Correlation and Regression PDF
Document Details
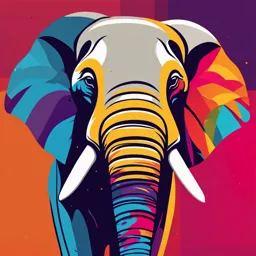
Uploaded by BriskPiccoloTrumpet
Taibah University
2024
Dr. Mansour Adam Mahmoud
Tags
Related
- Correlation and Regression PDF
- Descriptive Statistics - Correlation and Regression PDF
- Lecture -8- MSc-1 PDF
- BMS 511 Biostatistics & Statistical Analysis Chapter 4 PDF
- Research Design and Statistics Lecture 3 - Bivariate Correlation and Regression PDF
- MD115 Biostatistics Correlation and Linear Regression PDF
Summary
This document is a lecture on correlation and regression in biostatistics for pharmaceutical sciences. It covers topics including types of correlation, how to measure correlation, and regression analysis, with details like the Pearson Correlation Coefficient, types of regression, and how to interpret regression outputs. The material is specifically from Taibah University in Saudi Arabia for the 2024-1446 academic year.
Full Transcript
Correlation and Regression Biostatistics for Pharmaceutical Sciences - PHRM 103- Dr. Mansour Adam Mahmoud, PhD, CSPP Associate Professor Department of Pharmacy Practice, College of Pharmacy, Taibah University...
Correlation and Regression Biostatistics for Pharmaceutical Sciences - PHRM 103- Dr. Mansour Adam Mahmoud, PhD, CSPP Associate Professor Department of Pharmacy Practice, College of Pharmacy, Taibah University 2024-1446 1 Introduction Inferential statistics allow us to make predictions or inferences about a population based on a sample of data. Key Goals: Determine relationships between variables. Make predictions based on data trends. Real-World Examples: Relationship between hours studied and exam scores. Predicting sales based on advertising spend. 2 Correlation Definition: A statistical measure that describes the strength and direction of a relationship between two variables. Correlation is typically used for bivariate analysis (involving two variables) Types of Correlation: Positive Correlation: As one variable increases, the other also increases (e.g., height and weight). Negative Correlation: As one variable increases, the other decreases (e.g., exercise and weight loss). No Correlation: No consistent relationship between variables (e.g., shoe size and test scores) Correlation does not mean causation. 3 Correlation 4 How to Measure Correlation? Pearson Correlation Coefficient (r): measures the strength and direction of linear relationships between pairs of continuous variables. The sign of r denotes the nature of the association While the value of r denotes the strength of the association Range: -1 to +1 +1: Perfect positive correlation 0: No correlation -1: Perfect negative correlation 5 6 Regression Correlation shows a relationship, but regression quantifies it and allows predictions. Regression tells us how to draw the straight line described by the correlation The regression technique is concerned with predicting some variables by knowing others. More specifically it derives a mathematical equation that will allow us to predict one of the parameters if we know the value of the other. Example: If we know the correlation between study hours and grades, regression can help us predict grades based on study hours 7 Regression Analysis oThe process of predicting Y (dependent variable) using variable X (independent variable) oIn order to understand regression analysis fully, it’s essential to comprehend the following terms: Dependent Variable: This is the main factor that you’re trying to understand or predict. Independent Variables: These are the factors that you hypothesize have an impact on your dependent variable. 8 Regression Analysis oDraw a line through the middle of all of the data points on the chart. This line is referred to as your regression line.. oThe regression line represents the relationship between the independent variable and the dependent variable. 9 Best-fit Line ŷ = ax + b Linear regression aims to fit a slope straight line, ŷ = ax + b, to data that gives the best ε prediction of y for any value of x This will be the line that = ŷ, predicted value minimizes the distance = y i , true value between data and the fitted ε = residual error line, i.e the residuals 10 Types of Regression Simple Linear Regression: One independent variable predicts a dependent variable (continuous). Equation: y=b0+b1x+e y: Dependent variable x: Independent variable b0: Intercept b1: Slope (change in y for a one- unit change in x) called coefficient e: Error term 11 Types of Regression Multiple Linear Regression Multiple regression is used to determine the effect of a number of independent variables, x1, x2, x3, etc, on a single dependent variable, y The different x variables are combined in a linear way and each has its own regression coefficient: Multiple independent variables predict a dependent variable. Equation: y=b0+b1x1+b2x2+...+e 12 Interpreting Regression Output 1- R-squared (R2): Proportion of Variance Explained Definition: R-squared tells us how well the independent variable(s) explain the variation in the dependent variable. Range: 0 to 1 (or 0% to 100%). Closer to 1 (or 100%): The model explains most of the variation. Closer to 0 (or 0%): The model explains very little of the variation. 13 Interpreting Regression Output Example: Suppose we’re studying how the number of hours patients spend in a fitness program (independent variable, x) affects their weight loss (dependent variable, y). If the R-squared value is 0.75, it means that 75% of the weight loss variation among patients can be explained by the time spent in the fitness program. The remaining 25% is influenced by other factors (e.g., diet, age, metabolism). 14 Interpreting Regression Output 2. Coefficients: The Effect of Independent Variables: Definition: The coefficient of an independent variable indicates how much the dependent variable (y) changes for each one-unit increase in the independent variable (x). Positive Coefficient: y increases as x increases. Negative Coefficient: y decreases as x increases. 15 Interpreting Regression Output Example Let’s say the regression equation for the fitness program is: Weight Loss (kg)=2+0.8×Hours in Fitness Intercept (2): If a patient spends 0 hours in the program, they still lose 2 kg (possibly due to baseline activity). Coefficient for Hours (0.8): For every additional hour in the fitness program, a patient loses an additional 0.8 kg on average. If a patient spends 10 hours in the program: Predicted Weight Loss=2+(0.8×10)=10 kg 16 Interpreting Regression Output tells us how much of the R-squared variability is explained by R2 the model. tell us how and by how much the dependent Coefficients variable changes with the independent variable(s) 17