Sample Probabilities: Statistics for Business PDF
Document Details
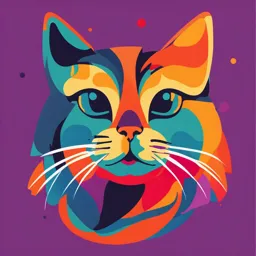
Uploaded by ImaginativeHeliotrope2235
Tags
Related
- Sampling Distributions PDF
- Business Statistics: A First Course Chapter 7 PDF
- Business Statistics: A First Course Chapter 7 PDF
- RESM2001 (2024-25) Lecture 2.1 - From Sample To Population PDF
- Statistics Chapter 13: Sampling Distributions PDF
- Sampling Distributions - BMS 511 Biostats & Statistical Analysis PDF
Summary
These student notes cover the concepts of sample probabilities, data analysis and interpretation, sampling distributions, and the Central Limit Theorem (CLT). The notes explore core statistical tools for business decision-making, and how to estimate the characteristics of the population.
Full Transcript
3b | Sample Probabilities Data Analysis and Interpretation Interpret data using statistics tools for business decision-making Intended Learning Outcomes: ❖ Explain the rationale and characteristics of a sampling distribution of the sample means. ❖ Explain the Central Limit Theorem (CLT). ❖ Analys...
3b | Sample Probabilities Data Analysis and Interpretation Interpret data using statistics tools for business decision-making Intended Learning Outcomes: ❖ Explain the rationale and characteristics of a sampling distribution of the sample means. ❖ Explain the Central Limit Theorem (CLT). ❖ Analyse the probability of a variable using sampling distributions. ❖ Compute the probability of an event for an unknown distribution. Often, research begins with a question about the entire population, but actual research is conducted using a sample. Ultimately, the goal of statistics is to draw inferences from sample to population1. Drawing inferences mean that: the findings observed between samples also exist in the general population. the findings in the samples are not due to the chance or random variability in your data (i.e., statistical noise) Imagine you're trying to pick the next viral meme from your friend's endless stream of cat videos. We can't look at every cat video but we can analyse a small collection (or sample) and use it to estimate the chances of finding that next internet sensation. Or think of it as sampling a bag of your favourite jellybeans to see which flavour is most likely to sweeten up your day. Source: Creative Commons (2024) 1 Recall in Topic 1 we talked about the role of samples in statistics. You may wish to re-visit it. BLO1001 Statistics for Business 1|P a g e Inferential statistics and sampling error How can a sample provide a complete picture of the population? For example, your lecturer randomly selects a sample (n) of 25 students from TP. We are aware that the sample should be representative of the entire student population, but there are some segments of the population that would be missed out from the sample. Any statistics computed for the sample will not be identical to the corresponding parameters for the entire population. For example, the average cGPA for the sample 25 students will not be the same as the overall mean of cGPA for the entire TP student population. The above example highlights the differences between sample statistics and the corresponding population parameters, or what we call in statistical terms, sampling error. Sampling error refers to the difference between the results obtained from a sample of a population and the actual characteristics of that population. A sampling error: occurs because a sample is only a subset of the larger population and thus may not perfectly represent it. As a result, estimates or conclusions drawn from the sample may differ from what would be found if the entire population were surveyed. is not due to mistakes or inaccuracies in data collection but rather the natural variation that happens between the sample mean and population mean. A larger, more representative sample can help reduce sampling error but it is often impossible to eliminate completely. Thus, we can never fully ‘prove’ that our sample is perfectly representative of the population but we can make calculated guesses (inferences) on how well the sample represents the population. Probability techniques and concepts such as the normal distribution and continuous probability help us make these educated guesses. BLO1001 Statistics for Business 2|P a g e Explain the rationale and characteristics of a sampling distribution of the sample means. Samples are variable as they are not the same and can change. If you were to take three separate samples (groups of students) from the same TP student population, your samples will be different. Can you point out some potential differences? Typically, thousands of different samples can be drawn from a single population. With so many samples available, it might seem impossible to find clear patterns that connect the samples to the overall