Qualitative Data Analysis PDF
Document Details
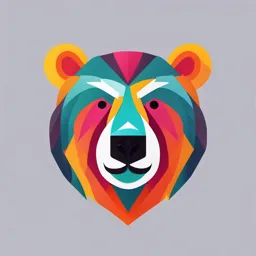
Uploaded by EntertainingCliff6883
Pamantasan ng Cabuyao
Tags
Summary
This document provides an overview of qualitative data analysis, outlining inductive and deductive approaches, steps, and examples. It explains how to immerse yourself in the data, code it, develop themes, and draw conclusions. The document also discusses the importance of being reflexive and considering your background and pre-existing knowledge within the research process.
Full Transcript
What is Qualitative data analysis QDA is based on interpretative policy to examine the symbolic and meaningful content of data. In other words, it is interpreting the qualitative data by many processes and procedures to transform them into great insights for taking dynamic decisions. The goal of qua...
What is Qualitative data analysis QDA is based on interpretative policy to examine the symbolic and meaningful content of data. In other words, it is interpreting the qualitative data by many processes and procedures to transform them into great insights for taking dynamic decisions. The goal of qualitative data analysis is to assemble or reconstruct the data in a meaningful or understandable method that is transparent and rigorous while keeping ‘true’ to the participants’ stories. There are two key approaches to analysing qualitative data – inductive analysis and deductive analysis. Inductive analysis involves coding data without trying to fit it into a pre-existing coding frame or the researcher’s analytic preconceptions. Deductive analysis is driven by theoretical interest and may provide a more detailed analysis of some aspects of the data. It tends to produce a less detailed description of the overall data. Inductive analysis is an exploratory approach to analyzing qualitative data, where patterns and themes emerge from the data itself without imposing predefined theories or hypotheses. The researcher begins with specific observations or data points and works toward identifying broader patterns, categories, and theories. Steps in Inductive Analysis: Data Familiarization: Immersing in the data by reading or reviewing it multiple times to understand its depth and context. Open Coding: Assigning initial codes to different parts of the data without any preconceived categories. Codes are labels that capture key ideas or elements in the data. Theme Development: Grouping similar codes into broader categories or themes that describe patterns in the data. Data Interpretation: Drawing conclusions based on the themes and patterns, and potentially developing a new theory or framework. Inductive analysis is an exploratory approach to analyzing qualitative data, where patterns and themes emerge from the data itself without imposing predefined theories or hypotheses. The researcher begins with specific observations or data points and works toward identifying broader patterns, categories, and theories. Example: In a study on employee satisfaction, researchers might conduct interviews with employees. They would read through the transcripts, identify codes (e.g., "support from management," "work-life balance"), and then group these codes into broader themes (e.g., "job satisfaction drivers"). From there, they might develop a theory about the factors influencing employee happiness. Deductive Analysis Deductive analysis is a structured approach to analyzing qualitative data, where the researcher begins with a predefined theory, hypothesis, or framework, and then tests it against the data. The aim is to see if the data confirms or challenges the initial assumptions. Steps in Deductive Analysis: Theory Selection: The researcher begins with a theoretical framework or hypothesis. Data Familiarization: Reviewing the data with the theoretical framework or hypotheses in mind. Coding with a Framework: Assigning codes based on pre-existing concepts or categories derived from the theory. Confirming or Disconfirming: Testing the data against the initial hypothesis or theory, noting whether the data supports or contradicts the expectations. Conclusion: Drawing conclusions based on how well the data fits the theoretical framework. Example: If a researcher wants to test Herzberg's two-factor theory of job satisfaction (hygiene factors and motivators), they might analyze employee interview data deductively. They would code the data according to the categories in the theory (e.g., hygiene factors like salary, motivators like recognition) and assess whether the data fits these categories. If a researcher aims to test Herzberg’s two-factor theory of job satisfaction, they would begin by using a deductive analysis approach, focusing on two key categories from the theory: hygiene factors and motivators. (1) Coding Based on Herzberg's Categories: The researcher would first create a codebook based on the existing framework of Herzberg’s theory. They would categorize responses under hygiene factors (which, according to the theory, prevent dissatisfaction but do not promote satisfaction) and motivators (which enhance job satisfaction). 2. Hygiene Factors: As per Herzberg’s theory, hygiene factors include elements such as salary, work conditions, job security, company policies, and supervision. The researcher would code employee comments that mention these aspects, such as "I’m happy with my salary, but the office environment is stressful" or "I have good job security, but the company’s policies are too rigid." By coding responses under hygiene factors, the researcher checks whether these factors align with Herzberg’s claim that improving hygiene factors reduces dissatisfaction but does not necessarily increase overall job satisfaction. 