MD115 Biostatistics Correlation and Linear Regression PDF
Document Details
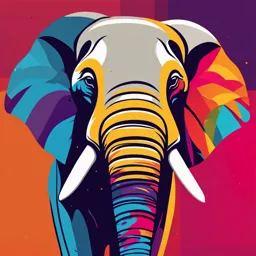
Uploaded by BreathtakingBinary924
European University Cyprus
Theodore Lytras
Tags
Summary
These lecture notes cover correlation and linear regression in biostatistics. The presentation details the aim of biostatistics, different types of analyses and examples, Pearson's correlation coefficient, and the assumptions of linear regression. It also addresses multiple linear regression, effect modification, and a reformulation of the linear model.
Full Transcript
MD115 Biostatistics: 8. Correlation between continuous variables. Linear regression. Theodore Lytras Assistant Professor of Public Health Recap: the aim of biostatistics 1. Distinguishing true effects from random error Hypothesis testing using p-values 2. Quantifying the rand...
MD115 Biostatistics: 8. Correlation between continuous variables. Linear regression. Theodore Lytras Assistant Professor of Public Health Recap: the aim of biostatistics 1. Distinguishing true effects from random error Hypothesis testing using p-values 2. Quantifying the random error around point estimates of effect Using the Standard Error ⇒ 95% Confidence Intervals Recap: the aim of biostatistics 1. Distinguishing true effects from random error Hypothesis testing using p-values 2. Quantifying the random error around point estimates of effect Using the Standard Error ⇒ 95% Confidence Intervals How this works: Calculate some quantity in a sample (point estimate) Then, under some distribution that this quantity follows: calculate its Standard Error calculate a p-value (probability of seeing such an “extreme” quantity under the null hypothesis) Recap: the aim of biostatistics 1. Distinguishing true effects from random error Hypothesis testing using p-values 2. Quantifying the random error around point estimates of effect Using the Standard Error ⇒ 95% Confidence Intervals How this works: Calculate some quantity in a sample (point estimate) Then, under some distribution that this quantity follows: calculate its Standard Error calculate a p-value (probability of seeing such an “extreme” quantity under the null hypothesis) The choice of statistical technique depends on the research question, and more specifically on the type of variables: outcome + exposure variable Different questions, different analyses: Continuous outcome, binary exposure (compar. between two means) Example: blood cholesterol levels between pre-menopausal and post-menopausal women Categorical outcome, categorical exposure Example: mortality by influenza type/subtype Continuous outcome, continuous exposure (and others!) Example: levels of diastolic blood pressure (mmHg) by Body Mass Index (kg/m2 ) Binary outcome, continuous exposure (and others!) Example: Occurrence of Chronic Obstructive Pulmonary Disease (COPD) by lifetime smoking pack-years Different questions, different analyses: Continuous outcome, binary exposure (compar. between two means) Example: blood cholesterol levels between pre-menopausal and post-menopausal women t-test / Mann-Whitney test Categorical outcome, categorical exposure Example: mortality by influenza type/subtype Chi-square / Fisher’s test Continuous outcome, continuous exposure (and others!) Example: levels of diastolic blood pressure (mmHg) by Body Mass Index (kg/m2 ) Linear regression Binary outcome, continuous exposure (and others!) Example: Occurrence of Chronic Obstructive Pulmonary Disease (COPD) by lifetime smoking pack-years Logistic regression Correlation Correlation Correlation is about the statistical relationship between two numeric variables Whether changes in one are reflected in changes to the other, and vice versa Correlation Example: association between income and happiness “Does money make you happy?” Self-reported happiness (from 1 to 10) 6 5 4 3 2 1 2,000 3,000 4,000 5,000 6,000 7,000 Annual income (€) Other similar examples we might think: Association between blood pressure and Body Mass Index Association between blood sugar control (glucosylated hemoglobin) and kidney function (Glomerular Filtration Rate) [see figure] Association between levels of two metabolites in the body... (add your own) 100 120 140 eGFR (ml/min) 80 