Chapter 8 Testing Means: One-Sample t Test PDF
Document Details
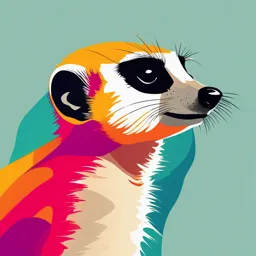
Uploaded by MasterfulHarpsichord
Tags
Summary
This chapter details the one-sample t-test, a statistical method used to analyze data from a single group when the population variance is unknown. It covers hypothesis testing and effect size calculations. The chapter explains that in behavioral science, the population variance is rarely known and therefore the one-sample t-test is utilized.
Full Transcript
Chapter 8 **Testing Means:** **One-Sample *t* Test** - Chapter 9 Learning Outcomes - Know when to use *t* statistic instead of *z*-score hypothesis test - Perform hypothesis test with *t* statistics - Evaluate effect size by computing Cohen's *d,* percentage of variance accounted f...
Chapter 8 **Testing Means:** **One-Sample *t* Test** - Chapter 9 Learning Outcomes - Know when to use *t* statistic instead of *z*-score hypothesis test - Perform hypothesis test with *t* statistics - Evaluate effect size by computing Cohen's *d,* percentage of variance accounted for (*r*^2^), and/or a confidence interval - The Problem with *z*-Scores - The *z*-score requires more information than researchers typically have available - Requires knowledge of the population standard deviation σ, which is needed to compute the standard error - Researchers usually have only the sample data available - **Going From *z* to *t*** - To compute a *z* score, population variance must be known - **In behavioral science it is rare that the variance in a population is known** - This substitution, called the estimated standard error, is the denominator of the test statistic for a *t* test - This is acceptable because sample variance is an unbiased estimator of the population variance - **Going From *z* to *t*** - **Estimated Standard Error**\--an estimate of the standard deviation of a sampling distribution of sample means selected from a population with an unknown variance. It is an estimate of the standard error or standard distance that sample means deviate from the value of the population mean stated in the null hypothesis - - **Going From *z* to *t*** - Using the substitution of sample variance for population variance, a new test statistic is introduced by Gosset - **The *t* statistic:** Used to determine the number of standard deviations in a *t* distribution that a sample deviates from the mean value or difference stated in the null - Introducing the *t* Statistic - The *t* statistic uses the estimated standard error in place of σ*~M~* *𝑡=𝑀−𝜇𝑠𝑀* - The t statistic is used to test hypotheses about an unknown population mean μ when the value of σ is also unknown - The *t* Distribution - "Family" of distributions, one for each possible number of degrees of freedom - Approximates the shape of a normal *z*-score distribution - Flatter than the normal *z*-score distribution - More spread out than the normal *z*-score distribution - More variability ("fatter tails") in *t* distribution - Use table of values of *t* in place of the unit normal table for hypothesis tests - Figure 9.1 Distributions of the *t* Statistic - Degrees of Freedom and the *t* Statistic - Computation of sample variance requires computation of the sample mean first - Only *n* -- 1 scores in a sample are independent - Researchers call *n* -- 1 the degrees of freedom - Degrees of freedom describe the number of scores in a sample that are independent and free to vary - Noted as *df* - *df* = *n* -- 1 - Figure 9.2 The *t* Distribution with *df* = 3 - 9-2 Hypothesis Tests with the *t*\ Statistic - The one-sample *t* test statistic (assuming the null hypothesis is true) - Figure 9.3 The Basic Research Situation for the *t* Statistic Hypothesis Test - **One-Sample *t* Test** - A statistical procedure used to test hypotheses concerning a single group mean in a population with an unknown variance - Three assumptions are made: - 1\. Normality\--assume data in the population being sampled is normally distributed - 2\. Random Sampling\--assume that the data were obtained using a random sampling procedure - 3\. Independence\--assume that probabilities of each measured outcome in a study are independent - Using the *t* Statistic for Hypothesis Testing Four steps - State the null and alternative hypotheses and select an alpha level - Locate the critical region using the *t* distribution table and the value for *df* - Calculate the *t* test statistic - Make a decision regarding *H*~0~ (null hypothesis) - **Example 9.2: One-Sample *t* Test** - Chang, Aeschbach, Duffy, and Czeisler (2015) report that reading from a light-emitting eReader before bedtime can significantly affect sleep and lower alertness the next morning. - To test this finding, a researcher obtains a sample of 9 volunteers who agree to spend at least 15 minutes using an eReader during the hour before sleeping and then take a standardized cognitive alertness test the next morning. - For the general population, scores on the test average μ = 50 and form a normal distribution. The sample of research participants had an average score of M= 46 with SS= 162. - Example 9.2 - STEP 1 - **Step 1: State the hypotheses** - Null hypothesis states that late-night reading from a light-emitting screen has no effect on alertness the following morning. - The alternative hypothesis states that reading from a screen at bedtime does affect alertness the next morning. - **Reading the t Table** - To locate probabilities and critical values in a *t* distribution, a *t* table is used. - We will use the *t* distribution and critical values listed in this table to compute *t* tests Need to know: *n,* α, and location of rejection region - Example 9.2 - STEP 2 - **Step 2: Set the criteria for making a decision** - Level of significance for this test is.05 - Since *n* = 9, the *df* = 8 (9 -- 1 = 8) - To locate the critical values that make the cutoffs under a *t* distribution equal to.05 in two tails combined, find 8 listed in the rows and go across the column for.05 in two-tails combined. - **The critical values are ± 2.306** - Figure 9.4 The Critical Region in the\ *t* Distribution for α =.05 and *df* = 8 - Example 9.2 - STEP 3 Step 3: Compute test statistic (t~obt~) - Example 9.2 - STEP 3 Step 3: Compute test statistic (t~obt~) e - Making a Decision - Example 9.2- STEP 4 - **Step 4: Make a decision** - The obtained *t* statistic of −2.67 falls into the critical region on the left-hand side of the *t* distribution. - Our statistical decision is to reject , which is evidence that reading from a light-emitting screen at bedtime does affect alertness the following morning. There is a tendency for the level of alertness to be reduced after reading a screen before bedtime. - **Example 9.2: One-Sample *t* Test** **Conclusion (in APA format):** The participants had an average of with on a standardized alertness test the morning following bedtime reading from a light-emitting screen. Statistical analysis indicates that the mean level of alertness was significantly lower than scores for the general population, *t* (8) = -2.67, p \< 0.05 - The Influence of Sample Size and Sample Variance - The larger the sample, the smaller the error - The larger the variance, the larger the error - Large variance means that you are less likely to obtain a significant treatment effect - Reduces the likelihood of rejecting the null hypothesis - 9-3 Measuring Effect Size for the *t* Statistic - Hypothesis test simply determines whether the treatment effect is greater than chance - "Chance" is measured by the standard error - No measure of the size of the effect is included - A very small treatment effect can be statistically significant - Therefore, results from a hypothesis test should be accompanied by a report of effect size such as Cohen's *d* - **Effect Size for the One-Sample *t* Test** - To estimate the size of the effect in the population, effect size is computed - Typically only calculated after finding a significant effect (reject null) - Three measures of effect size: - *Estimated Cohen's d\*\*\*\** - *Eta-Squared (proportion of variance)* - *Omega-Squared (proportion of variance)* - **Effect Size for the One-Sample *t* Test** - Estimated Cohen's *d\--*measure of effect size in terms of the number of SDs that mean scores shift above or below the population mean stated by the null hypothesis. - The larger the value of *d*, the larger the effect Example 9.2 : *M* = 46, μ = 50; S = 4.5 - **Effect Size for the One-Sample *t* Test** - **[Proportion of Variance-]**-measure of effect size in terms of the proportion or percent of variability in a dependent variable that can be explained or accounted for by a treatment - **[Treatment]**\--any unique characteristic of a sample or any unique way that a researcher treats a sample - Can change value of a dependent variable - Associated with variability in a study - Measuring the Percentage of Variance Explained, *r*^2^ - An alternative method for measuring effect size is to determine how much of the variability in the scores is explained by the treatment effect - *r*^2^ = 0.01 small effect - *r*^2^ = 0.09 medium effect - *r*^2^ = 0.25 large effect - **Effect Size for the One-Sample *t* Test** - Example of calculating Proportion of Variance in Ex. 9.2 - Eta-Squared (R2) *t* = −2.67; *df* = 8 46% of the variability in cognitive alertness (DV) can be explained by the fact that participants were using e-reader before bed (treatment). - Confidence Intervals for Estimating μ - Alternative technique for describing effect size - Estimates μ from the sample mean (*M*) - Based on the reasonable assumption that *M* should be "near" μ - The interval constructed defines "near" based on the estimated standard error of the mean (*s~M~*) - Can confidently estimate that μ should be located in the interval near to the statistic - **Confidence Intervals** - **Follow three steps to estimate the value of a population mean using a interval estimate:** - **Step 1: Compute the sample mean and standard error** - **Step 2: Choose the level of confidence and find the critical values at that level of confidence** - **Step 3: Compute the estimation formula to find the confidence limits** - **Estimation for the One-Sample *t* Test** - **You can use estimation as an [alternative] to each *t* test** - Estimation formulas: - One-sample *t*: - Figure 9.7 The Distribution of\ *t* Statistics for *df* = 8 - **Estimation for the One-Sample *t* Test (cont.)** - **[Step 1: Compute the sample mean and standard error ]** - *M* = 46 - - **[Step 2: Choose the level of confidence and find critical values at that level of confidence]** - You want to find 95% confidence interval, so choose a 95% level of confidence (α=.05) - **Note:** 99% confidence interval, so choose a 99% level of confidence (α=.01) - **Estimation for the One-Sample *t* Test (cont.)** - **[Step 3: Compute the estimation formula to find confidence limits for a 95% confidence interval]** 1\. Multiply *t critical* times the SE: 2\. Find the upper and lower limits Upper Limit: 45 + 3.459 = 49.459 Lower Limit: 45 -- 3.459 = 42.541 - Interpretation of Confidence Intervals - Factors Affecting the Width of a Confidence Interval - Desired confidence level - When more confidence is desired, the width of the interval increases - When less confidence is acceptable, the width of the interval decreases - Sample size - Larger sample smaller SE smaller interval - Smaller sample larger SE larger interval - 9-4 Directional Hypotheses and One-Tailed Tests - ***Non-directional*** (two-tailed) test is most commonly used - However, a directional test may be used for particular research situations (e.g., exploratory investigations or pilot studies) - Four steps of hypothesis test are carried out - The critical region is defined in just one tail of the *t* distribution - Example 9.6 - STEP 1 - **Step 1: State the hypotheses** - The null hypothesis states that alertness scores will not be lowered by reading from a screen late at night. In symbols,. - The alternative hypothesis states that the treatment will work. In this case, H1 states that alertness scores will be lowered by reading from a light-emitting screen before bedtime. - Figure 9.8 The One-Tailed Critical Region for the Hypothesis Test in Example 9.6 with *df* = 8 and α =.05 - Example 9.6 STEP 3 Step 3: Compute test statistic (t~obt~) e - Example 9.6- STEP 4 - **Step 4: Make a decision** - The obtained *t* statistic of −2.67 falls into the critical region on the left-hand side of the *t* distribution. REJECT NULL - Learning Check 2 (1 of 2) - The results of a hypothesis test are reported as follows: *t*(21) = 2.38, *p* \<.05. What was the statistical decision and how big was the sample? - - - - - Learning Check 2 -- Answer (1 of 2) - The results of a hypothesis test are reported as follows: *t*(21) = 2.38, *p* \<.05. What was the statistical decision and how big was the sample? - - - - - Learning Check 2 (2 of 2) - Decide if each of the following statements\ is **True** or **False**. - T/F - Sample size has a great influence on measures of effect size. - T/F - When the value of the *t* statistic is near 0, the null hypothesis should be rejected. - Learning Check 2 -- Answers (2 of 2) - False - Measures of effect size are not influenced to any great extent by sample size - False - When the value of *t* is near 0, the difference between *M* and μ is also near 0