3.Motivators: Motivators include factors like recognition, achievement, responsibility, growth, and work itself. Comments such as "I feel recognized when my manager appreciates my hard work" or "I enjoy taking on new responsibilities because it makes my job fulfilling" would be coded under motivators. The researcher would then assess whether the presence of motivators leads to increased job satisfaction, as predicted by Herzberg’s theory. 4. Assessing Fit to the Theory: After coding the data, the researcher would analyze whether employee satisfaction aligns with Herzberg’s prediction. For instance, they would check if employees who reported positive hygiene factors (e.g., high salary) but lacked motivators (e.g., no recognition) still experienced dissatisfaction. They would also examine whether employees who reported motivators (e.g., a sense of achievement or growth opportunities) felt higher satisfaction, even when hygiene factors were not fully addressed. 5.Conclusion: 1. Based on the analysis, the researcher could determine if the data fits Herzberg’s two-factor theory. If the theory holds true, the findings should show that hygiene factors help reduce dissatisfaction, while motivators are the primary drivers of employee satisfaction. By using this deductive approach, the researcher tests Herzberg’s predefined categories directly, comparing the employee responses to the expected outcomes outlined in the theory. When to Use Inductive vs. Deductive Analysis Inductive Analysis is best when: You do not have a pre-existing theory or framework. You want to explore new ideas or generate fresh insights. The goal is to let the data speak for itself. Deductive Analysis is best when: You have a specific hypothesis to test. You want to apply a known theory or framework to a new dataset. The research question is structured around validating or challenging an existing theory. What purpose does the qualitative data analysis plan perform? Unlike Quantitative data analysis, which is more of numbers and statistics, qualitative analysis is an analysis of subjective and non-numerical qualitative data. Hence it performs many functions including: *Organizing data · Interpreting data · Identifying patterns · Forming the basis for informed and verifiable conclusions · Ties research objectives to data After knowing the above fundamentals of Qualitative data analysis, it is time to choose the right data analysis plan. The plans can be selected for specific research design and can also be applied for a variety of research designs. Thematic analysis is a technique for finding, examining, classifying, and reporting themes in data collection. It involves identifying codes or units of analysis that emerge from the data. Thematic analysis is the most common form of qualitative data analysis TA is a flexible and non-research design specific data analysis plan. It generates themes from interview data and is useful in case studies, phenomenology, and generic qualitative and narrative analysis, among others. If you are a novice researcher, looking for an easy to follow analysis plan and rigorous enough to generate meaningful findings, TA is the right choice. Thematic analysis An example is the study by Danielson et al. 2015, which used thematic analysis to identify common concerns facing pharmacy experiential education (EE) programs.80 The themes identified were site capacity, workload/financial support, quality assurance, preceptor development, preceptor stipends, assessment, onboarding, and support/recognition from the administration.80 When to Use: When you want to identify, analyze, and report patterns (themes) within your data. When your research questions aim to understand commonalities and differences across data. When you need a flexible approach that can be applied across a range of theoretical frameworks. Characteristics: Involves coding data and identifying themes. Can be used for both inductive (data-driven) and deductive (theory-driven) analysis. Example: Analyzing interview transcripts to identify themes related to coping strategies for anxiety among college students. TA ANALYSIS Braun and Clarke (6 steps- next slide) Reflexive Thematic Analysis: Braun and Clarke emphasize a more flexible, reflexive approach, allowing the researcher to actively engage in the process of theme generation rather than passively reporting pre-existing themes in the data. Inductive vs. Deductive Approaches: Thematic analysis can be conducted in an inductive (data- driven) or deductive (theory-driven) manner, depending on the research question and goals. Flexibility: One of the method’s strengths is its flexibility. It is not tied to any particular theoretical framework and can be used with a range of research paradigms, from positivist to interpretivist. Application of TA- braun and Clarke Thematic analysis An example is the study by Danielson et al. 2015, which used thematic analysis to identify common concerns facing pharmacy experiential education (EE) programs. [refers to the task of recognizing and listing the typical challenges or issues that pharmacy experiential education programs encounter. Experiential education in pharmacy involves practical, hands-on training in real-world settings (e.g., internships, clinical placements, or practice-based learning)] Application of Braun and Clarke's Thematic Analysis: (1) Familiarization with the Data: 1. In this step, the researcher would immerse [reading,re-reading,listening carefully (audio/video data),developing “a feel” for the data, ] themselves in the data from the study by Danielson et al. (e.g., transcripts from interviews with educators, surveys, or focus groups). 