Empirical Banking - Finance and Growth (PDF)
Document Details
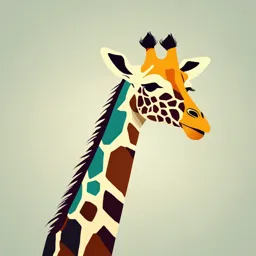
Uploaded by ProductiveThallium8177
Università degli Studi di Roma "Tor Vergata"
2024
Stefano Caiazza
Tags
Related
- Finance and Growth Introduction 2024-2025 PDF
- Finance and Growth: Theoretical and Empirical Definitions 2024-2025 PDF
- Empirical Banking: Finance and Growth (Goldsmith & Schumpeter) PDF
- Empirical Banking - Finance and Growth - 2024-2025 PDF
- Empirical Banking Finance and Growth Levine and Zervos PDF
- Finance and Growth (LLB, 2024-2025) PDF
Summary
This document provides an introduction to econometric tools for finance and growth, focusing on empirical banking. It details the design of an experiment, data sources, and various models such as cross-sectional data, along with examples.
Full Transcript
Empirical Banking Finance and Growth ECONOMETRIC TOOLS FOR FINANCE AND GROWTH Stefano Caiazza 2024-2025 Introduction Theory suggests that effective financial institutions and markets that help overcome market fr...
Empirical Banking Finance and Growth ECONOMETRIC TOOLS FOR FINANCE AND GROWTH Stefano Caiazza 2024-2025 Introduction Theory suggests that effective financial institutions and markets that help overcome market frictions introduced by information asymmetries and transaction costs can foster economic growth through several channels. Advances in computational capacity and the availability of large cross-country datasets with relatively large time dimensions have enabled researchers to rigorously explore the relationship between financial development and economic growth. Further, as more disaggregated datasets have become available, the finance and growth literature has proceeded from using country-level data, to using industry- and firm-level data, to more recently using household data. The design of the experiment The outcome of a paper is the result of a series of choices made by the researcher. First, the quality of the Research Question(s) and the literature review And then, the answer given to a series of problems (1) Time and spatial horizon; (2) Conditioning variables (literature); (3) Data source; Data processing (practical problem); Missing data (practical problem) Merge several datasets (practical problem); (4) Data analysis Outliers i) Unchanged data ii) Trimming iii) Winsorizing (5) Choice of the model (6) Robustness checks Data and Models (1) CROSS-SECTIONAL DATA. Data on different entities – workers, consumers, firms, governmental units, and so forth – taken at a given point in time (at the same time) are called cross-sectional data. Data on growth rates for different countries in a given year is another example of cross- sectional data. In this case, each row lists data for a different country collected on the same date The order of the rows is arbitrary, and the number of countries, called the observation number, is an arbitrarily assigned number that organizes data Analysis of cross-sectional data usually consists of comparing the differences among the subjects. Cross-Sectional Data obs_number code country_name growth btoti btot Data 1960-1995 (average) 1 ARG Argentina 0,618 63,8889 74,1596 2 AUS Australia 1,975 83,0754 92,6655 3 AUT Austria 2,889 97,318 98,4359 4 BGD Bangladesh 0,708 86,3752 5 BRB Barbados 2,653 91,8502 6 BEL Belgium 2,651 78,4184 92,0092 7 BOL Bolivia 0,355 10,2146 31,5974 8 BRA Brazil 2,930 47,4771 61,6758 9 CAN Canada 2,386 80,7844 88,997 10 CHL Chile 1,447 58,565 52,8085 11 COL Colombia 2,227 74,3253 80,1646 12 CRI Costa Rica 1,614 97,3479 72,8176 13 CYP Cyprus 5,384 93,0962 92,72 14 DNK Denmark 2,179 86,0352 88,0999 15 DOM Dominican Rep. 2,499 73,6196 73,3352 16 ECU Ecuador 2,388 64,7281 62,1616 17 SLV El Salvador -0,608 90,7292 72,0441 18 FJI Fiji 1,846 98,9383 96,9463 19 FIN Finland 2,798 95,8457 97,2227 20 FRA France 2,431 89,4408 96,5411 Country codes (WB&CIA) 21 DEU Germany 2,454 93,5074 97,571 https://wits.worldbank.org/wits/wits/witshelp/content/codes/country_codes.htm …. …. …. …. …. …. https://www.cia.gov/the-world-factbook/references/country-data-codes/ Data and Models (2) TIME SERIES DATA. Data for a single entity – person, firm, country – collected at multiple time periods. Because past events can influence future events and lags in behavior are prevalent in the social sciences, time is an important dimension in a time series data set. Unlike the arrangement of cross-sectional data, the chronological ordering of observations in a time series conveys potentially essential information. Another feature of time series data that can require special attention is the data frequency at which the data are collected. In economics, the