4. Contrast Categories & Concept Hierarchies copy.docx
Document Details
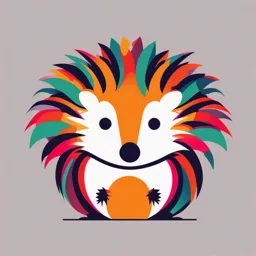
Uploaded by NoiselessPeace
Full Transcript
CRITICAL READING: CORNELL NOTES Contrast Categories & Concept Hierarchies Name: Date: 27 August 2023 Section: Lecture 4 Period: Questions/Main Ideas/Vocabulary Notes/Answers/Definitions/Examples/Sentences Category Learning Experiments Show that category structure influences the sp...
CRITICAL READING: CORNELL NOTES Contrast Categories & Concept Hierarchies Name: Date: 27 August 2023 Section: Lecture 4 Period: Questions/Main Ideas/Vocabulary Notes/Answers/Definitions/Examples/Sentences Category Learning Experiments Show that category structure influences the speed and success of categorisation decisions. More complicated categories take longer to learn. In some ways, these category structures are much simpler than real-world categories. They only vary along a limited number of dimensions. The dimensions are generally quite simple perceptual qualities. In some ways, these category structures are much more complicated than real-world categories. Ameel, Malt & Storms (2008) Compared the naming patterns of children aged 5-14 with adults, for common household objects (bottles, flasks, jars and dish, plate and bowl). Bottle set = 73 pictures. Dish set = 67 pictures. Presented with each picture in turn and asked to name it. The set of objects called fles (bottle) starts of very broad (over-extension) and gradually converges to the adult usage. Same with kom (bowl). As the children get older, new words are added to their vocabulary. The pattern of change doesn’t just reflect the addition of new words, but also a reorganisation of the categories that extends beyond the acquisition of new words. Learning Real-World Categories Take a long time to learn effectively. Change over time. Over-extended words (fles) narrow over time. Under-extended words (bus) broaden over time. Are culture, person and time specific. Have a much richer structure than the types of categories used in lab-based category learning tasks. Hierarchical Concept Structure Our conceptual representations often follow a hierarchical structure. The hierarchy can be described in terms of three levels: the subordinate, basic and superordinate. Items located at the same level of abstraction are called ordinates. The implication is that basic levels categories are generally the most useful: Because of the high level of abstraction, superordinate categories lack informativeness. Subordinate categories are highly informative but lack distinctiveness. Basic level categories appear to contain the best balance between informativeness and distinctiveness. Categories within taxonomies of concrete objects are structures such that there is generally one level of abstraction at which the most basic category cuts can be made. In general, the basic level in a taxonomy is the level at which categories carry the most information and are thus, the most differentiated from each other. Rosch, Mervis, Gray, Johnson & Boyes-Braem (1976) Asked people to list features for objects at all three levels. Very few features were generated for superordinate categories. Presumably, this is because these categories are fairly abstract (what are the features of furniture?). Most of the features generated were at the basic and subordinate level. Most of the subordinate features overlapped with each other (all chairs tend to share many features in common, whether they’re desk chairs or garden chairs or dining room chairs). Rosch et. al (1976) Asked participants to name pictures of objects drawn from a range of categories. Basic level names: 1595. Subordinate level names: 14. Superordinate level names: 1. The utility of category abstraction varies as a function of expertise: Tanaka and Taylor (1991) compared naming patterns of dog experts and bird experts. They hypothesised that the two groups would use subordinate names more regularly in their expert domain. Efficient Thinking Requires Choosing the Right Level of Abstraction We are more likely to say ‘look at that rabid dog’ than ‘look at that rabid sausage dog’, or ‘look at that rabid animal’. Other times, it makes sense to be more abstract. We say ‘Today I went to the zoo and saw lots of different animals’ rather than listing all the different animals you saw. Hierarchical Organisation Can Optimise Cognitive Economy Because a cat is a mammal, we can infer it has fur and lactates. We don’t need to explicitly associate these features with cat. Contrast Categories Our conceptual representations appear to have graded category structure. A robin in a typical bird. An emu is an atypical bird. Graded category structure appears to be due to the inter-item similarity relationships of the category members. Family resemblance/polymorphous concept predictions. Distance from centroid/average distance in MDS space. Rosch and Mervis (1975): Category structure should also be influenced by contrast categories. Contrast categories are categories that exist within the same domain at the same level of abstraction, and influence each other’s similarity structure. Fruits and vegetables are contrast categories within the domain food. Birds and mammals are contrast categories within the domain animals. Typical category members have high similarity to other members of their own category, and low similarity to members of contrast categories. A bat is an atypical member of the category mammals, as it has low relative similarity to other mammals, but a high relative similarity to members of contrast categories such as birds or insects. Verbeemen et. al (2001) Despite Rosch & Mervis’s plausible reasoning, there has been very little empirical support for the existence of contrast category effects. Verbeemen et. al applied the polymorphous concept model to the prediction of typicality and response times. The idea was as follows: Category members that have a high degree of overlap with the features representing their category should be highly typical. Category members that have a high degree of overlap with the features representing the contrast category should be high atypical. Typical vegetables should have lots of vegetable features and few fruit features. As could be expected from previous research, they found that within-category predictions were mostly significant and in the right direction. However, they found that the contrast-category predictions yielded few significant correlations. And that when the correlations were significant, they tended to be in the wrong direction. Dry & Storms (2011) Argued that the failure for previous studies to find contrast category effects is that the models don’t take distinctive feature information into account. Categories at the same level of abstraction within a common domain are likely to share particular features (we can assume that mammals, birds, etc, all share features relating to animal-ness). Therefore, it is the distinctive features which would allow for the greatest degree of differentiation between the categories within the same domain. In order to test this, we generalised the family resemblance and polymorphous concept models to take both common and distinctive feature information into account. We applied the models to the prediction of typicality gradients for categories within the domains animals and artifacts. The ratio of common to distinctive information employed by the models was controlled by a parameter θ. When θ was equal to 1, only common features were used, and when θ was equal to 0, only distinctive features were used. Found significant evidence of contrast category effects. Typical exemplars within one category would appear to have more features that are distinctive to their respective category (features that tend not to be found in the contrast category), and fewer features that are distinctive to the contrast category (features that tend not to be found in the target category). Common features tend to pull categories together; distinctive features tend to help delineate the borders of categories. The Contrast Model According to the contrast model, the similarity between the exemplars i and j can be calculated from the features common to the two exemplars, the features of i that aren’t present in j and the features of j that aren’t present in i. The family resemblance and polymorphous concept models are both special cases of the common features of the contrast model. These models can be generalised to include both common and distinctive feature information. Typicality predictions based upon within-category feature data are optimal when θ approaches 1 (the data weighted in favour of common feature information).