Transportation Supply and Demand Lecture 2 PDF
Document Details
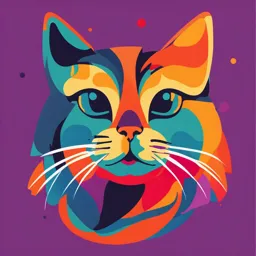
Uploaded by WittyPun
Tags
Summary
This document is a lecture on transportation supply and demand. It discusses the concepts of supply and demand in transportation systems, as well as different types of transportation models, including service and demand models as well as flows. The models and theoretical frameworks presented in this document should aid in basic transport planning and network analysis at a university level.
Full Transcript
Transportation supply and demand Lecture 2 Prediction of flows Flow prediction in TAF system Supply and demand function existence of equilibrium Transportation - demand Transportation - supply Equilibrium Service and demand functions Specifying T establishes...
Transportation supply and demand Lecture 2 Prediction of flows Flow prediction in TAF system Supply and demand function existence of equilibrium Transportation - demand Transportation - supply Equilibrium Service and demand functions Specifying T establishes a Service function, J S = J(T, V) Specifying A establishes a Demand function, D V = D(A, S) S => Level of service (TT, C, etc) V => Vol of travellers (Pax, Cars, etc) Flow Pattern, F consists of equilibrium volume, V0 using the system and level of service, S0 0 0 F = (V ,S ) Equilibrium Service and demand curves Existence of simple equilibrium Equilibrium between supply and demand Transportation intervention - Improvement in service If transportation system To is improved to T1 e.g. by adding traffic lanes. The service attribute S will also change and there will be equilibrium shift. Introduction of a new facility Example Service function Demand Function Flow pattern and Equilibrium Solution Determine F = (V, S) algebraically. Determine F = (V, S) using the graphical method Solving the problem gives V = 2,000 V/hr and t = 30 minutes. [ F (2000, 30] Consider a new service function t = 10 + 0.005V and solve the problem for the same Demand. Activity/system shifts (relations 2 and 3) These are shifts due to changes in both T and A. (T1, A1) [F1 = (V1, S1)] A11 Effect of activity-system changes Introduction of a new road – 10 yrs Prediction – Broader view Resource requirements Basic prediction models Service models i.e. S = J(T, V) Demand models i.e. V = D(A, S) Equilibrium models (Short term equilibration) Resource models – Resource requirements Activity shift models - long term changes as a consequence of short term flow patterns Basic prediction models Core of TSA Establishing relationship between TAF Project changes in Activity Propose changes in Transportation Predict resulting Flows And the associated Impact Google earth flow projection NexTA Model flow projection TransDNA flow projection TransCAD assignment model Overview of travel demand modeling The demand for transportation Lecture 3 What is demand for transportation? Transport demand is an inherent desire for movement of people and goods. People travel in order to satisfy a need (e.g. work, education, recreation, cultural, medical, etc.) and goods are transported as part of an overall economic activity within a setting so as to improve our status and capacity to fulfill our desires. Demand modeling Demand modeling involves studying the behavior of individuals in making decisions regarding the use of transportation services. Unlike other engineering models, demand modeling tools consider many disciplines like economics, psychology, geography, sociology, and statistics. Demand is a “derived demand” Demand for transportation is a derived demand, and not a need in itself. Users of transport are primarily consuming the service not because of its direct benefits, but because they wish to access other services. In other words, people travel not for the sake of travel, but to participate in activities at different locations – e.g. work, shopping, recreation, education, partying, tourism, etc. Demand is a “derived demand” Example, work-related activities commonly involve commuting between the place of residence and the workplace. There is a supply of workers in one location (residence) and a demand of labour in another (workplace). Transport (commuting) is directly derived from this relationship, hence it is a derived demand. Activity – derived demand Demand management - Levels of choice I. Activity patterns – People desire to undertake certain social patterns to ensure a full and satisfactory lifestyle; II. Locational choices– People need to be at particular locations; III. Travel patterns – People therefore eventually make decisions on when and how to travel. Transport demand management Class quiz Select 5 purposes of travel and answer questions for the conceptual map. Transport service variables (S) These are attributes of the transportation system that determine the level of service and hence influence the consumers decisions as to: – Whether or why – Where – When, and – How to make a trip. Examples of Services Variables Travel time (TT) – Out of vehicle travel time (OVTT) or waiting/walking time – In vehicle travel time (IVTT) Travel cost (TC) Comfort Reliability Safety or security etc. Travelers' behavior modeling - steps Explicit formulation of preferences; Identify available alternatives; Identify the attractors of each alternative; Evaluate the alternatives; Choose among the alternatives. Decision process - Concept of indifference Considers all the service attributes and their relative importance to the customer; Indifference curves indicate all combinations of service attributes or choices among which the consumer is indifferent. Indifference curve – travel cost and time An indifference curve is a graph showing combination of attributes that give the consumer equal satisfaction and utility. Each point on an indifference curve indicates that a consumer is indifferent between the two and all points give him the same utility or level of satisfaction. Indifference curves – travel cost and time Each point on the curve gives a bundle of values of the two service attributes Indifference concept Consumer is indifferent between either A and B OR C and D. Consumer has preference for A or B over C or D. Indifference curves may be expressed in functional form as a utility function. U = f(S, θ) S = vector of service attributes θ = Vector of parameters Consumer utility, Uni Uni is the utility (or net benefit or well-being) that individual n obtains from choosing alternative i; The behavior of indivuduals is such that utility is maximized; An individual n chooses the alternative that provides the highest utility. Forms of utility functions Product form U = αtβCγ Linear form U = αt + βC A utility value U is used to give combinations of t and C that satisfy the consumer. Different curves correspond to different values of U. Indifference curve forms t t C C Product form Linear form Properties of ICs The four properties of indifference curves are: 1. indifference curves can never cross, 2. the farther in an indifference curve lies, the higher the utility it indicates, 3. indifference curves always slope downwards, and 4. indifference curves are always convex. Utility value U = a measure of the degree of desire by the consumer for a particular combination of service attributes (e.g. t and C) U = positively valued If UA > UB, then the consumer prefers A to B. Mode choice modeling Lecture 4 Mode choice Mode choice This is a discrete choice problem where individual choice behavior is modeled econometrically using the principle of utility maximization. Individuals are modeled to choose the mode with the highest utility when confronted with a set of alternatives. Modeling mode choice is an essential element of the travel demand modeling framework. For trips made by a sample population, mode choice models predict the share of trips performed by each mode available. Basic relations of a transportation system Review - Impact prediction relationships Relation 1: Prediction of flows using the demand and supply relations – short term equilibration (T, A) F In other words, there is a transportation market in which service and demand reach equilibrium, thus establishing the flow pattern F = (Vo,So) This is called market - travel equilibration. Review – Impact prediction relationships Relation 2: The effect of current flow pattern in causing, over time, changes in the activity system. These changes in the activity systems take place as transport programmes are implemented. They include changes like population growth, increased income, car ownership, changes in economy. This is called activity - system equilibration. (T’, A’) [F’ = (V’,S’)] A” Long-term equilibration. Review – Impact prediction relationships Relation 3: The way the actual flows influence decisions of the system operators concerning transportation options with which they are concerned. It informs the operators on when and how to adjust these options of the transportation system. They include changing the vehicle schedules, fares, technological systems, vehicle quality, terminal efficiency, etc. Review – Impact prediction relationships Five models are required to predict these impacts. – Service models (For specified T options, determine S at various flow volumes V) – Demand models (For specified A options, determine V and its composition at various levels of service S) – Resource models – Determine resources consumed in providing the required LOS with specified T/A options – Equilibrium models – Predict actual flows (Fo) in a T for particular J and D functions – short term equilibrium – Activity - shift models – Predict long term changes in spatial distribution and structure of the activity system as a consequence of F – Feedback effect of transportation on land use and other elements of A. Demand and supply relations - I Demand functions V = 5000 – 100t Lower social economic status V = 7500 – 150t Higher social economic status Constant – Potential demand irrespective of level of service. Gradient – Sensitivity of volume of travel to level of service. Demand and supply relations - II Supply functions t = 10 + 0.01V Worse level of service t = 10 + 0.005V Better level of service Constant – Free flow travel time Gradient – Effect of congestion on travel time. Impact of transportation on SE activity Roads attract settlements at intersections; Small cities grow in a radial pattern and later circumferentially; These increase the activity patterns hence development of areas. Activity patterns in an area Activity patterns are characterized by: Employment – type of work, income and location of the work area. Residence – location of home, type of home, type of neighborhood, etc. Social amenities – schools available, access to shopping, interactions with neighbours, rent, mortgage rates, etc. Levels of choice - review Level Description Life style aspirations Each consumer has a way that is satisfying in life Desired activity Hence he will like to undertake particular activity patterns patterns Locational choices He therefore wants to be at particular locations at certain times e.g. residence, work place, cinema, etc. Travel choices This will drive the choice about when and how to travel there. Demand is derived The aforementioned choices lead to the demand or desire to travel. Hence