2. Re-reading the data to get a comprehensive understanding of the participants' perspectives on concerns related to pharmacy experiential education. 3. During this phase, the researcher may take notes or highlight initial thoughts and patterns that seem important. 2. Generating Initial Codes: The researcher would then go through the data and systematically identify significant elements by coding specific segments. For instance, if participants mentioned “lack of clinical placements” as a major challenge, this would be coded as “clinical placement issues.” Some initial codes in this case might include: Resource limitations (e.g., insufficient staffing, funding) Variability in student preparedness Communication challenges between institutions and sites Assessment difficulties (e.g., lack of standardized evaluation tools) 3. Searching for Themes: In this phase, the researcher would begin to group related codes to form potential themes. Codes like “insufficient staffing” and “funding issues” might be grouped under a broader theme like resource constraints. The themes might include: Resource Constraints: Discussing challenges around staffing, funding, and clinical placements. Student Preparedness: Concerns related to variability in the knowledge and skills of students entering experiential programs. Communication Gaps: Issues in coordination between educational institutions and experiential education sites. Evaluation and Assessment Challenges: Problems with evaluating student performance, especially with the lack of consistent or standardized evaluation metrics. 4. Reviewing Themes: The researcher would then review the preliminary themes to ensure they accurately represent the data. For example, if the theme communication gaps does not fully capture the variation issues around faculty-student relationships, it may need to be split or refined to better reflect the data. The data is examined both in relation to each theme and the overall dataset. The themes should be cohesive, but distinct from one another. 5. Defining and Naming Themes: At this stage, the researcher would define the specific themes and describe the essence of each one. FOR EXAMPLE: Resource Constraints: This theme encapsulates concerns about limited resources—such as staffing shortages and financial limitations—hindering the expansion and quality of experiential programs. Student Preparedness: Variations in the academic background and clinical readiness of students were noted as a recurring concern, affecting the success of EE programs. Communication Gaps: This theme reflects the breakdowns in communication between academic institutions, preceptors, and EE sites, leading to misunderstandings or misalignment in program expectations. Evaluation and Assessment Challenges: The lack of consistent methods to assess students’ performance was a major concern, with some participants suggesting the need for standardized tools. 6. Producing the Report: the researcher would write a detailed analysis of the themes, linking them back to the research question (i.e., the concerns facing pharmacy EE programs) and providing quotes or examples from the data to illustrate each theme. The report would highlight key findings and possibly suggest recommendations, such as improving communication channels or creating more standardized assessment tools for students in experiential education programs. 2. Interpretative Phenomenological Analysis (IPA) For the phenomenological research study, IPA is the right choice. It brings out the core of the phenomenon under review. Other plans like Moustakas’ Modified Van Kaam, Colaizzi, Giorgi, and Heuristic analysis also help to study phenomenological research. Even TA can be used, and the ultimate aim and focus should be to generate meaningful results. What Interpretive Phenomenological Analysis (IPA) is NOT? While IPA focuses on the lived experiences of individuals, it is not concerned with the more mundane aspects of everyday life. Instead, IPA explores transformative or emotionally charged experiences that can often leave a lasting impact – “a mark” – on the individual's life. Focuses on the subjective experience and meaning-making process of individuals following significant life events, rather than on routine experiences Focuses on the subjective experience and meaning-making process of individuals following significant life events, rather than on routine experiences Routine vs. Significant Experience 1. Routine Experience: 1. The initial example describes the experience of driving down the same street repeatedly. While it is an experience, it is largely unremarkable —you follow the same path, encountering nothing out of the ordinary. Such experiences are often taken for granted and do not prompt deeper reflection or change in behavior. HOWEVER, IF Significant Experience: In contrast, witnessing a traumatic event (like a cyclist getting hit) transforms an ordinary drive into a profoundly impactful experience. This event is jarring(shocking) and emotionally charged, leading to a strong reaction. It disrupts your routine and prompts a reassessment of that experience, resulting in a change in behavior (avoiding that street in the future). Focus of IPA Research Personal Sense-Making Process: IPA