Sampling Distributions PDF
Document Details
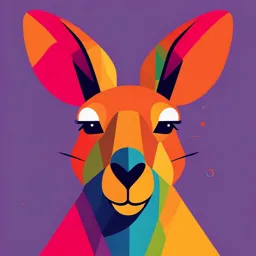
Uploaded by SelfDeterminationTroll168
2024
Dr. Ram Shanmugam
Tags
Related
Summary
This PowerPoint presentation covers the fundamentals of sampling distributions in statistics. It explains the concepts of individuals, variables, sampling, and probability theory. The presentation also touches on different sampling methods and analyzing data.
Full Transcript
What is Chapter 1 statistics? Data entries What is Sampling? Sampling is the process by which a researcher selects one or more cases out of some larger Population grouping for study....
What is Chapter 1 statistics? Data entries What is Sampling? Sampling is the process by which a researcher selects one or more cases out of some larger Population grouping for study. Sample 12/11/2024 Dr. Ram Shanmugam 4 The Sample A sample consists of A representative one or more sample accurately elements or cases reflects the selected from some distribution of larger grouping or relevant variables in population. the target population. 12/11/2024 Dr. Ram Shanmugam 5 Probability Theory and Sampling Distributions Probability theory focuses on determining the likelihood or probability that certain events will occur. A sampling distribution is a distribution of sample statistics. When we draw a random sample, the most likely outcome is a representative sample or one that is very close to representative. 12/11/2024 Dr. Ram Shanmugam 6 Sampling Distribution of Variable “Age”: 100 Samples N = 20 vs N = 200 Histogram of 100 Samples Size =20 From Population Size = 2000 Minimum 39.850 20 Maximum 50.550 Frequency 10 Q1 = 43.088 0 40 45 50 Population Mean = 45.2 Q3 = 46.762 Histogram of 100 Samples, Size =200 From Population Size = 2000 Minimum 43.290 20 Maximum 47.440 Frequency 10 Q1 = 44.749 0 Q3 = 45.613 43.4 43.8 44.2 44.6 45.0 45.4 45.8 46.2 46.6 47.0 47.4 Population Mean = 45.2 12/11/2024 Dr. Ram Shanmugam 7 Random Sampling Simple random sampling Systematic Sampling Example (with or without replacement) 257 258 Hildebrad Hilgren Brent Evelyn 259 Hill albert (SRS): each element in 260 Hill Arnold the population has an 261 Hill Darrell equal probability of Every 262 263 Hill Hill Eugene Kathleen 264 Hill Paul inclusion in the sample. 5th case 265 Hill Thomas 266 Hill William Systematic sampling: 267 268 Hillman Hines Frank Arnold variation on simple 269 270 Hirst Hoard Steven Larry random sampling 271 Hockings Mary 272 Hutchison Chad involves taking every kth element listed in a sampling frame. 12/11/2024 Dr. Ram Shanmugam 8 Stratifi ed Random Sampling Stratified sampling involves dividing the population into smaller subgroups, called strata, and then drawing separate random or systematic samples from each of the strata. 12/11/2024 Dr. Ram Shanmugam 9 Other sampling Simple random unistic sampling sampling Snowball Systematic sampling sampling Purposive Stratified random sampling sampling Quota sampling Cluster sampling Convenience/ accidental/opport 12/11/2024 Dr. Ram Shanmugam 10 How to collect information? Sources are: primary or recording secondary Using questionnaires: Three principles: Questionnaire is a collection relevant, of questions which are purposeful, responded face to face, by enhance validity proxy, by mail, by internet Questions are closed-ended Manners of finding (works in or open-ended qualitative studies): looking, watching, listening, reading, 12/11/2024 Dr. Ram Shanmugam 11 How Large a Sample? How many cases are needed for the research hypotheses? Precision: how much error can we accept? Population homogeneity: the more variability in the population to be sampled the larger the sample required. Sampling fraction: the number of elements in the sample relative to the number of elements in the population: (1- n/N) Sampling Technique 12/11/2024 Dr. Ram Shanmugam 12 Sampling Fraction Adjustment n’ = adjusted sample size n = estimated sample size n n [1 (n N )] ignoring the sampling fraction N = population size 12/11/2024 DR. RAM SHANMUGAM 13 Non-probability Sampling Types Availability sampling (convenience or accidental sampling): Snowball sampling (interactive sampling): rely on interaction of persons to generate sample. Quota sampling Purposive (or judgmental) sampling 12/11/2024 Dr. Ram Shanmugam 14 Spurious Relationship chool hS Person Reads Hi g Person than Total Sample Report Les s Quits Yes No Smoking 200 135 Yes -50% -27% 200 365 No -50% -73% 400 500 Totals -100% -100% Controlling for High Scho o l or M education, ore reading the report has no effect on quitting. DR. RAM SHANMUGAM 15 12/11/2024 