Unit 4: Functional Forms PDF
Document Details
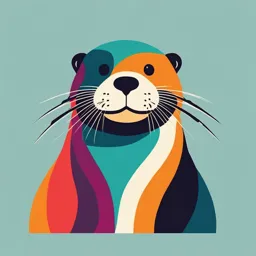
Uploaded by AwesomeCarnelian4810
Copenhagen Business School, Vienna University of Economics and Business
Martin / Zulehner
Tags
Related
- Introductory Econometrics for Finance PDF
- EEP/IAS 118 - Introductory Applied Econometrics, Section 2 PDF
- FBA1018 - Introductory Econometrics Autumn 2024 PDF
- Unit 2: Single Regression Model PDF
- Panel Data - Introductory Econometrics for Finance (PDF)
- 5EN306 - Aplikovane kvantitativnč metody Shrněč PDF
Summary
This document provides an outline of unit 4 on functional forms in introductory econometrics. It covers various topics like marginal effects, log specifications, dummy variables, and nonlinear regression functions, along with examples. Some of the concepts are related to testing scores compared to the student to teacher ratio and income.
Full Transcript
Unit 4: Functional forms Martin / Zulehner: Introductory Econometrics 1 / 24 Outline 1 Marginal eects How to calculate marginal eects Other specications 2 Log specications 3 Dummy variables 4 Results from wage regressions 5 Nonlinear regression func...
Unit 4: Functional forms Martin / Zulehner: Introductory Econometrics 1 / 24 Outline 1 Marginal eects How to calculate marginal eects Other specications 2 Log specications 3 Dummy variables 4 Results from wage regressions 5 Nonlinear regression functions Martin / Zulehner: Introductory Econometrics 2 / 24 Marginal eects Pk Coecient βj in yi = x ′i β + ui = j=1 βj xij + ui corresponds to partial derivative of conditional mean function ∂E [yi |x i ] βj = ∂xij ⇒ marginal eect (on the conditional mean function) if E [ui |x i ] = 0, this reects a ceteris paribus eect measure the impact that an instantaneous change in one variable has on the outcome variable while all other variables are held constant in an OLS model with linear eects, estimated coecients are always equal to marginal eects (eg, no interaction terms) ▶ example: yi = β0 + β1 × experience + β2 × experience2 + ui ▶ maginal eect: β1 + 2 × β2 × experience Martin / Zulehner: Introductory Econometrics 3 / 24 Marginal eects βj does generally not correspond to a marginal eect on yi ▶ in fact, ∂E [yi |xi ]/∂xij = ∂yi /∂xij only if ∂ui /∂xij = 0 ▶ we can deduce a causal relationship only when the assumption of exogeneity holds if exogeneity fails, there is endogeneity (or an issue of identication) → OLS gives biased estimate of the causal eect ▶ with endogeneity ∂ui /∂xij ̸= 0 ▶ omitted variable bias, endogeneity, simultaneity, measrurement error, selection causal eect is a complex concept a causal eect can be dened to be the eect measured in an ideal randomized controlled experiment ▶ ideal: all subjects follow the treatment plan ▶ randomisation: random assignment to the treatment ▶ controlled: having a control group allows measuring the treatment eect ▶ experiment: subjects have no choice, no reverse causality, no selection into treatment Martin / Zulehner: Introductory Econometrics 4 / 24 Example: test scores, student-teacher ratios and percentage english learners estimated regression line: test score =698.933 - 2.27× str (unit 1 page 56) estimated regression line: test score =686.03 - 1.10× str - 0.65× pctel districts with one more student per teacher on average have test scores that are 1.10 points lower Exercise: calculate the omitted variable bias Martin / Zulehner: Introductory Econometrics 5 / 24 Marginal eects in general In most general form, the model can be written as k X g (yi ) = βj hj (xij ) + εi , j=1 where g (·) and h(·) are independent observable functions of yi and xj , j = 1,... , k. Typical examples are logarithmic, exponential or polynomial functions. E.g., if g (.) and h(.) are logarithmic functions, then, ln yi = (ln x i )′ β + εi. - Martin / Zulehner: Introductory Econometrics 6 / 24 The three log regression specications 1 linear-log: yi = β0 + β1 ln (xi ) + ui 2 log-linear: ln(yi ) = β0 + β1 xi + ui 3 log − log: ln(yi ) = β0 + β1 ln(xi ) + ui ▶ the interpretation of the slope coecient diers in each case ▶ the interpretation is found by applying