researchers are interested in the aftereffects of such significant experiences. They do not focus on the drive itself, which is routine and predictable. Instead, they explore how individuals make sense of the event, how it affects their thoughts, feelings, and behaviors moving forward. Exploring Personal Meaning: If someone decides to avoid that street after witnessing the accident, IPA researchers would investigate the underlying reasons for this behavioral change. They would seek to understand the emotional, cognitive, and contextual factors that influence the person's reaction to the traumatic event. This could include feelings of fear, anxiety, guilt, or a reevaluation of safety. Interpretive Phenomenological Analysis (IPA) is well-suited for exploring a variety of significant life events and experiences in psychology. Loss of a Loved One Event: The death of a family member or close friend. Focus: Researchers could explore how individuals process grief, the impact of loss on their daily lives, and how they find meaning or cope with the absence. Chronic Illness Diagnosis Event: Receiving a diagnosis of a chronic or terminal illness. Focus: IPA can help understand how individuals come to terms with their diagnosis, their emotional responses, and changes in identity or lifestyle. Traumatic Experience Event: Experiencing or witnessing a traumatic event, such as violence, accidents, or natural disasters. Focus: Researchers might investigate how trauma affects mental health, coping strategies, and changes in behavior or perception of safety. Significant Life Transitions Event: Transitioning to parenthood, retirement, or moving to a new country. Focus: IPA can uncover how individuals navigate these changes, including their feelings of excitement, anxiety, or identity shifts. By focusing on how individuals interpret and make sense of their experiences, IPA can reveal deep insights into personal meaning, coping strategies, and the emotional impact of these events on individuals' lives. GROUNDED THEORY Unlike TA and IPA, the grounded theory uses specific data analysis plan. It requires you to use the analyzed data concurrently and applying a constant comparison method of analysis. It is because of its utilization of theoretical saturation. It goes beyond adding to the existing knowledge and developing a new theory about a phenomenon grounded on data. The data analysis plan used in grounded theory was primarily developed by Barney Glaser and Anselm Strauss in the 1960s. They introduced grounded theory as a qualitative research methodology in their seminal work, The Discovery of Grounded Theory (1967). HELPFUL VIDEO: TITLE: USING THE KJ METHOD / AQUENT GYMNASIUM https://www.youtube.com/watch?v=PwuPv7JwzCA Individual Brainstorming: Each person silently writes down their ideas, with each idea on a separate note. This allows for independent thinking without influence from others’ ideas. 2. Sharing Ideas: Once everyone has written down their ideas, they take turns sharing them and placing them in a visible space (like a board or wall) where the group can see all contributions. 3. Grouping or Clustering: Together, the team organizes similar ideas into clusters or groups based on common themes or concepts. Each group is then given a concise label—a word or phrase that captures its core meaning. This step helps to reveal patterns and themes among the ideas. 4. Voting: In the final step, team members vote on the ideas they find most important or relevant. Each person is given a set number of votes (sometimes represented as dots), which they place on their preferred concepts. The ideas or groups with the most votes are prioritized. Content analysis: is a method of unobtrusively investigating vast volumes of textual material to detect trends and patterns in words used, their frequency, their connections, and the structures and discourses of communication.71 It transforms qualitative input into quantitative data by quantifying words, messages or concepts and analyses the relationships between the concepts. The goal of content analysis is to explain the features of the document’s content by looking at who says what to whom and to what effect.72 Content analysis requires that the text be broken down into manageable codes for analysis. The analysis is conducted in the following stages, decontextualization, recontextualization, categorisation and compilation. Abroms et al. 2011 used content analysis to analyse the content of 47 iPhone applications (apps) for smoking cessation and their adherence to the U.S. Public Health Service’s 2008 Clinical Practice Guidelines for Treating Tobacco Use and Dependence.74 The study found that apps identified for smoking cessation had low levels of adherence to key guidelines in the index. 74 Content analysis: When to Use: When you want to quantify and analyze the presence, meanings, and relationships of certain words, themes, or concepts within qualitative data. When dealing with large volumes of text data. When you aim to systematically and objectively describe the content of the data. Characteristics: Involves coding data into categories and quantifying occurrences. Can be used for both manifest (explicit) and latent (underlying) content analysis. Example: Analyzing student essays to quantify and categorize references to stress and anxiety. Example: Analyzing student essays to quantify and categorize references to stress and anxiety. 