demand is a “derived demand”. In other words, the demand to travel derives from lifestyle aspirations that force an individual to undertake particular activity patterns which requires specific locational choices leading to decisions of where, when and how to travel. Choice of a single consumer – Utility based The choice of travel depends mainly on three major attributes of transportation, namely: Travel cost (c) In-vehicle travel time – IVTT (t) Out-of vehicle travel time – OVTT (x) The utility a customer uses to make choices is given by:- U = Wtt + Wxx + Wcc Wt, Wx and Wc are the relative weights a consumer attaches to the respective service attributes. Consider 2 competing freight modes Wt = -$2/day/ton Wx = -$4/day/ton Wc = -1 UR = -$13 and UT = -$10.2 Hence UT > UR and the consumer selects truck in preference to rail. If there are different consumers – e.g. shippers Different consumers faced with similar choice use different weights on service attributes to evaluate the available modes. Consider different shippers for the previous example. The choices are as indicated in the table below, with A and B selecting Truck (UT > UR), while C and D select rail since (UR > UT). Effect of change in level of service Change in LOS might cause a consumer to shift from one mode to another. This shift normally occurs at a point where UR = UT. If the cost of truck, cT is considered: cT for modal shift between rail and truck Effect of truck rate cT on modal choice for A Effect of cT on modal shift for the shippers The multiple consumers (A, B, C and D) Trade – offs among service attributes This is the process of compensating a change in service attribute by using another attribute to prevent modal shift of consumers. If the operator senses an increase in fares because of other factors, he might consider reducing the travel time or frequency to prevent modal shift. Reading assignment Aggregate behaviour Effect of change in socioeconomic characteristics e.g. income. Effect of change in service attributes. Stochastic behaviour The previous utility model assumes that an analyst has perfect information on all available alternatives, their attributes and static consumer behaviour; This model is therefore static and unrealistic because it does not allow for changes over time; Realistically, we need models allowing for biases, limited perceptions of consumers making decisions and time-varying behaviour; Hence the need for stochastic models. Randomization – Model II This model assumes that consumers have no perfect information; The utility function for mode m is expressed as Um = α + βtm + μcm + ε where ε is an error term to cater for uncertainty; We therefore must consider the probability that an individual i will choose modal alternative m; Models for individual or household behaviour with explicit randomness are termed as stochastic disaggregate models. Randomization If m = 1, 2 i.e. two competing modes The probability that the consumer chooses mode 1 is given by: P1 = Prob(U1 > U2) Correspondingly P2 = Prob(U2 > U1) Logit models (Logistic models) Assuming that ε is a random variable that is independent and identically distributed following a Weibull distribution. Then P1 and P2 are given by: Since there are two modes, these are called binomial logit models (BLM). Multinomial Logit models If there are M alternative models, where M > 2. This is called the multinomial logit model (MNL). Multinomial Logit models Probabilistic choice Class exercise - 1 Solution - 1 Class exercise – 2 Class exercise - 2 – cont. Solution Mode choice modeling – disaggregate/aggregate Lecture 5 Disaggregate prediction of behavior Disaggregate versus aggregate data Disaggregate data refers to Aggregate data is when the isolation of one or more multiple data sources are variables within a data set, combined into one set to highlighting specific features create a larger idea of a or populations and leading particular issue to different insights. Aggregate data provides a Disaggregated data allows high-level overview, for a deeper dive into revealing general trends. specific groups or details. Aggregated data It is collected from multiple sources and presented as a whole, often for statistical analysis or to preserve individual confidentiality. Purpose of aggregation is to: Gain general insights into specific groups based on variables like age, profession, or income. Preserve individual confidentiality by combining data. Facilitate statistical analysis and summary reporting. Examples: Uganda census, Kampala incidence study on reported child abuse and neglect, etc. Disaggregated data Itis derived from aggregated data and broken down into smaller units to highlight specific issues or trends. Purpose of disaggregation is to: Identify vulnerable populations or hidden trends that may not be apparent from aggregate data. Establish the scope of a problem and make vulnerable groups more visible to policymakers. Delve deeper into specific subsets of results or outcomes to inform policy and service development. Examples: Disaggregating data by gender, urban/rural location, income, socio-cultural or ethnic background, language, geographical location, political/administrative units, or age groups to provide more detailed insights. Disaggregate modeling Disaggregate models Disaggregate models explain the travel behaviour of individuals or households directly. Therefore, data are used at the disaggregate level at which they are collected, rather than averaged into large aggregates. Discrete (countable) choice models Discrete choice models, or qualitative choice models, describe, explain, and predict choices between