed one gene at a time, a process that could take months or even years, particularly for complex disorders involving multiple genes. For instance, sequencing a single gene with Sanger sequencing could take up to three months. For disorders like Brugada syndrome or long QT syndrome, which involve multiple genes, the diagnostic process was lengthy and often inconclusive. With NGS, the workflow has become more streamlined. Instead of analyzing one gene at a time, NGS enables the simultaneous sequencing of entire panels of genes associated with a phenotype. If initial analyses fail to identify causative mutations, the stored FASTQ files allow for reanalysis without requiring new samples. This flexibility is a significant advantage over Sanger sequencing, where negative results necessitate starting the process anew. Practical Applications and Case Studies The benefits of NGS are exemplified in cases like Brugada syndrome and long QT syndrome. For Brugada syndrome, caused by mutations in multiple genes, NGS facilitates the simultaneous analysis of all relevant genes, reducing diagnostic time and increasing the likelihood of identifying a causative mutation. Similarly, for long QT syndrome, which involves up to 20 genes, NGS allows researchers to analyze the entire set in a single sequencing run, providing comprehensive insights. NGS also supports familial segregation analyses, where identified mutations are traced within families to evaluate inheritance patterns and risks for Bassal 12 relatives. For example, in one case of long QT syndrome, NGS revealed a mutation inherited from the father, leading to preventive treatment for an asymptomatic sibling who also carried the mutation. Importance of Data Storage and Reanalysis The FASTQ file, the master file of the sequencing process, is crucial for long-term data storage. It retains all raw data and can be revisited for future analyses, whether to reanalyze additional genes or reevaluate a previously negative result in light of new scientific findings. This capability underscores the transformative impact of NGS on the diagnostic process, enabling iterative analyses and updates as knowledge evolves. Conclusion NGS has fundamentally transformed genetic diagnostics, offering speed, scalability, and flexibility unmatched by traditional methods like Sanger sequencing. The meticulous processes of primary, secondary, and tertiary analyses ensure that raw data are transformed into actionable clinical insights. While challenges remain, particularly in data interpretation and bioinformatic processing, the ability to analyze large datasets efficiently and revisit stored data has made NGS an indispensable tool in modern genomics. Lesson 5 – October 30th The Transition from Sanger Sequencing to Next-Generation Sequencing (NGS) in Genetic Diagnostics The introduction of next-generation sequencing (NGS) revolutionized genetic diagnostics, overcoming the limitations of Sanger sequencing. Sanger sequencing, while highly accurate with a 99.9% precision rate, is limited by its capacity to analyze only one gene at a time, making it cost-prohibitive and time-intensive. Diagnostic results often took months, and cases involving multiple gene associations frequently remained undiagnosed. This led to what was termed the “diagnostic odyssey,” reflecting a time-consuming process without actionable results. NGS has transformed this landscape by enabling massive parallel sequencing, analyzing multiple genes and samples simultaneously. This innovation significantly reduces diagnostic time, allowing for broader and deeper analyses, including entire gene panels associated with specific disorders. Moreover, data generated from NGS can be reevaluated using the FASTQ file, which contains comprehensive sequencing information. This capability eliminates the need for repeated DNA extractions or resequencing, streamlining the diagnostic workflow.and list of variant of class 4 and 5, then the conclusion and discussion related to the clinical suspicion. Introducing New Diagnostic Approaches: Validation and Sensitivity When implementing a new diagnostic approach like NGS in a laboratory, rigorous validation is required. The process involves comparing NGS results to those obtained through Sanger sequencing, the gold standard. According to guidelines from the European Society of Human Genetics, laboratories must validate NGS by analyzing samples with known genotypes—previously characterized by Sanger sequencing. This ensures that the same genetic variants are detected using the new approach. Validation includes assessing Bassal 13 repeatability within a single run and reproducibility across different runs. Repeatability involves running the same samples in triplicate within a single sequencing batch, expecting consistent results. Reproducibility tests the same samples in separate runs, often by different operators, ensuring that results are not operator-dependent. Any discrepancies highlight artifacts or errors introduced during sequencing or bioinformatics processing. A crucial aspect of validation is sensitivity. A sensitivity of 100% indicates no false negatives, ensuring that no pathogenic variants are missed. Specificity, while important, can tolerate some false positives because all detected variants undergo confirmation through Sanger sequencing before inclusion in clinical reports. This step guarantees that only accurate and clinically significant results are reported, mitigating the impact of NGS’s slightly lower specificity compared to Sanger sequencing. NGS Diagnostic Approaches: Targeted Panels, Whole Exome Sequencing, and Whole Genome Sequencing NGS offers three diagnostic approaches: targeted panels, whole exome sequencing (WES), and whole genome sequencing (WGS). The choice depends on the clinical scenario and the level of understanding of the molecular causes of the condition. Targeted Sequencing Targeted sequencing focuses on a predefined list of genes associated with a specific disorder. It is highly effective when the molecular cause is known, allowing for focused, high-coverage analyses. However, this approach requires new sequencing runs if additional genes need investigation in the future. Whole Exome Sequencing (WES) WES captures all coding regions of the genome, enabling comprehensive analysis. By filtering out irrelevant regions during data analysis, clinicians can focus on the genes of interest. WES is advantageous for its reusability, as the data can be revisited to examine additional genes without requiring new sequencing. However, it often results in lower coverage compared to targeted panels, necessitating larger sequencing runs for high-quality data. Whole Genome Sequencing (WGS) WGS provides the most extensive analysis, covering coding and non-coding regions. It is especially useful in research settings to identify novel genetic causes of diseases. However, WGS often generates lower coverage in specific regions of interest and is less efficient for diagnostic purposes compared to targeted panels or WES. Core Disease Gene Lists and Clinical Utility For diagnostic purposes, NGS typically focuses on core disease gene lists. These lists include genes with well-established links to specific clinical phenotypes, ensuring that analyses are clinically relevant. The primary goal of diagnostic laboratories is clinical utility: confirming diagnoses, guiding patient management, and identifying at-risk family members. For instance, consider a case involving a 13-year-old Bassal 14 boy with frequent cardiac events and no family history of cardiac disorders. Clinical suspicion pointed to