60 40 20 0 3 4 5 6 7 8 HbA1c (%) Correlation Correlation is about the statistical relationship between two numeric variables Whether changes in one are reflected in changes to the other, and vice versa Correlation Correlation is about the statistical relationship between two numeric variables Whether changes in one are reflected in changes to the other, and vice versa Note: It is NOT about causality! Correlation does not imply causation Direction of effect? (A causes B, or B causes A ?) Common cause? (C causes A, and C also causes B) In addition: confounding Spurious relationship (coincidence – random error) Even though it can be about prediction Read this: Altman, N., Krzywinski, M. Association, correlation and causation. Nat Methods 12, 899–900 (2015). https://doi.org/10.1038/nmeth.3587 Pearson’s correlation coefficient (r) Measures the degree of linear correlation between two numeric variables X and Y ∑n (x − x)(yi − y) Is calculated as: r = √∑i=1 i n i=1 (xi − x) (yi − y) 2 2 Ranges between −1 and 1 r = 0 stands for no correlation r = 1 stands for perfect positive correlation For a set increase in X, Y always increases by the same set amount r = −1 stands for perfect negative correlation For a set increase in X, Y always decreases by the same set amount Anything in-between essentially means that Y depends not only on X, but also on other, unaccounted factors Pearson’s correlation coefficient (r) Measures the degree of linear correlation between two numeric variables X and Y ∑n In R, it’s just: (x − x)(yi − y) Is calculated as: r = √∑i=1 i cor(x,y,type="Pearson") n i=1 (xi − x) (yi − y) 2 2 Ranges between −1 and 1 r = 0 stands for no correlation r = 1 stands for perfect positive correlation For a set increase in X, Y always increases by the same set amount r = −1 stands for perfect negative correlation For a set increase in X, Y always decreases by the same set amount Anything in-between essentially means that Y depends not only on X, but also on other, unaccounted factors Pearson’s correlation coefficient (r) Ranges between −1 and 1 r = 0 stands for no correlation r = 1 stands for perfect positive correlation r = −1 stands for perfect negative correlation Pearson's r = 0.00 Pearson's r = 0.50 Pearson's r = 0.95 Pearson's r = -0.00 Pearson's r = -0.50 Pearson's r = -0.95 Pearson’s correlation coefficient (r) The coefficient is measured in a sample. Therefore this is an estimate of an underlying population coefficient! Remember: anything we calculate in a sample is a sample estimate of the respective unknown quantity in the population (population value) We use r for the sample estimate and ρ for the population value Therefore r has an associated Standard Error (SE), which depends on the sample size, and also an associated 95% Confidence Interval We can also do hypothesis testing, H0 : ρ = 0 vs H1 : ρ ̸= 0 Pearson's r = 0.50 Pearson's r = 0.50 Pearson's r = 0.50 (95% CI: -0.19 to 0.86) (95% CI: 0.34 to 0.63) (95% CI: 0.45 to 0.54) Pearson’s is not a substitute for a good scatterplot... Anscombe’s quartet All four datasets have the same correlation coefficient r !! Relaxing the assumption of linearity Pearson’s r measures the extent of a linear relationship betw. X and Y The Spearman’s rank correlation coefficient rs is a “Pearson’s on ranks”, which measures any monotonic relationship between x and Y, and is less sensitive to outliers Linear regression Linear regression Again, we are interested in whether two numeric variables X and Y have a linear relationship Again, this isn’t necessarily about causation (though it can be about prediction) However, we now consider X → Y (i.e. X affects Y) From a mathematical standpoint: X = {x1 , x2 ,... , xn } is called the independent variable Y = {y1 , y2 ,... , yn } is called the dependent variable From an epidemiological standpoint: X is the exposure and Y is the outcome Again, X and Y are unlikely to be perfectly correlated We thus need to specify a model for their association The simple linear model What is a model? A (simplified) mathematical description of reality, that we take as ground truth By estimating its parameters, we can make interesting inferences Each observation of the dependent variable yi is assumed to depend on a