W7 Content PDF
Document Details
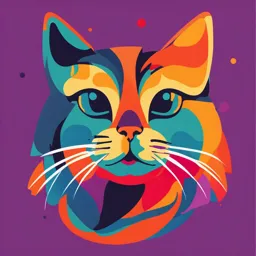
Uploaded by SweetheartClematis3453
Australian Catholic University
Tags
Summary
This document provides an overview of quantitative research study designs, including pseudo-randomized controlled trials, comparative studies, and case series. It also discusses important considerations for interpreting these studies, including potential biases and limitations.
Full Transcript
6.1 Quantitative -- but not an RCT Â **Learning Objectives** By the end of this week you will be able to: - Determine NHRMRC Levels of Evidence for all quantitative study designs - Appraise the utility of small n research designs - Identify appropriate means of graphical representati...
6.1 Quantitative -- but not an RCT  **Learning Objectives** By the end of this week you will be able to: - Determine NHRMRC Levels of Evidence for all quantitative study designs - Appraise the utility of small n research designs - Identify appropriate means of graphical representation of data - Demonstrate basic Excel skills in calculating and representing data   **NHMRC Levels of Evidence: Levels III and IV** - Pseudo-randomized controlled trial (III-1) - Comparative study with concurrent controls (III-2) - Comparative study without concurrent controls (III-3) - Case Series with pre-/post-test outcomes  Are they worth your appraisal? The answer is... YES!    **Level III & IV Evidence : NHMRC** Level III: What are Pseudo-Randomised Controlled and Comparative Studies?    **The Pseudo-Randomised Controlled Study Smith, Morrow & Ross (2015)**   Diagnosis: Test accuracy - Concurrent validity (when a standardised test is tested against another standardised test that we know works)  Alternate allocation (random ) Issues: Not blinded- for test accuracy , uneven grouping  N of 2 Communities: Matched pair and constrained randomisation (eg Public health)  **Allocation bias!** Multiple group case series Figure 8.3, Polgar and Thomas (2020)   - Trying to make sure community A and B are as similar as possible - Community A- didn't introduce program and community B was exposed to control program - Problems in allocation of group series  **What are Comparative Studies?** Time-Series Cohort - Calles quasi experimental designs\> especially interventions - Independent variable is the exposure of something to the group that either does or doesn't have a disease  Case Control - Time series cohort- starts at a period of time and moves forward, retrospective\> useful for groups that already have an impairment... e.g. we don't know who is and isn't going to get cancer - Look at the grouping- one group people who have the condition and other group people who don't have the condition - Look at other variables in society that could be linked to the condition - Case control- good for people who already have a problem or condition and is therefore useful for epidemiology and aetiology study  Historical control (III-3) - Reviewing a particular cohort and measure against controls that we already have information about at another time - Lower level of evidence than an RCT, however were looking at a group of people wth an intervention and comparing it to another intervention that is a gold standards - looking at types of outcomes... ensure new group of participants are very similar to the control group established in the other study   *Epidemiology* *Causal Relationships* - *Note: Both addressed in the graphic below* - *Vital in COMPARITIVE studies*      RCT: people who are or not exposed to particular factors- outcome overtime... start now and move forward in time Case CS: looking back at a case controlled study with factors that were present of absent for people who have or don't have the disease  Cross section sudied- study of people observed at a single point in time  **What to check for...** **Cohort and Case Control studies** - Are comparison groups well described/defined? - Were measures of exposure and outcomes collected in the same way (preferably blinded) for each cohort? - Were all possible confounds identified? - What was the follow-up period? - If you are using historical controls, what differences are there in possible confounds?  **Pseudorandomised**\> - Appraisal: allocation bias is likely  **Comparative studies**: failure to clearly define the comparison groups. Are the comparison groups well defined or described in similarity  **Measures of exposure or outcome:** are they collected in the same way and or blinded in the cohort study  - Possible confounds: if there are no confounds in the study than something is wrong... - Follow up periods: was there enough time of a follow up period in cohorts. What happens to them as time progresses in perspective of the independent variable. - Historical controls: possible confound of historical controls and the experimental groups of current date cohort.    6.2 Are 'lower level' studies useful? **What are the Cons?** - Small n → cannot be generalised to larger populations - Sampling Bias\> small sample, bigger application to larger samples - Maturation Bias\> small study... likely to be a similar type of people - Investigator/clinician blinding not possible, no control - Measurement/Observation Bias \> problem: small amount of people and investigators know who they are - Client blinding not possible - Hawthorne effect\> occurs when people are in a study because they know if they are in research... expecting something good to come out of it - Note: rates of satisfaction  - Smaller number= smaller power - Do authors address there is bias or are they trying to minimise boas - Small studies- no control= backbone that RCTs are based on    - Different research questions ask for different designs - Risk factors with cohorts, surveys- accuracy of symptoms, frequency or prevalence- emotions etc. RCT- does this intervention work for greater populations  **Why not RCT?