two or more discrete or finite set of alternatives, such as choosing between modes of transport; These models are used in situations where the potential outcomes are discrete or countable; They deal with situations having the question “which one amongst the available options should be chosen?” Discrete choice models Discretechoice models take many forms, including the following: 1. Binary Logit model, 2. Binary Probit model, 3. Multinomial Logit model, 4. Multinomial Probit model, 5. Nested Logit model, 6. Others Logit model The logit model of individual choice behavior has been the most prominent methodology used in disaggregate travel demand models. The logit model assumes that each individual makes selections from among a set of alternatives, often referred to as the choice set. From that set he chooses the alternative he prefers. In making the selection, he assigns a utility value to each alternative. Choice set The choice set is the set of alternatives that are available to the person. For a discrete choice model, the choice set must meet three requirements: 1. The set of alternatives must be collectively exhaustive, meaning that the set includes all possible alternatives. This requirement implies that the person inevitably does choose an alternative from the set. 2. The alternatives must be mutually exclusive, meaning that choosing one alternative means not choosing any other alternatives. This requirement implies that the person chooses only one alternative from the set. 3. The set must contain a finite number of alternatives. This third requirement distinguishes discrete choice analysis from forms of regression analysis in which the dependent variable can (theoretically) take an infinite number of values. Choice set As an example, the choice set for a person deciding which mode of transport to take to work includes driving alone, carpooling, taking bus, etc. The choice set is complicated by the fact that a person can use multiple modes for a given trip, such as driving a car to a train station and then taking train to work. In this case, the choice set can include each possible combination of modes. Alternatively, the choice can be defined as the choice of “primary” mode, with the set consisting of car, bus, rail, and others (e.g. walking, bicycles, etc.). Note that the alternative “others” is included in order to make the choice set exhaustive. Defining choice probabilities A discrete choice model specifies the probability that a person chooses a particular alternative; The probability is expressed as a function of observed variables that relate to the alternatives and the person. In its general form, the probability (Pr) that person, n chooses alternative, i is expressed as: Pni = Pr(person, n chooses the alternative, i) Defining choice probabilities In selecting a mode of transport for example, 1. attributes of the alternative modes (xni), can be travel time and cost, 2. characteristics of the consumer (sn), can be annual income, age, and gender, and can be used to calculate choice probabilities. The attributes of the alternative modes can differ over people; e.g., cost and time for travel to work by car, bus, and rail are different for each person depending on the location of home and work of that person. Defining choice probabilities - properties Utilization of the concept of utility using dummies The choice of the person is designated by dummy variables, yni, for each alternative: Consumer utility for dummies A person's choice depends on many factors, some of which are known and others are not known; The utility obtained from choosing an alternative is decomposed into a part that depends on known variables (systematic component) and a part that depends on variables that are unknown (random component); In a linear form, this decomposition is expressed as: Consumer utility for dummy variables Choice probability The choice probability is then Properties of discrete choice models implied by utility theory Only differences matter The probability that a person chooses a particular alternative is determined by comparing the utility of choosing that alternative to the utility of choosing other alternatives: As the last term indicates, the choice probability depends only on the difference in utilities between alternatives, not on the absolute level of utilities. Equivalently, adding a constant to the utilities of all the alternatives does not change the choice probabilities. Properties of discrete choice models implied by utility theory Scale must be normalized Since utility has no units, it is necessary to normalize the scale of utilities. The scale of utility is often defined by the variance of the error term in discrete choice models. This variance may differ depending on the characteristics of the dataset, such as when or where the data are collected. Normalization of the variance therefore affects the interpretation of parameters estimated across diverse datasets. Logit model Utility value, Vit Hypothetical attributes and SE Characteristics Example Using the hypothetical values (1 = AUTO, 2 = TRANSIT) Therefore AUTO is selected with a probability of 79.9% Aggregate prediction of behavior Aggregate models Aggregate models explain the travel of a group of households or individuals or firms, e.g., all households in a traffic analysis zone. Aggregate data are used in estimating the models. E.g. the average household trip frequency for a zone may be a function of the average household size and average auto ownership levels in the zone. Note that although data are usually available at the household (disaggregate) level, they are averaged before