Introductory Econometrics PDF - Winter Term 2024-25
Document Details
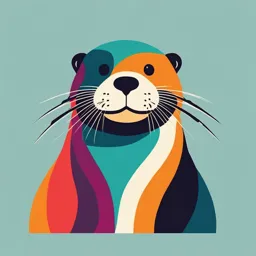
Uploaded by AwesomeCarnelian4810
University of Vienna
2024
Simon Martin
Tags
Summary
These are lecture notes on introductory econometrics. The course is taught by Simon Martin at the University of Vienna. It discusses various topics, including field experiments, policy evaluations, forecasting, data requirements, and data analysis. The lecture notes also include information on the course material, assessment procedure, and literature.
Full Transcript
Introductory Econometrics Simon Martin University of Vienna Winter Term 2024-25 The slides for this course are courtsey to Tomaso Duso (DIW Berlin), Martin Halla (WU Vienna)...
Introductory Econometrics Simon Martin University of Vienna Winter Term 2024-25 The slides for this course are courtsey to Tomaso Duso (DIW Berlin), Martin Halla (WU Vienna), Liyang Sun (CEMFI), Jesse M. Shapiro (Harvard and NBER), and Andrea Weber (CEU) and are based on Wooldridge (2022) and on slides provided by Pearson on Stock and Watson (2020). All errors are mine. S. Martin: Introductory Econometrics 1 / 64 Welcome to Introductory Econometrics Aims and content ▶ This course provides an understanding of standard econometric methods. Knowledge of these methods allows one to understand modern empirical economic literature and to perform one's own empirical analysis of cross-sectional, time series, and panel data. ▶ good working knowledge of After following this course, students will have a the key properties of standard econometric methods, including Least Squares Estimation, Instrumental Variables Estimation, and Maximum Likelihood, and their use in various applications. Target group: master students from Applied Economics (913 ), Banking and Finance (974), Research in Economics and Finance (953 ), and Philosophy and Economics (642). Preliminaries: the course is a rst-year master-level course in econometrics for students who already have a background in statistics and are familiar with the basic principles of probability theory, mathematical statistics and linear regression. S. Martin: Introductory Econometrics 2 / 64 Welcome to Introductory Econometrics The web-page of the course is: https://moodle.univie.ac.at/course/view.php?id=435528 Regular meetings: Tuesday 15-16.30h HS 14 and Thursday 15-16.30h HS 4 Uebung: Philipp Gersing and Klara Weinhappl in this class we will learn the methods and in the Uebung we will apply them to data R-Online Tutorial: Dario Cavarretta and Niklas Kirsamer ▶ https://moodle.univie.ac.at/course/view.php?id=436072 slides and other material are available in moodle S. Martin: Introductory Econometrics 3 / 64 Assignments and Evaluation Attendance ▶ unexcused absence from the rst session will automatically lead to deregistration in order to allow students on the waiting list to move up; ▶ if you are unable to attend the rst session, you must notify me in advance via email in order to continue attending the course Assessment ▶ The assessment consists of 2 tests during the semester (midterm, nal exam each 45%) and homework (2 exercises in groups of up to 4, each 5%). ▶ Dates for the 2 tests: 15.11.2024, and 31.01.2025 each taking 60 minutes ▶ Students who either failed (i.e., obtained less than 50%) or missed one of the two exams during the semester are eligible to participate in the retake exam on 12.02.2025, registration by 06.02.2025. The result of the retake exam replaces the worse of the two exams during the semester. S. Martin: Introductory Econometrics 4 / 64 Assignments and Evaluation Exams (midterm, nal exam each 45%) ▶ Onsite ▶ The questions of the 2 exams will refer to general material covered in the course, analytical derivations, and interpretations of empirical results. Homework (2 exercises in groups of 3 to 4, each 5%) ▶ Exercise due dates: November 22nd and January 24th ▶ Upload a video with answers for the 2 exercises ▶ Self selection of groups and submission via Moodle - make sure to arrange groups early! ▶ Further details on exercises in a few weeks Grading ▶ To pass the course, a minimum level of 50% has to be reached. ▶ 1: [87.5-100%]; 2: [75-87.5%); 3: [62.5-75%); 4: [50-62.5%); 5: [0-50%) S. Martin: Introductory