Unit 9: Multicollinearity, Heteroskedasticity, GLS and Clustering, PDF
Document Details
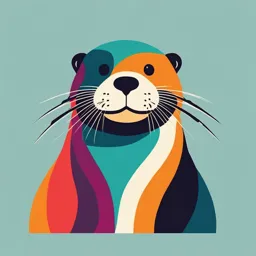
Uploaded by AwesomeCarnelian4810
Martin / Zulehner
Tags
Summary
This document provides an outline and introduction to Unit 9 on multicollinearity, heteroskedasticity, generalized least squares (GLS), and clustering and autocorrelation in Introductory Econometrics. It covers potential examples of perfect multicollinearity, symptoms, and methods for solving multicollinearity issues.
Full Transcript
Unit 9: Multicolliniarity, Heteroskedasticity, GLS and Clustering and Autocorrelation Martin / Zulehner: Introductory Econometrics 1 / 42 Outline 1 Multicollinearity Multicollinearity and Variance What to Do? 2 Heteroskedasticity Variance Estimat...
Unit 9: Multicolliniarity, Heteroskedasticity, GLS and Clustering and Autocorrelation Martin / Zulehner: Introductory Econometrics 1 / 42 Outline 1 Multicollinearity Multicollinearity and Variance What to Do? 2 Heteroskedasticity Variance Estimator Inference 3 Generalized least squares Weighted least squares Feasible Generalized Least Squares 4 Correlation in the Residuals: Clustering Three Variance Estimators 5 Appendix: GLS more Formally Martin / Zulehner: Introductory Econometrics 2 / 42 1. Multicollinearity Recall the assumption MLR.3 that rank(X) = K + 1, i.e. that the there are no exact linear relationships among the independent variables Perfect multicollinearity means that rank(X) ̸= K + 1 ′ This implies that the matrix X X is not invertible. Therefore we can not " identify" our β parameter as a function of population moments in observable variables y = Xβ + u ′ X y = (X′ X)β + X′ u E (X′ y) = E (X′ X)β + E (X′ u) E (X′ y) = E (X′ X)β , by MLR4 Hence if MLR3 doesn't hold we cannot write: β = [E (X′ X)]−1 E (X′ y) Martin / Zulehner: Introductory Econometrics 3 / 42 Multicollinearity and Variance Possible examples of perfect multicollinearity: ▶ If we simultaneously use "weekly working hours" and "monthly working hours", since the latter is just a linear transformation of the former ▶ If we use all the dummies dened from a categorical variable (e.g. "black", "white", "hispanic", and "others") since they sum to 1 and are therefore identical to the constant Non-perfect multicollinearity arises when two explanatory variables in a regressions are (very) highly but not perfectly correlated, i.e. the determinant of the matrix ′ (X X) is close to zero Symptom: the overall t of the regression is good (R 2 is high) but the standard errors of the single coecient estimates are high. Why? Martin / Zulehner: Introductory Econometrics 4 / 42 Multicollinearity and Variance Think of the variance of the estimator β̂j σ2 Var (β̂j ) = for j = 1, 2,... , K SSTj 1 − Rj2 It depends on three factors 1 The error variance σ2. This is a population parameter and it is the smaller the more independent variables we use 2 The total sample variation in the xj (SSTj ), i.e. we want to have a lot of variation in the X, for instance by increasing the sample size 3 The linear relationship among the independent variables Rj2 Martin / Zulehner: Introductory Econometrics 5 / 42 Multicollinearity and Variance Rj2 is the R2 obtained from the regression: xj = α0 + α1 x1 +... + αj−1 xj−1 + αj+1 xj+1 +... + αn xn + ϵj ▶ Think of a model with only two regressors x1 and x2 ▶ A high R12 indicates that x2 and x1 are highly correlated 2 ▶ As R1 increases, Var (β̂1 ) increase 2 Rj → 1 then Var (β̂1 ) → ∞ and we have some problems with the Only if asymptotic properties and statistical inference Non-perfect multicollinearity violates none of our assumptions, hence the "problem" is not really well-dened 1 ▶ Variance ination factor: VIFj = 1−Rj2 (rule of thumb: VIFj > 10 implies serious multicollinearity; no problem if maxj (VIFj ) < 5) ▶ low values of det(X′ X) indicate multicollinearity One would be tempted to drop variables which are highly correlated Martin / Zulehner: Introductory Econometrics 