most common frequencies are daily, weekly, monthly, quarterly, and annually. Stock prices can be recorded at daily intervals or at high- frequency data, transaction after transaction (https://www.truefx.com/streaming-market-data-truefx/). Data on growth rates for a single country is another example of time series data. For example, the data on growth rates for the US for 84 periods of time. The observations begin in the first quarter of 2000 – 2000:I – and end in the fourth quarter of 2020 – 2020:IV. Each period in this data set is a quarter of a year. The data in each row correspond to a different period (quarter). Time series data can be used to study the evolution of variables over time and to forecast future values of variables of interest. Time Series Data obs_number Date growth 1 2000:I 6.27% 2 2000:II 7.57% 3 2000:III 6.52% 4 2000:IV 5.51% 5 2001:I 4.68% 6 2001:II 3.43% 7 2001:III 2.71% 8 2001:IV 2.15% 9 2002:I 2.99% 10 2002:II 2.72% 11 2002:III 3.64% 12 2002:IV 3.76% 13 2003:I 3.62% 14 2003:II 3.91% 15 2003:III 5.60% 16 2003:IV 6.43% …. …. 81 2020:I 2.28% 82 2020:II -8.51% 83 2020:III -1.70% 84 2020:IV -1.00% Data and Models (3) PANEL DATA, Also called longitudinal data, are data for multiple entities where each entity is observed at two or more periods. Panel data require replication of the same units over time. For example, the growth rate of different countries over time. The number of entities in a panel dataset is denoted as N, and the number of periods is indicated as T. If we collect data for 78 countries (N=78) for 7 years (T=7), the total number of observations is 546 (N×T =78×7). Panel data can be used to learn about economic relationships from the experiences of the many different entities in the data set and from the evolution over time of the variables for each entity. (4) POOLED CROSS-SECTIONS DATA They present a structure similar to panel data, but entities change over time. For example, suppose that two cross-sectional household surveys were taken in the United States, one in 1985 and one in 1990. In 1985, a random sample of households was surveyed for variables such as income, savings, family size, etc. In 1990, a new random sample of households was taken using the same survey questions. By combining the two years, we can form a pooled cross-section to increase our sample size. Panel Data id1 id2 code country time var1 1 1 ARG Argentina 1991 0,03 1 2 ARG Argentina 1992 0,02 1 3 ARG Argentina 1993 0,01 1 4 ARG Argentina 1994 0,01 1 5 ARG Argentina 1995 -0,04 1 6 ARG Argentina 1996 -0,01 1 7 ARG Argentina 1997 0,04 2 8 AUS Australia 1991 0,03 2 9 AUS Australia 1992 0,04 2 10 AUS Australia 1993 0,01 2 11 AUS Australia 1994 0,02 2 12 AUS Australia 1995 0,02 2 13 AUS Australia 1996 0,01 2 14 AUS Australia 1997 0,02 …. …. …. …. …. 540 ZWE Zimbabwe 1991 0,00 541 ZWE Zimbabwe 1992 0,05 542 ZWE Zimbabwe 1993 0,02 543 ZWE Zimbabwe 1994 -0,02 544 ZWE Zimbabwe 1995 0,01 545 ZWE Zimbabwe 1996 0,00 546 ZWE Zimbabwe 1997 -0,02 Dummy Variable (right-hand side) A dummy variable takes on the value of one or zero (and only those values) depending on whether a specified condition is met. Yi = β 0 + β1 Di + ui Because Di is not continuous, it is not useful to think of β1 as a slope. It is convenient to refer to β 1 as the coefficient multiplying Di. β0 is the population mean when Di =0 (Because E(ui|Di)=0, the conditional expectation of Yi when Di= 0 is E( Yi|Di=0) = β0 ; that is β0 is the population mean value of the dependent variable when Di=0). β0 + β1 is the population mean when Di =1. Because β0 + β1 is the population mean of Yi when Di =1 and β0 is the population mean of Yi when Di=0, the difference [(β0 + β1 ) - β0 ] = β1 is the difference between these two means. In other words, β1 is the difference between the conditional expectation of Yi when Di=1 and when Di=0, or β1 = E(Yi|Di=1) - E(Yi|Di=0). Because β1 is the difference in the population means, it makes sense that the OLS estimator β1 is the difference between the sample averages of Yi in the two groups, and, in fact, this is the case. Dummy Variable (right-hand side) Yi = β 0 + β1 X i + β 2 Di + ui The dummy changes the intercept depending on the value of Di, but the slopes remain constant no matter what value Di takes. You can use a dummy variable to create a numerical variable for a qualitative one (male/female; yes/no; etc.) You can also create a dummy variable to obtain a list of observations. Pay attention to the dummy trap. The interaction term Suppose you want to study the impact of Financial Development on growth during the SARS-CoV-2 virus pandemic. How would you do it? (1) You can run your model conditioned to the pandemic