estimating aggregate models. Aggregate groups Consumers are similar in their preferences and characteristics and hence will respond similarly to changes in transportation. Dissimilar groups are made distinct from each other. Segmentation can be made using geographical zones basing on: 1. Income 2. Automobile availability 3. Household size 4. Occupation of family head 5. Trip purpose Form of aggregate demand models Aggregate demand functions take the form V = D(A, S) 1. V = vector of volumes or numbers of consumers making particular choices; 2. A = Social, economic and other characteristics of the activity system or individuals in a group; 3. S = Service attributes that characterize the transportation choices open to prospective trip makers. Thus the function D gives the volumes and flow composition between two (or more) points as a function of the service attributes experienced during movement in an activity system between those points. Examples of aggregate demand functions Gravity models; Intercity mode choice models; The four – step urban transportation model system 1. Trip generation (Travel frequency) 2. Trip distribution (Where trips go) 3. Modal split (share amongst nodes) 4. Network assignment (allotment to routes in the network) Policies to control movements in a system Premise It is prudent to have a proposition that travel behaviors of individuals lead to: Forecasting future travel demand Evaluating the effectiveness of policies Predicting the response to new technologies or services Anticipating possible unintended consequences “Demand” v. “Behavior” Definitions Utility – state of being Disutility – the shortcomings desirable. In other words, of a commodity in satisfying utility is a want-satisfying human needs. capacity of a commodity. Why do People Travel? Why did the cock cross the road? To get where it wants to be! Hence, “Travel is a derived demand” – i.e. the demand for travel is derived from the demand for spatially-separated activities. Corollary: Travel is a disutility, that people try to minimize. Assumed Implications - I Saved travel time and cost are benefits, hence serve as basis for valuing transportation improvements. This is a very crucial benefit component in most cost-benefit analyses We can reduce travel by: making it more expensive congestion levies, fuel taxes, parking levies Assumed Implications - II We can reduce travel by: bringing activities closer together increasing density and mixture of land uses using ICT to conduct the activity remotely telecommuting, -conferencing, -shopping, education, medicine, justice Wecan better forecast travel by under-standing people’s activity engagement – the so-called “activity- based approach” to modeling travel demand. But is that the only reason why people travel -- to get somewhere in particular? Why Would Travel be Intrinsically Desirable? Exercise, physical/mental therapy; Curiosity, variety-, adventure-seeking; conquest; Sensation of speed or even just movement; Exposure to the environment, information; Enjoyment of a route, not just a destination; Symbolic value (status, independence); Buffer between activities, synergy with multiple activities. Assertions Those characteristics apply not only to undirected (recreational) travel, but to directed travel as well varying by mode, purpose, individual, circumstantial Even if “derived”, travel can simultaneously be intrinsically valued in which case, people will be less inclined to reduce it than an evaluation of its “derived” nature alone would suggest. When one thinks about it, virtually ALL policies are intended to affect behavior, whether they are... … supply-oriented, or ….demand-oriented Supply-oriented Policies Expand physical infrastructure This stimulates the realization of latent demand? More effectively manage existing supply - - (Transportation Supply Management, TSM) Increase supply or reduce costs to underserved populations of using non-auto modes Demand-oriented Policies Generally intended to reduce demand, by changing the cost (i.e. raising travel costs!) changing land use planning to bring activities closer together promoting ICT substitution Collectively referred to as Transportation Demand Management (TDM) strategies Summary People travel for many reasons besides the obvious one; it is a fundamental human need; Worldwide trends are towards more travel, not just due to population growth, but per capita; It is a challenge to balance the human need for mobility against the need for sustainability; We need to better understand the need to travel for its own sake, and reasons behind various travel decisions; Implications for modeling, evaluation, policy Urban transportation demand Lecture 6 Planning and operation of transportation Modes in the Components of Development of system a mode transport The route components Highways The vehicle Planning Railways Terminal Evaluation Waterways Operation Design control Construction Airways Operation Others Maintenance Typical Urban Transportation Modes Walking by foot Bicycle Motorized two wheeler (M.T.W) Personal car Private taxi (M.Th.W & M.F.W) Shared taxi (M.Th.W & M.F.W) Bus (express & local) Rail (Light rail, Express rail, Rapid transit) Trucks (Light, Heavy – semi trailers, tractor-trailers) Modes ranked based on User’s point of view Ranking of modes – user’s perspective Speed of the mode (IVTT & OVTT); Accessibility; Cost of travel; Level of comfort; Security; Reliability; Etc. Ranking modes based on accessibility Ranking modes based on cost per Pkm Ranking modes based on level of comfort Modes ranked based on Planner’s point of view Ranking of modes – planner’s perspective