6 / 42 What to do? The choice of whether or not to include a particular variable in a regression model can be made by analyzing the tradeo between bias and variance (e.g. eciency) ▶ Think of the two regressions ŷ = βˆ0 + βˆ1 x1 + βˆ2 x2 and ỹ = β˜0 + β˜1 x1 then: σ2 σ2 Var (β̂1 ) = 2 > = Var (β̃1 ) SST1 (1 − R1 ) SST1 ▶ However, if x2 should be part of the model, omitting it would bias the estimate of β̂1 (omitted variable bias) Yet, since the variances decrease as N increases, the best solution to multicollinearity is to use all "relvant" variables and increase the number of observations Martin / Zulehner: Introductory Econometrics 7 / 42 2. Heteroskedasticity Our assumption of homoskedasticity MLR.5 implis that the variance of the unobserved error u (σ 2 ) is constant, conditional on the explanatory variables If this is not true, that is if the variance of u is dierent for dierent values of the x 's, then the errors are heteroskedastic Example When we estimate returns to education and the ability is unobservable (i.e. part of the the error terms), we might think the the variance in ability (i.e. the variance of the error term) might dier by educational attainment Martin / Zulehner: Introductory Econometrics 8 / 42 A general Example of Heteroskedasticity Martin / Zulehner: Introductory Econometrics 9 / 42 Another Example of Heteroskedasticity Exploring the data can be very instructive to detect heteroskedasticity Figure plots family income on age of househod head (SHIW data) As age increases, the dispersion around regression line increases, suggesting heteroskedasticity (why?) Martin / Zulehner: Introductory Econometrics 10 / 42 Variance-Covariance Matrix of the Error Term In the presence of heteroskedasticity, and under the i.i.d. assumption MLR.2, the variance-covariance matrix of the error term u is: 2 σ1 0... 0 0 σ22... 0 Var (u|X) = E (uu′ ) = . =Ω .... ....... 0 0... σN2 Martin / Zulehner: Introductory Econometrics 11 / 42 Why Worry About Heteroskedasticity? We showed that OLS is unbiased and consistent, even if we do not assume homoskedasticity The standard errors of the estimates are biased if we have heteroskedasticity If the standard errors are biased, we can not use the usual t statistics or F statistics or LM statistics for drawing inference Martin / Zulehner: Introductory Econometrics 12 / 42 2.1 Variance Estimator under Heteroskedasticity For the simple linear regression (SLR) case, Pn 1 (xi − x̄)ui β̂1 = β1 + Pi= n 2 i=1 (xi − x̄) And: Pn Pn 2 1 (xi − x̄)ui i=1 (xi − x̄) Var (ui ) Var (β̂1 ) = Var β1 + Pi= = 2 2 2 n i=1 (xi − x̄) Pn i=1 (xi − x̄) − x̄)2 σi2 Pn i=1 (xi = SSTx2 If σi2 ̸= σ 2 , a valid estimator for the variance is − x̄)2 ûi2 Pn i=1 (xi Var d (β̂1 ) = SSTx2 where ûi2 are the OLS residuals Martin / Zulehner: Introductory Econometrics 13 / 42 Variance Estimator under Heteroskedasticity For the general multiple regression model, a valid estimator of Var (β̂j ) with heteroskedasticity is rˆij2 ûi2 P Var d (β̂j ) = SSRj2 rˆij is the i th residual from regressing xj on all other independent variables (i.e. rˆij = xj − αˆ0 − αˆ1 x1 −... − αj− ˆ 1 xj−1 − αj+ ˆ 1 xj+1 −... − αˆn xn ) SSRj is the sum of squared residuals from this regression Martin / Zulehner: Introductory Econometrics 14 / 42 Variance Estimator under Heteroskedasticity White (1980) developed a robust estimator for the variance covariance matrix of the OLS estimator β̂ :1 Var (β̂) = E [(β̂ − β)(β̂ − β)′ ] = E [((X′ X)−1 X′ u)((X′ X)−1 X′ u)′ ] = (X′ X)−1 X′ E [uu′ ]X(X′ X)−1 = (X′ X)−1 X′ ΩX(X′ X)−1 The OLS residual for the i-th observation is: ûi = yi − xi β̂ Therefore we have that ··· û1 û1 0 0 0 û2 û2 ··· 0 Ω̂ = ........ .... 