Econometrics 5 / 64 Example: Field experiments general procedure: treatment vs control group ▶ random (or quasi-random) assigment ▶ treatment and control group Paper: Eects of market size and competition in two-sided markets: evidence from online dating (Fong 2024) ▶ Online Dating with network eects ▶ in general: dicult to measure ⇒ randomized experiment with treatment and control group regarding information provision S. Martin: Introductory Econometrics 6 / 64 Fong 2024: App/Experiment "mobile online dating app that has millions of active users worldwide" S. Martin: Introductory Econometrics 7 / 64 Example: Policy evaluation industrial policy: Identifying Agglomeration Spillovers: Evidence from Winners and Losers of Large Plant Openings (Greenstone, Hornbeck and Moretti 2010) winners and runner-ups S. Martin: Introductory Econometrics 8 / 64 Example: Cash transfer program in Kenya Moscona, Jacob, and Awa Ambra Seck. "Age Set versus Kin: Culture and Financial Ties in East Africa." American Economic Review 114, no. 9 (2024): 2748-2791. S. Martin: Introductory Econometrics 9 / 64 Example: Forecasting The methods we discuss are the basis for heavily used analysis forecasting tools, e.g., demand, banking,... S. Martin: Introductory Econometrics 10 / 64 Why to attend this class? Working with data is important and also makes fun (most of the times) to try to extrapolate information and make sensible claims about things you observe i. Does higher education causes higher income? ii. Does beauty implies higher chances to get a job? iii. Does regulation in the mobile telecommunication markets implies lower prices, better quality, and higher welfare for consumers iv. Does a minimum wages increase or decrease employment? As for everything nice there is a price to pay... the use of formal language and derivations are unavoidable + data work After completing the module you will command the basic technical tools which enable you to understand and assess empirical studies These skills are also very valuable in practice for the assessment of any (economic) policy discussion + helpful when writing a thesis with an empirical focus S. Martin: Introductory Econometrics 11 / 64 Literature Main books Stock, J. H., and Watson, M. W. (2020), Introduction to Econometrics, Global Edition. Pearson Education Limited Wooldridge, Jerey M. Introductory econometrics: A modern approach. 7th edition, Cengage learning, 2020. Additional books: Angrist, J.D. and Pischke, J.-S. (2009): Mostly Harmless Econometrics: An Empiricst's Companion, Princeton University Press. Cunningham, Scott. Causal inference: The mixtape. Yale university press, 2021. Greene, W.H. (2019): Econometric Analysis, 8th edition, Pearson. Wooldridge, Jerey M. Econometric analysis of cross section and panel data. MIT press, 2010. Hanck, C., Arnold, M., Gerber, A., and Schmelzer, M. (2020): Introduction to Econometrics with R, Online book on : https://www.econometrics-with-r.org/. Based on Stock, J. H., and Watson, M. W. (2015), Introduction to Econometrics, Global Edition. Pearson Education Limited. Heiss, F. (2020): Using R for Econometrics. Online book on http://www.urfie.net/. Based on Wooldridge, J.M. (2019), Introductory Econometrics, Cengage Learning, Boston, MA. S. Martin: Introductory Econometrics 12 / 64 Course Plan Basic econometric tools 1 Linear regression model with single regressor 2 Multivariate regression 3 OLS estimator & its properties 4 Dierence-in-dierence, experiments 5 Problems: Multicollinearity, heteroskedasticity, GLS, clustering 6 OLS with time series More advanced econometric tools 1 Panel data methods 2 Endogeneity and instrumental variables estimation (IV) 3 Systems of Equations 4 Quasi-experiments 5 Maximum likelihood estimation 6 Discrete choice models 7 Sample selection Advanced time series analysis 1 Forecasting 2 Dynamic causal eects S. Martin: Introductory Econometrics 13 / 64 Unit 1: Introduction to estimation and testing S. Martin: Introductory Econometrics 14 / 64 Outline 1 Introduction 2 Identication, estimation, testing 3 Data structure 4 Appendix Construction of means Appendix: Condence intervals and statistical testing S. Martin: Introductory Econometrics 15 / 64 The purpose of scientic research Formulate a research