catecholaminergic polymorphic ventricular tachycardia (CPVT), a condition caused by mutations in specific genes. Using NGS, a large panel of cardiac disorder-associated genes was sequenced, revealing a single nucleotide variant in the RYR2 gene. This mutation, a known cause of CPVT, confirmed the diagnosis. Family testing revealed that the mutation was de novo, not present in the parents or sister, confirming its pathogenicity and indicating no increased familial risk. Incidental Findings in Genomic Sequencing A key consideration in NGS is minimizing incidental findings—genetic variants unrelated to the clinical question being investigated. The American College of Medical Genetics and Genomics (ACMG) defines incidental findings as genetic variations identified during sequencing but not linked to the patient’s presenting condition. While some laboratories choose to report incidental findings for specific genes (e.g., the ACMG’s list of 50 genes), many prefer targeted sequencing or focused data analysis to avoid these findings entirely. Incidental findings can pose ethical and psychological challenges. For example, detecting a BRCA1 mutation during cardiac disorder testing might cause unnecessary anxiety without offering immediate clinical utility. Diagnostic laboratories prioritize findings directly related to the clinical question to maintain focus on actionable results. Classification of Genetic Variants According to ACMG Guidelines Genetic variants identified during NGS analysis are classified into five categories based on guidelines established by the American College of Medical Genetics and Genomics (ACMG). These categories help standardize the interpretation of variants and provide a framework for their clinical significance: 1. Class 1: Benign Variants Variants classified as benign are those with substantial evidence indicating they do not cause disease. These include common variants found at high frequencies in healthy populations or variants that do not alter protein function. For instance, silent mutations, which do not change the amino acid sequence of a protein, often fall into this category. Criteria such as a high minor allele frequency (MAF) in population databases like gnomAD and lack of co-segregation with disease strongly support a benign classification. 2. Class 2: Likely Benign Variants Likely benign variants have evidence that leans toward non-pathogenicity, though it may not be as definitive as for benign variants. These are often observed in healthy individuals but lack enough data to conclusively classify them as benign. Examples include variants with slight functional changes that are not linked to any phenotype or variants in non-conserved regions of the genome. 3. Class 3: Variants of Uncertain Significance (VUS) Variants of uncertain significance are the most challenging to interpret. These are variants for which the current evidence is insufficient to determine whether they are pathogenic or benign. Factors such as a lack Bassal 15 of population data, conflicting in silico predictions, or absence of functional studies contribute to this uncertainty. Class 3 variants do not have immediate clinical utility because their potential impact on disease remains unclear. Despite this, they are often included in reports, especially when familial segregation studies may provide additional insights. 4. Class 4: Likely Pathogenic Variants Likely pathogenic variants are strongly suspected to contribute to disease based on available evidence, though they fall short of the rigorous criteria for pathogenicity. These variants may include those with strong in silico predictions of a damaging effect, co-segregation with disease in multiple family members, or partial experimental validation. 5. Class 5: Pathogenic Variants Pathogenic variants are those with overwhelming evidence supporting their direct role in causing disease. These include variants identified in multiple affected individuals in different families, those associated with well-characterized functional disruptions (e.g., frameshifts, nonsense mutations), or those already documented in clinical and population databases as causative for a particular disorder. Criteria for Classification and Scoring The ACMG guidelines outline several criteria for evaluating and scoring genetic variants. Each criterion is assigned a weight (strong, moderate, or supporting), and the cumulative evidence determines the final classification: 1. Frequency in Population Databases A variant’s frequency in the general population is crucial. Common variants (e.g., MAF >5%) are usually classified as benign, while rare variants may be pathogenic. This criterion relies on databases like gnomAD or ExAC. 2. Type of Mutation The nature of the genetic change significantly influences its classification. For example: Frameshift mutations and nonsense mutations often result in loss of function and are classified as pathogenic. Silent mutations that do not alter amino acid sequences are generally benign. Missense mutations require further analysis, as they may have varying effects. 3. In Silico Predictions Computational tools such as PolyPhen, SIFT, and MutationTaster predict the impact of a variant on protein function. A consistent prediction of damaging effects across multiple tools adds weight toward pathogenicity, but discrepancies require cautious interpretation. 4. Phenotypic Associations Bassal 16 Variants in genes with established links to the patient’s phenotype carry more weight. For example, a variant in a cardiac gene identified in a patient with a cardiac disorder supports pathogenicity. 5. Conservation of Amino Acid Residues Highly conserved amino acid residues across species suggest functional importance. Changes in these residues are more likely to be pathogenic compared to changes in non-conserved regions. 6. Experimental Evidence Functional studies demonstrating that a variant alters gene or protein function significantly support pathogenicity. The absence of experimental data, however, often limits classification. 7. Segregation and Familial Studies Co-segregation of a variant with disease in multiple affected family members provides strong evidence for pathogenicity. Conversely, its presence in unaffected individuals might suggest a benign classification. Operator-Dependent Nature of Classification While the ACMG provides structured guidelines, classification often involves subjective interpretation, making it operator-dependent. This limitation means two operators might assign different scores based on the same data. To address this, many laboratories employ double-blind analyses, where two independent operators classify variants and compare results for consistency. Clinical Utility and Reporting For diagnostic purposes, laboratories prioritize reporting class 4 (likely pathogenic) and class 5 (pathogenic) variants due to their immediate clinical relevance. Class 3 variants (VUS) may or may not be reported, depending on the laboratory’s policy and the potential for future reclassification. Class 1 and 2 variants are generally excluded from clinical reports unless their benign nature is explicitly relevant to the case. Reclassification of variants is an ongoing process, as new data from population studies, functional experiments, or computational analyses emerge. Laboratories must maintain comprehensive records, particularly of class 3 variants, to facilitate future updates. Lesson 6 – November 5th Genetic testing has become a cornerstone of diagnostic medicine, especially in identifying and managing inherited cardiac disorders. These disorders pose unique