Environmental concerns in terms of air pollution caused; Energy consumption of the mode. Sustainability of the mode Ranking based on Air pollution per Pkm Ranking based on energy consumption per Pkm Recapitulation/ summary What is the study of transportation system analysis about? What are the factors on which the travelers base to rank the different modes of transportation? What are the major modal characteristics which form the basis for ranking of the different transport modes for purposes of transportation systems planning? Factors affecting travel demand Demographic and social factors - demand Population; Household composition; Age of the travelers; Cultural aspects; Gender; Etc. Population of Uganda 1948 - 2002 Rural – urban population - Uganda Global urbanization trend Economic factors - Demand Employment; Income; Vehicle ownership; Land use patterns; Etc. Effect of influencing factors on travel pattern over time Factors influencing urban travel behaviour over time Demand is spread over time and space Urban Transportation Planning (UTP) - Concept Urban transportation demand modeling includes: Understanding the factors influencing urban travel demand; Development of the relationships between these factors and the travel demand; Use of the relationships to predict the future demand for travel and the resulting transport infrastructure needs. Prediction of urban flows is done in 4 steps Trip generation – trips made by a particular market segment for each zone of the region studied; Trip distribution - trips originating at each zone are distributed among possible destinations; Modal split – trips from a particular zone to a particular destination are split amongst possible available modes; Network assignment – Trips for each origin – destination – mode combination are assigned to paths in the network. Four step urban transport model Four step urban transport model Aggregation of demand Consumers are aggregated into zones based on: Average income per household; Average automobile ownership per house; Household size; Occupation of breadwinner per household; Composition of family in a household; Geographic location; Trip purpose. Zoning in urban transportation Trip generation analysis A trip for the purpose of analysis is a uni-directional motorized or non-motorized movement from an origin to a destination: Trip generation analysis The principle task of trip generation analysis is to relate the intensity of trip making to and from zones to measures of type and intensity of land use; Two types of trip generation analyses are carried out: Trip production (P) analysis Trip attraction (A) analysis Home and Non-home based trips Urban travel demands are made up of a number of different trip types that have specific spatial (space) and temporal (time) characteristics; The first level of trip classification, used normally, is a broad grouping into: – Home based trips – Non-home based trips Home and non-home based Home based trips are those trips that have one trip end at a household. Otherwise they are non-home based; Examples of home based trips are trips between work place and a home; Examples of non-home based trips are those between work and shopping area, business trips between two activity centers. Productions and attractions Productions are the home ends of the home based trips, or the origins of the non-home based trips; Attractions are the non-home ends of the home-based trips, or the destinations end of the non-home based trips. Trip productions and attractions Trip type illustration Trip generation Illustration of trip types – name them! Illustration of trip types Examples of home based trips Work trips; Educational trips; Shopping trips; Personal business trips; Social and recreational trips. Trip production modeling Modeling trip production involves relating a dependent variable (trip production) to a set of independent variables (factors representing household characteristics); The most common method used is regression analysis; Relevant household characteristics include: – Car ownership – Income of the household head – etc. Trip generation Trip distribution This step matches trip makers’ origins and destinations to develop a “trip table”, a matrix that displays the number of trips going from each origin to each destination. Trip distribution table Where: T ij = trips from origin i to destination j. Note that the practical value of trips on the diagonal, e.g. from zone 1 to zone 1, is zero since no intra-zonal trip occurs. Examples of models used Gravity models Others Modal split The varying proportions of different transport modes which may be used at any one time; The choices of modes may be determined by the costs, destinations, capacities, and frequencies of the modes together with the nature of the goods carried and their destinations; Modes of transport may be seen as competing services, and particularly so in the rivalry between the private car and public transport systems. Modal split Modal split models aim to determine the number of trips on different modes given the travel demand between different pairs of nodes (zones); These models try to mathematically describe the mode choice phase of the sequential demand analysis procedure; Generally, choice models are used for modal split analysis. Route assignment Route assignment, route choice, or traffic assignment concerns the selection of routes (alternative paths) between origins and destinations in transportation networks; To determine facility needs and costs and benefits, we need to know the number of travelers on each route and link of the network (a route is simply a chain of links between