1. Decontextualization: This is the initial phase where you immerse yourself in the data and identify units of meaning. Process: Read through each student essay carefully to identify all references to stress and anxiety. These references could be explicit mentions of the words "stress" or "anxiety" or implicit references (e.g., "I feel overwhelmed" or "I can't sleep because of exams"). Highlight or underline every segment related to stress and anxiety. These are the meaning units—phrases or sentences directly addressing stress, anxiety, their causes, effects, and coping strategies. Keep the original context in mind but extract these units from the full essay for further analysis. Example: Analyzing student essays to quantify and categorize references to stress and anxiety. Example of Meaning Units: "I feel stressed when I have too much homework." "My anxiety gets worse before exams." "I get headaches because of the pressure to succeed." 2. Recontextualization: This stage involves returning to the original context to ensure that the extracted meaning units capture the essence of the essays without losing context. Process: Go back to the entire essay to confirm that the highlighted meaning units accurately represent the students’ experiences of stress and anxiety. Check if any references to stress and anxiety were missed in the initial extraction. If new references are found, add them to the decontextualized list. Make sure that the extracted meaning units are not taken out of context and still represent the student’s intended message. Example: Ensure that the phrase "I feel stressed when I have too much homework" is understood as academic-related stress, and "I get headaches" is linked to physical effects of stress. 3. Categorization: At this stage, the decontextualized meaning units are grouped into broader categories that reflect common themes or patterns in how students talk about stress and anxiety. Process: Develop a coding scheme based on the different aspects of stress and anxiety. Categorize the meaning units into the following categories: 1. Causes of Stress/Anxiety: Academic stress (e.g., homework, exams) Social stress (e.g., peer pressure, social interactions) Personal stress (e.g., family issues, self-expectations) 2. Effects of Stress/Anxiety: ▪ Physical effects (e.g., headaches, fatigue) ▪ Emotional effects (e.g., feeling sad, frustrated) ▪ Cognitive effects (e.g., lack of concentration, overthinking) 3. Coping Mechanisms: ▪ Healthy coping (e.g., talking to friends, time management) ▪ Unhealthy coping (e.g., procrastination, avoidance) 4. Intensity of Stress/Anxiety: ▪ Mild (occasional worry or stress) ▪ Moderate (frequent stress, impacts mood) ▪ Severe (overwhelming anxiety, daily disruptions 4. Compilation: This is the final stage where you compile and analyze the data, interpreting the results and identifying patterns or trends across the categorized meaning units. Process: Quantify the frequency of each category based on how often they appear in the student essays. For example: Academic Stress: 45 references Social Stress: 12 references Physical Effects: 25 references Healthy Coping: 18 references Severe Anxiety: 10 references DISCOURSE ANALYSIS investigates language in use instead of psychological factors such as attitudes, memories, or emotions. It involves examining how language—spoken, written, or symbolic—creates meaning and reflects social structures and practices. Language is studied in discourse analysis in terms of construction and function and is viewed as a tool for producing reality. Thus, discourse analysis investigates how particular concerns are produced in people’s narratives and the variety in these accounts to examine the link between language and social reality The article by Brooks et al. 2019, utilised discourse analyses as one of the analytical strategies to analyse the relationships and relationality with companion animals as therapeutic agents in the context of people’s wider social networks. DISCOURSE ANALYSIS When to Use: When you want to study language use and its role in social practices. When exploring how language constructs social realities and power dynamics. When interested in the context and function of language in communication. Characteristics: Involves examining language, text, and interaction in detail. Focuses on the social and cultural contexts of language use. Example: Analyzing classroom discussions to understand how students talk about and construct the concept of anxiety. DISCOURSE ANALYSIS Example: Analyzing classroom discussions to understand how students talk about and construct the concept of anxiety.( here’s a Sample dataset of classroom discussion snippets) Sample Data: Classroom Discussion Snippets on Anxiety Student A: "Anxiety, I think, is just feeling nervous about what’s coming. Like, when you have an exam, it’s that constant worry that you might fail." Student B: "Yeah, but it's more than that too. Sometimes it feels like you can't breathe, like you're trapped in a cycle of what-ifs." Student C: "My mom always says anxiety is something we create in our heads. But for me, it feels real. Like, my body reacts, you know?" Student D: "I feel like anxiety is everywhere now. Social media makes it worse. Everyone's comparing themselves, and it just builds up." Student E: "But is anxiety always bad? Like, maybe some anxiety helps us work harder or stay aware of things?" Teacher: "Interesting points! So, what do you think makes anxiety helpful or harmful?" five potential themes: 1. Anxiety as Anticipation and Worry Student A views anxiety as a form of anticipation and worry tied to specific outcomes, like exams. Anxiety, in this context, is seen as a response to potential failure and is connected to the fear of future events. 