Unit 2: Single Regression Model PDF
Document Details
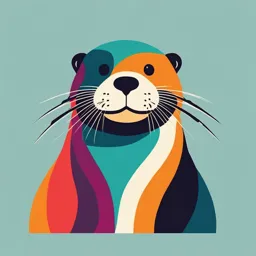
Uploaded by AwesomeCarnelian4810
Copenhagen Business School, Vienna University of Economics and Business
Martin / Zulehner
Tags
Summary
This document details a single regression model, focusing on introductory econometrics. It covers simple linear regression, OLS estimation, variance, goodness of fit, and related exercises. The document also highlights different examples, theoretical background, and practical applications related to the material.
Full Transcript
Unit 2: Single regression model Martin / Zulehner: Introductory Econometrics 1 / 60 Outline 1 Simple linear regression model 2 OLS estimator Estimation Properties of OLS 3 Variance of the OLS estimator Assumption Variance estimation 4 Goodness of f...
Unit 2: Single regression model Martin / Zulehner: Introductory Econometrics 1 / 60 Outline 1 Simple linear regression model 2 OLS estimator Estimation Properties of OLS 3 Variance of the OLS estimator Assumption Variance estimation 4 Goodness of fit 5 Exercises Exercise 1: Properties of the summation operator Exercise 2: derive the OLS estimator Exercise 3: Properties of the OLS estimator and its variance Martin / Zulehner: Introductory Econometrics 2 / 60 The simple linear regression model (SLR) A linear model representing the relationship between two variables x and y : y = β0 + β1 · x + u (1) β0... intercept (parameter) β1... slope parameter u... error term (capturing all unobserved factors) We are interested in the “population” parameters, examples: life expectancy and health expenditures: life expectancy = β0 + β1 · health expenditures + u test score and student-teacher ratio: test score = β0 + β1 · STR + u wages and education: wages = β0 + β1 · education + u Martin / Zulehner: Introductory Econometrics 3 / 60 Terminology of the SLR unsystematic part z}|{ y= β0 + β1 · x +u (2) | {z } systematic part y x u dependent variable independent variable error term explained variable explanatory variable disturbance response variable control variable predicted variable predictor variable regressand regressor lhs variable rhs variable Martin / Zulehner: Introductory Econometrics 4 / 60 Least Squares Assumptions for Causal Inference Let β1 be the causal effect on y of a change in x : yi = β0 + β1 · xi + ui , i = 1...N (3) where N is the sample size We assume that 1 model is linear in parameters: y = β0 + β1 · x + u 2 (xi , yi ) : i = 1...N are independently and identically distributed (i.i.d.) 3 the sample variation in the independent variable is not 0, ie, Var(x) 6= 0 4 the conditional distribution of u given x has mean zero, that is, E (u | x) = 0 the average value of u, the error term, in the population is 0 large outliers in x or y are rare Martin / Zulehner: Introductory Econometrics 5 / 60 The SLR as a strategy for identification The counterfactual question, which is based on the economic model: ’How large would yi have been, had xi (ceteris paribus) realized a different value (e.g. double or half as high)?’ Since for every observation unit i there is only one observation pair (yi , xi ), the implicit situation coming from the counterfactual question is not observable Martin / Zulehner: Introductory Econometrics 6 / 60 Causal effect in SLR we are interested in the effect of x on the distribution of y , other relevant things held constant the causal effect on y of a unit change in x is the expected difference in y as measured in an ideal randomized controlled experiment, where I i) all subjects follow the treatment plan, ii) random assignment to treatment, iii) having a control group allows measuring the treatment effect, and iv) subjects have no choice, no reverse causality, no selection into treatment most often (but not always) we are interested in