Introductory Econometrics for Finance PDF
Document Details
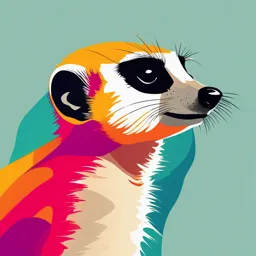
Uploaded by AdoredMusicalSaw
L'Université Internationale de Rabat (UIR)
Zakaria Elouaourti
Tags
Related
- Introduction to Financial Econometrics PDF
- Introductory Econometrics for Finance PDF
- Chapter 3: Classical Linear Regression Model PDF
- Chapter 4: Multiple Linear Regression (PDF)
- Chapter 5 Classical Linear Regression Model Assumptions and Diagnostics PDF
- Panel Data - Introductory Econometrics for Finance (PDF)
Summary
Introductory Econometrics for Finance course from UIR. The course introduces various concepts like econometrics, finance, regression analysis, and includes learning outcomes, course philosophy, and syllabus.
Full Transcript
Introductory Econometrics for Finance ZAKARIA ELOUAOURTI Knowledge assessment SOFTWARE USAGE EXPERIENCE WITH REAL DATA 02 03 01 PREVIOUS EXPERIENCE IN...
Introductory Econometrics for Finance ZAKARIA ELOUAOURTI Knowledge assessment SOFTWARE USAGE EXPERIENCE WITH REAL DATA 02 03 01 PREVIOUS EXPERIENCE IN STATISTICS Course Philosophy: Bridging Theory and Practice 1.Econometrics is not just about mastering statistical techniques 2. Start with the basics: Use statistical analysis, including classical regression models, to estimate financial parameters, predict outcomes, and test hypotheses with quantitative data. 3. Produce and Interpret descriptive statistics and basic linear regression models. Understand the basic assumptions of the classical linear regression model and identify and address violations such as autocorrelation and heteroscedasticity. 4.Use R statistical software and EXCEL effectively. Learning outcomes 1.Empirical Work: Our graduates will work with real data and develop the skills necessary for conducting empirical research. 2.Software Proficiency: Our graduates will gain proficiency in using R statistical software for data analysis. 3.Regression Analysis: Our graduates will be able to apply regression analysis to various data sets and test the robustness of their predictions. 4.Data Interpretation: Our graduates will be capable of interpreting descriptive statistics and basic linear regression models, estimating economic parameters, and predicting financial outcomes. Course outline : Syllabus Presentation Course 1 1.1 Introduction to Econometrics in Finance The Nature, Purpose, and Importance of Econometrics: What is Econometrics? Main Fields of Econometrics What is Financial Econometrics? The Four of Financial Econometrics: 1) Financial Theory; 2) Financial Data; 3) Methods or Models; 4) Tools (Econometrics Software: R, STATA, etc.) (roadmap) Examples of Problems Solved by Econometricians 1.2 Financial Data: Types and Notation Continuous and Discrete Data Cross-Sectional Data Time Series Data Panel Data Special Characteristics of Financial Data Sources of Financial Data 1..3 A Practical Example of Financial Data Manipulation Using R Get started : R philosophy Get started : What is a packages? Get started with R : interface Data manipulation with R (from extracting/importing data to interpreting descriptive statistics) Course outline : Syllabus Presentation Course 2 2.1 The Concept of a Model in Econometrics What is Regression Analysis? Structure of a Linear Model Key Notation and Terminology (Dependent vs. Independent Variables) Why Include a Disturbance Term? 2.2 Simple Regression Model Presentation of the Model Determining Regression Coefficients (Mathematical Demonstration) Parameter Estimation: Model and Hypotheses Ordinary Least Squares (OLS): How it Works Assumptions of the Classical Linear Regression Model (CLRM) Estimator Properties: Unbiased and Convergent (Mathematical Demonstration) 2.3 Simple Application of a Linear Regression Model Real-World Example Step-by-Step OLS Estimation using R Software Interpretation of Results: Understanding the Estimated Coefficients Course outline : Syllabus Presentation Course 3 3.1. Assessing the Quality of Regression Goodness-of-fit measures: R-squared (R²), Adjusted R-squared. Analyzing residuals and their implications. Significance tests for regression coefficients (t-tests, F-tests). 3.2. Classical linear regression model assumptions and diagnostics: Multicollinearity, heteroscedasticity, and autocorrelation in regression models. Multicollinearity Heteroscedasticity Autocorrelation 3.3. Classical linear regression model assumptions and diagnostics using R Course outline : Syllabus Presentation Course 4 4.1 Applying econometrics to financial theory Returns in Financial Modelling Log Returns The Capital Asset Pricing Model (CAPM) and Stock Returns 4.2 Application of the CAPM (Capital Asset Pricing Model) Introduction to the CAPM: Theory of the model and its importance in finance The relationship between an asset's expected return and its systematic risk (beta) Estimating an Asset's Beta: Using linear regression to estimate beta in relation to a market index 4.3 Practical exercise: Estimate the beta of a stock and interpret the results within the CAPM framework Pedagogical methodology Experimental 01 Lecture-based 03 Approache Including the use of real data to instruction support theoretical concepts, Present the fundamental basics enhance both the relevance and of econometrics for finance in a understanding of the subjects clear, directive manner over the studied. This method bridges 3 sessions for the entire cohort theory and practice, allowing students to grasp complex ideas through practical, data- driven analysis. 