linear combination of the corresponding independent variable xi , plus a random error term ϵi ϵi is assumed to be normally distributed yi = β0 + β1 xi + ϵi where: ϵi ∼ N(0, σϵ ) β0 and β1 are collectively called the regression coefficients β0 is called the intercept β1 is called the slope The simple linear model yi = β0 + β1 xi + ϵi where: ϵi ∼ N(0, σϵ ) We are interested in using our sample observations (xi , yi ) to estimate β0 and β1 (especially the slope β1 ) Again, β0 and β1 are sample estimates of a corresponding population quantity Therefore they have an associated standard error We might be interested both in creating a 95% Confidence Interval for β1 and in testing the hypothesis H0 : β1 = 0 vs H1 : β1 ̸= 0 i.e. testing whether the variables are correlated or not The simple linear model yi = β0 + β1 xi + ϵi where: ϵi ∼ N(0, σϵ ) We are interested in using our sample observations (xi , yi ) to estimate β0 and β1 (especially the slope β1 ) Again, β0 and β1 are sample estimates of a corresponding population quantity Therefore they have an associated standard error We might be interested both in creating a 95% Confidence Interval for β1 and in testing the hypothesis H0 : β1 = 0 vs H1 : β1 ̸= 0 i.e. testing whether the variables are correlated or not Important note: The above model is taken as the “ground truth”, i.e. we assume a priori that it is true, and are seeking to estimate its coefficients But keep in mind: “all models are wrong...” George E.P. Box (1919 – 2013), British statistician: “All models are wrong, but some are useful” “Now it would be very remarkable if any system existing in the real world could be exactly represented by any simple model. However, cunningly chosen parsimonious models often do provide remarkably useful approximations. For example, the law PV = nRT relating pressure P, volume V and temperature T of an ”ideal” gas via a constant R is not exactly true for any real gas, but it frequently provides a useful approximation and furthermore its structure is informative since it springs from a physical view of the behavior of gas molecules. For such a model there is no need to ask the question ”Is the model true?”. If ”truth” is to be the ”whole truth” the answer must be ”No”. The only question of interest is ”Is the model illuminating and useful?”.” Simple linear regression yi = β0 + β1 xi + ϵi where: ϵi ∼ N(0, σϵ ) The standard way of estimating our regression coefficients is least ∑ 2 squares estimation, i.e. identifying the coefficients that minimize ϵi ϵi = yi − (β0 + β1 xi ) are called the residuals (observed – fitted values) The computer (R) does this for us, and provides for us a point estimate and a Standard Error for each regression coefficient 100 120 140 eGFR (ml/min) 80 60 40 Regression line 20 95% Confidence Bands Residuals 0 3 4 5 6 7 8 HbA1c (%) Interpreting the regression coefficients yi = β0 + β1 xi + ϵi where: ϵi ∼ N(0, σϵ ) The intercept β0 is the expected value of Y if X = 0 The slope β1 is the expected unit change in the dependent variable Y for every unit change in the independent variable X We can construct a 95% Confidence Interval (CI) for β1 by going ±1.96 × SE(β1 ) around its point estimate β̂1 We can also test the null hypothesis H0 : β1 = 0 by calculating |βˆ1 | β̂1 /SE(β1 ) and its associated p-value: 2 × Φ(− SE(β 1) ) (Φ is the probability function of the Standard Normal distribution: pnorm() in R) Confidence vs prediction bands 95% confidence band: expresses the uncertainty around the slope β1 95% prediction band: expresses the additional uncertainty around yi themselves, i.e. how spread out around the fitted value β0 + β1 xi each observed value yi is And how close to the expected value β0 + β1 xi each additional new observation yi is predicted to be Self-reported happiness (from 1 to 10) 6 5 4 3 2 Regression line 95% Confidence Bands 1 95% Prediction Bands 2,000 3,000 4,000 5,000 6,000 7,000 Annual income (€) Assumptions of linear regression There are four assumptions that must be met, for linear regression to be valid: Linearity Between X and the expected value (mean) of Y Homoscedasticity The variance around the expected value of Y is the same throughout the range of X Independence Observations are independent of