** - Some variables unchangeable (eg age, cancer, gender) - Ethical reasons (eg withholding Tx) \> experimental arm and no-treatment control (seen as withholding therapy from someone- may drop out) - Expensive \> tools to analyse statistics, very big to have power to prove effectiveness in treatment - Different research questions need different designs - RCTs measure change -- they rarely explain change \> dony explain why it occurs - RCTs measure populations -- dont address individual differences - RCTs -- biomedical model, not psychosocial  \"Absence of a randomised trial is not absence of evidence\" (Rosenbek, 2016) - Need to collect evidence to try and account for those changes we have to make in clinics  [PCTs are not cased on a psychosocial models because in allied health we are more concerned with behaviours rather than treatments and cures ]  **Smaller n Studies and Survey Designs** Case Series Surveys Single Case Experimental Designs  **Case Series (Level IV, NHMRC, 2008)**  **What?** - AKA clinical experiment (intervention or independent variable) - One participant group ('cases'), no controls - Prospective, with protocol \> protocol in collecting the outcome (blind raters- people who are blind and rate outcomes). What have they done to minimise biases?  **Why**? - 'Hypothesis-generating' (Hoffman et al., 2024) \> good idea that a modification to treatment to try and reduce the confounds as much as possible - **Pilot study**\> smaller studies guide bigger studies - Useful in targeting specific populations/quality improvement - Useful for complex populations 'Understanding unusual patient conditions' (Portney & Watkins, 2015) e.g. population with high comorbidities  **Surveys** What**?** - Questionnaires \> questioning to address quality improvement - Interviews - Can include open ended questions to collect qualitative data  Why? - Quantifies attitudes, beliefs - Characteristics of populations \> what are common wants, needs, barriers - Demographic characteristics  **Single Subject Experimental Designs (SSEDs) or Single Case Experimental Designs (SCEDs)**   - Experimental designs of study - Powerful for studies that only have one person: what makes them different? - Rigorous structure that involves an AB multiple design - Baseline period- no treatment and then go into a baseline period (with drawl design)  **Single Subject Experimental Designs (SSEDs) or Single Case Experimental Designs (SCEDs)** Multiple SSEDs = Meta-analysis Focus on specific dependent variable \> only looking at a very narrow thing and use small element to make change Protocol minimises other confounds 'Experimental' means not a case study \> unbiases scheme of cause and effect Empirical research SCED guidelines: The Single-Case Reporting Guideline In Behavioural Interventions (SCRIBE)  **What is a Case Study? Is it OK?** - AKA 'Phase I' study - Detailed observations - Behaviours and outcomes measured quantitatively - Natural, real-life \> done in clinics and community - ++variables = causal effects unknown - Good for complex clients/rare conditions - Good to observe **treatment processes**  6.3 Creating Useful Graphics: Building Your Excel Skills **Important Concepts and Skills for ALHT211** - How to create basic graphs, tables and charts - How to consider the right visual for the data  - Quantitative (**descriptive** & inferential) - Qualitative Data (themes)         **Presenting data** - **Bar graphs** can show nominal data (variables with qualitative characteristic e.g., ethnic group, gender), categorical data, or percentages as shown in figure 1. - The length of the bars are proportional to the frequency or percentage of the category. - You should be able to see precise values for the bars on the axis.   **Presenting data** - Histograms typically display the differences in frequencies or percentages among categories of interval/ratio variables. - The width of the bars are proportional to the width of the category and height proportional to the frequency or percentage of that category. - The histogram can be used to display the relative frequencies. - It can also show the nature of the **distribution** of the data; i.e., whether the data are **normally distributed.**    **Presenting data** - Pie charts or pie graph stypically show the differences in frequencies or percentages among categories of nominal or ordinal variables. - The pie "slices" add up to 100% of the total frequencies   **Scatterplot** The scatterplot shows the distribution of two variables simultaneously. The relationship (or correlation) between two continuous variables is estimated.    **Presenting data** - **Boxplots** display two common measures of the variability or spread in a data set. Range: largest value minus smallest value, and IQR (interquartile range) : Q3 minus Q1. - The bottom whisker goes from Q1 to the smallest non-outlier in the data set, and the top whisker goes from Q3 to the largest non-outlier. - The body of the boxplot consists of a \"box\" (hence, the name), which goes from the lower quartile (Q1) to the upper quartile (Q3). - If the data set includes one or more outliers, they are plotted separately as points on the chart. - Within the box the line is the median of the data set. Two vertical lines, called whiskers, extend from the top and bottom of the box.   