question Choose the research method and answer the questions with the help of i. Theoretical models ii. Empirical models and data Reality too complex ⇒ simplifying assumptions As simple as possible, as complex as necessary Good research ⇒ right balance Model, Research design/methodology S. Martin: Introductory Econometrics 16 / 64 Objectives and methods of economic research Traditionally the tasks are 1 Explanation of human behavior under shortages/limited resources 2 Explanation of economic relations 3 Forecast developments Shortages in terms of materiel and non-material aspects Theoretical economic research uses (mathematical) models Empirical economic research uses econometric models/methods Econometrics combines 1 Economic theory 2 Mathematics 3 Statistics S. Martin: Introductory Econometrics 17 / 64 History of econometrics The term was formed by Ragnar Fischer and Joseph Schumpeter in the early 1930's Foundation of the Econometric Society and the Journal Econometrica in 1933 Famous Nobel prize Laureates 1980 Lawrence R. Klein, for "the probability theory foundations of econometrics and the analysis of simultaneous economic structures" 1989 Trygve Haavelmo, for "the analysis of economic uctuations and economic policies" 2000 James J. Heckman, Daniel L. McFadden, for "the analysis selective samples and discrete selection problems" 2003 Robert F. Engle III and Clive Granger, for "the analysis of economic time series (ARCH-Models and Co-integration)" 2021 David Card, Joshua Angrist, and Guido Imbens for "their methodological contributions to the analysis of causal relationships" S. Martin: Introductory Econometrics 18 / 64 Why do we need econometrics? Two purposes: 1 To try predict the future (forecasting) ▶ Central banks engage routinely in this exercise ▶ Market makers too: e.g. useful to know what tomorrow interest rate (or stock market) is likely to be: can allow making huge prots ▶ Whatever helps predict what you want to predict should be used 2 To understand what causes what and to answer questions of the sort: if X occurs then what happens to y? ▶ Economic theory is about causation, but there are many conicting theories ▶ Even if only one theory is available, theory (normally) provides only qualitative statements about direction of eects, not their size ▶ To sort out which explanation is true, or to pin down the size of the eects we rely on econometrics. ▶ Causality is critical for policy S. Martin: Introductory Econometrics 19 / 64 Cause and Eect Correlation vs. Causality Example (Forecasting rain and explaining rain) ▶ You may be interested in rain determinants if you want to raise rain fall in sub-Saharan countries as a development policy ▶ You observe in your city (Washington, where the World Bank is located?) that when many people carry their umbrellas it usually rains ▶ You could be tempted to propose endow sub-Saharans with umbrellas or subsidize their adoption ⇒ not a great policy! ▶ Problem: reverse causality Two important points 1 Correlation and causality are two dierent things 2 y(rain) may cause X(people with umbrellas) even if X takes place before y (reverse causality) S. Martin: Introductory Econometrics 20 / 64 Cause and Eect Figure: Causality determinants of the speed of the truck 1 causation: speed of the truck = f(strength of the person on the left) 2 correlation: speed of the truck = f(strength of the person on the right) S. Martin: Introductory Econometrics 21 / 64 Cause and Eect Linear regression let us estimate the population regression line and its slope, eg. in a single regression model: y = β0 + β1 · x + u wage function: wage = β0 + β1 · education + u , where we expect β1 ≥ 0 Example: education and income (positive correlation) 1 Causal relation: education → income 2 Inverse causal relation: income → education 3 Confounding factor: e.g. intelligence/ability S. Martin: Introductory Econometrics 22 / 64 How can we Estimate Causal Eects? Want eect of X on distribution of y, other relevant things being held constant Most often (but not always) we are interested in eect on mean of y, i.e.: ∂E (y |X , ?) (1) ∂X Problem in econometrics: how do we get a reliable estimate of this partial derivative? Reliable means: reects the eect on y of a change in X rather