0 0 ··· ûN ûN 1 It follows from β̂ = β + (X′ X)−1 X′ u ⇒ β̂ − β = (X′ X)−1 X′ u. Martin / Zulehner: Introductory Econometrics 15 / 42 2.2 Inference under Heteroskedasticity Now that we have a consistent estimate of the variance, the square root can be used as a standard error for inference Typically we call these robust standard errors. Sometimes the estimated variance is corrected for degrees of freedom by multiplying by N/(N − K − 1) As N→∞ this doesn't matter anyways In Stata, robust standard errors are easily obtained using the robust option of reg reg y x1 x2..., vce(robust) Now we can simply generate robust t-statistic using the robust standard errors and test signicance of our coecients or other hypotheses Martin / Zulehner: Introductory Econometrics 16 / 42 A Robust LM Statistic Run OLS on the restricted model and save the residuals û Regress each of the excluded variables on all of the included variables (these are q dierent regressions!) and save each set of residuals rˆ1 , rˆ2 ,... , rˆq Regress a variable dened to be = 1 on rˆ1 û, rˆ2 û,... , rˆq û , with no intercept (it seems weird but there is a reason to do it!) The LM statistic is N − SSR1 , where SSR1 is the sum of squared residuals from this nal regression This statistic is χ-squared distributed with q degrees of freedom Martin / Zulehner: Introductory Econometrics 17 / 42 Testing for Heteroskedasticity: The Breusch-Pagan Test We want to test H0 : Var (u|x1 , x2 ,... , xk ) = σ 2 which is equivalent to H0 : E (u 2 |x1 , x2 ,... , xk ) = E (u 2 ) = σ 2 If we assume the relationship between u2 and xj is linear, then we can test this hypothesis as a linear restriction Hence, for u 2 = δ0 + δ1 x1 +... + δK xK + ν , this means testing H0 : δ1 = δ2 =... = δk = 0 Martin / Zulehner: Introductory Econometrics 18 / 42 The Breusch-Pagan Test We do not observe the errors (u ), but we can estimate them with the residuals from the OLS regression (û ) We now regress the residuals squared on all of the x 's 2 û = δ0 + δ1 x1 +... + δK xK + ν We use the R2 to form an F or LM test The F statistic is just the reported F statistic for overall signicance of the regression, [R 2 /K ] F = [(1 − R 2 )/(N − K − 1)] which is FK ,N−K −1 distributed The χ2K distributed LM statistic is: LM = NR 2 Martin / Zulehner: Introductory Econometrics 19 / 42 Testing for Heteroskedasticity: The White Test The Breusch-Pagan test will detect any linear form of heteroskedasticity The White test allows for nonlinearities by using squares and cross products of all the x 's û 2 = δ0 + δ1 x1 + δ2 x12 + δ3 x1 x2 + δ4 x1 x3 +... + δK +1 x1 xK δK +2 x2 + δK +3 x22 +... + ν We still just use an F or LM to test whether all the xj , xj2 , and xj xh are jointly signicant This can get to be cumbersome pretty quickly, especially if we have a lot of regressors Martin / Zulehner: Introductory Econometrics 20 / 42 Alternative Form of the White Test Consider that the tted values from the OLS, ŷ , are a function of all the x 's 2 Thus, ŷ will be a function of the squares and cross products of the xi 's and ŷ and ŷ 2 can proxy for all of the xi , xi2 , and xi xj Thus, regress the residuals squared on ŷ and ŷ 2 and use the R2 to form an F or LM statistic û 2 = δ0 + δ1 ŷ + δ2 ŷ 2 + ν where ŷ = β̂0 + β̂1 x1 + β̂2 x2 +... + β̂K xK Note, we are now only testing for 2 restrictions Martin / Zulehner: Introductory Econometrics 21 / 42 3. Generalized Least Squares While it is always possible to estimate robust standard errors for OLS estimates, if we know something about the specic form of the heteroskedasticity, we can obtain more ecient estimates than OLS (e.g. with smaller variance!) We will look for generalized forms of our OLS estimator to account for this heteroskedasitcity One of the basic idea is to transform the model into one that has homoskedastic errors We will look at two particular cases: 1 When we know the form of heteroskedasticity: the Weighted Least Squares (WLS) estimator 2 When we estimate the form of heteroskedasticity: the Feasible Generalized Least Squares (FGLS) estimator Martin / Zulehner: Introductory Econometrics 22 / 42 3.1 Weighted Least Squares Suppose the heteroskedasticity can be modeled as Var (u|x) = σ 2 h(x), where h(xi ) = hi is known. Hence we have ▶ E √ui |x = 0, since E (ui |x) = 0 