CLRM Assumptions & Diagnostics PDF
Document Details
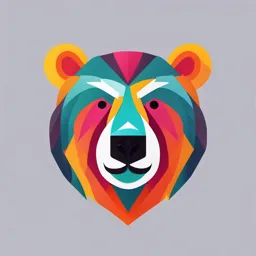
Uploaded by DelectableLearning8178
null
Chris Brooks
Tags
Related
Summary
This document explores the assumptions of classical linear regression models (CLRM) and diagnostics for potential violations. It covers topics such as homoscedasticity, autocorrelation, multicollinearity, and functional form specification. The author also provides tests for detecting these issues and discusses the remedies available.
Full Transcript
Chapter 4 Classical linear regression model assumptions and diagnostics 1 Violation of the Assumptions of the CLRM Recall that we assumed of the CLRM disturbance terms: 1. 𝐸(𝜀𝑡 ) = 0 2. 𝑉𝑎𝑟(𝜀𝑡 ) = 𝜎 2 < ∞...
Chapter 4 Classical linear regression model assumptions and diagnostics 1 Violation of the Assumptions of the CLRM Recall that we assumed of the CLRM disturbance terms: 1. 𝐸(𝜀𝑡 ) = 0 2. 𝑉𝑎𝑟(𝜀𝑡 ) = 𝜎 2 < ∞ 3. 𝑐𝑜𝑣(𝜀𝑖 , 𝜀𝑗 ) = 0 4. The X matrix is non-stochastic or fixed in repeated samples 5. 𝜀𝑡 N(0,2) 2 Investigating Violations of the Assumptions of the CLRM We will now study these assumptions further, and in particular look at: - How we test for violations - Causes - Consequences In general we could encounter any combination of 3 problems: - the coefficient estimates are wrong - the associated standard errors are wrong - the distribution that we assumed for the test statistics will be inappropriate - Solutions - the assumptions are no longer violated - we work around the problem so that we use alternative techniques which are still valid 3 Assumption 1: E(𝜀𝑡 ) = 0 Assumption that the mean of the disturbances is zero. For all diagnostic tests, we cannot observe the disturbances and so perform the tests of the residuals. The mean of the residuals will always be zero provided that there is a constant term in the regression. 4 Assumption 2: Var(𝜀𝑡 ) = 2 < We have so far assumed that the variance of the errors is constant, 2 - this is known as homoscedasticity. ˆ + t If the errors do not have a constant variance, we say that they are heteroscedastic e.g. say we estimate a regression. calculate the residuals, (𝜀𝑡 ) and x 2t - 5 Detection of Heteroscedasticity: The GQ Test Graphical methods Formal tests: There are many of them: we will discuss Goldfeld-Quandt test and White’s test test is carried out as follows. 1. Split the total sample of length T into two sub-samples of length T1 and T2. The regression model is estimated on each sub-sample and the two residual variances are calculated. 2. The null hypothesis is that the variances of the disturbances are equal, H 0: 1 2 2 2 6 The GQ Test (Cont’d) 3. The test statistic, denoted GQ, is simply the ratio of the two residual variances where the larger of the two variances must be placed in the numerator. s12 GQ 2 s2 4. The test statistic is distributed as an F(T1-k, T2-k) under the null of homoscedasticity. 5. A problem with the test is that the choice of where to split the sample is that usually arbitrary and may crucially affect the outcome of the test. 7 Detection of Heteroscedasticity using White’s Test White’s general test for heteroscedasticity is one of the best approaches because it makes few assumptions about the form of the heteroscedasticity. The test is carried out as follows: 1. Assume that the regression we carried out is as follows yt = 1 + 2x2t + 3x3t + 𝜀𝑡 And we want to test Var(𝜀𝑡 ) = 2. We estimate the model, obtaining the residuals, 𝜀𝑡Ƹ 2. Then run the auxiliary regression ˆt2 1 2 x2t 3 x3t 4 x22t 5 x32t 6 x2t x3t vt 8 Performing White’s Test for Heteroscedasticity 3. Obtain R2 from the auxiliary regression and multiply it by the number of observations, T. It can be shown that T R2 2 (m) where m is the number of regressors in the auxiliary regression excluding the constant term. 4. Decision rule: If the 2 test statistic from step 3 is greater than the corresponding value from the statistical table then reject the null hypothesis that the disturbances are homoscedastic. 