effect on mean of y, i.e.: ∂E (y |x, u) (4) ∂x problem: how do we get a “reliable” estimate of this partial derivative? reliable means: reflects the effect on y of a change in x rather than something else Martin / Zulehner: Introductory Econometrics 7 / 60 Identification assumptions In the SLR, we make the following identification assumptions: There exists a linear relationship in the population between the explanatory variable x and the dependent variable Y , where X influences y and not the other way round This relationship also holds for observation pairs which are not observed All other observation pairs serve as control group for one particular observation pair Martin / Zulehner: Introductory Econometrics 8 / 60 Random sample (xi , yi ) , i = 1,... , n are i.i.d this arises automatically if the entity (individual, district) is sampled by simple random sampling: I the entities are selected from the same population, so (xi , yi ) are identically distributed for all i = 1,... , n I the entities are selected at random, so the values of (x, y ) for different entities are independently distributed the main place we will encounter non-i.i.d. sampling is when data are recorded over time for the same entity (panel data and time series data) - we will deal with that complication when we cover panel data Martin / Zulehner: Introductory Econometrics 9 / 60 Variance of x the sample variation in the independent variable is not 0, ie, Var(x) 6= 0 if Var(x) = 0, then there is no variation in the independent variable and we would not be able to identify β1 from β0 Martin / Zulehner: Introductory Econometrics 10 / 60 Zero conditional mean assumption We need to make a crucial assumption about how u and x are related We want that they are completely unrelated, i.e if we know something about x this does not give us any information about u, so that: E (u|x) = E (u) = 0 This is a similar but somehow stronger assumption than E (xu) = 0 and it is often called the “orthogonality condition” Under these assumptions we have specified the conditional expectation of y or population regression function: E (y |x) = E (β0 + β1 · x + u|x) = β0 + β1 x Martin / Zulehner: Introductory Econometrics 11 / 60 Zero mean assumption The average value of u, the error term, in the population is 0. That is, E (u) = 0 This is not a restrictive assumption, since we can always use β0 to normalize E (u) to be 0 Martin / Zulehner: Introductory Econometrics 12 / 60 Outliers Large outliers in x or y are rare – a large outlier is an extreme value of x or y technically, if x and y are bounded, then x and y have finite fourth moments (E x 4 < ∞ and E y 4 < ∞) outliers can result in meaningless values of β̂1 → Look at your data! If you have a large outlier, is it a typo? Does it belong in your data set? Why is it an outlier? Martin / Zulehner: Introductory Econometrics 13 / 60 Population regression line in the SLR Figure: E (y |x) as a linear function of x, where for any x the distribution of y is centered on E(y |x) Martin / Zulehner: Introductory Econometrics 14 / 60 An example - I We are interested in the linear relationship between health expenditures and life expectancy: life expectancy = β0 + β1 · health expenditures + u (5) The dependent variable is the life expectancy at birth The independent variable are health expenditures How should we interpret β0 and β1 ? Do you think β1 ≶ 0? Martin / Zulehner: Introductory Econometrics 15 / 60 An example - II How do we estimate β0 and β1 ? Let’s assume that we have a random sample from the population of N units with (xi , yi ) being an observation where i = 1,... , N life expectancyi = β0 + β1 · health expendituresi + ui (6) There are different methods to obtain estimates β0 and β1 The Ordinary Least Squares (OLS) estimator suggests to pick β0 and β1 such