4. Basics of Classifcation (Hypothesis Space, Bias, Generalization, Evaluation).pdf
Document Details
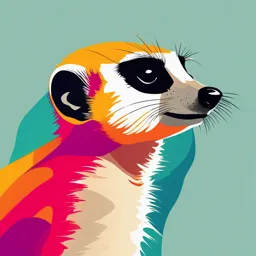
Uploaded by FinerLimeTree
University of Southern Denmark - SDU
Tags
Related
- classification-criteria-igg4-related-disease-complete-article-2019.pdf
- Classification of Partially Edentulous Arches PDF
- Lecture 5 Remote Sensing Image Interpretation PDF
- COMP9517 Computer Vision 2024 Term 2 Week 4 Pattern Recognition Part 2 PDF
- 04-sl-overview-handout.pdf
- chap02.concepts_apprentissage.pdf
Full Transcript
Classification - Supervised Learning ► For some domain V and a set of classes c = {c1..... q }, k 2: 2, each o E 'D belongs uniquely to some c E C, i.e., there is a function/: V-+ C (see Slide 55). ► Given a set of objects o = { u 1. 02..... u n } -. Water X Forecast = T'...
Classification - Supervised Learning ► For some domain V and a set of classes c = {c1..... q }, k 2: 2, each o E 'D belongs uniquely to some c E C, i.e., there is a function/: V-+ C (see Slide 55). ► Given a set of objects o = { u 1. 02..... u n } -. Water X Forecast = T' X Positive/negative example An example x is positive, if f(x) = Yes, negative otherwise. (Note that we knowf on the training data only.) Hypothesis A hypothesis defines a subset of D. We write a hypothesis as vector containing ► specific values for attributes ► wildcards ('?') indicating that for some attribute any attribute value is acceptable ► (J indicating t hat no attribute value is acceptable. Coverage of Data Instances by Hypotheses ► For example the hypothesis (Sunny. :. 1. ·t. Cool. 1) defines all examples where Sky=Sunny and Water=Cool. ► If we are interested in hypotheses about positive ·n examples, we can interpret (Sunny. ·t.·:. t. Cool. as a rule: if Sky=Sunny and Water = Cool then EnjoySport=Yes ► An example x satisfies a hypothesis h, if and only if f(x) = Yes. The Assumptions of a Learning Algorithm Define the Hypothesis Space ,ji.. iii_ A.. · ;',_ 1 ' I.. "1.:fl,;. I', 1" ,I( !I! 11 1 (Sunny.?,?, Strong, 0 , ?).t1 - {Sunny, Warm, High, Strong, Cool. Same) h (Sunny.·!,·:.. ?.·;, ·n x, - (Sunny, Warm, High, Ligl1t, Warm, Sarne) h_ - (Sunny. ·t 1 ?, ?. Cool.'!) Definition 5.1 For any two hypotheses, hi and h k , over X, h1 is more general than or equal to h k if and only if: \/x EX: hk(x) =Yes ⇒ h j (.i:) = Yes Basic Algorithm Algorith m 5. 1 (Find-S [M itchell, 1 997]) 1. Initialize h to the most specific hypothesis in H 2. For each positive training instance x For each attribute constraint a; in h If the constraint o; is satisfied by.r Then do nothing Else replace a; in fl by the next more general constraint that is satisfied by x 3. output hypothesis h Discussion : ► Finds the m ost specific hypothesis consistent with the positive examples in the training data - is it the only consistent hypothesis ? ► W hy sho uld we prefer more specific hypotheses over more general ones ? Possible H ypoth eses U nd e r the Ass u m pti o n of a Conj u n ctive Co n cept The following conjunctive hypotheses describe the concept "Yes" correctly for the training data : \IS unny, ·.1. "!.. ·..1.· 1. ?. ) \I S unny. ·.1. ·.1. St rong.."I , "!.) /\ ?· , warm..-,.. ?.. ·). , ·.) ) \n· , Warm, ?., St rong...-,,..? ) 1\ S unn y , warm , ?., ?. ,.? ,.·) ) ,sunny, Warm, ? , Stro ng, ? , ? ) Reduce the Bias? For some other training data, our model assu mptions seem too strict: ► No consistent hypothesis is possible under our ass umptions : ► the most specific hypothesis for positive examples is (? , War m , Normal , Strong, Cool, Ch ange) ► this hypothesis covers also the neg ative example Bias-free Learning ► Sho u ld we allow for more complex/generous assumptions to reduce the bias (i.e., to get rid of restrictions of the hypothesis space) ? ► Allow di sjunctions ? Negations? ► A disjunctive hypothesis "if Sky=Sunny or Sky=Cloudy, then Yes" can list all positive examples. ► We could actually cover any concept with an arbitrarily complex hypothesis. ► Is th is what we want?