challenges due to their genetic and clinical complexity, requiring a careful integration of molecular diagnostics, bioinformatics, and clinical judgment. This document delves into the intricacies of inherited cardiac disorders, the role of genetic testing, and the broader implications for patients and their families. Inherited Cardiac Disorders: Structural and Arrhythmogenic Variants Inherited cardiac disorders can be broadly divided into two categories: structural disorders, characterized by physical changes in the heart muscle, and arrhythmogenic (electrical) disorders, which primarily involve abnormalities in the heart’s electrical conduction system. Bassal 17 Structural Disorders Structural cardiac disorders are associated with alterations in the cardiac muscle. These changes can often be detected using imaging techniques such as echocardiography. Examples of structural disorders include hypertrophic cardiomyopathy (HCM), characterized by thickened myocardial walls; dilated cardiomyopathy (DCM), where the heart muscle becomes enlarged and weakened; and arrhythmogenic right ventricular dysplasia (ARVD), marked by the replacement of myocardial cells with fibrofatty tissue, primarily affecting the right ventricle. These structural abnormalities can lead to electrical conduction issues, further complicating the clinical picture. Arrhythmogenic Disorders Arrhythmogenic disorders, on the other hand, are primarily electrical in nature. They involve disruptions in the heart’s conduction system without significant structural changes. Examples include Long QT Syndrome (LQTS), Brugada Syndrome, and Catecholaminergic Polymorphic Ventricular Tachycardia (CPVT). These conditions are often undetectable through routine clinical evaluations but may be identified via an electrocardiogram (ECG), which can reveal abnormalities in the electrical conduction of the heart. Despite their distinct features, structural and arrhythmogenic disorders share a common thread: both can result in severe outcomes such as heart failure or sudden cardiac arrest, often occurring at a young age and without prior symptoms. This shared clinical endpoint underscores the importance of genetic testing in uncovering the underlying causes and guiding patient management. Phenotypic Variability: Incomplete Penetrance and Variable Expressivity A hallmark of inherited cardiac disorders is their phenotypic variability, driven by two key genetic mechanisms: incomplete penetrance and variable expressivity. Incomplete penetrance refers to cases where individuals carry a pathogenic mutation but do not exhibit the associated phenotype. For example, in LQTS, only about 20% of individuals with a mutation actually show clinical symptoms, such as prolonged QT intervals or arrhythmias. This phenomenon deviates from the classical Mendelian inheritance patterns seen in monogenic disorders, complicating risk assessment for asymptomatic carriers. Variable expressivity, on the other hand, describes the variation in phenotype severity among individuals with the same genetic mutation, even within the same family. For instance, one individual with LQTS may experience sudden cardiac arrest, another may show only a prolonged QT interval, and another may remain asymptomatic. This variability makes counseling and managing families particularly challenging, as genetic test results do not always correlate with clinical presentation. These genetic phenomena highlight a critical gap in our understanding of the molecular underpinnings of these disorders. Research aimed at unraveling these complexities is essential for improving diagnostic accuracy and enabling more precise risk stratification. The Genetic Basis of Cardiac Disorders: Oligogenic Inheritance and Detection Rates Bassal 18 Inherited cardiac disorders often exhibit oligogenic inheritance, meaning that multiple genes contribute to the clinical phenotype. For example, Brugada Syndrome is associated with mutations in more than 20 genes, while DCM involves over 50 associated genes. This genetic heterogeneity complicates both diagnosis and management. Despite extensive gene panels targeting known causative mutations, detection rates remain relatively low in many cardiac disorders. For instance, in Brugada Syndrome, the detection rate is approximately 30%, meaning that 70% of patients lack a definitive genetic diagnosis even after testing all currently known associated genes. This contrasts sharply with monogenic conditions like cystic fibrosis, where a single gene (CFTR) accounts for nearly 100% of cases. It is crucial to communicate to patients that genetic testing in cardiac disorders serves to confirm a diagnosis rather than exclude it; negative results do not definitively rule out the presence of a condition. Overlapping Genetics and Clinical Presentations Adding to the complexity, inherited cardiac disorders exhibit significant genetic and clinical overlap. For example, the SCN5A gene, which encodes a sodium ion channel, is implicated in Brugada Syndrome, LQTS, and DCM. This overlap means that mutations in the same gene can lead to entirely different clinical phenotypes, depending on other genetic and environmental factors. This genetic overlap underscores the necessity of starting with a clear clinical characterization before initiating genetic testing. Without a well- defined clinical suspicion, interpreting genetic findings becomes challenging, often leading to inconclusive or ambiguous results. Laboratory Workflow for Genetic Testing Genetic testing for inherited cardiac disorders involves a meticulous and multi-step process to ensure accuracy and reliability: 1. Sample Collection and Quality Control: DNA is extracted from peripheral blood or saliva, and its quality is rigorously assessed before sequencing. 2. Library Preparation and Sequencing: Libraries are prepared for next-generation sequencing (NGS), focusing on panels of genes associated with inherited cardiac disorders. 3. Bioinformatics Analysis: Sequencing data is aligned to a reference genome, and variants are identified and prioritized based on established guidelines, such as those from the American College of Medical Genetics and Genomics (ACMG). 4. Variant Classification and Confirmation: Variants classified as pathogenic or likely pathogenic (Class 4 or 5) are confirmed using Sanger sequencing. Variants of uncertain significance (Class 3) are noted but may lack immediate clinical utility. 5. Clinical Reporting: Bassal 19 A detailed report is generated, including the methodology, sequencing quality parameters, and identified variants relevant to the clinical suspicion. Challenges in Interpretation: The Role of Variants of Uncertain Significance A significant challenge in genetic testing for cardiac disorders is the prevalence of variants of uncertain significance (VUS). In a study involving 130 patients, 108 genetic variants were identified across 38 genes, but only three were classified as pathogenic. Seventy percent of cases involved VUS, underscoring the difficulty in drawing clinically actionable conclusions from genetic data. A Real-World Case Study: Complexities in Clinical Application Consider a case of a young asymptomatic boy with a family history of sudden cardiac death. Despite comprehensive clinical evaluations, no abnormalities were detected. Genetic testing revealed a VUS in the RYR2 gene, a known causative gene for CPVT. Further familial segregation analysis showed the same variant in multiple relatives, but its role in the phenotype remained uncertain. This case illustrates the limitations of genetic testing in the absence of a clear