that they minimize the sum of the squared residuals Martin / Zulehner: Introductory Econometrics 16 / 60 Life expectancy and public health expenditures To study this relationship we use the following data: Unit of interest: OECD member states Data type: Cross-sectional data for the year 2000 Source: OECD Factbook 2007 Descriptive statistics: Variable Obs. Mean S.D. Min Max Life expectancy 30 77.34 2.54 70.5 81.2 Public health expenditure 30 1,410.8 660.69 235 2,663 per capita in PPP Martin / Zulehner: Introductory Econometrics 17 / 60 Scatterplot of life expectancy and public health expenditures How shall we put a linear function in this graph? Martin / Zulehner: Introductory Econometrics 18 / 60 Deriving the ordinary least squares (OLS) estimator - I Define a fitted value for y when x = xi as: yˆi = β̂0 + β̂1 xi (7) Define a residual for the observation i as the difference between the actual y1 and its fitted value ûi = yi − ŷi = yi − β̂0 − β̂1 xi (8) Now chose βˆ0 and βˆ1 such that the sum of squared residuals: N X N X ûi 2 = (yi − β̂0 − β̂1 xi )2 (9) i=1 i=1 is as small as possible, i.e.: N X min (yi − β̂0 − β̂1 xi )2 (10) β̂0 ,β̂1 i=1 Martin / Zulehner: Introductory Econometrics 19 / 60 Graphical illustration of the OLS estimator Martin / Zulehner: Introductory Econometrics 20 / 60 Example 2: wage function we are interested in the linear relationship b/w wages and education I wages = β0 + β1 · education + u I how should we interpret β0 and β1 ? I do you think β1 ≶ 0? how do we estimate β0 and β1 ? let’s assume that we have a random sample from the population of N units with (xi , yi ) being an observation where i = 1,... , n I wagesi = β0 + β1 educationi + ui the Ordinary Least Squares (OLS) estimator suggests to pick β0 and β1 such that they minimize the sum of the squared residuals Martin / Zulehner: Introductory Econometrics 21 / 60 Deriving the OLS estimator - II To solve this minimization problem, we look at the first order conditions (FOC), i.e. the partial derivatives of (8) with respect to β̂0 and β̂1 must be zero PN N ∂ i=1 ûi2 X = −2 (yi − β̂0 − β̂1 xi ) = 0 (11) ∂ β̂0 i=1 PN N ∂ i=1 ûi2 X = −2 (yi − β̂0 − β̂1 xi )xi = 0 (12) ∂ β̂1 i=1 Note that (11) can be written as ȳ = β̂0 + β̂1 x̄ (13) PN PN where ȳ = N −1 i=1 yi and x̄ = N −1 i=1 xi Martin / Zulehner: Introductory Econometrics 22 / 60 Deriving the OLS estimator - III From (13) we can see that once we have β̂1 , β̂0 can easily be obtained: β̂0 = ȳ − β̂1 x̄ (14) Plugging (14) into (12) yields: N X xi yi − ȳ − β̂1 x̄ − β̂1 xi = 0, (15) i=1 which upon rearrangements, gives: N X N X xi (yi − ȳ ) = β̂1 xi (xi − x̄) (16) i=1 i=1 Martin / Zulehner: Introductory Econometrics 23 / 60 Deriving the OLS estimator - IV Therefore, β̂1 can be written as: PN xi (yi − ȳ ) β̂1 = Pi=1 N (17) i=1 xi (xi − x̄) Properties of the summation operator (xi − x̄)2 and PN PN i=1 xi (xi − x̄) = i=1 PN PN i=1 xi (yi − ȳ ) = i=1 (xi − x̄) (yi − ȳ ) Hence, we can rewrite (17) as follows: PN i=1 (xi − x̄) (yi − ȳ ) β̂1 = PN 2 (18) i=1 (xi − x̄) Martin / Zulehner: Introductory Econometrics 24 / 60 Deriving the OLS estimator - V Equation (17) is simply the sample covariance between x and y divided by the sample variance of x: Cov (x, y ) β̂1 = (19) Var (x) Thus, if the correlation between x and y is positive, β̂1 will be positive, too The intercept βˆ0 can be easily obtained as: β̂0 = ȳ − β̂1 x̄ (20) Martin / Zulehner: Introductory Econometrics 25 / 60 Deriving the OLS estimator - VI Example (Reverse Causality) Simple linear regression model : Y = β0 + β1 X + u Reverse causal relation: X = γ0 + γ1 Y + η. The resulting estimators for both directions are: σ̂XY σ̂XY β̂1 = 2 and γ̂1 = 2 σ̂X σ̂Y The difference lies in the normalization of the estimated covariance The correlation coefficient σ̂XY σ̂X σ̂Y is independent