period only, 2020-2021. What are the advantages and disadvantages? (2) You can use a dummy variable that takes value 1 in the pandemic years and zero otherwise. What are the advantages and disadvantages? (3) You can use an interaction term between the pandemic dummy (point 2) and the Financial Development. What are the advantages and disadvantages? A hypothetical dataset Suppose you want to study the impact of Financial Development (FD) in the United States since 2000, and you want to do a test on what happened during the pandemic period. Let's see what happens to the data in the three previous hypotheses (we do not consider control variables in this example). Hypothesis 1 (a) (b) (c) (d) (a) (b) (c) (d) obs Years Growth Financial obs Years Growth Financial Develpment Develpment 1 2000 0,0408 0,4897 1 2000 0,0408 0,4897 2 2001 0,0095 0,5017 leads to 2 2001 0,0095 0,5017 3 2002 0,0170 0,5035 3 2002 0,017 0,5035 4 2003 0,0280 0,5149 4 2003 0,028 0,5149 ….. ….. 20 2019 0,0229 0,5218 20 2019 0,0229 0,5218 21 2020 -0,0340 0,5457 21 2020 -0,0340 0,5457 22 2021 0,0567 22 2021 0,0567 23 2022 23 2022 A hypothetical dataset Suppose you want to study the impact of FD in the United States since 2000 and want to do a robustness check on the academy period. Let's see what happens to the data in the three previous hypotheses we do not consider control variables in this example. Hypothesis 2 Hypothesis 3 (a) (b) (c) (d) (e) (a) (b) (c) (d) (e) (f) obs Years Growth Financial Pandemic obs Years Growth Financial Pandemic Intercation Develpment dummy Develpment dummy term (d × e) 1 2000 0,0408 0,4897 0 1 2000 0,0408 0,4897 0 0 2 2001 0,0095 0,5017 0 2 2001 0,0095 0,5017 0 0 3 2002 0,0170 0,5035 0 3 2002 0,0170 0,5035 0 0 4 2003 0,0280 0,5149 0 4 2003 0,0280 0,5149 0 0 ….. ….. 20 2019 0,0229 0,5218 0 20 2019 0,0229 0,5218 0 0 21 2020 -0,0340 0,5457 1 21 2020 -0,0340 0,5457 1 0,5457 22 2021 0,0567 1 22 2021 0,0567 1 23 2022 1 23 2022 1 Estimation with two key variables Estimation with three key variables The interaction term An interaction term is an independent variable in a regression equation that is the multiple of two or more other independent variables. Each interaction term has its own regression coefficient, so the result is that the interaction term has three or more components. Such interaction terms are used when the change in Y with respect to one independent variable (in the case below, X) depends on the level of another independent variable (in the case below, D). Yi = β 0 + β1 X i + β 2 Di + β3 X i Di + ε i Interaction terms can involve two quantitative variables or two dummy variables, but the most frequent application of interaction terms involves one quantitative variable and one dummy variable. The interaction term allows the slope of the relationship between the dependent variable and an independent variable to be different depending on whether the condition specified by a dummy variable is met. This is in contrast to an intercept dummy variable, which changes the intercept, but does not change the slope when a particular condition is met. The interaction term Let’s check the slope of Y with respect to X in the presence of the interaction term: Yi = β 0 + β1 X i + β 2 Di + β3 X i Di + ε i When D=0, ∆Y = β1 ∆X ∆Y When D=1, = ( β1 + β3 ) ∆X The coefficient of X changes when the condition specified by D is met. The interaction term A critical question is whether or not to include all constitutive terms when specifying multiplicative interaction. By constitutive terms, I mean each of the elements that constitute the interaction term. Thus, X and D are the constitutive terms in the previous equation. The answer is: YES, except in very rare circumstances. The figure below illustrates a scatterplot of 500 obs generated by the process implied in the eq., where β0=2, β1=0, β2=2, and β3=2 Predicted values of Y from the fully specified model Y = β 0 + β1 X + β 2 D + β 3 XD + ε Predicted values of Y from the under specified model Y = γ 0 + γ 1 X + γ 3 XD +ν + Observations when D=1 - Observations when D=0 The interaction term In which circumstances is it possible to omit one of the constitutive terms? 1) The researcher must have a strong theoretical expectation that the omitted variable (D, for example) has no effect on the dependent variable in the absence of the other modifying variable (X = 0). Note, though, that the only situation in which this theoretical expectation can be justified a priori is if X is measured with a natural zero. This is because the coefficients on constitutive terms depend on how an analyst scales these variables. For example, using a measure of democracy such as the Polity score that is scaled from -10 to 10 in an interaction model will generate a different coefficient on the variable that it interacts with than the same measure of democracy that is scaled from 0 to 20. 