an origin and destination). Urban transportation planning The 4 – step model (4SM) Lecture 7 Recall – transport system analysis Manheim (1979) and Florian et al (1988) Analytical steps Trip generation analysis – How many trips are generated? Trip distribution analysis – Where do these trips go? Captive model split analysis – How many use a particular mode? Choice model split analysis – What mode is used by others? Route assignment by mode – What is the route of each trip? 4 step transport planning model The 4SM – urban transport Trip generation What is a trip? A trip, for the purpose of analysis, is a one way movement from an origin to a destination. Trip versus a Tour Trip generation For each discrete spatial unit (geographical zone) it is estimated the extent for which it is an origin and destination for movements. The output is usually the number of trips produced and attracted by a given spatial unit. Trip generation Study area - GKMA map as an example Trip generation analysis The principal task here is to relate the intensity of trip making, to and from each traffic zone, to measures of the type and intensity of land use. There are two types of trip generation analysis carried out: i. Trip production analysis ii. Trip attraction analysis Home and Non-home based Urban travel demands are made up of a number of different trip types that have specific spatial (space) and temporal (time) characteristics; The first level of trip classification, used normally, is a broad grouping into: Home based trips Non-home based trips Home and non-home based Home based trips are those trips that have one trip end at a household. Otherwise they are non-home based; Examples of home based trips are trips between work place and a home; Examples of non-home based trips are those between work and shopping area, business trips between two activity centers. Productions and attractions Productions are the home ends of the home based trips, or the origins of the non-home based trips; Attractions are the non-home ends of the home- based trips, or the destinations end of the non- home based trips. Trip productions and attractions What is a household? A group of persons, who normally live together and take their meals from a common kitchen unless the demands of work prevents them from doing so. The persons in a household may be related or unrelated to one another or a mix of both. Institutional household A group of unrelated persons who live in an institution and take their meals from a common kitchen. Examples include – hostels, rescue homes, orphanages, jails, hotels, etc. Characteristics affecting demand Characteristic Examples 1 Socio-economic - Income, education, occupation, living conditions, etc. 2 Demographic - Population, gender, income level, marital status, ethnic origin, education level, etc. 3 Land use Residential, commercial, government, institutional, recreation, green space, mixed, etc. Modeling trip productions Modeling trip production Process of relating the trips produced by households to the factors influencing trip production by appropriate analytical techniques. Most important household characteristics related to trip production include: i. Household size and composition, ii. Number of employed persons, iii. Number of students, iv. Household income, v. Vehicle ownership, and vi. Others. HB trip classifications Classifications used for HB trips in urban transportation planning studies include: i. Work trips, ii. Educational trips, iii. Shopping trips, iv. Personal business trips, v. Work related business trips, vi. Social and recreation trips, etc. Modeling trip production In essence, it involves relating a dependent variable (trip production) to a set of independent variables (factors representing household characteristics); The most common analytical tools used for this purpose are: i. Regression analysis ii. Category analysis or cross classification analysis Regression analysis The major goal of regression analysis is to develop a statistical model that can predict the values of a dependent (response) variable based upon the values of the independent (explanatory) variables. Simple regression: A statistical model that utilizes one quantitative independent variable “X” to predict the quantitative dependent variable “Y.” Multiple regression A statistical model that utilizes two or more quantitative and qualitative explanatory variables (x1,..., xp) to predict one quantitative dependent variable Y. Caution: There must be at least two or more quantitative explanatory variables (rule of thumb). Multiple regression Multiple linear regression model Hypothesis H0: 1 = 2 = 3 =... = P = 0 H1: At least one regression coefficient is not zero Multiple regression models Multiple Regression Models Non- Linear Linear Dummy Inter- Linear action Variable Poly- Square Log Reciprocal Exponential Nomial Root Category analysis Salient definitions: Social class - A grouping based on social factors like wealth, income, education, and occupation. These factors affect how much power and prestige a person has. Karl Max Conflict theory asserts that society is made of two social classes – the Proletariats (the poor selling labour for wages) and the Bourgeoisies (the rich purchasing labour and use it to gain profit. Category analysis The concepts of social class often assume three general economic categories: A very wealthy and powerful upper class that owns and controls the means of production; A middle class of professional workers, small business owners and low-level managers; and A lower class, who rely on low-paying jobs for their livelihood and experience poverty. Category analysis