from the assumptions about the causal direction Martin / Zulehner: Introductory Econometrics 26 / 60 Regression functions The population regression function (PRF) E (y |x) = β0 + β1 x, (21) is fixed but unknown The sample regression function, ŷ = β̂0 + β̂1 x, (22) is obtained for a given data sample Note: a new sample will generate different estimates of β0 and β1 Martin / Zulehner: Introductory Econometrics 27 / 60 Summary of the OLS estimator The slope estimate (β̂1 ) is the sample covariance between x and y divided by the sample variance of x If x and y are positively correlated, the slope will be positive If x and y are negatively correlated, the slope will be negative Intuitively, OLS is fitting a line through the sample points such that the sum of squared residuals is as small as possible, hence the term least squares The residual, û, is an estimate of the error term, u, and is the difference between the fitted line (sample regression function) and the sample points Martin / Zulehner: Introductory Econometrics 28 / 60 Back to our data Life Public health No. Country expectancy expenditure 1 AUS 79.3 1653 2 AUT 78.1 1863 3 BEL 78.3 1726 4 CAN 79.3 1760 5 CZE 75 887 6 DNK 76.9 1962 7 FIN 77.6 1289 8 FRA 79 1858 9 DEU 78 2097 10 GRC 78.1 849 11 HUN 71.7 606 12 ISL 80.1 2166 13 IRL 76.5 1326 14 ITA 79.6 1499 15 JPN 81.2 1599 16 KOR 76.4 359 17 LUX 78 2663 18 MEX 74.1 235 19 NLD 78 1424 20 NZL 78.7 1252 21 NOR 78.7 2541 22 POL 73.8 413 23 PRT 76.6 1178 24 SVK 73.3 532 25 ESP 79.2 1088 26 SWE 79.7 1928 27 CHE 79.8 1768 28 TUR 70.5 284 29 GBR 77.8 1502 30 USA 76.8 2017 Martin / Zulehner: Introductory Econometrics 29 / 60 OLS estimates by Stata Martin / Zulehner: Introductory Econometrics 30 / 60 OLS estimates by R Life Expectancy Output ## lm(formula = lifeexpectancy ~ publicexpenditure, data = lifeexpectancynew) ## ## Residuals: ## Min 1Q Median 3Q Max ## -3.7857 -1.1256 0.1631 1.2351 3.3537 ## ## Coefficients: ## Estimate Std. Error t value Pr(>|t|) ## (Intercept) 7.352e+01 7.987e-01 92.040 < 2e-16 *** ## publicexpenditure 2.708e-03 5.143e-04 5.265 1.34e-05 *** ## --- ## Signif. codes: 0 '***' 0.001 '**' 0.01 '*' 0.05 '.' 0.1 ' ' 1 ## ## Residual standard error: 1.83 on 28 degrees of freedom ## Multiple R-squared: 0.4975, Adjusted R-squared: 0.4796 ## F-statistic: 27.72 on 1 and 28 DF, p-value: 1.344e-05 Martin / Zulehner: Introductory Econometrics 31 / 60 Scatterplot with regression line, year 2000 Source: OECD Factbook 2007 Martin / Zulehner: Introductory Econometrics 32 / 60 Back to our data Life Public health Fitted No. Country Residual expectancy expenditure value 1 AUS 79.3 1653 77.992 1.308 2 AUT 78.1 1863 78.561 -0.461 3 BEL 78.3 1726 78.190 0.110 4 CAN 79.3 1760 78.282 1.018 5 CZE 75 887 75.918 -0.918 6 DNK 76.9 1962 78.829 -1.929 7 FIN 77.6 1289 77.007 0.593 8 FRA 79 1858 78.548 0.452 9 DEU 78 2097 79.195 -1.195 10 GRC 78.1 849 75.815 2.285 11 HUN 71.7 606 75.158 -3.458 12 ISL 80.1 2166 79.382 0.718 13 IRL 76.5 1326 77.107 -0.607 14 ITA 79.6 1499 77.575 2.025 15 JPN 81.2 1599 77.846 3.354 16 KOR 76.4 359 74.489 1.911 17 LUX 78 2663 80.727 -2.727 18 MEX 74.1 235 74.153 -0.053 19 NLD 78 1424 77.372 0.628 20 NZL 78.7 1252 76.907 1.793 21 NOR 78.7 2541 80.397 -1.697 22 POL 73.8 413 74.635 -0.835 23 PRT 76.6 1178 76.706 -0.106 24 SVK 73.3 532 74.957 -1.657 25 ESP 79.2 1088 76.463 2.737 26 SWE 79.7 1928 78.737 0.963 27 CHE 79.8 1768 78.304 1.496 28 TUR 70.5 284 74.286 -3.786 29 GBR 77.8 1502 77.584 0.216 30 USA 76.8 2017 78.978 -2.178 Martin / Zulehner: Introductory Econometrics 33 / 60 Assumptions We will now study the statistical properties of the OLS estimator Therefore, we make four assumptions 1 Assumption SLR.1 - Linear in parameters y = β0 + β1 x + u 2 Assumption SLR.2 - Random sampling yi = β0 + β1 xi + ui where 1 = 1, 2,... , n. 3 Assumption