2) The second condition that must be met before omitting a constitutive term is that the analyst should estimate the fully specified model outlined in Eq. (16) and find that β2 is zero. Note that even if β2 is statistically indistinguishable from zero, the other parameters of interest will still be estimated with bias to the extent that β2 is not exactly zero if the constitutive term is dropped. The use of Logarithm (variables transformation) If data are very skewed, the linear model in levels can provide very poor prediction. A typical example is medical expenditure data that are right-skewed. Log eliminates skewness. While the mean and the median will always be greater than the mode in a right-skewed distribution, the mean may not always be greater than the median. The use of Logarithm (variables transformation) Dependent and independent variable (double-log functional form) Log: To transform a non-linear relationship into a linear one Yi = e β X iβ eε → log (Yi ) = β 0 + β1 log ( X i ) + ε i 0 1 i ∆ ( log Y ) ∆Y / Y The coefficients express the elasticities βi = = and since regression coefficients are constant, ∆ ( log X i ) ∆X i / X i the model has constant elasticity. The way to interpret βi in a double-log equation is that if Xi increases by 1% while the other Xs are held constant, then Y will change by βi percent. Independent variable (semilog functional form) Yi = β 0 + β1 log ( X 1i ) + β 2 X 2i + ε i It’s a very common form. If β1 >0, the impact of changes in X1 on Y decreases as X1 gets bigger. Thus, the semilog functional form should be used when the relationship between X1 and Y is hypothesized to have this “increasing at a decreasing rate” form. A 1% change in X1 is associated with a change in Y of 0.01B1 Dependent variable (semilog functional form) log (Yi ) = β 0 + β1 X 1 + β1 X 2 + ε i The derivative of the log gives you the growth rate (prove it!) If X1 increases by one unit (∆X=1) , then Y will change in percentage terms (100β1%) Example CDS5Y≡Ln(CDS) CDS5Y≡Ln(CDS) Example Outliers An outlier is an observation that does not appear to follow the pattern of the other data points. From a practical perspective, outlying observations can occur for two reasons. 1) The easiest case to deal with is when a mistake has been made in entering the data. It is always a good idea to compute summary statistics, especially minimums and maximums, in order to catch mistakes in data entry. Unfortunately, incorrect entries are not always obvious. 2) Outliers can also arise when sampling from a small population if one or several members of the population are very different in some relevant aspect from the rest of the population. The decision to keep or drop such observations in a regression analysis can be a difficult one. OLS is susceptible to outlying observations because it minimizes the sum of squared residuals: large residuals (positive or negative) receive a lot of weight in the least squares minimization problem. If the estimates change by a practically large amount when we slightly modify our sample, we should be concerned. Outliers Is it correct to drop out tout-court the outliers without analyzing the phenomenon? No. For example outliers can be the result of incorrect functional shapes. Outliers One possibility of treating outliers is trimming or winsorizing your dataset. Trimming allows you to truncate data, ruling out observations with values lower or greater than a certain percentile. For example, you can rule out all observations with a value larger than the 99th percentile and lower than the 1st percentile for each variable of your analysis. Winsorizing allows you to replace data with a certain percentile. For example, you can decide to replace all observations with a value larger than the 99th percentile with the value of the 99th percentile and replace all observations with values lower than the 1st percentile with the value of the 1st percentile. Trimming reduces the size of your sample, while winsorizing keeps it. However, winsorizing changes your empirical distribution. Pre - estimates Trimming (2,98) Winsorizing / Replacing (2,98) Stata * Winsoring local var cds_5y esg roa stock lever size hml smb esmr tslope gdpg infl debtgdp tabstat $var, stats(N min p1 p5 mean p50, p75 p90 p99 max, sd) winsor2 `var', replace cuts(2 98) tabstat $var, stats(N min p1 p5 mean p50, p75 p90 p99 max, sd) * Trimming winsor2 `var', replace cuts(2 98) trim Post - estimates The general problem The econometrics of finance