2. Anxiety as a Physical and Emotional Experience Student B and Student C emphasize anxiety's physical manifestations, describing sensations like difficulty breathing and bodily reactions. They highlight that anxiety isn’t just a mental construct but is felt physically and impacts the body. Social Media and Modern Pressures Student D connects anxiety to social media, suggesting that online comparisons heighten anxiety. This highlights the perception that contemporary life and digital presence may intensify feelings of anxiety through constant comparison and self-assessment. Anxiety as Self-Constructed vs. Real Student C contrasts a view of anxiety as “created in our heads” (something self- imposed) with their personal experience of its genuine physical effects. This theme reflects the struggle to reconcile societal or family beliefs about anxiety as self-made with the reality of how it is experienced individually. 5. Anxiety as Potentially Beneficial Student E raises the idea that anxiety could have positive effects, suggesting that a certain level of anxiety might be functional by motivating individuals to perform better or stay alert. This theme explores the notion that anxiety might have adaptive purposes, challenging the view of it as solely negative.. Acknowledge Your Role Explanation: A researcher is not a neutral observer; their experiences, beliefs, and assumptions shape the research process. Being reflexive means acknowledging how your identity and standpoint might affect data collection and interpretation. Example: A psychologist studying gender roles in workplace dynamics may realize their own gender and experiences of discrimination influence how they interpret participants’ stories. For instance, a female researcher might relate strongly to accounts of bias, which could lead to a deeper probing in certain interviews. 2. Consider Your Background Explanation: A researcher’s background—education, race, gender, and affiliations—can influence interactions with participants and interpretations of data. Example: A researcher with a Western academic background conducting a study in an indigenous Filipino community might recognize how their education frames concepts like "individual success" differently from how the community values "collective harmony." Being reflexive, the researcher actively learns about and incorporates local cultural perspectives. 3. Consider Pre-existing Knowledge Explanation: Researchers must critically evaluate how their prior knowledge or learning during the study shapes their approach. Example: A graduate student conducting research on mental health interventions might have a bias toward cognitive-behavioral therapy (CBT) due to academic training. Reflexivity requires acknowledging this preference and remaining open to participants’ experiences with alternative approaches, like mindfulness or traditional healing practices. 4. Consider the Perspectives of Others Explanation: Understanding the participants' and stakeholders' diverse perspectives enriches the study and mitigates the researcher’s biases. Example: A researcher studying parenting styles in multicultural families recognizes that their concept of "authoritative parenting" might not align with participants from collectivist cultures who prioritize respect for elders and family hierarchy. Reflexivity involves seeking to understand and respect these different perspectives. 5. Keep a Reflexive Journal Explanation: A reflexive journal is a tool for recording thoughts, reflections, and decision- making processes during the research. It provides a space for critical analysis of the researcher’s influence. Example: While analyzing interview data, a researcher logs in their journal: “I felt drawn to highlight Participant A’s emphasis on independence because it resonates with my personal values. I must ensure I don’t overlook other participants’ values of interdependence.” 6. Communicate Your Reflexivity Explanation: Researchers should openly share their reflections, biases, and decisions in publications to enhance the study’s trustworthiness. Example: In a study on body image, the researcher writes in their methodology section: “As a researcher with a history of body image issues, I was particularly empathetic toward participants with similar experiences. To counteract this potential bias, I sought peer debriefing and participant feedback to ensure balanced interpretations.” 7. Integrate Reflexivity into Team Dynamics Explanation: Reflexivity is not just an individual exercise; it should be practiced collectively within the research team. Example: A team studying refugee experiences discusses how their diverse ethnic and professional backgrounds influence their interpretations. For example, a sociologist on the team may focus on structural inequalities, while a psychologist may emphasize individual resilience. These discussions ensure a comprehensive approach. Imagine conducting research on the experiences of LGBTQ+ individuals in conservative communities. Reflexivity involves: Acknowledging how your own sexual orientation and beliefs influence the research. Being mindful of cultural and religious sensitivities within the community. Recognizing how participants’ stories might challenge or reinforce your assumptions. Keeping a journal to document these reflections. Explaining in your publication how your identity shaped the research process and your efforts to mitigate bias.