Displaying graphs The class objectives today are: Picturing Distributions with Graphs with Individuals and variables Two types of data: categorical and quantitative Ways to chart categorical data: bar graphs and pie charts Ways to chart quantitative data: histograms, dot plots and stem plots Interpreting histograms Time plots Individuals and variables Individuals are the objects described by a set of data. Individuals may be people, animals, or things. Freshmen, 6-week-old babies, golden retrievers, fi elds of corn, cells A variable is any characteristic of an individual. A variable can take different values for different individuals. Age, gender, blood pressure, blood type, leaf length, fl ower color Two types of variables A variable can be either quantitative Something that can be counted or measured for each individual. We can then report the average of all individuals measured. Age ( in years), blood pressure ( in mm Hg ), leaf length ( in cm) or categorical Something that falls into one of several categories. We can then report the count or proportion of individuals in each category. Gender ( male, female), blood type ( A, B, AB, O), fl ower color ( white, yellow, red ) How do you decide if a variable is categorical or quantitative? Ask: What are the n individuals examined (in the sample or population)? What is being recorded about those n individuals? Is that a number ( quantitative) or a statement ( categorical)? Categorical Quantitative Each individual is Each individual is assigned to one of attributed a several categories numerical value Individuals in sample DIAGNOSIS AGE AT DEATH Patient A Heart disease 56 Patient B Stroke 70 Patient C Stroke 75 Patient D Lung cancer 60 Patient E Heart disease 80 Patient F Accident 73 Patient G Diabetes 69 Ways to chart categorical data When a variable is categorical, the data in the graph can be ordered any way we want (alphabetical, by increasing value, by year, by personal preference, etc.). Most common ways to graph categorical data: Bar graphs Each category is represented by a bar that represents the counts of individuals in that category or their relative frequency (percent of all categories shown). Pie charts Each category is represented by a slice of the whole pie that represents its relative frequency. Peculiarity: The slices must represent the parts of one coherent whole. Example: Top 10 causes of death in the United States, 2001 Percent of Percent of Rank Causes of death Counts top total 10s deaths 1 Heart disease 700,142 37% 29% 2 Cancer 553,768 29% 23% 3 Cerebrovascular 163,538 9% 7% 4 Chronic respiratory 123,013 6% 5% 5 Accidents 101,537 5% 4% 6 Diabetes mellitus 71,372 4% 3% 7 Flu and pneumonia 62,034 3% 3% 8 Alzheimer’s disease 53,852 3% 2% 9 Kidney disorders 39,480 2% 2% 10 Septicemia 32,238 2% 1% All other causes 629,967 26% For each individual who died in the United States in 2001, we record what was the cause of death. The table above is a summary of that information. Bar graph Here the bar’s height shows the count of individuals for that particular category. 800 700 600 Top 10 causes of death in the U.S., 2001 Counts (x1000) 500 400 The number of individuals who died of 300 an accident in 2001 is 200 approximately 100 100,000. 0 r e ia ia s rs rs y s us la or nt se as on em e ce lit cu de t rd ea se ra el an m as ic so ci pi m di is eu pt C Ac v di s d s ro Se 's pn re te rt ey er eb ea ic be & m dn er on H ia u ei Ki C Fl hr D zh C Al Counts (x1000) H ea rt d 100 200 300 400 500 600 700 800 0 is e as es C an C ce er rs Counts (x1000) eb ro C v as hr cu 0 100 200 300 400 500 600 700 800 Ac on la Al ci ic r zh de re ei m nt s s pi er ra 's to di ry se as e Ac ci C D de an ia nt C ce be s er rs te eb s ro m va Fl el C sc u litu hr on ul & s ar pn ic re Al eu sp ira zh m D to ei m on ia ry ia be er te 's s m di se Fl el Ki u lit dn as & us pn ey e Easy to analyze eu di m so on rd H Top 10 causes of death in the U.S., 2001 ia Much less useful ea er rt s Sorted alphabetically di Se Bar graph sorted by rank se pt Ki as ic dn es em ey di ia so rd er s Se pt ic em ia Pie chart Each slice represents a piece of one whole. The size of a slice depends on what percent of the whole this category represents. Percent of people dying from top 10 causes of death in the U.S., 2001 Make sure all percents add up to 100. Percent of deaths from top 10 causes Make sure the labels match the data. Percent of deaths from all causes Common ways to chart quantitative Histograms data This is a summary graph for a single variable. Histograms are useful to understand the pattern of variability in the