SLR.3 - Sample variation in the independent variable Var (x) 6= 0 4 Assumption SLR.4 - Zero conditional mean E (u|x) = 0 Technical assumption: finite fourth moments (E x 4 < ∞ and E y 4 < ∞ Martin / Zulehner: Introductory Econometrics 34 / 60 Theorem 1 - Unbiasedness of OLS Under assumptions SLR.1 through SLR.4, E (β̂0 ) = β0 , and E (β̂1 ) = β1 , (23) for any values of β0 and β1. In other words, the OLS estimators β̂0 and β̂1 are unbiased estimates for the population parameters β0 and β1 If any of the assumptions SLR.1 to SLR.4 fails, β̂0 and β̂1 are biased SLR.4 is likely to fail (why?) Martin / Zulehner: Introductory Econometrics 35 / 60 Unbiasedness of OLS - I In order to prove unbiasedness, we need to rewrite our estimator in terms of the population parameter Start with a simple rewrite of the formula as: PN i=1 (xi − x̄)yi β̂1 = Sx2 where Sx2 = Ni=1 (xi − x̄)2 P Martin / Zulehner: Introductory Econometrics 36 / 60 Unbiasedness of OLS - II N X N X (xi − x̄)yi = (xi − x̄)(β0 + β1 xi + ui ) i=1 i=1 N X N X N X = (xi − x̄)β0 + (xi − x̄)β1 xi + (xi − x̄)ui i=1 i=1 i=1 N X N X N X = β0 (xi − x̄) + β1 (xi − x̄)xi + (xi − x̄)ui i=1 i=1 i=1 Properties of the summation operator PN i=1 (xi − x̄) = 0 PN PN i=1 (xi − x̄)xi = i=1 (xi − x̄)2 = Sx2 Martin / Zulehner: Introductory Econometrics 37 / 60 Unbiasedness of OLS - III Thus, the numerator of β̂1 can be written as: PN β1 Sx2 + i=1 (xi − x̄)ui β̂1 = Sx2 PN i=1 (xi − x̄)ui = β1 + Sx2 Then, taking expectations conditional on x N X E (β̂1 |x) = β1 + 1/Sx2 (xi − x̄)E (ui |x) = β1 i=1 A similar proof can be done for βˆ0 Martin / Zulehner: Introductory Econometrics 38 / 60 Unbiasedness Summary The OLS estimates of β0 and β1 are unbiased Proof of unbiasedness depends on our 4 assumptions - if any assumption fails, then OLS is not necessarily unbiased Remember unbiasedness is a description of the estimator - in a given sample we may be “near” or “far” from the true parameter Martin / Zulehner: Introductory Econometrics 39 / 60 The variance of the OLS estimator In addition to knowing that the sampling distribution of β̂1 is centered around β1 (e.g. β̂1 is unbiased), it is important to know how far we can expect β̂1 to be away from β1 on average We are interested in the variance or the standard deviation of the OLS estimator This helps to think about the efficiency of estimators If we assume that the unobservable term u has a constant variance (homoskedasticity) then it is easier to describe the variance of the OLS estimator The opposite of homoskedasticity is heteroskedasticity Martin / Zulehner: Introductory Econometrics 40 / 60 Homoskedastic case Martin / Zulehner: Introductory Econometrics 41 / 60 Heteroskedastic case - I Martin / Zulehner: Introductory Econometrics 42 / 60 Heteroskedastic case - II Martin / Zulehner: Introductory Econometrics 43 / 60 Assumption SLR.5 - Homoskedasticity Given any value of the explanatory variable, the error u has always the same variance. In other words Var (u|x) = σ 2 (24) This assumption plays no role for the unbiasedness of the OLS estimator If Var (u|x) depends on x, the error term is said to exhibit heteroskedasticity Martin / Zulehner: Introductory Econometrics 44 / 60 Theorem 2 - Sampling variances of the OLS estimators Under assumptions SLR.1 through SLR.5, ! σ2 Var (β̂1 ) = PN (25) 2 i=1 (xi − x̄) and ! σ 2 n−1 N 2 P i=1 xi Var (β̂0 ) = PN (26) 2 i=1 (xi − x̄) where these are conditional on the sample values {x1 ,... , xN }. These formulas are invalid in the case of heteroskedasticity. Note that σ 2 is unobserved Martin / Zulehner: Introductory Econometrics 45 / 60 The larger the error variance, σ 2 , the larger the variance of the slope estimate The larger the variability in the xi , the smaller the variance of the slope estimate As a result, a larger sample size