and growth can be summarized in the following simple regression model: (1) g ( i, t ) ≡ y ( i, t ) − y ( i, t − 1) = α + β f ( i, t ) + γ C ( i, t ) + µ ( i ) + ε ( i, t ) Where y is the log of real GDP per capita or another measure of welfare, g is the growth rate of y, f is an indicator of financial development, C is a set of conditioning information. i is the observational unit – be it a country, an industry, a firm or a household – and t is the period. ε is a white-noise error with a mean of zero, μ is a country-specific element of the error term that does not necessarily have a mean of zero. μ is called country-fixed-effect and it captures the time-invariant individual-specific effect. The explanatory variables are measured either as an average over the sample period or as an initial value. The sign and significance of the coefficient β is at the center of the debate. Standard Errors Heteroskedasticity arises when the variance of the error term is not constant: 𝑣𝑣𝑣𝑣𝑣𝑣 𝜀𝜀𝑖𝑖 ≠ σ2. Heteroskedasticity causes OLS to no longer be the minimum variance estimator. Moreover, OLS is no longer asymptotically efficient. ^ Heteroskedasticity causes the OLS estimates of the SE(β )s to be biased, leading to unreliable hypothesis testing and confidence intervals. One possible solution is to correct standard errors. While biased, corrected SEs are typically more accurate than uncorrected standard errors for large samples (White or Huber-White procedure). If the regression errors are autocorrelated, then the usual heteroscedasticity-robust standard error formula for cross-section regression is no longer valid. In this case, you can use clustered standard errors. The term clustered arises because these standard errors allow the regression errors to have an arbitrary correlation within a cluster or grouping but assume that the regression errors are uncorrelated across clusters. Clustered standard errors allow for heteroscedasticity and arbitrary autocorrelation. Heteroskedasticity-robust standard errors and clustered standard errors are valid whether or not there is heteroskedasticity, autocorrelation, or both in regression with cross-sectional data. Example Dependent: CAR(0,1) Model 1: no correction Model 2: robust (Hubert-White procedure) Model 3: cluster at firm-level Model 4: cluster at firm- and industry-level Stata * I define global control variables Global var size profit lever * Model 1 reg cds_5y co2 $var * Model 2 reg cds_5y co2 $var, vce(robust) * Model 3 reg cds_5y co2 $var, vce(cluster id) * Model 4 vcemway reg cds_5y co2 $var, cluster (id industry) Cross-sectional regressions The early finance and growth literature has used standard cross-country OLS regressions, with data for each country averaged over the sample period, including the lagged dependent variable as a control variable: (2) g ( i ) ≡ y ( i, t ) − y ( i, t − 1) = α + β f ( i ) + γ C ( i ) + δ y ( i, t − 1) + ε ( i, t ) Regression (2) has thus only a cross-country, but not a time series, dimension. The log of initial income per capita is included to control for convergence. Including other country characteristics, such as initial levels of human or physical capital, and policy variables, such as government consumption or trade openness, in a set of conditioning information allows testing for an independent partial correlation of finance with growth. Cross-sectional regressions OLS estimates, however, are consistent if the following orthogonality conditions hold: (3) E C ( i ) ε ( i ) = 0; E y ( i, t − 1) ε ( i ) = 0; E f ( i ) ε ( i ) = 0 ' ' ' Endogeneity is a violation of one (or more) of these conditions and can arise for several reasons. Variables correlated with the error term are called endogenous variables, while variables uncorrelated with the error term are called exogenous variables. First, the presence of an unobserved country-specific effect μ(i) – as in regression (1) – results in a positive correlation of the lagged dependent variable with the error term as, unlike the error term ε(i), μ(i) does not have a mean of zero, so that: (4) E y ( i, t − 1) µ ( i ) + ε ( i ) ≠ 0 ' Omitted variable bias can also arise if other explanatory variables are correlated with the unobserved country-specific effect or if explanatory variables that should be included in regression (2) are: (i) not included; and (ii) correlated with included explanatory variables, so that: (5) E C ( i ) ε ( i ) + ε ( i ) ≠ 0 ' Omitted variables 𝑦𝑦 = 𝛽𝛽1 + 𝛽𝛽2 𝑋𝑋2 + 𝛽𝛽3 𝑋𝑋3 + 𝜀𝜀 true model 𝑦𝑦 = 𝐵𝐵1∗ + 𝐵𝐵2∗ 𝑋𝑋2∗ + 𝜀𝜀 ∗ estimated model 𝜎𝜎23 2∗ = 𝛽𝛽2 + 𝛽𝛽3 𝐸𝐸 𝛽𝛽 where 𝜎𝜎23 is cov(x2,x3) and 𝜎𝜎22 is var(x2) 𝜎𝜎22 σ23>0 σ230 positive distorsion negative distorsion β3