data, especially for large data sets. Dot plots and stem plots These are graphs for a the raw data. They are useful to describe the pattern of variability in the data, especially for small data sets. Line graphs: time plots Use them when there is a meaningful sequence, like time. The line connecting the points helps emphasize any change over time. Other graphs to display numerical summaries (see chapter 2) Histograms The range of values that a variable can take is divided into equal-size intervals. The histogram shows the number of individual data points that fall in each interval. The first column represents all states with a percent Hispanic in their population between 0% and 4.99%. The height of the column shows how many states (27) have a percent Hispanic in this range. The last column represents all states with a percent Hispanic between 40% and 44.99%. There is only one such state: New Mexico, at 42.1% Hispanic. How to create a histogram It is an iterative process—try and try again. What bin size should you use? Not too many bins with either 0 or 1 counts Not overly summarized that you lose all the information Not so detailed that it is no longer summary Rule of thumb: Start with 5 to10 bins. Look at the distribution and refine your bins. (There isn’t a unique or “perfect” solution.) Same data set Not summarized enough Too summarized Interpreting histograms When describing a quantitative variable, we look for the overall pattern and for striking deviations from that pattern. We can describe the overall pattern of a histogram by its shape, center, and spread. Histogram with a line connecting each Histogram with a smoothed curve column too detailed highlighting the overall pattern of the distribution Most common distribution shapes A symmetric distribution the right and left sides of the histogram are approximately mirror images of each other Left skewed Right skewed the left side extends the right side (side with larger much farther out than values) extends much farther out the right side. than the left side. Symmetri c Skewed to the right Complex, bimodal distribution Not all distributions have a simple shape (especially with few observations). An important kind of deviation is an outlier. Outliers are Outliers observations that lie outside the overall pattern of a distribution. Always look for outliers and try to explain them. Fairly symmetric but 2 states clearly don’t belong to the main trend Alaska and Florida have unusual percents of elderly in their population. Alaska Florida A large gap in the distribution is typically a sign of an outlier. Stem plots How to make a stem plot: STEM LEAVES 1)Separate each observation into a stem, consisting of all but the fi nal (rightmost) digit, and a leaf, which is that remaining fi nal digit. Stems may have as many digits as needed, but each leaf contains only a single digit. 2)Write the stems in a vertical column with the smallest value at the top, and draw a vertical line at the right of this column. 3)Write each leaf in the row to the right of its stem, in increasing order out from the stem. Original data: 9, 9, 22, 32, 33, 39, 39, 42, 49, 52, 58, 70 State Percent State Percent Percent of Hispanic residents Alabama 1.5 Maine 0.7 Alaska 4.1 WestVirginia 0.7 Arizona 25.3 Vermont 0.9 Arkansas California 2.8 32.4 NorthDakota Mississippi 1.2 1.3 in each of the 50 states Colorado 17.1 SouthDakota 1.4 Connecticut 9.4 Alabama 1.5 Delaware 4.8 Kentucky 1.5 Florida 16.8 NewHampshire 1.7 Georgia 5.3 Ohio 1.9 Hawaii 7.2 Montana 2 Idaho 7.9 Tennessee 2 Illinois 10.7 Missouri 2.1 Indiana 3.5 Louisiana 2.4 Iowa 2.8 SouthCarolina 2.4 Kansas 7 Arkansas 2.8 Kentucky 1.5 Iowa 2.8 Louisiana 2.4 Minnesota 2.9 Maine 0.7 Pennsylvania 3.2 Maryland 4.3 Michigan 3.3 Massachusetts 6.8 Indiana 3.5 Michigan 3.3 Wisconsin 3.6 Minnesota 2.9 Alaska 4.1 Mississippi Missouri 1.3 2.1 Step 1: Maryland NorthCarolina 4.3 4.7 Step 2: Montana 2 Virginia 4.7 Nebraska 5.5 Delaware 4.8 Nevada 19.7 Oklahoma 5.2 NewHampshire 1.7 Georgia 5.3 NewJ ersey NewMexico 13.3 42.1 Sort the Nebraska Wyoming 5.5 6.4 Assign the NewYork NorthCarolina 15.1 4.7 data Massachusetts Kansas 6.8 7 values to stems and NorthDakota 1.2 Hawaii 7.2 Ohio 1.9 Washington 7.2 leaves Oklahoma 5.2 Idaho 7.9 Oregon 8 Oregon 8 Pennsylvania 3.2 RhodeIsland 8.7 RhodeIsland 8.7 Utah 9 SouthCarolina 2.4 Connecticut 9.4 SouthDakota 1.4 Illinois 10.7 Tennessee 2 NewJ ersey 13.3 Texas 32 NewYork 15.1 Utah 9 Florida 16.8 Vermont 0.9 Colorado 17.1 Virginia 4.7 Nevada 19.7 Washington 7.2 Arizona 25.3 WestVirginia 0.7 Texas 32 Wisconsin 3.6 California 32.4 Wyoming 6.4 