and hi is a function of x hi σ 2 hi (x) ▶ Var √ui |x = hi (x) = σ2 hi √ So, if we divided our whole equation by hi we would have a model where the error is homoskedastic: yi 1 xi 1 xi 2 xiK ui √ =β0 √ + β1 √ + β2 √ +... + βK √ + √ hi hi hi hi hi hi ∗ yi∗ =β0 xi∗0 + β1 xi∗1 + β2 xi∗2 +... + βK xiK + ui∗ Martin / Zulehner: Introductory Econometrics 23 / 42 Weighted Least Squares - examples if the heteroskedasticity can be modeled as Var (u|x) = σ 2 xik2 , ie. h(xik ) = xik2 , then the transformed regression model for GLS is yi 1 xi 1 xi 2 xiK ui =β0 + β1 + β2 +... + βK + xik xik xik xik xik xik 1 weight applied to each observation: x ik if the heteroskedasticity can be modeled as Var (u|x) = σ 2 xik , ie. h(xi k) = xik , then the transformed regression model for GLS is yi 1 xi 1 xi 2 xiK ui √ =β0 √ + β1 √ + β2 √ +... + βK √ + √ xik xik xik xik xik xik 1 weight applied to each observation: √x ik Martin / Zulehner: Introductory Econometrics 24 / 42 Generalized & Weighted Least Squares To estimate the transformed equation by OLS is one possible example of generalized least squares (GLS) estimator ▶ GLS will be BLUE in this case ▶ GLS is a weighted least squares (WLS) procedure where each observation is weighted by the inverse of the squared root of hi and each squared residual weighted by inverse of hi idea is that less weight is given to observations with a higher error variance (OLS gives each observation the same weight because it is best when the error variance is identical for all partitions of the population) Martin / Zulehner: Introductory Econometrics 25 / 42 Generalized & Weighted Least Squares mathematically, the WLS estimators are the values of the βj that make n (yi − β0 − β1 xi 1 − β2 xi 2 −... − βK xiK )2 /hi X i=1 as small as possible. Bringing the square root of 1/hi inside the squared residual shows that the weighted sum of squared residuals is identical to the sum of squared residuals in the transformed variables: n ∗ 2 X (yi∗ − β0 xi∗0 − β1 xi∗1 − β2 xi∗2 −... − βK xiK ) i=1 the squared residuals in are weighted by 1/hi , whereas the transformed variables are √ weighted by 1/ hi Martin / Zulehner: Introductory Econometrics 26 / 42 Generalized & Weighted Least Squares While it is intuitive to see why performing OLS on a transformed equation is appropriate, it can be tedious to do the transformation Weighted least squares is a way of getting the same thing, without the transformation idea is to minimize the weighted sum of squares (weighted by 1/hi , each √ observations is weighted by 1/ hi ) WLS is great if we know what Var (ui |xi ) looks like ▶ Example: data is aggregated, but the model is on individual level ▶ We want to weight each aggregate observation by the inverse of the number of individuals Martin / Zulehner: Introductory Econometrics 27 / 42 3.2 Feasible Generalized Least Squares More typical is the case where we do not know the form of the heteroskedasticity In this case, we need to estimate h(xi ) Typically, we start with the assumption of a fairly exible model, for example (but there can be many others!) Var (u|x) = σ 2 exp(δ0 + δ1 x1 +... + δK xK ) If we knew the δ 's, we could use WLS but it is more realistic to assume that we don't and hence we estimate them. To do that, we will try to linearize the above model Martin / Zulehner: Introductory Econometrics 28 / 42 Feasible GLS Our assumption implies that u 2 = Var (u|x) = σ 2 exp(δ0 + δ1 x1 +... + δK xK )ν Where E (ν|x) = 1 If assume that ν is independent of x then we can write: ln(u 2 ) = α0 +δ1 x1 +... + δK xK + |{z} e (1) |{z} ln(σ 2 )+δ0 ln(ν) Where E (e) = 0 and it is independent of x and therefore the Gauss-Markov assumptions hold. Hence, by using û as an estimate of u we can now estimate the δj by OLS What we need are the tted values of from regression (1) (call them ĝi ), and then the estimate for hi are simply ĥi = exp(ĝi ) and their inverse are the weights ▶ the squared residual for observation i gets weighted by 1/ĥi ▶ if we instead