population. But, this vast collection of samples actually follow a simple and predictable pattern and this then allows us to estimate the characteristics of individual samples with reasonable accuracy. This predictability is based on the distribution of sample means. Distribution of sample means is the collection of sample means for all the possible random samples of a particular size (n) that can be obtained from a population. Done by: a) Select random sample of specific size (n) b) Calculate sample mean. c) Place sample mean in a frequency distribution (topic 2b) d) Repeat steps (a) to (c) for the next sample. A sampling distribution is a distribution of sample means, given a specific sample size. Example Suppose we have a population of 6 people, whose ages range between 12 to 22. Assume that the population is normally distributed. The population mean () = 17 years old We randomly select any 3 people (so n2 = 3). What are all the combinations of samples of 3 people we can possibly get? 2 The letter n is referring to sample size. BLO1001 Statistics for Business 3|P a g e We can see from the right figure that the total number of groups we can derive from the population is 20. The sample mean is calculated for each group and we see it is different across the groups. 1. With all these sample 2. When we apply what we 3. Applying what we have means collected, we have learnt in Topic 2a, the learnt in Topic 2b and can put them into a Descriptive Statistics and when we group the table, looking like this: the outcome will be: sample means in a frequency distribution table, it will look like this: Sample # Sample Mean Sample Mean Sample Mean Frequency 1 14.00 13 up to 14 1 2 14.67 Mean 17 14 up to 15 3 3 15.33 Standard Error 0.3504 15 up to 16 3 4 16.00 Median 17 16 up to 17 6 5 15.33 Mode 16 17 up to 18 3 6 16.00 Standard Deviation 1.5672 18 up to 19 3 7 16.67 Sample Variance 2.4561 19 up to 20 1 8 16.67 Kurtosis -0.4471 Total 20 9 17.33 Skewness 0.0000 10 18.00 Range 6 11 16.00 Minimum 14 12 16.67 Maximum 20 13 17.33 Sum 340 14 17.33 Count 20 15 18.00 16 18.67 We see the mean (average) of 17 18.00 the sample means (x̅) is equal 18 18.67 to the population mean () of 19 19.33 17. 20 20.00 BLO1001 Statistics for Business 4|P a g e And when we use a histogram to present the distribution of the sample means, we have a normal distribution3. In this example, the collection of samples follows a predictable pattern and this then allows us to estimate the characteristics of individual samples with reasonable accuracy. Characteristics of the Distribution of Sample Means As seen from the earlier example: The sample means should be relatively close to the population mean Even though samples are different from one another but they are representative of the population. The sample means should tend to form a normal distribution if the population is normally distributed or the sample size is large enough (i.e. 30 and above). The larger the sample size, the closer the sample means are to the population mean In other words, the sample means obtained with a large sample size should cluster relatively close to the population mean while the means obtained from small samples should be more widely scattered. The Central Limit Theorem (CLT) The Central Limit Theorem is a powerful concept used widely in statistics. Put simply, it describes the sample behaviour of averages (means) drawn from a larger population. If all samples of a particular size are selected from any population, the sampling distribution of the sample mean is approximately a normal distribution. This approximation improves with larger samples. Imagine you have a collection of data and you take many random samples of a certain size from that data. The Central Limit Theorem tells us that, regardless of the original data's shape (skewed, uniform, etc.), the distribution of the means of these samples will tend towards a bell-shaped curve, known as the normal distribution, as the sample size increases. 3 Recall from Topic 3a on the characteristics of a normal distribution. BLO1001 Statistics for Business 5|P a g e This is crucial in probability because it allows us to predict how averages of samples will behave, even if the original data is messy or unpredictable. By understanding this pattern, we can make more accurate predictions about a whole population just by analysing samples. Graph depicting the shapes of distribution according to CLT Source: ChatGPT (2024) Shape of distribution according to CLT As mentioned above, distribution of the means of these samples will tend towards a normal distribution. This is true based on the Central Limit Theorem as long as either one of the following two conditions is satisfied: ❶ The population from which the samples are selected follows a normal distribution. ❷ The sample size (n) is large enough, i.e. at least 30 (n ≥ 