NewMexico 42.1 Stem plots versus histograms Stem plots are quick and dirty histograms that can easily be done by hand, therefore, very convenient for back of the envelope calculations. However, they are rarely found in scientifi c or laymen publications. IMPORTANT NOTE: Your data are the way they are. Do not try to force them into a particular shape. It is a common misconception that if you have a large enough data set, the data will eventually turn out nice and symmetrical. Dot plots Like stem plots, dot plots show the entire raw data and are well suited for describing small data sets. Each individual in the data set is shown as one dot on the horizontal axis representing the variable’s scale. Individuals with identical value are superimposed vertically. Skin healing rates of 18 anesthetized newts. Each newt is shown as a dot. The plot indicates no obvious outlier. Line graphs: time plots Time always goes on the horizontal (x) axis. The variable of interest goes on the vertical (y) axis. Look for an overall trend and cyclical patterns. Overall upward trend in pricing over time: It could simply be reflecting inflation trends or more fundamental changes in this industry. Regular pattern of yearly variations: Seasonal variations in fresh orange pricing most likely due to similar seasonal variations in the production. Scales matter Death rates from cancer (US, 1945-95) How you stretch the axes and choose your scales can 250 give a different impression. Death rate (per thousand) 200 Death rates from cancer (US, 1945-95) 150 250 Death rate (per 200 100 thousand) 150 100 50 50 0 0 1940 1950 1960 1970 1980 1990 2000 1940 1960 1980 2000 Years Years Death rates from cancer (US, 1945-95) 250 Death rates from cancer (US, 1945-95) A picture is worth a 200 220 thousand words, Death rate (per thousand) Death rate (per thousand) 150 200 180 BUT 100 160 there is nothing like hard numbers. 50 140 0 120 Look at the scales. 1940 1960 1980 2000 1940 1960 1980 2000 Years Years Dispersion and box plots Today’s class objectives are: Describing distributions with numbers and Measure of center: mean and median Measure of spread: quartiles and standard deviation The fi ve-number summary and box plots IQR and outliers Dealing with outliers Choosing among summary statistics Organizing a statistical problem Measure of center: the mean The mean or arithmetic average To calculate the average (mean) of a data set, add all values, then divide by the number of individuals. It is the “center of mass.” x 1 x 2 .... xn x n Learn right away how to get the mean using your calculators. woman height woman height Heights (inches) of 25 women (i) (x) (i) (x) i=1 x1= 58.2 i = 14 x14= 64.0 i=2 x2= 59.5 i = 15 x15= 64.5 i=3 x3= 60.7 i = 16 x16= 64.1 i=4 x4= 60.9 i = 17 x17= 64.8 i=5 x5= 61.9 i = 18 x18= 65.2 i=6 x6= 61.9 i = 19 x19= 65.7 i=7 x7= 62.2 i = 20 x20= 66.2 i=8 x8= 62.2 i = 21 x21= 66.7 i=9 x9= 62.4 i = 22 x22= 67.1 i = 10 x10= 62.9 i = 23 x23= 67.8 i = 11 x11= 63.9 i = 24 x24= 68.9 i = 12 x12= 63.1 i = 25 x25= 69.6 i = 13 x13= 63.9 n=25 S=1598.3 Your numerical summary must be meaningful Height of 25 women in a class x 63.9 The distribution of women’s height appears coherent and symmetric. The mean is a good numerical summary. Here the shape of x 69.6 the distribution is wildly irregular. Why? Could we have more than one plant species or phenotype? Height of plants by color 5 x 70.5 red 4 pink Number of plants blue 3 2 1 0 58 60 62 64 66 68 70 72 74 76 78 80 82 84 Height in centimeters x 63.9 x 78.3 A single numerical summary here would not make sense. Measure of center: the median The median is the midpoint of a distribution—the number such that half of the observations are smaller and half are larger. 1 1 0.6 1 2 1 2 0.6 1.2 1. Sort observations from smallest to largest. 2 2 1.2 3 3 1.6 n = number of observations 3 3 1.6 4 4 1.9 ______________________________ 4 5 4 5 1.9 1.5 5 5 1.5 6 6 2.1 6 6 2.1 7 8 7 8 2.3 2.3 2. If n is odd, the median is 7 8 7 8 2.3 2.3 9 9 2.5 observation (n+1)/2 down the list 9 9 2.5 10 10 2.8 10 10 2.8 11 11 2.9 n = 25 11 11 2.9 12 13 12 3.3 3.4 (n+1)/2 = 26/2 = 13 12 13 3.3 3.4 14 1 3.6 Median = 3.4 14 1 3.6 15 2 3.7 15 2 3.7 16 3 3.8 16 3 3.8 17 4 3.9 3. If n is even, the median is the 17 4 3.9 18 5 4.1 mean of the two center observations 18 5 4.1 19 6 4.2 19 6 4.2 20 7 4.5 n = 24 20 7 4.5 21 8 4.7 21 8 4.7 22 9 4.9 n/2 = 12 22 9 4.9 23 10 5.3 Median = (3.3+3.4)/2 = 3.35 23 10 5.3 24 11 5.6 24 11 5.6 25 12 6.1 Comparing the mean and the median The mean and the median are the same only if the distribution is symmetrical. The median is a measure