02 Interactive Approache Group projects, and debates foster collaboration, critical thinking, and the practical application of financial concepts, making them essential tools for student engagement and understanding. Team projects on financial model applications using R and EXCEL For the team projects on financial model applications using R and Excel, each group of five students is expected to prepare a project that covers the various econometric concepts we will discuss. The project should focus on a real-world phenomenon and begin with (structure of the project): I. The theoretical foundation II. Descriptive analysis (source of your Data…) III. Data visualization using R and Excel. IV. Performing a linear regression model in R to examine the chosen phenomenon. V. Financial and economic significance of the results: Interpretation of the results. Examples of phenomena that can be explored using a simple regression model (with one dependent variable Y and one independent variable X) include: 1. Inflation and interest rates : Y = Inflation, X = interest rates 2. Stock prices and interest rates: Y = stock prices, X = interest rates. 3. Company revenue and advertising expenditure: Y = company revenue, X = advertising expenditure. 4. GDP growth and inflation: Y = GDP growth rate, X = inflation rate. 5. Electricity consumption and temperature: Y = electricity consumption, X = average temperature…. Main References Stock, J. H., & Watson, M. W. (2019). Introduction to econometrics (4th ed.). Pearson. 9780136879787 Brooks, C. (2019). Introductory econometrics for finance (4th ed.). Cambridge University Press. https://doi.org/10.1017/9781108524872 Chapter 1: Introduction ‘Introductory Econometrics for Finance’ © 12 Chris Brooks 2008 Bridging Theory and Practice: The Essential Tools for Economists Introduction: The Nature and Purpose of Econometrics What is Econometrics? Literal meaning is “measurement in economics”. Definition of financial econometrics: The application of statistical and mathematical techniques to problems in finance. ‘Introductory Econometrics for Finance’ © Chris Brooks 2008 Main fields of econometrics Modeling: Correlation Statistical vs causality inference 01 02 Econometrics helps reveal This involves drawing relationships between economic conclusions about a broader variables that might not be population based on the immediately obvious or characteristics of a sample anticipated Main fields of Forecasting Simulation econometrics To predict future financial trends Assessing how changes in one based on historical data, helping us anticipate and prepare for future developments. 04 03 variable can impact another, providing a way to model different scenarios and their potential outcomes. ‘Introductory Econometrics for Finance’ © Chris Brooks 2008 Examples of the kind of problems that may be solved by an Econometrician 1. Forecasting Asset Prices: Predicting future prices of assets like gold, currencies, or cryptocurrencies (e.g., Bitcoin) based on past trends and market data. 2. Measuring the Impact of Interest Rates: Analyzing how changes in interest rates affect stock market prices or housing markets. 3. Evaluating Investment Risk: Estimating the risk associated with different investments, such as stocks, bonds, or mutual funds, to help investors make informed decisions. 4. Predicting Company Stock Performance: Using financial data to estimate how a company’s stock price might react to new earnings reports or major news events. 5. Assessing Portfolio Diversification: Analyzing how mixing different types of investments (stocks, bonds, etc.) can reduce risk while maximizing returns. 6. Analyzing Market Trends: Identifying long-term trends in financial markets (e.g., stock market or commodity prices) to guide investment strategies. 7. Evaluating Economic Policies: Studying how government policies (like tax changes) impact inflation, currency values, or stock market performance. ‘Introductory Econometrics for Finance’ © Chris Brooks 2008 Financial econometrics The four pillars of financial econometrics Econometrics software Financial Data Models Financial theory (R, STATA…) Financial Data: What are the Special Characteristics Financial data refers to the quantitative information related to the financial performance, position, and transactions of an individual, organization, or economy. 