30). Mean of the distribution according to CLT According to CLT, the mean of the distribution of sample means 4 is always equal to the population mean. x̅ = where is population mean and x̅ is mean of the sampling distribution 4 Also termed as Sampling Distribution BLO1001 Statistics for Business 6|P a g e Standard Error of the Mean according to CLT The Standard Error of the Mean is the Standard Deviation of the distribution of the sample means. Just like the standard deviation we learnt in Topic 2a (measures of dispersion), the standard error describes the distribution of the sample means. When the standard error is small, all the sample means are close together and have similar values. If the standard error is large, the sample means are scattered over a wide range and there are larger differences from one sample to another. The standard error also helps us to understand how closely an individual sample mean reflects the overall mean for the sampling distribution. Although a sample mean should be representative of the population mean, there is usually some error between the sample and the population. The standard error also tells us how the distance is between an individual sample mean (x̅) and the population mean (). Formula: 𝝈 Standard Error → x̅ = √𝒏 Used when population standard deviation () is known. The standard error is determined by two factors: (1) the size of the sample and (2) the standard deviation of the population from which the sample is selected. Let’s see how the standard error differs with different sample sizes in the below example using two samples. Example The time taken for students who live in Clementi to travel to TP is normally distributed with a mean of 100 minutes and a standard deviation of 10 minutes. Calculate and compare the standard error of one sample of 30 students with another sample of 100 students. Let x be the time taken to travel from Clementi to TP. Given population = 100, = 10 (a) Sample size (n) = 30 (b) Sample size (n) = 100 Since population is normally distributed and sample size ≥ 30, the sampling distributions of both samples are also normal (based on the CLT). 𝜎 10 10 𝜎 10 x̅ = = = = 1.83 x̅ = = = 1.00 √𝑛 √𝟑𝟎 5.477 √𝑛 √𝟏𝟎𝟎 BLO1001 Statistics for Business 7|P a g e The blue curve represents the distribution The green curve represents the distribution for a sample size of 30 with a standard error for a sample size of 100 with a standard of 1.83. The wider spread indicates greater error of 1.00. This spread is narrower and variability in the sample means. reflects what was indicated earlier, where the sample means obtained with a large sample size should cluster relatively close to the population mean. There may be situations where we do not have access to the population standard deviation, such as an incomplete dataset of the target population or we are working with data from a sample5. In such cases, we use the standard deviation (s)6 of that individual sample instead to compute the standard error. Formula: 𝒔 Standard Error → sx̅ = √𝒏 When population standard deviation () is unknown. Sample standard deviation is known. 5 These can be small studies or experiments. Or they can also be pilot studies conducted to test the data and its collection methods. 6 The sample standard deviation (s) is not the same as standard error. Sample standard deviation is from one sample, whereas standard error looks across the sampling distribution. BLO1001 Statistics for Business 8|P a g e Analyse the probability of a variable using sampling distributions With the Central Limit Theorem, we can analyse the probability of a variable using sampling distributions. This involves a number of steps. Step 1: Define the variable x. (x describes the parameter that we are interested in finding out.) Step 2: State the mean, standard deviation and sample size. Step 3: Determine the Sampling Distribution Use this decision flow chart to determine if the Central Limit Theorem (CLT) can be applied. Decision flow to determine sampling distribution Mean of the sampling distribution: x̅ = where is population mean and x̅ is mean of the sampling distribution Standard Error: when population standard deviation () is known. 𝝈 x̅ = √𝒏 Standard Error: when population standard deviation () is unknown. 