rst transform all variables and run OLS, each variable gets p multiplied by 1/ ĥi including the intercept Martin / Zulehner: Introductory Econometrics 29 / 42 Feasible GLS - summary Summarizing, what did we do? 1 We run the original OLS model 2 Save the residuals,û , square them and take the log ln û 2 3 Regress on all of the independent variables and get the tted values, ĝ 4 Determine the WLS estimator using 1/exp(ĝ ) as the weight Having to estimate hi using the same data that we use to estimate our β means that the FGLS is biased (hence it is not BLUE) However, one can show that the FGL estimator is consistent and asymptotically more ecient than OLS Martin / Zulehner: Introductory Econometrics 30 / 42 WLS wrap up When doing F tests with WLS, form the weights from the unrestricted model and use those weights to do WLS on the restricted model as well as the unrestricted model Remember we are using WLS just for eciency - OLS is still unbiased and consistent OLS and WLS estimates will still be dierent due to sampling errors, but if they are very dierent then it is likely that some other Gauss-Markov assumption is violated as well Martin / Zulehner: Introductory Econometrics 31 / 42 4. Correlation in the Residuals: Clustering In many situations individuals are aected by variables that operate at a higher level e.g. industry, region, economy: call this higher-level a group or cluster Hence, our assumption of random sampling MLR.2, i.e. error terms ui are i.i.d., might be invalid Example: individual behavior may depend on some group-level variable: ▶ Individual school performance may be aected by school level variables ▶ Individual rm performance may depend on average productivity in their cluster ▶ Individual in dierent countries are subject to the same laws, etc. In general individual observations may be correlated because of unobserved strata eect Martin / Zulehner: Introductory Econometrics 32 / 42 Correlation in the Residuals This creates correlation in the error terms within clusters: hence the variance covariance matrix of the error term will no longer be diagonal Typical case: errors are correlated within a cluster but uncorrelated across cluster, i.e. we will assume random sampling across clusters! ▶ Students in same school have correlated errors, but across schools they are independent Example: 4 observations with two clusters a and b and heteroskedasticity 2 σ1 ρa 0 0 ρa σ22 0 0 Ω= σ32 0 0 ρb 0 0 ρb σ42 Martin / Zulehner: Introductory Econometrics 33 / 42 Clustering In this case the OLS standard errors will be too low and inference will be invalid Intuition: by assuming that errors are independent across observations in the same cluster you are overstating the amount of information that each observation provides (which shows as too low standard errors) ▶ Hence, the standard errors should be inated to account that some of the information from each observation in one cluster is essentially the same STATA has an option to compute standard errors adjusted for clustering: reg y x, vce(cluster clusterid ) where clusterid is the identier for the cluster (e.g. region, state, province, school, etc.) Martin / Zulehner: Introductory Econometrics 34 / 42 Three Variance Estimators: OLS, robust, and robust cluster 1 OLS variance estimator: N 1 ûi2 (X′ X)−1 X Var d (β̂) = N −K −1 i=1 2 Robust (unclustered) variance estimator: " N # d Robust (β̂) = (X′ X)−1 (ûi xi )′ (ûi xi ) (X′ X)−1 X Var i=1 3 Robust cluster variance estimator: Ncluster d Cluster (β̂) = (X′ X)−1 êj′ êj (X′ X)−1 X Var j=1 P where êj = jcluster ûj xj Martin / Zulehner: Introductory Econometrics 35 / 42 Three Variance Estimators: Discussion If Var d Cluster (β̂) < Var d Robust (β̂), ▶ Then the cluster sums of ei xi have less variability than the individual ei xi ⇒ when you sum the ei xi within a cluster, some of the variation gets canceled out, and the total variation is less ▶ A big positive is summed with a big negative to produce something small ⇒ there