than something else What can threaten the causal interpretation of marginal eets? ▶ omitted variables: ability (wage regression), unobserved product charateristics (demand) ▶ selection into treatment: evaluation of policies ▶ endogeneity (vs exogeneity): demand and supply S. Martin: Introductory Econometrics 23 / 64 Empirical work: Derive statements about the population from a random sample 1 Identication: Choose appropriate assumptions 2 Estimation: Approximation of the parameter of interest 3 Testing: Goodness and precision of the estimation 1. Considerations based on economic theory ▶ which variable is the dependent variable, which are the explanatory variables ▶ functional form ▶ omitted variables bias/confounders ▶ endogeneity/exogeneity ▶ → regression equation: yi = β0 + β1 x1... + βk xk + u (we later also discuss identication of β1 in the context of enough variation in the data of x1 ) 2. and 3. are done using statistical tools S. Martin: Introductory Econometrics 24 / 64 Identication Identication assumptions: A minimal amount of (non-testable) assumptions have to be made (theoretical model → direction of causality, functional form) Balance between too low and too strong restrictions ▶ no assumption(s): don't know what is the dependent variable, what the explanatory variable ▶ too strong assumptions: no estimations are necessary anymore Restrictive identication assumptions: ▶ assumption(s) correct: precise answers; precision increases with number of observations (N ) ▶ assumption(s) wrong: meaningless answers The higher N, the less restrictive assumptions are needed BUT: If the identication assumptions are fundamentally wrong (e.g. the direction of causality) N has no inuence S. Martin: Introductory Econometrics 25 / 64 To estimate, estimator, estimation To estimate/Estimation: The systematical attempt to draw preferably exact conclusions about the population (e.g. its moments) from a sample Estimator: A dened calculation rule. Estimators are random variables Estimate: Is a realization of the random variable (estimator) based on the data from a certain sample Identication → estimation: examples wage regression: individuals invest in their eduction to get higher wages, rms pay more educated indivduals higher wages as they expect them to be more productive → wage function: wage = β0 + β1 · education + u , where we expect β1 ≥ 0 (signialing theory gives same prediction) demand for cars: individuals maximize their utility and buy the car that gives them highest utilty → discrete choice models Phillips curve: relation between ination and unemployment, can be derived from a model of aggrgated demand and supply, short-run vs long-run S. Martin: Introductory Econometrics 26 / 64 Selection of the estimator Which estimator to choose? Relevant criteria ▶ Unbiasedness ▶ Eciency ▶ Consistency All criteria are based on the idea that repetitions of: 1 Drawing the sample 2 Estimating 3 Saving the results normally does not give identical results. All estimations will be dispersed around a certain value. This will give a Frequency distribution S. Martin: Introductory Econometrics 27 / 64 Unbiasedness Unbiasedness An estimator β̂ is unbiased if the expected value of the estimator is equal to the true unknown value β in the population E (β̂) − β = 0 E (β̂) − β is called the bias S. Martin: Introductory Econometrics 28 / 64 Erwartungstreue Identication f(b) verzerrter Schätzer unverzerrter Schätzer b ß Figure: Biased and unbiased estimator at E (β̂) = β Abbildung: Verzerrter und unverzerrter Schätzer bei E (β̂) = β Martin Halla (JKU) KS Empirische Wirtschaftsforschung - 2 7 / 58 S. Martin: Introductory Econometrics 29 / 64 Eciency The unbiasedness does not allow any statement about the deviation of an estimator around its expected value Eciency An unbiased estimator is ecient if it has the smallest variance among all alternative unbiased estimators β̃ Var (β̂) < Var (β̃) with E (β̂) = E (β̃) = β Among all unbiased estimators, the one with the smallest variance is to be preferred, it is the ecient one S. Martin: Introductory Econometrics 30 / 64 Effizienz Eciency f(b) b ß Figure: Which estimator is ecient? Abbildung: Welcher Schätzer ist der ineffiziente? Martin Halla (JKU) KS Empirische Wirtschaftsforschung - 2 9 / 58 S. Martin: Introductory Econometrics 31 / 64 Mean squared error The mean squared error (MSE) considers both,the dispersion (eciency) and the bias: MSE (β̂) = E (β̂ − β)2 2 = E (β̂) − β + Var (β̂) (2) Calculation: 2 MSE (β̂) = E (β̂ − β) h i2 =E β̂ − E (β̂) + E (β̂) − β h i2 h ih i h i2 =E (β̂ − E (β̂) + 2E (β̂ − E (β̂) E (β̂) − β +E E (β̂) − β | {z } =2[E (β̂)−E (β̂)][E (β̂)−β ]=0 h i2 2 2 =E (β̂ − E (β̂) + E (β̂) − β = Var (β̂) + E (β̂) − β | {z } =Var (β̂) S. Martin: Introductory Econometrics 32 / 64 Consistency Often it is cumbersome/impossible to obtain an unbiased estimator An estimator β̂N is consistent if, for an increasing number of observations (i.e. N → ∞), there is only a small probability that the estimator is not very close to the true value β (convergence in probability) In other words, if for an arbitrary ε > 0 the following holds: lim P |β̂N − β| > ε = 0 (3) N→∞ Unbiased estimators with a variance converging to zero for an increasing number of observations are consistent S. Martin: Introductory Econometrics 33 / 64 Consistency Figure: this sequence of estimators is consistent: the estimators are getting more and more concentrated near the true value; at the same time, these estimators are biased (Source: Wikipedia) S. Martin: Introductory Econometrics 34 / 64 Consistency Wooldridge says While not all useful estimators are unbiased, virtually all economists agree that consistency is a minimal requirement for an estimator. The famous econometrician Clive W.J. Granger once remarked: "If you cannot get it right as N goes to innity, you shouldn't be in this business". The implication is that, if your estimator of a particular population parameter is not consistent, then you are wasting your time. S. Martin: Introductory Econometrics 35 / 64 Data requirements Three central requirements have to be fullled: 1 Objectivity: The result of a measurement is independent from the observer 2 Reliability: Repeated measurements of the variables lead to the same (very similar) results 3 Validity: The measured variable are a valid operator for the theoretically examined quantities Empirical strategy Check with the help of descriptive statistics: ▶ the minimum and the maximum in particular ▶ mutual consistency Elimination of some data point ▶ impossible realizations ▶ typos/errors ▶ potential outliers S. Martin: Introductory Econometrics 36 / 64 Data - Units of observation Individuals Households Firms Cities Federal states Countries ⇒ The choice depends on the research question! S. Martin: Introductory Econometrics 37 / 64 How to get data? National statistical agencies / national banks International organizations ▶ European Union (statistical agency of the European Union(Eurostat), European Central Bank, etc.) ▶ United Nations (World Health Organization, World Bank, etc) ▶ World Trade Organization (WTO) Databases ▶ Inter-University Consortium for Political and Social Research (ICPSR) ▶ National Bureau of Economic Research (NBER), Data Library S. Martin: Introductory Econometrics 38 / 64 Data structure Cross-sectional data ▶ Information about several units of observation at one time period Pooled cross-sectional data ▶ Combination of cross-sectional data for several time periods Time series ▶ Information about one unit of observation for several time periods Panel- or Longitudinal data ▶ Information about the same units of observation at several time periods S. Martin: Introductory Econometrics 39 / 64 Cross-sectional data Sample of individuals, households, companies, regions or other units of observations, collected at a certain point in time. Ideally: Representative sample of independent units of a population Often these assumptions are violated ▶ Sample selection by self-selection; e.g. Wages of women. ▶ Peer group eects or neighborhood eects; e.g. learning success of pupils S. Martin: Introductory Econometrics 40 / 64 Cross-sectional data - Example Example Number Year Age Education Wage per hour 1 2008 23 9 9.60 2 2008 47 13 16.10 3 2008 48 12 14.20 4 2008 19 9 8.50 5 2008 56 9 13.90............... 