of center that is resistant to skew and outliers. The mean is not. Mean and median for a symmetric distribution Mean Median Mean and median for skewed distributions Left skew Mean Mean Right skew Median Median Mean and median of a distribution with outliers x 3.4 x 4.2 Percent of people dying Without the outliers With the outliers The mean is pulled to the The median, on the other hand, right a lot by the outliers is only slightly pulled to the right (from 3.4 to 4.2). by the outliers (from 3.4 to 3.6). Impact of skewed data Mean and median of a symmetric distribution Disease X: x 3.4 Med 3.4 Mean and median are the same. and a right-skewed distribution Multiple myeloma: x 3.4 Med 2.5 The mean is pulled toward the skew. Measure of spread: quartiles 1 1 0.6 2 2 1.2 3 3 1.6 4 4 1.9 The first quartile, Q1, is the value in 5 5 1.5 6 6 2.1 the sample that has 25% of the data 7 7 2.3 Q1= first quartile = 2.2 8 1 2.3 at or below it. 9 2 2.5 10 3 2.8 11 4 2.9 12 5 3.3 Med = median 13 3.4 = 3.4 14 1 3.6 15 2 3.7 16 3 3.8 17 4 3.9 18 5 4.1 The third quartile, Q3, is the value in 19 6 4.2 Q3= third quartile = 4.35 20 7 4.5 21 1 4.7 the sample that has 75% of the data 22 2 4.9 at or below it. 23 3 5.3 24 4 5.6 25 5 6.1 Measure of spread: standard deviation The standard deviation is used to describe the variation around the mean. To get the standard deviation of a SAMPLE of data: 1) Calculate the variance s2. mean 1 n ± 1 s.d. s2 n 1 1 ( xi x ) 2 x 2) Take the square root to get the standard deviation s. 1 n s n 1 1 ( xi x ) 2 Women’s height (inches) i xi x (xi-x) (xi-x)2 Calculations … 1 59 63.4 −4.4 19.0 2 60 63.4 −3.4 11.3 1 n i( ) 2 3 61 63.4 −2.4 5.6 s x x n 1 1 4 62 63.4 −1.4 1.8 5 62 63.4 −1.4 1.8 6 63 63.4 −0.4 0.1 7 63 63.4 −0.4 0.1 Mean = 63.4 8 63 63.4 −0.4 0.1 Sum of squared deviations from mean = 85.2 9 64 63.4 0.6 0.4 Degrees freedom (df) = (n − 1) = 13 10 64 63.4 0.6 0.4 11 65 63.4 1.6 2.7 s = variance = 85.2/13 = 6.55 inches squared 2 12 66 63.4 2.6 7.0 s = standard deviation = √6.55 = 2.56 inches 13 67 63.4 3.6 13.3 14 68 63.4 4.6 21.6 Mean Sum Sum 63.4 0.0 85.2 We’ll never calculate these by hand, so make sure you know how to get the standard deviation using your calculator. Center and spread in box plots 25 6 6.1 Largest = max = 6.1 24 5 5.6 23 4 5.3 Boxplot 22 3 4.9 21 2 4.7 20 1 4.5 Q3= third quartile 19 6 4.2 18 5 4.1 = 4.35 17 4 3.9 16 3 3.8 15 2 3.7 14 1 3.6 13 3.4 12 6 3.3 M = median = 3.4 11 5 2.9 10 4 2.8 9 3 2.5 8 2 2.3 7 1 2.3 6 6 2.1 Q1= first quartile 5 5 1.5 = 2.2 4 4 1.9 3 3 1.6 2 2 1.2 1 1 0.6 Smallest = min = 0.6 “Five-number summary” Boxplots for skewed data Comparing box plots for a normal and a right-skewed distribution 15 14 13 12 11 10 Years until death 9 Boxplots remain true 8 7 to the data and clearly 6 5 depict symmetry or 4 3 skewness. 2 1 0 Disease X Multiple myeloma IQR and outliers The interquartile range (IQR) is the distance between the fi rst and third quartiles (the length of the box in the boxplot) IQR = Q3 - Q1 An outlier is an individual value that falls outside the overall pattern. How far outside the overall pattern does a value have to fall to be considered a suspected outlier? Low outlier: any value < Q 1 – 1.5 IQR High outlier: any value > Q 3 + 1.5 IQR 25 6 7.9 24 5 5.6 * 23 4 5.3 8 22 3 4.9 21 2 4.7 20 1 4.5 Distance to Q3 19 6 4.2 Q3 = 4.35 7.9-4.35 = 3.55 18 5 4.1 17 4 3.9 16 3 3.8 15 2 3.7 Interquartile range 14 1 3.6 Q3 – Q1 13 3.4 12 6 3.3 4.35-2.2 = 2.15 11 5 2.9 10 4 2.8 9 3 2.5 8 2 2.3 7 1 2.3 6 6 2.1 Q1 = 2.2 5 5 1.5 4 4 1.9 3 3 1.6 2 2 1.2 Individual #25 has a survival of 7.9 years, which is 3.55 years 1 1 0.6 above the third quartile. This is more than 1.5 x IQR = 3.225 years. Individual #25 is a suspected outlier. Dealing with outliers What should you do if you fi nd outliers in your data? Well, it depends in part on what kind of outliers they are: Human error in recording information Human error in experimentation or data collection Unexplainable but apparently legitimate wild observations Are you interested in ALL individuals? Are you interested only in typical individuals? Choosing among summary statistics Because the mean is not resistant to Height of 30 women outliers or skew, use it to describe 69 distributions that are fairly symmetrical 68 67 and don’t have outliers. 