1) Frequency & quantity of data Stock market prices are measured every time a transaction takes place or someone publishes a new quotation (“supply vs. demand”). ‘Introductory Econometrics for Finance’ © Chris Brooks 2008 Financial Data: What are the Special Characteristics 2) Quality While recorded asset prices reflect the actual transaction prices, financial data can be quite "noisy." This means that even though we have precise transaction prices, the data can include random fluctuations and errors, making it sometimes hard to interpret (Inefficiency of Markets Theory). o Behavioral Biases: Investors often act irrationally, with biases like overconfidence o Information Asymmetry: Not all market participants have access to the same information, causing prices to adjust unevenly. o Market Frictions: Transaction costs, taxes, and liquidity constraints delay the adjustment of prices to new information. ‘Introductory Econometrics for Finance’ © Chris Brooks 2008 Financial Data Types and structures Data Types 1. The data may be quantitative (e.g. exchange rates, stock prices, number of shares outstanding), qualitative (e.g. day of the week). 2. The data may be discrete (e.g. number of trades, number of bonds) or continuous (e.g. stock prices, interest rates). 3. The data may be ordinal (e.g. risk levels like low, medium, high) or nominal (e.g. different investment types like stocks, bonds, and real estate). Data Structure 1. The data may be time series (e.g. daily stock prices over a year), cross-sectional (e.g. stock prices of different companies on a single day), or panel data (e.g. stock prices of the same companies tracked monthly over several years). Financial Data Types Financial Data Structures Financial Data Data type Time Panel series Datadata Cross-sectional data Allows us to take into consideration: Time-indexed 1- The impactdata, of individual for use in No information on the impact of Refers No information to data observed on adjustment at the same Panel data advantages Definition Limits problems characteristics involving (individual time series: individual characteristics time for different dynamicsindividuals. forecasting, modeling, etc. heterogeneity) 2- Adjustment dynamics Financial Data structure: Time series As shown in the following table, we have the same entity 'i' observed across different time periods 't' Financial Data structure: Time series Financial Data structure: Cross- sectional data In contrast to the time series structure, cross-sectional data pertains to the observation of different entities (denoted as "i") during the same period “t”. Financial Data structure: Cross- sectional data Cross-sectional data captures a snapshot of many things at one point in time. This figure presents the popularity of different cryptocurrencies at a specific moment. Financial Data structure: Panel Data The combination of the two previous types of data—time series and cross-sectional data— is referred to as panel data Source of Financial Data 1. The World Bank : https://data.worldbank.org/ 2. International Monetary Fund: https://data.imf.org/ 3. Yahoo Finance: finance.yahoo.com 4. Our World in Data: https://ourworldindata.org/ Practical exercices: quantitative Data Exercise 1: Data Manipulation and Handling Missing Values (Excel) Objective: Introduce students to basic data manipulation and handling missing values using Excel, with a focus on distinguishing between different data structures. Task: I. Download Financial Data: II. Go to the World Bank website: https://data.worldbank.org/ 1. Download three types of data structures: ◦ Time Series Data: Market capitalization of listed domestic companies (current US$); Listed domestic companies, total; GDP (constant 2015 US$) for Morocco from 2010 to 2022. ◦ Cross-Sectional Data: Market capitalization of listed domestic companies (current US$); Listed domestic companies, total; GDP (constant 2015 US$) for North African countries for the year 2022. ◦ Panel Data: Market capitalization of listed domestic companies (current US$); Listed domestic companies, total; GDP (constant 2015 US$) for North African countries from 2010 to 2023. III.Import Data into Excel: 1. Open Excel and import the downloaded datasets. https://data.worldbank.org/ interface https://data.worldbank.org/ search toolbar Search results Specify search results for specific countries: Morocco Financial Data extraction Data Bank interface Practical exercices Reorganize imported data in three structures 1. Time Series Data: Market capitalization of listed domestic companies (current US$); Listed domestic companies, total; GDP (constant 2015 US$) for Morocco from 2010 to 2022. 2. Cross-Sectional Data: Market capitalization of listed domestic companies (current US$); Listed domestic companies, total; GDP (constant 2015 US$) for North African countries for the year 2022. 