9 Consequences of Using OLS in the Presence of Heteroscedasticity OLS estimation still gives unbiased coefficient estimates, but they are no longer BLUE. The Ordinary Least Squares (OLS) estimator is the Best Linear Unbiased Estimator. This means: - Best: It has the smallest variance among all linear estimators. - Linear: It is a linear function of the data. - Unbiased: It gives the correct estimate on average (the expected value equals the true parameter). - Estimator: it is used to estimate the coefficients (β). This property holds when certain assumptions (such as no perfect multicollinearity, homoscedasticity, and no autocorrelation of errors) are met. 10 Consequences of Using OLS in the Presence of Heteroscedasticity This implies that if we still use OLS in the presence of heteroscedasticity, our standard errors could be inappropriate and hence any inferences we make could be misleading. Whether the standard errors calculated using the usual formulae are too big or too small will depend upon the form of the heteroscedasticity. 11 How Do we Deal with Heteroscedasticity? If the form (i.e. the cause) of the heteroscedasticity is known, then we can use an estimation method which takes this into account (called generalised least squares, GLS). A simple illustration of GLS is as follows: Suppose that the error variance is related to another variable zt by var t 2 zt2 To remove the heteroscedasticity, divide the regression equation by zt yt 1 x x 1 2 2t 3 3t vt zt zt zt zt t where vt is an error term. zt t var t 2 zt2 Now var vt var 2 2 2 for known zt. zt zt zt So the disturbances from the new regression equation will be homoscedastic. 12 Background – The Concept of a Lagged Value t yt yt-1 yt 1989M09 0.8 - - 1989M10 1.3 0.8 1.3-0.8=0.5 1989M11 -0.9 1.3 -0.9-1.3=-2.2 1989M12 0.2 -0.9 0.2--0.9=1.1 1990M01 -1.7 0.2 -1.7-0.2=-1.9 1990M02 2.3 -1.7 2.3--1.7=4.0 1990M03 0.1 2.3 0.1-2.3=-2.2 1990M04 0.0 0.1 0.0-0.1=-0.1............ 13 Autocorrelation We assumed of the CLRM’s errors that Cov (𝜀 i , 𝜀 j) = 0 for ij, i.e. This is essentially the same as saying there is no pattern in the errors. Obviously we never have the actual u’s, so we use their sample counterpart, the residuals (the 𝜀𝑡Ƹ ’s). If there are patterns in the residuals from a model, we say that they are autocorrelated. Some stereotypical patterns we may find in the residuals are given on the next 3 slides. 14 Positive Autocorrelation + û t û t + - + uˆ t 1 Time - - Positive Autocorrelation is indicated by a cyclical residual plot over time. 15 Negative Autocorrelation + û t û t + - + uˆ t 1 Time - - Negative autocorrelation is indicated by an alternating pattern where the residuals cross the time axis more frequently than if they were distributed randomly 16 No pattern in residuals – No autocorrelation û t + + û t - uˆ t +1 - - No pattern in residuals at all: this is what we would like to see 17 Detecting Autocorrelation: The Durbin-Watson Test The Durbin-Watson (DW) is a test for first order autocorrelation - i.e. it assumes that the relationship is between an error and the previous one 𝜀𝑡 = 𝜌𝜀𝑡−1 + 𝑣𝑡 , where vt N(0, v2). The DW test statistic actually tests H0 : =0 and H1 : 0 The test statistic is calculated by σ𝑇 𝜀𝑡 −ො𝜀𝑡−1 )2 𝑡=2(ො 𝐷𝑊 = σ𝑇 ො 𝑡2 𝑡=2 𝜀 Rearranging for DW would give 0DW4. If DW = 2. So roughly speaking, do not reject the null hypothesis if DW is near 2 i.e. there is little evidence of autocorrelation Unfortunately, DW has 2 critical values, an upper critical value (du) and a lower critical value (dL), and there is also an intermediate region where we can neither reject nor not reject H0. 18 The Durbin-Watson Test: Interpreting the Results Conditions which Must be Fulfilled for DW to be a Valid Test 1. Constant term in regression 2. Regressors are non-stochastic 3. No lags of dependent variable 19 Another Test for Autocorrelation: The Breusch-Godfrey Test It is a more general test for rth order autocorrelation: 𝜀𝑡 = 𝜌1 𝜀𝑡−1 + 𝜌2 𝜀𝑡−2 + 𝜌3 𝜀𝑡−3 +... +𝜌𝑟 𝜀𝑡−𝑟 + 𝑣𝑡 𝑣𝑡 ~𝑁(𝜎𝑣2 ,0) The null and alternative hypotheses are: H0 : 1 = 0 and 2 = 0 and... and r = 0 H1 : 1 0 or 2 0 or... or r 0 The test is carried out as follows: 1. Estimate the linear regression using OLS and obtain the residuals, 𝜀𝑡Ƹ. 2. Regress 𝜀𝑡Ƹ on all of the regressors from stage 1 (the x’s) plus 𝜀𝑡−1 Ƹ , 𝜀𝑡−2 Ƹ ,... , 𝜀𝑡− Ƹ Obtain R2 from this regression. 