clinical context. While the findings offered some insights, they also introduced significant psychological stress for the family without directly influencing clinical management or outcomes. Conclusion Inherited cardiac disorders are among the most challenging conditions in genetic diagnostics due to their phenotypic variability, genetic heterogeneity, and overlapping clinical features. While genetic testing is an invaluable tool for confirming diagnoses and informing management, its limitations, particularly the prevalence of VUS and low detection rates, must be acknowledged. Effective genetic testing requires a strong clinical foundation, highlighting the importance of collaboration between clinicians, geneticists, and bioinformaticians. Ongoing research into these disorders is essential to enhance diagnostic accuracy, improve risk stratification, and provide better care for patients and their families. Lesson 7 – November 6th Undiagnosed Diseases The study and management of undiagnosed diseases represent a critical area of focus within genetic diagnostics. While rare diseases are defined as disorders present at a low frequency in the population (for example, the European Union defines rare disorders as those occurring in fewer than 5 in 10,000 individuals), they are distinct from undiagnosed diseases. Rare diseases have known genetic backgrounds, characterized symptoms, and identifiable clinical features, which facilitate their diagnosis and management. In contrast, undiagnosed diseases are conditions that elude diagnosis, leaving patients and clinicians in a state of uncertainty. These conditions may lack molecular characterization, have overlapping phenotypes with other disorders, or involve complex symptoms that make it difficult to assign a definitive diagnosis, prognosis, or therapy. The implications of undiagnosed diseases are significant. From a clinical perspective, Bassal 20 diagnosis is the first and most critical step in managing a patient. Without a clear diagnosis, it is nearly impossible to develop an effective treatment plan or improve the patient’s quality of life. For patients with undiagnosed diseases, this lack of clarity can lead to years of uncertainty, ineffective treatments, worsening symptoms, and, in some cases, a poor prognosis. From a systemic perspective, the absence of a diagnosis places a substantial burden on healthcare systems, leading to unnecessary testing, ineffective therapies, and associated costs. Addressing the Problem: The UDP Initiative Recognizing the challenges posed by undiagnosed diseases, several national and international efforts have emerged to address this issue. One notable initiative is the Undiagnosed Disease Program (UDP), established more than a decade ago by the National Institutes of Health (NIH). The UDP specifically focuses on meeting the clinical needs of patients with undiagnosed diseases, rather than those with rare but characterized disorders. This distinction is crucial, as the UDP addresses a subset of conditions that are often more complex and enigmatic than those typically classified as rare. The UDP operates as a network of clinical and research centers, supported by the NIH, with the goal of identifying diagnoses and elucidating the molecular basis of undiagnosed diseases. Within the first two years of its establishment, the UDP received thousands of applications from individuals and healthcare providers seeking answers for unexplained symptoms and conditions. The program has succeeded in identifying new syndromes, uncovering novel clinical features of known syndromes, and correcting misdiagnoses in numerous cases. The Diagnostic Workflow of the UDP The UDP employs a structured diagnostic workflow that begins with the submission of a recommendation letter, which describes the clinical case. This submission can come from healthcare providers or directly from the patients themselves. The cases are collected by the program’s coordinating center, and weekly interactive calls are held among the participating centers to evaluate and prioritize the submitted cases. Given the high volume of applications, prioritization is essential, and cases with a higher likelihood of resolution based on prior data or clinical clarity are selected for further investigation. Once selected, patients undergo detailed clinical evaluations at one of the seven clinical sites in the program, with no cost to the patient. Depending on the clinical findings, various diagnostic approaches are employed, including genome sequencing, exome sequencing, proteomics, and metabolomics. In some cases, if sequencing has already been performed elsewhere, previous data are reanalyzed using advanced tools or novel approaches. The comprehensive collection of clinical, genetic, and biochemical data allows for a holistic reevaluation of the case, aiming to identify a diagnosis or at least better characterize the underlying condition. Collaboration and Data Sharing A central tenet of the UDP is the importance of data sharing. The program maintains a public website where information about identified variants, genetic mutations, and clinical characterizations of evaluated patients Bassal 21 is made available. This transparency serves a dual purpose: it allows other physicians and researchers to recognize similar cases, potentially leading to more diagnoses, and it fosters a collaborative environment in which knowledge can be expanded. This shared information often includes detailed descriptions of clinical symptoms, genetic findings, and proposed diagnostic hypotheses. For example, cases with overlapping phenotypes, such as dementia, cardiomyopathy, or metabolic abnormalities, may be published alongside molecular findings, enabling global comparisons and collaborations. The goal is to improve diagnostic success rates and facilitate more accurate classifications of undiagnosed conditions. The Importance of Standardized Terminology: HPO Terms One critical tool in the field of undiagnosed diseases is the Human Phenotype Ontology (HPO), a database that provides standardized terms for describing human symptoms. Each symptom is assigned an HPO term, which includes a unique numerical identifier. This standardization ensures that clinicians and researchers worldwide can refer to the same symptom using identical terminology, minimizing ambiguities. For example, a symptom like ventricular tachycardia can be further categorized into specific types (e.g., bidirectional, polymorphic, or torsades de pointes) using HPO terms. This level of granularity is particularly useful in complex cases, such as neurological disorders, where overlapping symptoms may have varying severities or presentations. The HPO database allows for more precise communication among medical professionals and is especially valuable in research settings, where prioritizing data and correlating phenotypes with genetic findings are critical. Diagnostic Outcomes and Classification of Findings The UDP has made significant progress in diagnosing undiagnosed diseases. Diagnoses fall into several categories: Unusual presentations of already characterized syndromes: In 58% of cases, the diagnosis involved a known syndrome with atypical symptoms, highlighting the importance of reexamining clinical assumptions. New syndromes associated with known genes: In 12% of cases, the identified condition represented a novel manifestation of a gene already linked to other disorders. New syndromes associated with new genes or regions: Some cases revealed entirely new syndromes linked to previously uncharacterized genes or genomic regions. Novel