Most traffic analysis zones tend to contain a mix of social and economic classes of people; Use of regression based on aggregate measures of zonal characteristics, tends to submerge important characteristics of travel demand; Hence a number of transportation planners use households, rather than traffic zones, as the basic unit of trip making. Category analysis The trip production technique based on household and its characteristics is known as Category analysis; Households are sorted into a number of separate categories according to properties that characterize them; The results of category analysis are then tabulated for analysis. Hypothetical category analysis data Category analysis – previous data Category analysis The information in the table and figure can easily be translated into zonal trip production estimates; The number of households within each traffic zone, that is expected to fall within each cell of the matrix, are estimated and multiplied by the trip rate and these productions summed up to give the zonal trip production. Modeling trip attractions Trip attraction analysis Land use characteristics influence the trip attraction rate in urban areas. Common types of land use include: Residential, Administrative (Offices, courts, etc), Commercial (markets, garages, shops), Industrial (Manufacturing, etc.), Institutional (schools, hospitals, service, etc.), Recreational (stadia), and Others. Modeling trip attraction 1. Use Regression analysis 2. Causal variables Retail trade floor area; Service and office floor area; Manufacturing and wholesale floor area; Number of employment opportunities; Number of activity centres, e.g. schools, hospitals, etc. Example using regression Develop a trip attraction equation using the data below. Solution from regression analysis y = daily work trips attracted X1 = No. of employment opportunities in manufacturing X2 = No. of employment opportunities in service Trip distribution Commonly a spatial interaction model that estimates movements (flows) between origins and destinations and which can consider constraints such as distance. The output is a flow matrix table between spatial units or geographical study zones. Trip distribution Trip distribution is a model of the number of trips that occur between each origin zone and each destination zone. It uses the predicted number of trips originating in each origin zone (trip production model) and the predicted number of trips ending in each destination zone (trip attraction model). Trip distribution matrix Where: T ij = trips from origin i to destination j. Note that the practical value of trips on the diagonal, e.g. from zone 1 to zone 1, is zero since the assumption is that no intra-zonal trip occurs. The gravity model The most widely used and documented trip distribution model is the gravity model. It states that the number of trips between two zones is directly proportional to the number of trips generated by the zones and inversely proportional to a function of time of travel between the two zones. The gravity model Determination of the F factors Illustration of the gravity model Consider a study area consisting of three zones. The data have been determined as follows: i. the productions and attractions have been computed for each zone by methods of trip generation, and ii. the average travel times between each zone have been determined. iii. Assume Kij is the same unit value for all zones. Collected data Example illustrated Collected data Solution of the gravity model Modal split Movements between origins and destination are then disaggregated by modes. This function depends on the availability of each mode, their respective level of service attributes, and social preferences by the users. Mode choice effectively factors the trip tables from trip distribution to produce mode-specific trip tables. Modal split The most common models used are the logit model (see work on discrete choice models and nested logit models elsewhere). These mode choice models can reflect a range of performance variables and trip-maker characteristics, but produce disaggregate results which must then be aggregated to the zonal level prior to route choice. See Ortuzar and Willumsen, 1994 Discrete choice models Discrete choice models take many forms, including the following: 1. Binary Logit model, 2. Binary Probit model, 3. Multinomial Logit model, 4. Multinomial Probit model, 5. Nested Logit model, 6. Others Network assignment – route choice All the estimated trips by origin, destination and mode and then “loaded” on the transportation network, mainly with the consideration that users want to minimize their travel time or have to flow through existing transit networks. If the traffic exceeds the capacity of specific transport segments (which is often the case), congestion occurs and negatively affects travel time. Route choice In this last of the 4SM, an equilibration of demand and performance is finally present. Modal O-D trip matrices are loaded on the modal networks usually under the assumption of user equilibrium (UE). All paths utilized for a given O-D pair have equal impedances. For off-peak assignments, stochastic assignment is often used which tends to assign trips across more paths better reflecting observed traffic volumes in uncongested periods. Route choice The basic user equilibrium solution is obtained by the Frank-Wolfe algorithm which involves the computation of minimum paths and all-or-nothing (AON) assignments to these paths. Subsequent AON assignments (essentially linear approximations) are weighted to determine link volumes and thus link travel times for the next iteration.