3. It can be shown that (T-r)R2 2(r) If the test statistic exceeds the critical value from the statistical tables, reject the null hypothesis of no autocorrelation. 20 Consequences of Ignoring Autocorrelation if it is Present The coefficient estimates derived using OLS are still unbiased, but they are inefficient, i.e. they are not BLUE, even in large sample sizes. Thus, if the standard error estimates are inappropriate, there exists the possibility that we could make the wrong inferences. R2 is likely to be inflated relative to its “correct” value for positively correlated residuals. 21 “Remedies” for Autocorrelation If the form of the autocorrelation is known, we could use a GLS procedure – i.e. an approach that allows for autocorrelated residuals e.g., Cochrane-Orcutt. But such procedures that “correct” for autocorrelation require assumptions about the form of the autocorrelation. If these assumptions are invalid, the cure would be more dangerous than the disease! - see Hendry and Mizon (1978). However, it is unlikely to be the case that the form of the autocorrelation is known, and a more “modern” view is that residual autocorrelation presents an opportunity to modify the regression. 22 Multicollinearity This problem occurs when the explanatory variables are very highly correlated with each other. Perfect multicollinearity Cannot estimate all the coefficients - e.g. suppose x3 = 2x2 and the model is yt = 1 + 2x2t + 3x3t + 4x4t + 𝜀𝑡 Problems if Near Multicollinearity is Present but Ignored - R2 will be high but the individual coefficients will have high standard errors. - The regression becomes very sensitive to small changes in the specification. - Thus confidence intervals for the parameters will be very wide, and significance tests might therefore give inappropriate conclusions. 23 Measuring Multicollinearity Method 1: The easiest way to measure the extent of multicollinearity is simply to look at the matrix of correlations between the individual variables. e.g. Corr x2 x3 x4 x2 - 0.2 0.8 x3 0.2 - 0.3 x4 0.8 0.3 - But another problem: if 3 or more variables are linear - e.g. x2t + x3t = x4t Note that high correlation between y and one of the x’s is not muticollinearity. Method 2: Variance inflationary factor 24 Measuring Multicollinearity Solutions to the Problem of Multicollinearity “Traditional” approaches, such as ridge regression or principal components. But these usually bring more problems than they solve. Some econometricians argue that if the model is otherwise OK, just ignore it The easiest ways to “cure” the problems are - drop one of the collinear variables - transform the highly correlated variables into a ratio - go out and collect more data e.g. - a longer run of data - switch to a higher frequency 26 Adopting the Wrong Functional Form We have previously assumed that the appropriate functional form is linear. This may not always be true. We can formally test this using Ramsey’s RESET test, which is a general test for mis-specification of functional form. Essentially the method works by adding higher order terms of the fitted values (e.g. yt2 , yt3 etc.) into an auxiliary regression: Regress 𝜀𝑡Ƹ on powers of the fitted values: 𝑝 𝜀𝑡Ƹ = 𝛽0 + 𝛽1 𝑦ො𝑡2 + 𝛽2 𝑦ො𝑡3 +... +𝛽𝑝−1 𝑦ො𝑡 + 𝑣𝑡 Obtain R2 from this regression. The test statistic is given by TR2 and is distributed as a 2 ( p 1). So if the value of the test statistic is greater than a 2 ( p 1) then reject the null hypothesis that the functional form was correct. 27 But what do we do if this is the case? The RESET test gives us no guide as to what a better specification might be. One possible cause of rejection of the test is if the true model is yt 1 2 x2t 3 x22t 4 x23t t In this case the remedy is obvious. Another possibility is to transform the data into logarithms. This will linearise many previously multiplicative models into additive ones: yt Axt e t ln yt ln xt t 28 Testing the Normality Assumption Why did we need to assume normality for hypothesis testing? Testing for Departures from Normality The Bera Jarque normality test A normal distribution is not skewed and is defined to have a coefficient of kurtosis of 3. The kurtosis of the normal distribution is 3 so its excess kurtosis (b2-3) is zero. Skewness and kurtosis are the (standardised) third and fourth moments of a distribution. 