presentations of new syndromes: These comprised 18% of the diagnoses, emphasizing the complexity of undiagnosed conditions. To date, the UDP has described 31 new syndromes, each representing a step forward in understanding rare and undiagnosed diseases. These findings have significant implications for patient care, enabling more effective treatments, lifestyle recommendations, and genetic counseling for affected families. Case Study: A Pediatric Cardiac Disorder Bassal 22 One notable case involved a two-year-old boy who suffered sudden cardiac arrest at home. Resuscitated and hospitalized, the boy was found to have an elongated QT interval (575 ms) and recurrent ventricular tachycardia. Despite the implantation of a cardioverter-defibrillator and various antiarrhythmic treatments, the boy experienced multiple cardiac events daily. His siblings had died of unexplained causes at ages two and three, raising suspicions of a familial genetic disorder. Initial genetic testing for Long QT Syndrome (LQTS) was negative, but whole-exome sequencing (WES) revealed a mutation in the TRDN gene, encoding triadin, a key protein in cardiac calcium regulation. Functional studies using zebrafish models demonstrated that the mutation disrupted calcium handling in cardiomyocytes, leading to abnormal heart rhythms. The case led to the identification of a new condition, triadin knockout syndrome, characterized by malignant arrhythmias in young children. The Role of Animal Models in Understanding Undiagnosed Diseases In some cases, genetic findings must be validated and studied using animal models. The UDP has incorporated research centers specializing in the development of models such as zebrafish and Drosophila (fruit flies). Zebrafish, in particular, are advantageous for studying cardiac and skeletal muscle disorders due to their rapid development, transparent embryos, and similarities in cardiac physiology to humans. For example, a mutation identified in a patient may be introduced into zebrafish to observe its effects on heart function or skeletal development. Functional studies in these models help establish a causative link between a genetic variant and a phenotype, providing essential evidence for a diagnosis. Broader Implications The work of the UDP has had far-reaching implications, not only for individual patients and families but also for the broader scientific community. By addressing the challenges of undiagnosed diseases, the program has paved the way for advancements in genetic and clinical research, created opportunities for international collaboration, and fostered a deeper understanding of human genetics. Conclusion In summary, undiagnosed diseases represent a unique and challenging subset of conditions that require specialized approaches for diagnosis and management. The UDP’s comprehensive framework, from data sharing to advanced genetic analyses and functional studies, serves as a model for addressing these enigmatic disorders. While much work remains to be done, the program’s success underscores the importance of continued investment and collaboration in the field of genetic diagnostics. Lesson 8 – November 13th The diagnosis of genetic diseases often involves the identification of insertions and deletions (indels), a class of genetic mutations that range from small alterations involving a few base pairs to large structural changes spanning entire genomic regions or chromosomes. The choice of diagnostic methodology depends on the size and nature of these mutations, as different approaches offer varying strengths and limitations. Bassal 23 This comprehensive overview integrates key insights from the transcript and notes, detailing the principles, applications, and limitations of various diagnostic techniques while ensuring every nuance is retained. The Role of Sequencing Technologies in Indel Detection Sequencing technologies, particularly first- and second-generation sequencing, are the most widely used tools in diagnostic laboratories. They excel at identifying small-scale genetic mutations, including single- nucleotide variants and small indels ranging from two to 20 base pairs. However, their efficacy diminishes with larger genetic alterations. Amplification-based sequencing methods cannot detect deletions spanning multiple exons because these regions fail to produce amplicons during the sequencing process. Similarly, large structural variations involving several megabases or entire chromosomes remain undetectable. For these large-scale alterations, cytogenetic methods such as karyotyping, fluorescence in situ hybridization (FISH), or comparative genomic hybridization (CGH) arrays are better suited. These approaches provide the resolution necessary to identify gross chromosomal abnormalities but are less effective for medium- sized indels. This diagnostic gap necessitates specialized methodologies that complement the capabilities of sequencing and cytogenetics. Diagnostic Pathways for Indel Detection Based on Size The identification of indels requires selecting a methodology tailored to the size of the alteration. For small indels, sequencing approaches are sufficient, whereas chromosomal-level insertions or deletions necessitate cytogenetic tools. Medium-sized indels, such as those involving multiple exons or parts of a gene, demand alternative methodologies that offer precision and quantification. These include Multiplex PCR of Short Fluorescent Fragments (MPSF), Quantitative Multiplex PCR of Short Fluorescent Fragments (QMPSF), Multiplex Amplification and Probe Hybridization (MAPH), and Multiplex Ligation-Dependent Probe Amplification (MLPA). Each method provides unique advantages suited to specific diagnostic challenges. Multiplex PCR of Short Fluorescent Fragments (MPSF) MPSF was developed as an early method for identifying large indels that fall outside the detection range of sequencing technologies. It was initially used to analyze the MLH1 and MSH2 genes, which are associated with Lynch syndrome and colorectal cancers. These conditions frequently involve large deletions or duplications that traditional sequencing approaches failed to detect. MPSF employs multiplex PCR to amplify multiple regions of a gene simultaneously. Primers labeled with fluorescent tags enable the amplified products (amplicons) to be visualized and analyzed using automated sequencers. The height of each fluorescence peak corresponds to the number of copies of the target DNA region. By comparing the fluorescence signal from the patient’s sample to that of a wild-type control, the presence of deletions or duplications can be determined. For example, a 1:1 ratio between the patient and control indicates a normal copy number, while a 0.5 ratio suggests a heterozygous deletion, and a 1.5 ratio implies a duplication. MPSF requires the simultaneous analysis of control samples alongside patient samples to ensure accurate Bassal 24 quantification. This approach has proven particularly useful for diagnosing conditions where deletions or duplications play a significant role, such as hereditary cancers. Quantitative Multiplex PCR of Short Fluorescent Fragments (QMPSF) QMPSF builds on MPSF by addressing specific challenges in analyzing technically complex genomic regions, such as BRCA1 and BRCA2, which are associated with hereditary breast and ovarian cancers. These genes are difficult to amplify due to their high GC content and homology with other genomic regions. To overcome these challenges, QMPSF uses chimerical primers with six-nucleotide extensions at their 5’ ends. These extensions help standardize melting temperatures across