66 Plot the mean and use the standard 65 Height in inches 64 deviation for error bars. 63 62 61 60 Otherwise, use the median in the five- 59 number summary, which can be plotted 58 Box Boxplot plot Mean Mean +± s.d. /- sd as a boxplot. Disease X Software output for summary Mean 3.312 Standard Error 0.288 statistics: Median 3.4 Excel Mode 2.3 2.3 =QUARTILE(A1:A25,1) Standard Deviation 1.439 4.2 =QUARTILE(A1:A25,3) Sample Variance 2.070 Kurtosis -0.682 CrunchIt! Skewness 0.056 Range 5.5 Column n Mean Std. Dev. Median Min Max Q1 Q3 Minimum 0.6 Disease X 25 3.312 1.4388422 3.4 0.6 6.1 2.3 4.2 Maximum 6.1 Sum 82.8 Count 25 Descriptive Statistics: Disease X Minitab Variable Count Mean StDev Minimum Q1 Median Q3 Maximum IQR Disease X 25 3.312 1.439 0.600 2.200 3.400 4.350 6.100 2.150 SPSS Percentiles Percentiles 5 10 25 50 75 90 95 Weighted DiseaseX.780 1.380 2.200 3.400 4.350 5.420 5.950 Average(Definition 1) Tukey's Hinges DiseaseX 2.300 3.400 4.200 Organizing a statistical problem State: What is the practical question, in the context of a real-world setting? Formulate: What specific statistical operations does this problem call for? Solve: Make the graphs and carry out the calculations needed for this problem. Conclude: Give your practical conclusion in the real-world setting. Today’s class objectives for learning are The importance of data dispersions, Different types of data dispersions, Implications of skewness and kurtosis in the data, How non-parametric approaches help? The usefulness of Z scores. 12/11/2024 61 Dispersion & Distributions Measures of Dispersion (Variability) Range Semi-Interquartile Deviation (SIQD) Sum of Squares Variance Standard Deviation Distributions Skewness Kurtosis Z-Scores 12/11/2024 62 Dispersion Spread Variability Measures the degree to which scores in distribution are spread out or clustered around the central point 12/11/2024 63 Non-parametric Measures of Dispersion Range Semi-Interquartile Deviation (SIQD) 12/11/2024 64 Range “Difference between upper and lower real limits” Real limits: The boundaries that form the intervals Separate 2 adjacent scores halfway between scores Easy formula: Range=largest # - smallest # Ex: Range b/w 32 seconds & 35 seconds? 35-32=3 12/11/2024 65 Range Infl uenced by extreme scores Works for Ordinal Data Is considered non-parametric statistic because it does not require one to estimate a parameter using a statistic such as the mean Least useful measure of dispersion because of the infl uence of extreme scores 12/11/2024 66 Semi-Interquartile Deviation (SIQD) Calculated from values for Quartiles Three scores (Q1, Q2, Q3) divide the distribution into 4 equal parts called quartiles (Q3 Q1) SIQD 2 12/11/2024 67 Semi-Interquartile Deviation (SIQD) Not infl uenced by extreme scores Because of the use of Q1 and Q3 values in the equation, extreme scores are ignored or not involved in the calculation Non-parametric because it does not require an estimation of a parameter using a statistic like the mean 12/11/2024 68 Parametric Measures of Dispersion Sum of Squares (SS) Variance Standard Deviation (SD) 12/11/2024 69 Sum of Squares In a frequency table, it is the sum of all of squared deviation scores Two formulas Theory Hand Calculator 12/11/2024 70 Sum of Squares Formula X mean 2 12/11/2024 71 Sum of Squares Hand Calculator Formula X 2 SS X 2 N 12/11/2024 72 Example Table X Subtract the Mean Deviation Squared Deviation Subject 1 2 -3 -1 1 Subject 2 4 -3 1 1 Subject 3 1 -3 -2 4 Subject 4 5 -3 2 4 Total 12 0 10 Number of subjects: n = 4 Sum of all Scores: x=12 Mean: x =12/4=3 12/11/2024 73 Sum of Squares Hand Calculator Formula X 2 SS X 2 N x2= 4+16+1+25 = 46 x=12 so (x)2 = (12)2 SS 46 - 12 2 10 4 12/11/2024 74 Variance Variance = SS/(N-1) = 10/(4-1) = 3.33 Which can be written as (1.8)2 That means: standard deviation = 1.8. 12/11/2024 75 Sum of Squares Parametric because we are calculating the mean Carries information about dispersion forward into formulas for more advanced statistical questions Will be seen as a part of the formula for most parametric statistical procedures 12/11/2024 76 Variance & Standard Deviation Both use the Sums of Squares Most important measures of dispersion 12/11/2024 77 Variance Population Variance: Variance X mean SS 2 N N Sample Variance: SS variance 12/11/2024 n 1 78 Standard Deviation Population Standard Deviation: 2 X mean Sd N SS Sd variance N Sample Standard Deviation: SS S n 1 12/11/2024 79 Variance & Standard Deviation Sd variance 2 Sd variance 12/11/2024 80 Why (n – 1)? This is a necessary adjustment to correct for the bias in sample variability Then, the square root of variability is calculated to create the standard deviation The standard deviation is a standardized measurement that can be compared to other studies 12/11/2024 81 Central Tendency & Dispersion Central Tendency Dispersion Parametric Mean Sums of Squres (SS) Variance Standard Deviation Non-Parametric Median Range Mode Semi-Interquartile Deviation 12/11/2024 82 Observations Central Tendency – Mean What happens to the mean if you add/subtract a constant to every score? What happens to the mean if you multiply/divide a constant to every score? 