should decrease the variance of the slope estimate Problem: the error variance is unknown But we observe the residuals, ûi We can use the residuals to get an estimate of the error variance Martin / Zulehner: Introductory Econometrics 46 / 60 The estimated residuals are: ûi = yi − β̂0 − β̂1 xi = (β0 + β1 xi + ui ) − β̂0 − β̂1 xi = ui − (β̂0 − β0 ) − (β̂1 − β1 )xi PN An unbiased estimator of σ 2 = E (u 2 ) is N −1 i=1 ui2 However we do not observe u but only an OLS estimate û. Hence an unbiased estimator for the variance is: n 1 X SSR σ̂ 2 = ûi2 = N − K − 1 i=1 (N − K − 1) The term (N − K − 1) is the degrees of freedom (df ) adjustment for the general OLS problem with N observations and K independent variables. In the SLR case it equals 2 Martin / Zulehner: Introductory Econometrics 47 / 60 √ σ̂ = σ̂ 2 is the standard error of the regression q Recall that the standard deviation sd(β̂1 ) = Var (β̂1 ) = √σ SSTx If we substitute σ̂ for σ then we have the standard error of β̂1 : σ̂ se(β̂1 ) = 1/2 PN i=1 (xi − x̄)2 t-statistic: H0 : βj = 0 and H1 : βj 6= 0 I if the population error u is independent of the explanatory variable x1 and normally distributed (β̂i )−βj with zero mean and variance σ 2 (additional assumption), then tβ̂ = se(β̂i ) ∼ tN−K −1 i I for now rule of thumb: if the t-statistic is larger than 2, we reject the null hypothesis that βj = 0, and we typically say “βj is statistically significant different from zero” Martin / Zulehner: Introductory Econometrics 48 / 60 (Explained) variation in the dependent variable We can think of each observation as being made up of an explained part and an unexplained pert.Let’s define some quantities: Total sum of squares (SST): N 2 P i=1 (yi − ȳ ) I Total sample variation in yi I "Total variation" PN Explained sum of squares(SSE): i=1 (ŷi − ȳ )2 I Sample variation in yˆi I "Explained variation" PN Residual sum of squares(SSR): i=1 ûi2 I Sample variation in ûi I "Unexplained variation" It follows that: SST = SSE + SSR (27) Martin / Zulehner: Introductory Econometrics 49 / 60 Goodness of fit -I How well does the model explain the variation of y? From (25) it follows that SSE SSR + =1 (28) SST SST The fraction of the total sum of squares (SST) that is explained by the model, is called the R-squared of regression. The R-squared is defined as follows SSE SSR R2 = =1−. (29) SST SST Martin / Zulehner: Introductory Econometrics 50 / 60 Goodness of fit -II We can also think of R 2 as being equal to the squared correlation coefficient between the actual yi and the values ŷi P 2 N − ȳ )(ŷi − ŷ¯ ) i=1 (yi R 2 = P P (30) N 2 N ¯ 2 i=1 (yi − ȳ ) i=1 (ŷi − ŷ ) The R-squared is a number between zero and one Proportion of sample variation that is explained by the model It never decreases when another independent variable is added Because R 2 will usually increase with the number of independent variables, it is not a good way to compare models Martin / Zulehner: Introductory Econometrics 51 / 60 Example 2: wage function with CPS 2015 in Stata. reg ahe bachelor Source SS df MS Number of obs = 7,098 F(1, 7096) = 1199.88 Model 150896.659 1 150896.659 Prob > F = 0.0000 Residual 892387.992 7,096 125.7593 R-squared = 0.1446 Adj R-squared = 0.1445 Total 1043284.65 7,097 147.003614 Root MSE = 11.214 ahe Coef. Std. Err. t P>|t| [95% Conf. Interval] bachelor 9.233924.2665732 34.64 0.000 8.711361 9.756487 _cons 16.38111.1933203 84.74 0.000 16.00214 16.76007 R2 = SSE SST = 150896.659 1043284.65 = 0.1446 R2 = 1 − SSR SST 892387.992 = 1 − 1043284.65 = 0.1446 Martin / Zulehner: Introductory Econometrics 52 / 60 Example 2: wage function with CPS 2015 in R Output bachelor ## Residuals: ## Min 1Q Median 3Q Max ## -23.574 -6.766 -1.958 4.292 80.154 ## ## Coefficients: ## Estimate Std. Error t value Pr(>|t|) ## (Intercept) 16.3811 0.1933 84.74