primers, enabling uniform amplification of all target regions. Similar to MPSF, QMPSF relies on fluorescence peaks to indicate copy number variations, requiring a comparison with control samples for interpretation. This method has significantly improved diagnostic accuracy for genes like BRCA1 and BRCA2, where primer specificity and amplification efficiency are critical. Multiplex Amplification and Probe Hybridization (MAPH) MAPH was developed to detect medium-sized indels, particularly in genes like DMD, which is associated with Duchenne and Becker muscular dystrophies. These conditions often involve deletions or duplications spanning multiple exons. In MAPH, genomic DNA is denatured and hybridized to a set of specific probes immobilized on a nylon membrane. After hybridization, unbound DNA and probes are washed away, leaving only hybridized probes. These are subsequently amplified and analyzed. The amount of amplified probe correlates with the copy number of the target DNA region. However, MAPH has notable limitations. It requires a large amount of DNA (approximately one microgram), which can be challenging to obtain in clinical scenarios such as prenatal testing. Furthermore, the washing steps increase the risk of artifacts, leading to potential false positives or negatives. Despite these limitations, MAPH was instrumental in identifying copy number variations in genes associated with muscular dystrophies and served as the foundation for more advanced techniques like MLPA. Multiplex Ligation-Dependent Probe Amplification (MLPA) MLPA addresses the key limitations of MAPH while retaining its core principles. It performs hybridization in solution, eliminating the need for a nylon membrane and reducing the risk of artifacts. Additionally, MLPA requires significantly less DNA (in the nanogram range), making it suitable for various diagnostic applications, including prenatal testing. In MLPA, two adjacent probes are designed to hybridize to a target DNA region. If both probes bind successfully, they are ligated to form a single probe, which is then amplified using universal primers. The amplified product is analyzed to determine copy number variations. A distinctive feature of MLPA is the inclusion of a stuffer region in each probe. This region, which is not complementary to the target DNA, varies in length across probes, allowing precise identification of amplified products. Without this stuffer region, all probes would produce amplicons of identical length, Bassal 25 making it impossible to distinguish between them. MLPA has emerged as the gold standard for detecting medium-sized indels. It is widely used in diagnosing hereditary breast and ovarian cancers, Long QT syndrome, and chromosomal aneuploidies such as Down syndrome. Its accuracy, versatility, and ease of use make it an indispensable tool in modern diagnostics. The Function of the Stuffer Region in MLPA A unique feature of MLPA is the incorporation of a stuffer region in each probe. The stuffer region, characterized by variable lengths, ensures that amplified probes differ in size, enabling their precise identification. Without the stuffer region, all probes would produce amplicons of identical length, leading to ambiguity in the results. This feature allows MLPA to achieve unparalleled specificity and accuracy in identifying copy number variations. Practical Applications and Comparative Insights The methodologies discussed—MPSF, QMPSF, MAPH, and MLPA—each address specific challenges in the detection of indels. While MPSF and QMPSF are ideal for analyzing regions with high GC content or sequence homology, MAPH and MLPA excel in detecting larger deletions and duplications. MLPA, in particular, stands out for its robustness, accuracy, and ability to work with minimal DNA. It has become a cornerstone in the diagnostic workflow for conditions characterized by copy number variations. By integrating these methodologies, diagnostic laboratories can achieve comprehensive indel detection, ensuring accurate diagnoses and informed genetic counseling. These tools collectively bridge the gap between sequencing and cytogenetics, ensuring that mutations of all sizes are effectively identified and characterized. Lesson 9 – November 14th Overview of Mendelian inheritance Today, I am going to discuss the genetic testing and the molecular diagnostic approach for breast and ovarian cancer. Before we begin, let's briefly recap some key concepts in medical genetics, which you may already be familiar with. In the Mendelian disorders, we observe a straightforward genetic feature associated with a single genetic locus that is causative for a specific clinical phenotype. In classical Mendelian disorders, the causative gene is responsible for 100% of the cases affected by that disorder. Mendelian inheritance can follow different patterns for inheritance, including autosomal dominant, autosomal recessive, or X linked inheritance. However, even in Mendelian cases, certain genetic features may be causative of a heterogeneous phenotype. As we discussed in the context of cardiomyopathies, these features include penetrance and expressivity. Incomplete penetrance and the variable expressivity can create a heterogeneous scenario, where patients affected by the same disorder, or familial members carrying the same mutation, may exhibit different degrees or severities of a specific clinical phenotype. Inherited Human Diseases Bassal 26 This is the typical picture of a classical Mendelian disorder. However, in the human genetics, we also recognize the existence of multifactorial disorders, which differ from the heterogeneous disorders. In the multifactorial disorders, both genetic background and environmental factors contribute to the onset of the clinical phenotype. You can imagine a triangle representing this relationship. At one point of the triangle, we have polygenic disorders, where multiple genes are associated with the phenotype. At another point, we have single - gene disorders, where a single gene serves as the co-molecular cause of the disorder. Finally, at the third point, we have the environmental factors. When only one gene is responsible for a clinical onset, we classify it as classical Mendelian disorder. If we encounter a heterogeneous scenario within a Mendelian disorder, features such as reduced penetrance, variable expressivity, etc. may introduce variability into the clinical presentation. Similarly, in phylogenic case, a heterogeneous scenario may arise because multiple genes contribute to the same clinical phenotype. When environmental factors are also involved, the result is a multifactorial disorder. Therefore, to conduct effective diagnostic testing for our patients, it is essential to thoroughly understand the molecular basis we need to investigate. Etiology of Phenotypic Traits: Factors Influencing The Phenotype So far, Mendelian disorders have been relatively well-characterized, making genetic testing possible in many cases. The problem, based on our current knowledge, lies with disorders influenced by polygenic and environmental factors. These cases involve multiple genes associated with a disorder and various environmental factors - such as lifestyle, diet, and other external factors - that contribute to variability in the onset of the disorder. When the roles and relative weights of genetic and environmental factors are unclear, the clinical utility of genetic testing remains limited. This is particularly evident in oncological disorders. In these cases, a panel of genes may be associated with the same phenotype - such as breast and ovarian cancer, as I will