12/11/2024 83 Observations Dispersion – Standard Deviation What happens to the standard deviation if you add/subtract a constant to every score? What happens to the standard deviation if you multiply/divide every score by a constant? 12/11/2024 84 Frequency Tables A listing in order of the magnitude of each score and the number of times that score appears Gives some order to a set of data Can examine data for outliers – Outlier: value that is substantially different from the rest of the distribution 12/11/2024 85 Distributions Normal Distribution Skewness Kurtosis 12/11/2024 86 Normal Distribution Symmetrical, bell-shaped curve 0.45 0.4 0.35 0.3 0.25 0.2 0.15 0.1 0.05 0 48 54 60 66 72 78 84 12/11/2024 87 Skewness = (mean –median)/standard deviation Asymmetry of the distribution – Right/Positive Skewness Mean is greater than the median – Left/Negative Skewness Mean is smaller than the median 12/11/2024 88 Right/Positive Skewness The majority of the population is near the lower end The tail of the distribution is on the right side The mean will be greater than the median which will be greater than the mode Example: Income level in America 12/11/2024 89 Left/Negative Skewness The majority of the distribution is near the higher end The tail of the distribution is on the left side The mean will be less than the median which will be less than the mode 12/11/2024 90 Kurtosis How peaked or flat the curve is Can still be symmetrical shape Two types: – Leptokurtic: more peaked (like it leaps up) – Platykurtic: flatter (flat like a plate) 12/11/2024 91 Normal Distribution The normal distribution will have: – Mean = Median = Mode – Skewness = 0 – Kurtosis = 0 12/11/2024 92 Z-Scores Transformed raw score Shows relative position in distribution compared to all other scores Standardizing a distribution – if a distribution is asymmetrical, z- scores will transform the distribution into a normal shape 12/11/2024 93 Z-Scores X Mean Z 12/11/2024 94 Z-Score & Distribution A Z-Score of +1 to –1 accounts for approximately 68% of the distribution A Z-Score of +2 to –2 accounts for approximately 95% of the distribution A Z-Score of +3 to –3 accounts for approximately 99% of the distribution 12/11/2024 95 Look at Normal Table 97.5th percentile = 1.96 2.5th percentile = - 1.96 Median = mean = mode = 0 12/11/2024 96 Look at normal table Area on the greater side of 2.75 is 0.003. Can we say 99.7th percentile is 2.75 12/11/2024 97 Z-Scores Your grade is based on a z-score We do not use percentages because they are twice as likely as being wrong as a raw score Your score (x) will be the number of problems that you answer correctly on the exam 12/11/2024 98 Z-Scores & Your Grade A = Z-Score greater than 1 B = Z-Score between 0 and 1 C = Z-Score between –1 and 0 D = Z-Score between –2 and –1 F = Z-Score less than -2 12/11/2024 99 Today’s class objectives are …. Learn how to compute and interpret confidence intervals, Learn on how to test hypothesis testing Learn probability, sensitivity, specificity, positive predictive and negative predictive values with examples!! What is probability? Probability is a quantitative speculation of an uncertain future event (that is, outcome) Probability is a value and it is always in a closed bracket [0, 1] Probability P(A) = 30/100=0.3 Now have Now no breast breast P(A bar)=1-P(A) =0.7 cancer (A) cancer (A bar) P(AB) = 10/100=0.1 P(B) = 40/100 =0.4 Did 10 30 P(B bar) = 1- P(B) = undergo screening? 60/100 =0.6 (B) Is P(AB) = P(A) P(B)? No Did not 20 40 undergo P(A/B)=P(AB)/P(B) = screening? 10/40 = 0.25 (B bar) P(A bar/B bar) =40/60=0.67 Are A and B independent? No Probability P(A) = 30/100=0.3 Now have Now no breast breast P(A bar)=1-P(A) =0.7 cancer (A) cancer (A bar) P(AB) = 10/100=0.1 P(B) = 40/100 =0.4 Did 10 30 P(B bar) = 1- P(B) = undergo screening? 60/100 =0.6 (B) Is P(AB) = P(A) P(B)? No Did not 20 40 undergo P(A/B)=P(AB)/P(B) = screening? 