is negative correlation within cluster Comparing (1) to (2) or (3) is trickier ▶ In (1) the squared residuals are summed, but in (2) and (3) the residuals are multiplied by the x's and then square and summed ⇒ dierence has to do with correlations between the residuals and the x's ▶ If big (in absolute value)ei are paired with big xi , then the Var d Robust (β̂) > Var d (β̂) ▶ If, Var d Robust (β̂) < Var d (β̂) what's happening is not clear but has to do with some odd correlations between the residuals and the x's ▶ If all the assumptions of the OLS model are true, then the expected values of (1) and (2) are approximately the same Martin / Zulehner: Introductory Econometrics 36 / 42 Scatter plot birthweight 6000 4000 birthweight 2000 0 0 1000 2000 3000 id Exercise: code in R Martin / Zulehner: Introductory Econometrics 37 / 42 Comparison of standard errors. reg birthweight educ age smoker alcohol unmarried Source SS df MS Number of obs = 3,000 F(5, 2994) = 36.53 Model 60470480.6 5 12094096.1 Prob > F = 0.0000 Residual 991149523 2,994 331045.265 R-squared = 0.0575 Adj R-squared = 0.0559 Total 1.0516e+09 2,999 350656.887 Root MSE = 575.37 birthweight Coef. Std. Err. t P>|t| [95% Conf. Interval] educ 7.308416 5.591695 1.31 0.191 -3.655537 18.27237 age -2.292542 2.306962 -0.99 0.320 -6.815933 2.230848 smoker -185.2025 27.9281 -6.63 0.000 -239.9627 -130.4423 alcohol -39.49944 77.08665 -0.51 0.608 -190.6476 111.6487 unmarried -244.3864 28.52471 -8.57 0.000 -300.3164 -188.4564 _cons 3442.335 81.19958 42.39 0.000 3283.123 3601.548. reg birthweight educ age smoker alcohol unmarried, robust Linear regression Number of obs = 3,000 F(5, 2994) = 33.34 Prob > F = 0.0000 R-squared = 0.0575 Root MSE = 575.37 Robust birthweight Coef. Std. Err. t P>|t| [95% Conf. Interval] educ 7.308416 5.587443 1.31 0.191 -3.647199 18.26403 age -2.292542 2.487134 -0.92 0.357 -7.169207 2.584122 smoker -185.2025 28.17357 -6.57 0.000 -240.444 -129.961 alcohol -39.49944 76.91142 -0.51 0.608 -190.304 111.3051 unmarried -244.3864 31.79623 -7.69 0.000 -306.731 -182.0417 _cons 3442.335 85.86685 40.09 0.000 3273.971 3610.699. Exercise: code in R Martin / Zulehner: Introductory Econometrics 38 / 42 Appendix: GLS more Formally Assume that our homoskedasticity assumption MLR.5 does not hold The variance-covariance matrix of the error terms is Var (u) = Ω ̸= σ 2 I where Ω is a general positive denite matrix 2 One can show that for Ω −1 there exists a matrix Ω −1/2 , such that −1/2 −1/2 −1 Ω Ω =Ω In such a situation, we can prove that the following Generalized Least Squares (GLS) estimator (β̂β GLS ) is BLUE: β GLS = (X′Ω −1 X)−1 X′Ω −1 y β̂ Ω−1/2 X)′ (Ω = [(Ω Ω−1/2 X)]−1 (Ω Ω−1/2 X)′ (Ω Ω−1/2 y) Martin / Zulehner: Introductory Econometrics 39 / 42 GLS vs. OLS If we multiply our regression equation y = Xβ + u with Ω −1/2 we obtain a transformed model Ω −1/2 y = Ω −1/2 Xβ + Ω −1/2u ⇒ y∗ = X∗β + u ∗ The OLS estimation of the transformed model gives us the GLS estimator: β GLS = (X′∗ X∗ )−1 X′∗ y∗ β̂ 2 where β GLS ) = σ̂u∗ Var (β̂ (X′∗ X∗ )−1 Martin / Zulehner: Introductory Econometrics 40 / 42 Feasible Generalized Least Squares The matrix Ω is generally not known and must be estimated In the case of heteroskedastic error terms it is a diagonal matrix with σi2 as an element of the diagonal and 0 elsewehre.: σ̂12 0 0 0 0 σ̂22 0 0 Ω= Ω̂ ........ .... 0 0 0 σ̂N2 However, we have N unknown parameters and only N observations! Therefore, we generally assume a functional form for the heteroskedasticity such as σi2 = α1 zi2 where zi2 is a observable variable Martin / Zulehner: Introductory Econometrics 41 / 42 Weighted Least Squares (WLS) In case of heteroskedasticity, the GLS estimator simplies to the Weighted Least Squares (WLS) estimator ′ −1 Pn 1 ′ Ω X Since X Ω̂ = i=1 σ 2 )xi xi , then: i n X −1 X n ′ 2 2 β WLS = β̂ xi xi /σi xi yi /σi i=1 i=1 If σi2 = α1 zi we obtain: n X −1 X n ′ β WLS = β̂ xi xi /zi xi yi /zi i=1 i=1 Martin / Zulehner: Introductory Econometrics 42 / 42