demonstrate - and environmental factors also play a significant role. Environmental factors may ultimately determine whether the clinical phenotype manifests, even when genetic alterations are present. Consequently, it is often challenging to perform effective risk stratification to evaluate an individual's likelihood of developing the disorder, even if they carry one or more altered genes. I will present two contrasting examples: breast and ovarian cancer, where the influence of environmental factors is not yet well-characterized, and thrombophilia, where the role of environmental factors is well-established. In the case of thrombophilia, risk stratification for individuals carrying genetic mutations is feasible, even if the individual has not yet developed the disorder, allowing for predictive testing. For breast and ovarian cancer, while the goal is to achieve a similar level of predictive accuracy, our current limited understanding means we can only partially assess the risk for a healthy individual with a genetic predisposition. Human Breast and Ovarian Cancer So, let's start with breast and ovarian cancer, where we don't have causative genes but rather susceptibility genes. Breast cancer can be considered as two distinct forms of disease. What does it mean? It means that Bassal 27 there is a relatively rare form with an early onset and a genetic inheritance pattern similar to Mendelian inheritance. It is possible to recognize an autosomal dominant inheritance in these cases. On the other hand, there is a more common form, characterized by a late onset and associated genetic factors. However, it is more challenging to identify a clear pattern of genetic inheritance in these cases. Therefore, genetic counseling is essential to characterize the family history of the individual or patient. By analyzing the pedigree, we can determine whether the case falls into the rare familial form or the more common sporadic form. From a clinical perspective, both forms present the same features, phenotype and treatment strategies. However, at the molecular level, they represent two distinct scenarios. In one case, the genetic testing may be useful, whereas in the other, its role in clinical management is limited. Sporadic Form For example, consider a pedigree with only one affected individual in the family, aged 70 years old. This case suggests a late-onset, sporadic form of breast cancer, where only one family member is affected. What might be the pathogenic mechanism in this case? Likely, the individual has two wild-type alleles, which are healthy alleles - gene that function normally. Over the course of their life, exposure to environmental factors such as diet, radiation, smoking, etc., leads to the accumulation of somatic mutations. When enough mutations accumulate in the relevant organ, genetic alterations may result in the onset of the tumor. Familial Form Now, consider a different pedigree where multiple family members are affected. This suggests an autosomal dominant inheritance pattern. What might be the pathogenic mechanism here? In this scenario, one mutation is already present at birth, inherited as a germline mutation. Exposure to environmental factors then leads to the accumulation of additional mutations, resulting in tumor development. In this case, there is an early onset of disease, and multiple family members may be affected. This familial form contrasts with the sporadic form. In the familial form, diagnostic genetic testing can be clinically useful and provides utility for managing the patient. Background and Overview of HBOC Unfortunately, breast and ovarian cancer are among the most prevalent oncological disorders worldwide, affecting a broad age range, typically between 20 and 50 years of age. Individuals carrying genetic mutations, or those with a genetic predisposition, have a higher risk of developing breast cancer than the general population. For example, if we plot age (years) on the X-axis, the estimated risk of developing breast cancer is significantly higher for mutation carriers compared to the wild-type population. What about the prevalence of sporadic and familial forms? As mentioned earlier, inherited forms are rarer than sporadic forms for both breast and ovarian cancer. Familial cases, where diagnostic genetic testing has clinical utility, represent the minority of cases. Gene Associated To Higher Susceptibility To Breast Cancer Bassal 28 Which are the main genes associated with breast and ovarian cancer? The most well-known genes are BRCA1 and BRCA2, first described and characterized by Dr. Henry Lynch, the same doctor who later identified Lynch syndrome, a form of colorectal cancer. Dr. Lynch identified the loci for these genes on chromosomes 17 and 13, where BRCA1 and BRCA2 are located. BRCA1 and BRCA2-Associated Cancers: Lifetime Risks Mutations in BRCA1 and BRCA2 are associated with a significantly increased lifetime risk of developing cancer, often in different organs. Breast cancer accounts for 85% of these cases, typically with early onset. The second most affected organ is the ovary. In some cases, males with these mutations may also face an increased risk of prostate and pancreatic cancer. This creates a heterogeneous clinical scenario associated with mutations in these two genes. Genetic counseling is crucial in these cases. By studying the pedigree, we can hypothesize whether a case is BRCA1- or BRCA2-positive. Specific clinical features observed in the pedigree can help differentiate between the two. Family Harboring BRCA1 Mutation For example, let's examine this pedigree. Look at this family. Our proband is here, indicated by the arrow, as usual. Analyze the pedigree: what do you observe? What features stand out? The analysis of the pedigree always starts with the proband. So, what can we say about the proband? She is a 30-year-old breast cancer survivor, affected by right breast cancer. Now, moving on to other family members: are there additional affected individuals? Yes, there is a positive family history. Based on this, we can initially hypothesize that this is a rare form with an early onset-likely a familial case. Next, who else is affected in this family? The proband's aunt was affected by both breast and ovarian cancer, with an early onset at 40 years old. Unfortunately, she passed away. The grandmother was affected as well, with breast cancer at the age of 52-slightly older than the other family members. She is still alive. From this pedigree, we can hypothesize autosomal dominant inheritance. This would mean that the proband's father is a carrier of the mutation but is not affected himself. What is the key takeaway from the analysis of this BRCA1 pedigree? If we have a BRCA1 mutation segregating in the family, we may observe lower penetrance in men. This means men can carry the mutation without being affected. Additionally, we observe phenotypic variability, with differences in the age of onset and types of cancer. In this case, some individuals have only breast cancer, while others have both breast and ovarian cancer. This variability highlights the clinical or phenotypic heterogeneity associated with BRCA1 mutations. Family Harboring BRCA2 Mutation Bassal 29 So, let's do the same exercise with a BRCA2 pedigree. Starting from there, who is the proband? The proband is a 52-year-old male. This is notable because we now have a male who is affected, which is completely different from the previous scenario. Additionally, the proband's father is also affected, having prostate cancer, and the grandmother had breast cancer with an early onset at 36 years old. She