each other Normality For any particular value of X, Y is normally distributed We can test these on the residuals of the regression (=the differences between fitted and observed values) e.g. by drawing a Q-Q plot: > qqnorm(residuals(m)) These fundamentally stem from the specification of the linear model: yi = β0 + β1 xi + ϵi , ϵi ∼ N(0, σϵ ) Multiple linear regression (or: multivariable linear regression) This whole idea extends to more than one independent variable! E.g. for K variables we have: yi = β0 + β1 x1i + β2 x2i +... + βK xKi + ϵi ϵi ∼ N(0, σϵ ) ∑ yi = β0 + βk xki + ϵi We can also incorporate non-numeric variables, e.g. binary (dichotomous) variables where xk is either 0 or 1 We can also incorporate categorical variables in the regression model, by using dummy variables For λ categories, we use λ − 1 dummies comparing each category to a reference category, e.g.: v1 v2 Normal BMI 0 0 Overweight 1 0 Obese 0 1 Multiple linear regression – example 8 Men Women FEV1 = 5 + 1.4 × Male − 0.03 × age Interpret the above regression 7 equation: Forced Vital Capacity (FVC) (l) 6 What is the meaning of the intercept (5) ? 5 What is the average FVC for a 30 year old female? 4 What is the average FVC for a 30 year old male? 3 2 20 30 40 50 60 Age (y) Multiple linear regression – example 8 Men Women FEV1 = 5 + 1.4 × Male − 0.03 × age Interpret the above regression 7 equation: Forced Vital Capacity (FVC) (l) 6 What is the meaning of the intercept (5) ? 5 What is the average FVC for a 30 year old female? 4 What is the average FVC for a 30 year old male? 3 Very important note: 2 We should not really be “extending” the regression line beyond the range of the 20 30 40 50 60 data! Age (y) Effect modification We can also add interactions between independent variables, e.g: yi = β0 + β1 x1i + β2 x2i + β12 x1i x2i + ϵi That is particularly relevant for binary variables (or the product of one binary and one numeric variable) We can thus estimate the additional unit change in the dependent variable Y if BOTH independent variables X1 and X2 are nonzero Essentially: how X2 modifies the effect of X1 and vice-versa Effect modification – example 8 Men Women FEV1 = 4.9 + 1.7 × Male − 0.03 × 7 age − 0.01 × Male × age Forced Vital Capacity (FVC) (l) 6 Interpret the above regression equation: 5 What is the meaning of the intercept (4.9) ? 4 What is the meaning of the interaction term 3 (−0.01 × Male × age) ? 2 What is the average FVC for a 50 year old male? 20 30 40 50 60 Age (y) Is there effect modification or not? To answer this question, we should do a Likelihood Ratio Test comparing the two models (with and without interaction term) H1 : FEV1 = β0 + β1 × Male + β2 × age + β3 × Male × age H0 : FEV1 = β0 + β1 × Male + β2 × age If p < 0.05 the null model (without interaction term) is rejected and we accept the alternative model (with interaction term) as having a better “fit” to the data NOTE: This is NOT the same as accepting a prior model H1 and testing whether β3 = 0... A reformulation of the linear model: ∑ So far, we got: yi = β0 + βk xki + ϵi where ϵi ∼ N(0, σϵ ) ∑ Equivalently: yi ∼ N(β0 + βk xki , σϵ ) The latter means that each observation yi follows a certain distribution (here, the normal distribution, whose parameter (in this case, the mean) depends on a linear predictor of covariates This idea generalizes to other types of outcome variables and other distributions, leading to Generalized Linear Models (GLMs) Such as logistic regression and Poisson regression A reformulation of the linear model: ∑ So far, we got: yi = β0 + βk xki + ϵi where ϵi ∼ N(0, σϵ ) ∑ Equivalently: yi ∼ N(β0 + βk xki , σϵ ) The latter means that each observation yi follows a certain distribution (here, the normal distribution, whose parameter (in this case, the mean) depends on a linear predictor of covariates This idea generalizes to other types of outcome variables and other distributions, leading to Generalized Linear Models (GLMs) Such as logistic regression and Poisson regression Next time: Next time: We will generalize the concept of regression to other types of dependent variables (outcomes) We will explain what confounding is, and how this idea (Generalized Linear Models) allows us to adjust for it Thank you!