99 2008 36 10 13.20 100 2008 39 13 15.30 S. Martin: Introductory Econometrics 41 / 64 Pooled cross-sectional data Pooled cross-sectional data have a time dimension. At dierent points in time a sample is taken from the population. Advantage: Higher number of observations, which yields more precision. Evaluation of economic policy measures is possible. S. Martin: Introductory Econometrics 42 / 64 Pooled cross-sectional data - Example Example Number Year Age Education Wage per hour 1 2008 23 9 9.60 2 2008 47 13 16.10 3 2008 48 12 14.20 4 2008 19 9 8.50............... 101 2009 56 9 14.90 102 2009 36 10 13.70 103 2009 39 13 15.60 103 2009 39 13 15. 90 S. Martin: Introductory Econometrics 43 / 64 Data maintenance - GSOEP Representative sample from private households in Germany In 2004, more than 12,000 households with nearly 24,000 persons participated in the census of GSOEP Eastern Germany was added in 1994/1995 by an immigration-sample To analyze only subgroups of the entire population, additional samples are possible The survey is divided in two parts: 1 Constant questions, e.g. employment and family history, life satisfaction 2 Yearly changing topics, e.g. environmental behavior, further education S. Martin: Introductory Econometrics 44 / 64 Data scale (Repetition) 1 Nominal scale ⇒ qualitative data ▶ Realizations can not be ordered, e.g. industry classes 2 Ordinal scale ▶ Realizations can be ordered, dierences have no meaning ▶ Often recoded into binary data in empirical analysis 3 Cardinal/interval scale ▶ Realizations can be expressed as multiples ▶ Optimal from an analytical point of view ▶ Realizations allow distinct quantitative interpretations S. Martin: Introductory Econometrics 45 / 64 Data preparation: Example from the current population survey The Current Population Survey (CPS) is a monthly survey of about 50,000 US households conducted by the Bureau of the Census for the Bureau of Labor Statistics The survey has been conducted for more than 50 years It is the primary source of information on the labor force's characteristics of the U.S. population It includes information on socio-demographic characteristics and (un)employment details Supplemental questions on a variety of topics are added often to the regular questionnaire S. Martin: Introductory Econometrics 46 / 64 Wir CPSstarten mit CPS-Rohdaten raw data Id Sex Age Race Married Highest grade Wage Working hours No. of own kids 1 male 70 white yes high school 6811 15 0 2 female 73 white yes high school 0 0 0 3 male 52 white yes high school 26000 40 0 4 female 61 white yes high school 0 0 0 5 male 47 white yes high school 20000 0 1 6 female 35 white yes high school 19000 40 1 7 female 14 white no none 0 0 1 8 male 46 white yes high school 0 60 0 9 female 38 white yes college 0 25 0 10 male 48 white yes college 35000 40 0 11 female 43 white yes college 13000 0 0 12 male 19 white no high school 2500 0 0 13 male 66 white yes college 6700 20 0 14 female 63 white yes high school 1400 0 0 15 male 69 white yes college 0 0 0 16 female 75 white yes college 0 0 0 17 male 41 white yes college 50000 50 3 18 female 30 white yes college 0 0 3 19 male 4 white no not finished 0 0 3 20 female 1 white no not finished 0 0 3 21 female 1 white no not finished 0 0 3 Martin Halla (JKU) KS Empirische Wirtschaftsforschung - 2 54 / 58 S. Martin: Introductory Econometrics 47 / 64 1st step: variable codication / 2nd step: variable names chritt: Variablenkodierung 2. Schritt: Variablennamen Information Variablen-Typ Information Variable-Name Personal id nominal Personal id id Sex nominal/binär Sex female Age kardinal Age age Race nominal/binär Race white Married nominal/binär Married married Highest grade attended ordinal Highest grade attended educ Wage kardinal Wage wage Working hours kardinal Working hours hours No. of own kids kardinal No. of own kids kids Kurz, sprechend, gebräuchlich! Martin Halla (JKU) KS Empirische Wirtschaftsforschung - 2 55 / 58 Martin Halla (JKU) KS Empirische Wirtschaftsforschung - 2 S. Martin: Introductory Econometrics 48 / 64 Bearbeiteter Prepared dataDatensatz set id female age white married educ wage hours kids 1 0 70 1 1 1 6811 15 0 2 1 73 1 1 1 0 0 0 3 0 52 1 1 1 26000 40 0 4 1 61 1 1 1 0 0 0 5 0 47 1 1 1 20000 0 1 6 1 35 1 1 1 19000 40 1 7 1 14 1 0 0 0 0 1 8 0 46 1 1 1 0 60 0 9 1 38 1 1 2 0 25 0 10 0 48 1 1 2 35000 40 0 11 1 43 1 1 2 13000 0 0 12 0 19 1 0 1 2500 0 0 13 0 66 1 1 2 6700 20 0 14 1 63 