passed away at that age. In BRCA2 pedigrees, we often observe variable expressivity, with several organs potentially being affected. Prostate and pancreatic cancers, for example, are more commonly seen in male individuals. This highlights that males can also present with clinical manifestations of the condition. Another important point is the genetic and phenotypic heterogeneity associated with BRCA2. Thousands of different mutations have been identified and described as causative mutations in this gene. Unlike some genes with mutational hotspots, BRCA2 mutations are distributed along the entire length of the gene. This means that when performing genetic testing and analysis for these genes, it is necessary to sequence the entire length of the gene. Types of Mutations What kinds of mutations are associated with breast and ovarian cancer? Several types of mutations have been identified, including missense mutations, frameshifts, and nonsense mutations. Additionally, deletions in BRCA1 and BRCA2 have been described in association with this phenotype. These deletions can involve part of the gene or, in some cases, the entire gene. To detect these types of alterations, we know that sequencing alone is not always sufficient. Therefore, additional methods must be considered when creating a comprehensive diagnostic pathway for these patients. The Role of Genetic Testing Genetic testing, particularly diagnostic genetic testing, can be useful in familial cases. However, we know that familial cases are much rarer than sporadic ones. As a result, genetic testing results cover only a small proportion of familial cases, which themselves represent only a small percentage of all cases of breast and ovarian cancer. Even in familial cases, BRCA1 and BRCA2 mutations are responsible for only a fraction of cases. Additionally, some patients carry mutations in other genes that have been associated with these disorders and are currently analyzed in genetic testing. Despite the increasing number of genes associated with breast and ovarian cancer, the majority of cases remain without a positive genetic testing result. This applies to the broader context of breast and ovarian cancer as a whole, where familial genetic cases are a small fraction. In the more common sporadic form, a genetic predisposition may still exist, similar to familial cases, but environmental factors-whose roles and contributions are not yet well-characterized-likely play a significant part. This makes it challenging to determine the precise predisposition in these cases. Bassal 30 While BRCA1 and BRCA2 remain the two primary causative genes, a panel of other genes has also been associated with breast and ovarian cancer, exhibiting varying levels of penetrance (high, moderate, or low). Some of these genes are responsible for mutations in a majority of familial cases, while others show causative mutations only in a small subset of patients. Nevertheless, BRCA1 and BRCA2 remain the principal genes and are the first to be analyzed in affected cases. Moreover, the genes in this causative panel are often associated with other human disorders, such as Fanconi anemia, Lynch syndrome, and inherited gastric cancer. This reflects the genetic heterogeneity we discussed earlier, where the same gene can be implicated in different disorders. Having a clear understanding of the clinical picture and the molecular basis of a disorder allows us to define how diagnostic genetic testing should be implemented. By identifying causative mutations and considering their broader implications, we can tailor the diagnostic pathway to the needs of each patient. Diagnostic Criteria and ACMG Guidelines There are specific criteria that defined by the ACMG (American College of Medical Genetics and Genomics) that indicate the main conditions under which diagnostic testing for breast and ovarian cancer is recommended. While it is not essential to memorize these criteria, it is important to understand the conditions in which genetic testing is warrant. For example, ACMG criteria include the following scenarios: Two first-degree relatives with breast cancer diagnosed at age less than or equal to 50 years old. This suggests two first degree relatives assigned for a familial form with early onset. Three first- or second-degree relatives affected by breast cancer, regardless of the age at diagnosis. This may also indicate an inherited form, even if the onset is not early. One relative affected by breast cancer and another affected by ovarian cancer. Despite the clinical heterogeneity of these conditions, testing is recommended if two different organs are affected within the same family. One case of bilateral breast cancer, regardless of age. Although this may represent a sporadic form, the bilateral nature increases suspicion of a genetic predisposition. Two first- or second-degree relatives affected by ovarian cancer, regardless of age at diagnosis. One case of synchronous or metachronous breast and ovarian cancer. This means the cancers occurred simultaneously or at different times in the same individual. One case of male breast cancer. If a male is affected by breast cancer, possibly along with other cancers like prostate or pancreatic cancer, a BRCA2 mutation is strongly suspected. One case of female breast cancer diagnosed at or before age 13. While this is extremely rare, the early onset supports the need for genetic testing. Bassal 31 The take-home message is that when genetic counseling reveals a suspicion of inherited cancer, genetic testing should be considered, evaluated, discussed with the patient, and, if appropriate, performed. As discussed previously, in cases involving susceptibility genes, it is preferable to perform genetic testing on the affected patient first. This increases the likelihood of identifying a causative mutation. Once the mutation is detected, family segregation studies can help determine whether other family members, including healthy individuals, also carry the mutation. This approach allows for risk evaluation in relatives. Conversely, performing genetic testing in a healthy individual without a positive family history may reveal genetic variants of unknown significance, but it will not reliably determine their risk of developing the clinical phenotype. Therefore, the ACMG strongly recommends testing only when there is a suspicion of a familial form. In cases where no family history exists, genetic testing can still be performed, but its clinical utility remains limited. If you ask whether it is possible to perform predictive testing for breast and ovarian cancer, the answer is: it depends. It depends on the characterization of the individual's clinical history. For this reason, decisions about genetic testing should be made after consulting with a medical geneticist, who can explain the risks, benefits, and limitations of testing. For example, in thrombophilia, another multifactorial disorder, both genetic and environmental predispositions play a role. However, the clinical utility of testing is much clearer in healthy individuals because the interplay of risk factors is better understood. In contrast, for breast and ovarian cancer, we currently lack the knowledge to accurately calculate risk predispositions. We hope that in the future, advancements in research will enable us to improve this scenario and better assess risk. However, for now, it is best to adhere to existing recommendations and evaluate each case thoroughly with the help of a medical geneticist. Diagnostic Pathway in the Laboratory So, what is the diagnostic pathway in the diagnostic laboratory?