29 Testing for Normality Bera and Jarque formalise this by testing the residuals for normality by testing whether the coefficient of skewness and the coefficient of excess kurtosis are jointly zero. It can be proved that the coefficients of skewness and kurtosis can be expressed respectively as: 𝐸[𝜀3 ] 𝐸[𝜀4 ] 𝑏1 = 𝑎𝑛𝑑 𝑏2 = (𝜎2 )3/2 (𝜎 2 )2 The Bera Jarque test statistic is given by b12 b2 32 W T ~ 2 2 6 24 We estimate b1 and b2 using the residuals from the OLS regression, 𝜀𝑡Ƹ. 30 What do we do if we find evidence of Non-Normality? It is not obvious what we should do! Could use a method which does not assume normality, but difficult and what are its properties? Often the case that one or two very extreme residuals causes us to reject the normality assumption. An alternative is to use dummy variables. e.g. say we estimate a monthly model of asset returns from 1980-1990, and we plot the residuals, and find a particularly large outlier for October 1987: ‘Introductory Econometrics for Finance’ © Chris Brooks 2013 31 What do we do if we find evidence of Non-Normality? (cont’d) û t + Oct Time 1987 - Create a new variable: D87M10t = 1 during October 1987 and zero otherwise. This effectively knocks out that observation. But we need a theoretical reason for adding dummy variables. 32 Omission of an Important Variable or Inclusion of an Irrelevant Variable Omission of an Important Variable Consequence: The estimated coefficients on all the other variables will be biased and inconsistent unless the excluded variable is uncorrelated with all the included variables. Even if this condition is satisfied, the estimate of the coefficient on the constant term will be biased. The standard errors will also be biased. Inclusion of an Irrelevant Variable Coefficient estimates will still be consistent and unbiased, but the estimators will be inefficient. 33 Parameter Stability Tests So far, we have estimated regressions such as yt = 1 + 2x2t + 3x3t + ut We have implicitly assumed that the parameters (1, 2 and 3) are constant for the entire sample period. We can test this implicit assumption using parameter stability tests. The idea is essentially to split the data into sub-periods and then to estimate up to three models, for each of the sub-parts and for all the data and then to “compare” the RSS of the models. There are two types of test we can look at: - Chow test (analysis of variance test) - Predictive failure tests 34 The Chow Test The steps involved are: 1. Split the data into two sub-periods. Estimate the regression over the whole period and then for the two sub-periods separately (3 regressions). Obtain the RSS for each regression. 2. The restricted regression is now the regression for the whole period while the “unrestricted regression” comes in two parts: for each of the sub- samples. We can thus form an F-test which is the difference between the RSS’s. RSS RSS1 RSS2 T 2k The statistic is RSS1 RSS2 k 35 The Chow Test (cont’d) where: RSS = RSS for whole sample RSS1 = RSS for sub-sample 1 RSS2 = RSS for sub-sample 2 T = number of observations 2k = number of regressors in the “unrestricted” regression (since it comes in two parts) k = number of regressors in (each part of the) “unrestricted” regression 3. Perform the test. If the value of the test statistic is greater than the critical value from the F-distribution, which is an F(k, T-2k), then reject the null hypothesis that the parameters are stable over time. 36 A Chow Test Example Consider the following regression for the CAPM (again) for the returns on Glaxo. Say that we are interested in estimating Beta for monthly data from 1981-1992. The model for each sub-period is 1981M1 - 1987M10 0.24 + 1.2RMt T = 82 RSS1 = 0.03555 1987M11 - 1992M12 0.68 + 1.53RMt T = 62 RSS2 = 0.00336 1981M1 - 1992M12 0.39 + 1.37RMt T = 144 RSS = 0.0434 37 A Chow Test Example - Results The null hypothesis is H0 : 1 2 and 1 2 The unrestricted model is the model where this restriction is not imposed 00434. 00355. 000336. 144 4 Test statistic 00355. 000336. 2 = 7.698 Compare with 5% F(2,140) = 3.06 We reject H0 at the 5% level and say that we reject the restriction that the coefficients are the same in the two periods. 38