1 1 1 1400 0 0 15 0 69 1 1 2 0 0 0 16 1 75 1 1 2 0 0 0 17 0 41 1 1 2 50000 50 3 18 1 30 1 1 2 0 0 3 19 0 4 1 0 0 0 0 3 20 1 1 1 0 0 0 0 3 21 1 1 1 0 0 0 0 3 Martin Halla (JKU) KS Empirische Wirtschaftsforschung - 2 57 / 58 S. Martin: Introductory Econometrics 49 / 64 3. step: 3rd descriptive Schritt: statistics Deskriptive Statistik Variable Obs. Mean S.D. Min Max female 70,721 0.47 0.50 0 1 age 70,721 38.60 12.89 15 90 white 70,721 0.88 0.33 0 1 married 70,721 0.61 0.49 0 1 educ 70,721 1.95 0.23 0 2 wage 70,721 20,814 18,836 0 199,998 hours 70,721 37.53 15.56 0 99 kids 70,721 0.81 1.10 0 9 Two or three decimal points are normally sucient! Punkt vs. Komma! Zwei oder drei Kommastellen snid üblicherweise ausreichend! S. Martin: Introductory Econometrics 50 / 64 Appendix: Inference for the 1st moment of the population distribution The counterpart of the expected value of the population is the sample mean: N 1 X X̄ = Xi. N i=1 X̄ is an unbiased estimator for E (X ): N ! 1 X E (X̄ ) = E Xi N i=1 1 = E (X1 + X2 +... + XN ) N 1 = [E (X1 ) + E (X2 ) +... + E (XN )] N 1 = N · µX = µ X N S. Martin: Introductory Econometrics 51 / 64 Appendix: Variance of the sample mean The variance of the sample mean is N ! N ! 1 X 1 X Var (X̄ ) = Var Xi = Var (Xi ) N i=1 N2 i=1 1 2 1 2 = NσX = σX N2 N The variance decreases with an increasing number of observations N and converges to zero with N → ∞, i.e. it holds that plimX̄ = E (X ) ⇒ X̄ is a consistent estimator for the expected value of a random variable X. S. Martin: Introductory Econometrics 52 / 64 Appendix: Inference for the 2nd moment of the population distribution For the variance σX2 of a random variable an unbiased estimator σ̂X2 is: N 1 σ̂X2 = (Xi − X̄ )2. X N −1 i=1 The sum of squared dierences between the random variable X and its expected value X̄ is divided by a normalizing factor the number of degrees of freedom of the estimation The degrees of freedom correspond to the number of observations minus the number of estimated parameters used during the estimation In this case, one estimated parameter is used: µX was rst estimated by the sample mean and then included in the estimation of σX2 S. Martin: Introductory Econometrics 53 / 64 Condence interval Estimators are continuous random variables, which are calculated based on a random sample That values should approximate the true parameter of the population (point estimator) Theynever (only by coincidence) exactly match the true value Condence interval: An interval based on the sample constructed such that it includes the true value with a given probability The higher the probability level (condence level), the broader is the interval At this point one needs to know more (or make assumptions) about the probability distribution S. Martin: Introductory Econometrics 54 / 64 Appendix: Critical value - I Assumption: The random variable X is normally distributed, with unknown expected value µx and known variance σX2 −1 PN It can be shown that X̄ = N i=1 Xi is normally distributed as well with expected value = µx and variance = σX2 /N The realization x̄ does not have to be exactly µx To construct the condence interval, we have to "standardize" the normally distributed sample mean Reason: The standard normal distribution is entirely tabulated: The critical values that specify the boundaries for parts of the probability mass underneath the density function of the standard normal distribution can be taken from that table S. Martin: Introductory Econometrics 55 / 64 Critical value - II The standardized sample mean Z̄ is: X̄ − µx Z̄ = √ σx / N The random variable Z̄ 's expected value is 0 and variance is 1 One can obtain dierent condence intervals for Z̄ for dierent condence levels (e.g. 99%) The condence level is 1-α, where α is the corresponding probability of making a wrong decision - level of signicance (e.g. 5%). Because of the symmetry of the standard normal distribution, the critical value for the left boundary (−kα/2 ) is exactly the same as the critical value for the right boundary (+kα/2 ) Formally: P(−kα/2 ≤ Z̄ ≤ kα/2 ) = 1 − α S. Martin: Introductory Econometrics 56 / 64 Konfidenzintervall Appendix: Condence interval - III 95% Wahrscheinlich− keitsmasse 2,5% Wahrscheinlich− 2,5% Wahrscheinlich− keitsmasse keitsmasse X −k 0 k Abbildung: Verteilung der Wahrscheinlichkeitsmasse unter einer Figure: Distribution of the probability mass under standard normal distribution. Critical values:−k and k. They cut o Kritische Standardnormalverteilung. 