3. Panel Data: Market capitalization of listed domestic companies (current US$); Listed domestic companies, total; GDP (constant 2015 US$) for North African countries from 2010 to 2023. Financial Data structure: Time series As shown in the following table, we have the same entity 'i' observed across different time periods 't' Financial Data structure: Time series Financial Data structure: Cross- sectional data In contrast to the time series structure, cross-sectional data pertains to the observation of different entities (denoted as "i") during the same period “t”. Financial Data structure: Cross- sectional data Financial Data structure: Panel Data The combination of the two previous types of data—time series and cross-sectional data— is referred to as panel data Financial Data structure: Panel Data Practical exercices: quantitative Data I. Data Manipulation Tasks: Use the time series data for Morocco (2010-2022) for further analysis 1. Rename Variables: Ensure the column names are descriptive and clear. 2. Handle Missing Data: ◦ Identify any missing values in all datasets. ◦ Decide on a method to handle missing data (e.g., remove rows with missing values or use mean imputation). ◦ Apply the chosen method to each dataset accordingly. 3. Create New Variables: ◦ For the time series data: Calculate percentage changes or growth rates as needed. II. Save Your Work: ◦ Save the manipulated datasets and ensure all changes are clearly documented. Handling missing values Create new variables: Calculate percentage changes or logarithms Definition: A percentage change measures the relative change in a value over time, expressed as a percentage of the initial value. It's often used to compare changes in variables between two specific points in time. For the time series Market capitalization of listed domestic companies (current US$) and GDP (constant 2015 US$) for Morocco: Calculate percentage in Excel Create New Variables: Calculate percentage changes Exercise 2: Descriptive Statistics, Plotting Financial Data, and Correlation/Covariance (R) Objective: Analyze financial data using Excel to perform descriptive statistics, create visualizations, and compute correlation and covariance. Task 1: Descriptive Statistics 1.Import Data: 1. Load the time series datasets for the three countries on “Market capitalization of listed domestic companies (current US$); Listed domestic companies, total; GDP (constant 2015 US$) ” into R. 2.Descriptive Statistics: 1. Calculate the following descriptive statistics for each dataset: 1. Mean 2. Median 3. Standard deviation 4. Minimum and maximum values Get started : R philosophy R is a software for econometrics and data analysis, equipped with several essential features for both professional and academic environments. The R software is available for free and provides empirical solutions to major contemporary issues through programming. The philosophy of programming languages such as R is as follows: everything that can be automated should be automated. This approach minimizes manual manipulations by utilizing pre-prepared packages within the software. The goal is to spend as much time as possible thinking about how to develop your package rather than focusing on the details of the package structure. Automation refers to the use of technology to perform repetitive tasks with minimal human intervention, improving efficiency and reducing errors. Download R Studio: https://posit.co/download/rstudio-desktop/ Get started : What is a packages? Packages in R Packages in R Programming language are a set of R functions, compiled code, and sample data. These are stored under a directory called “library” within the R environment. By default, R installs a group of packages during installation. Once we start the R console, only the default packages are available by default. Other packages that are already installed need to be loaded explicitly to be utilized by the R program that’s getting to use them. Thus the need to use the command : Packages source: The Comprehensive R Archive Network (CRAN) “more than 20.000 packages” install.packages(“name of the package") library(name of the package) Get started with R : interface Import Data I. Importing Data in R Load the time series datasets for three countries on: Market Capitalization of Listed Domestic Companies (current US$) Listed Domestic Companies, Total GDP (constant 2015 US$) To import data into R, you can use two methods: Method 1: Using the R Toolbar Path: File > Import Dataset > From Excel Method 2: Using the readxl package 1. Install the package: install.packages("readxl") 2. Load the "readxl" package: library(readxl) 3. Use the "read_excel" function to specify the path to your file: "C:\Users\Zakaria\Desktop\UIR\Financial Data Example.xlsx“If this command generates an error message, use the following command : data