𝒔 sx̅ = √𝒏 BUT Sample standard deviation is known. BLO1001 Statistics for Business 9|P a g e Step 4: Define the probability statement. (Note: As we are dealing with sampling distributions, the symbol to represent the raw value of the sample mean is x̅.) Step 5: Sketch the sampling distribution, mark the x̅-value and shade the appropriate area of interest below the distribution. Step 6: Convert the sampling distribution into the Standard Normal Distribution. Transform the x̅-value into z-score using this formula: (Note: The mean in a z-score is always zero 07.) ̅ − 𝝁𝒙̅ 𝒙 ̅ − 𝝁𝒙̅ 𝒙 𝒛= 𝒛= 𝝈𝒙̅ 𝒔𝒙̅ (when population standard deviation is known) (when population standard deviation is unknown) where: x̅ = raw value of the sample mean x̅ = mean of the sampling distribution x̅ or sx̅ = standard error of the sampling distribution z = location of the x̅-value in the standard normal distribution Step 7: Add the z-axis and locate the necessary z-score/s. Step 8: Find the probability from the Standard Normal Distribution table which gives the probability of 𝑝 =𝑃(0 ≤ 𝑧 ≤ 𝑍) Did you observe any similarities between the above steps and what you learnt in Topic 3a Continuous Probabilities and Normal Distribution? 7 Recall from Topic 3a when we talked about Standard Normal Distribution. BLO1001 Statistics for Business 10 | P a g e Example The amount of time spent by teenagers on physical activities is normally distributed with a mean () of 60 minutes and standard deviation () of 12 minutes. For a sample of 15 randomly selected teenagers, find the probability that: a) the sample mean time they spend on physical activities is greater than 63 minutes. Step 1: Define the variable x. Let x be the amount of time spent by teenagers on physical activities. Step 2: State the mean, standard deviation and sample size. Given = 60 minutes, = 12 minutes and sample size (n) = 15 Step 3: Determine the Sampling Distribution Given x is normally distributed, x̅ will also be normally distributed. Mean of the sampling distribution: x̅ = = Standard error: 𝝈 x̅ = 𝟏𝟐 = √𝟏𝟓 = 3.098 √𝒏 Step 4: Define the probability statement. p (x̅ > 63) BLO1001 Statistics for Business 11 | P a g e Step 5: Sketch the sampling distribution, mark the x̅-value and shade the appropriate area of interest below the distribution. Step 6: Convert the sampling distribution into the Standard Normal Distribution. Transform the x̅ -value into z-score using this formula: ̅ − 𝝁𝒙̅ 𝒙 𝒛= 𝝈𝒙̅ 𝟔𝟑−𝟔𝟎 p (x̅ > 63) = p (z > 𝟑.𝟎𝟗𝟖 ) = p (z > 0.97) Step 7: Add the z-axis and locate the necessary z-score/s. Step 8: Find the probability from the Standard Normal Distribution table which gives the probability of p = p (0 ≤ z ≤ 0.97) = 0.3340 When z-score is 0.97, the probability is 0.3340. 𝟔𝟑−𝟔𝟎 p (x̅ > 63) = p (z > 𝟑.𝟎𝟗𝟖 ) = p (z > 0.97) = 0.5 – 0.3340 = 0.166 We conclude with the statement – The probability that the sample mean time spent on physical activities is greater than 63 minutes is 0.166. BLO1001 Statistics for Business 12 | P a g e b) the sample mean time spent on physical activities is between 55 and 63 minutes. 𝟓𝟓−𝟔𝟎 𝟔𝟑−𝟔𝟎 p (55 ≤ x̅ ≤ 63) = p ( 𝟑.𝟎𝟗𝟖 ≤ z ≤ 𝟑.𝟎𝟗𝟖 ) = p (-1.61 ≤ z ≤ 0.97) = 0.4463 + 0.3340 = 0.7803 The probability that the sample mean time spent on physical activities between 55 and 62 minutes is 0.7803. Compute the probability of an event for an unknown distribution We talked about sampling error earlier and we know that it is not due to mistakes or inaccuracies in data collection but rather the natural variation that happens between the sample mean and population mean. To estimate how well the sample represents the population, we can also apply CLT, use the standard normal distribution and compute the probability of a sampling error occurring. Example Nike’s annual report says that the average American buys 6.5 pairs of sports shoes per year. The report did not describe the shape of the population distribution. A sample of 81 customers is surveyed and the standard deviation of sports shoes they purchased per year is 2.1. What is the probability that the difference between this sample mean and the population mean is less than 0.25 pair? Step 1: Define the variable x. Let x be the number of pairs of sports shoes purchased by the average American per year. Step 2: State the mean, standard deviation and sample size. Given = 6.5, s = 2.1 and n = 81 Step 3: Determine the Sampling Distribution The population distribution and standard deviation are unknown, but sample size is ≥ 30 (n = 81). Hence we apply the Central Limit Theorem (CLT) and assume the sampling distribution is normally distributed. BLO1001 Statistics for Business 13 | P a g e Mean of the sampling distribution: x̅ = = 𝒔 𝟐.𝟏 Standard error: sx̅ = = √𝟖𝟏 = 0.2333 √𝒏 Step 4: Define the probability statement. P (6.25 < x̅ < 6.758) Step 5: Sketch the sampling distribution, mark the x̅-value and shade the appropriate area of interest below the distribution. Step 6: Convert the sampling distribution into the Standard Normal Distribution. Transform the x̅ -values into z-scores using this formula: ̅ − 𝝁𝒙̅ 𝒙 𝒛= 𝒔𝒙̅ 𝟔.𝟐𝟓−𝟔.𝟓 𝟔.𝟕𝟓−𝟔.𝟓 p (6.25 < x̅ < 6.75) = p ( 𝟎.𝟐𝟑𝟑𝟑 < 𝒛 < ) 𝟎.𝟐𝟑𝟑𝟑 = p (-1.07 < z < 1.07) Step 7: Add the z-axis and locate the necessary z-score/s. Step 8: Find the probability from the Standard Normal Distribution table which gives the probability of p (0 < z < z1) = 0.3577 When z-score is +1.07 or -1.07, the probability is 0.3577. 𝟔.𝟐𝟓−𝟔.𝟓 𝟔.𝟕𝟓−𝟔.𝟓 p (6.25 < x̅ < 6.75) = p (