2.5% of the Werte: −k und probability mass k.toSie right, and to the grenzen left insgesamt 5% der Wahrscheinlichkeitsmasse nach links bzw. rechts ab. Martin Halla (JKU) KS Empirische Wirtschaftsforschung - 2 24 / 58 S. Martin: Introductory Econometrics 57 / 64 Appendix: Condence interval- IV The probability that X̄ lies between certain boundaries yields from the critical √ values. It is determined by inserting Z̄ = (X̄ − µX )/(σX / N) into σX σX P µX − kα/2 · √ ≤ X̄ ≤ µX + kα/2 · √ =1−α N N Given that the expected value µX is generally unknown, more interesting is the condence interval for µX : σX σX P X̄ − kα/2 · √ ≤ µX ≤ X̄ + kα/2 · √ =1−α N N S. Martin: Introductory Econometrics 58 / 64 Appendix: Condence interval- V Generally, the variance of X is normally unknown and also needs to be estimated We can then use the estimated variance σ̂X It can be shown that the standardized sample mean is now t -distributed with N −1 degrees of freedom. The condence interval is therefore: σ̂X σ̂X P X̄ − tα/2 · √ ≤ µX ≤ X̄ + tα/2 · √ =1−α N N where tα/2 is the critical value from the t -distribution. Rule of thumb: for a 95% condence level: σ̂X [X̄ ± 1.96 √ N ] S. Martin: Introductory Econometrics 59 / 64 Appendix: Hypotheses testing & condence intervals Now we know how to construct a condence interval Sometimes it is useful to be able to answer "a question" by yes or no: to test Hypothesis There should always be a Null hypothesis (e.g. H0 : µX = 0) and an alternative Hypothesis (e.g. H1 : µX ̸= 0) Two types of errors can occur: ▶ Type I error: reject H0 , even though it is true ▶ Type II error: don't reject H0 , even though it is wrong We can dene the error probabilities α is the tolerance level for the type I error In practice, we will examine whether the value of the Null hypothesis lies in between the condence interval With a small α, the constructed interval is wide. Hence, the type I error probability decreases and the discriminatory power shrinks S. Martin: Introductory Econometrics 60 / 64 Appendix: The t -test X̄ −µX The random variable t with t= √ σ̂/ N is t- distributed wit N −1 degrees of freedom The test statistic for the Null hypothesis H 0 : µX = 0 and the alternative Hypothesis H1 : µX ̸= 0 is: X̄ − 0 X̄ t= √ = √ ∼ tN−1 σ̂/ N σ̂/ N If the absolute value |t|, calculated from the sample with a given level of signicance, is bigger than the critical value from the t -distribution with N −1 degrees of freedom, the Null hypothesis has to be rejected With the t -test, any Null hypothesis for a single parameter can be tested S. Martin: Introductory Econometrics 61 / 64 Der The t-Test t -test 95% Wahrscheinlich− keitsmasse 2,5% Wahrscheinlich− 2,5% Wahrscheinlich− keitsmasse keitsmasse X −t(0,025)=−1,96 0 t(0,025)=1,96 Figure: Region of rejection for a two sided t -test with a 5% level of signicance Abbildung: Ablehnungsbereich eines zweisseitigen t-Tests bei einer Irrtumswahrscheinlichkeit von 5% Martin Halla (JKU) KS Empirische Wirtschaftsforschung - 2 30 / 58 S. Martin: Introductory Econometrics 62 / 64 Appendix: t -test for the BMI Example (BMI) The estimated expected value of the BMI (25.34) leads to the question of weather it is signicantly dierent from 25, which is the lower threshold for over-weighted persons The Null hypothesis in this case is H0 : E [BMI ] = 25. The t -value for this test is 25.34 − 25 t= √ = 29.63 4.29/ 139, 733 |t| = 29.63 is larger than the critical value from the t -distribution with a signicance level of 5% and 139,732 degrees of freedom. We can reject the null hypothesis in favor for the alternative hypothesis H1 : E [BMI ] ̸= 25 S. Martin: Introductory Econometrics 63 / 64 Appendix: One sided t -test One sided t -tests - e.g. H 0 : µX = x and H 1 : µX > x or H 1 : µX < x are very reasonable, if -out of economic considerations- a down- or upwards deviation from the null hypothesis is very meaningful. For a constant signicance level α, the critical value is shifted correspondingly S. Martin: Introductory Econometrics 64 / 64