10/40 = 0.25 (B bar) P(A bar/B bar) =40/60=0.67 Are A and B independent? No Sensitivity & Specificity Sensitivity: The ability of a test to correctly identify those with the disease (true positives) Specificity: The ability of a test to correctly identify those without the disease (true negatives) Ideal Screening Test 100% sensitive = No false negatives 100% specific = No false positives In the real world… TEST RESULTS NEGATIVE (-) POSITIVE (+) Actual diagnosis Actual diagnosis Not Diseased Diseased Not Diseased Diseased True Negative False Negative False Positive True Positive (TN) (FN) (FP) (TP) CORRECT CORRECT Oops! Should not have these Step 3: Calculating the Sensitivity True Diagnosis Diseased Not Diseased Total a b Positive 12 3 15 Test Result c d Negative 8 57 65 Total 20 60 80 a True Positives Sensitivity = (a + c) = True Positives + False Negatives Sensitivity = 12/20 = 60% Step 4: Calculating the Specificity True Diagnosis Diseased Not Diseased Total a b Positive 12 3 15 Test Result c d Negative 8 57 65 Total 20 60 80 d True Negatives Specificity = (b + d) = False Positives + True Negatives Specificity = 57/60 = 95% Efficiency (EFF) Formula: EFF = 100 (TP+TN)/(TP+TN+FP+FN) In our data, EFF = 100 (12+57)/(12+57+ 3+8) =86.25. PPV (Positive Predictive Value) Formula: PPV=100(TP)/(TP+FP) In our data: PPV =100 (12)/(12+3)=80 NPV (Negative Predictive Value) Formula: NPV=100(TN)/(TN+FN) In our data, NPV=100(57)/(57+8)=87.6 Another Example (for homework) Test Result True Status Diseased Healthy Total Diseased 49 1 50 Healthy 38 912 950 Total 87 913 1000 Answers to the Example (continued) Sn = 49/50 = 0.98 Sp = 912/950 = 0.96 PPV = 49/87 = 0.56 NPV = 912/913 = 0.999 Sensitivity & specificity are close, but PPV smaller because prevalence of disease is smaller, namely 50/1000 or 5%. Density of bacteria in solution Measurement equipment has standard deviation s = 1 million bacteria/ml of fluid. 3 measurements: 24, 29, and 31 million bacteria/ml of fluid Mean: x = 28 million bacteria/ml. Find the 96% and 70% CI. 96% confidence interval for the 70% confidence interval for the true density, z* = 2.054, and write true density, z* = 1.036, and write = 28 ± 1.036(1/√3) = 28 ± 2.054(1/√3) x z * x z * n = 28 ± 1.2 n = 28 ± 0.6 [26.8, 29.2] million bacteria/ml [27.4, 28.6] million bacteria/ml P-value in one-sided and two-sided tests One-sided (one-tailed) test Two-sided (two-tailed) test To calculate the P-value for a two-sided test, use the symmetry of the normal curve. Find the P-value for a one-sided test and double it. Do poor mothers have under weight babies? The national average birth weight is 120 oz: N(natl =120, = 24 oz). An SRS of n=100 poor mothers gave x̅ = 115 oz. Are these statistically different at the 5% significance level? At 1% level? Hypotheses: H0: poor = 120 oz (no difference with natl ) Ha: poor < 120 oz If H0 were true, the sampling distribution of x̅ would be N(120, 24/√100). x 115 120 We calculate the z score for x̅: z 2.083 n 24 100 In Table B, z = -2.08 gives left area = 0.0188 P-value 1.88%. In Table C, we find that the P-value is between 1% and 2%. Random variation from the random sampling process alone would produce such a low average birth weight (of 115 oz OR LESS) in 1.88% of the cases. H0 can be rejected at a 5% (p ≤ a), but not at a 1% (p > a). The P-value The packaging process is Normal with standard deviation s = 5 g. H0: µ = 227 g versus Ha: µ ≠ 227 g The average weight from your 4 random boxes is 222 g. What is the probability of drawing a random sample such as yours or even more extreme if H0 is true? Tests of statistical significance quantify the chance of obtaining certain random sample results if the null hypothesis were true. This quantity is the P-value. This is a way of assessing the “credibility” of the null hypothesis given the evidence provided by a random sample. Does the packaging machine need revision? H0: µ = 227 g versus Ha: µ ≠ 227 g What is the probability of drawing a random sample such as yours or worse if H0 is true? x 222g 5g n 4 x 222 227 z 2 n 5 4 From Table A, area left of z is 0.0228. Sampling distribution if P-value = 2*0.0228 = 4.56%. H0 were true σ/√n = 2.5g The probability of getting a random 2.28% 2.28% sample average so different from 217 222 227 232 237 µ is so low that we reject H0. Average package weight (n=4) The machine does need recalibration. x, µ (H0) z 2 From table C, 2-sided P-value is between 4% and 5% (use |z|). The weight of single eggs varies Normally with standard deviation 5g. Think of a carton of 12 eggs as an SRS of size 12. What is the distribution of the sample means x ? Normal (mean m, standard deviation s/√n) = N(?g,1.44g). Find the middle 95% of this sampling distribution. Roughly ± 2 standard deviations (± 2.88g) from the unknown mean μ. You buy one carton of 12 eggs. The average egg weight is x̅ = 64.2g in this SRS. What can you infer about the mean µ of this population? There is a 95% chance that the population mean µ is roughly within ± 2s/√n of x̅, or 64.2g ± 2.88g.