the general "before and after" rule: gure out the change in y for a given change in x” ▶ each case has a natural interpretation (for small changes in x ) Model Dep. Var. Indep. Var. β1 Interpretation of linear / level-level y x ∆y = β1 ∆x linear-log / level-log y ln(x) ∆y = (β1 /100)%∆x log-linear/log-level ln(y) x %∆y = (100β1 )∆x log-log ln(y) ln(x) %∆y = β1 %∆x exploit: ln(x + ∆x) − ln(x) = ln(1 + ∆x ) ∼ = ∆x x x Martin / Zulehner: Introductory Econometrics 7 / 24 Linear-log population regression function compute y before and after changing x : y = β0 + β1 ln(x) (before) now change x: y + ∆y = β0 + β1 ln(x + ∆x) (after) subtract (after) - before): ∆y = β1 [ln(x + ∆x) − ln(x)] now ln(x + ∆x) − ln(x) ∼ = ∆x x ▶ ∼ β1 so ∆y = ∆x x ▶ or ∼ ∆y ( small ∆x) β1 = ∆x/x Yi = β0 + β1 ln (xi ) + ui ▶ for small ∆x : β ∼ ∆y 1 = ∆x/x ▶ now 100 × ∆x x = percentage change in x , so a 1% increase in x (multiplying x by 1.01) is associated with a.01 × β1 change in y ▶ 1% increase in x →.01 increase in ln(x) →.01 × β1 increase in y Martin / Zulehner: Introductory Econometrics 8 / 24 Example: test scores and income Linear-log population regression function: test score vs. ln(income) ▶ rst dening the new regressor, ln(income) ▶ the model is now linear in ln(income), so the linear-log model can be estimated by OLS: test scorei =557.8 + 36.42 × ln(incomei ) (3.8) (1.40) so a 1% increase in income is associated with an increase of 0.36 points in test score standard errors, condence intervals, R2 - all the usual tools of regression apply here true also for log-linear and log-log model Martin / Zulehner: Introductory Econometrics 9 / 24 Log-linear population regression function ln(y ) = β0 + β1 x now change X : ln(y + ∆y ) = β0 + β1 (x + ∆x) subtract (a) − (b) : ln(y + ∆y ) − ln(y ) = β1 ∆x ∆y ∼ ▶ so: y = β1 ∆x ▶ or: β1 ∼ = ∆y /y ∆x ( small ∆x) ln(yi ) = β0 + β1 xi + ui ▶ for small∆x, β1 ∼ ∆y /y = ∆y ▶ now 100 × ∆y y = percentage change in y , so a change in x by one unit (∆x = 1) is associated with a 100 × β1 % change in y ▶ a 1 unit increase in X → β1 increase in ln(y ) → 100 × β1 % increase in y Martin / Zulehner: Introductory Econometrics 10 / 24 Log-log population regression function ln(y ) = β0 + β1 ln(x) now change x : ln(y + ∆y ) = β0 + β1 ln(x + ∆x) subtract: ln(y + ∆y ) − ln(y ) = β1 [ln(x + βx) − ln(x)] ▶ ∆y so y ∼ = β1 ∆x x ▶ or β1 ∼ = ∆y /y ∆x/x ( small ∆x) ln(yi ) = β0 + β1 ln(xi ) + ui ▶ for small ∆x : β1 ∼ ∆y /y = ∆x/x ∆y ∆x ▶ now 100 × y = percentage change in y , and 100 × x = percentage change in x , so a 1% change in x is associated with a β1 % change in y ▶ in the log-log specication, β1 has the interpretation of an elasticity. Martin / Zulehner: Introductory Econometrics 11 / 24 Example: test scores and income log-log: ln(test score) = 6.336 (0.006) + 0.0554× ln(income) (0.0021) (eg: an 1% increase in income is associated with an increase of.0554% in test score) log-log looks slightly better Martin / Zulehner: Introductory Econometrics 12 / 24 Changes in log points vs percentages example: ln(y ) = β0 + β1 × female with female a 0/1 dummy variable ▶ β1 = −.05 (-5 log points) → % change −0.0488 × 100 = −4.8% ▶ β1 = −.15 (-15 log points) → % change −0.1392 × 100 = −13.9% ▶ β1 = −.30 (-30 log points) → % change −0.2592 × 100 = −25.9% ▶ β1 =.30 (+30 log points) → % change 0.3499 × 100 = 35.0% percentage changes, if β 's are small ▶ log changes are roughly equal to percentage changes percentage changes, if β 's are larger: 100 ∗ (exp(βj ) − 1) ▶ → ln(yF ) = β0 + β1 and ln(yM ) = β0 ▶ → ln(yF ) − ln(yM ) = β1 ▶ → ln( yyMF ) = β1 ▶ → yyMF = exp(β1 ) yF −yM ▶ → yM = exp(β1 ) − 1 Martin / Zulehner: Introductory Econometrics 13 / 24 Dummy variables a dummy variable is a variable that takes either 0 or 1 as values, eg female = 1 if individual is a women and = 0 else suppose you have a set of multiple binary (dummy) variables, which are mutually exclusive and exhaustive that is, there are multiple categories and every observation falls in one and only one category (Freshmen, Sophomores, Juniors, Seniors, Other). if you include all these dummy variables and a constant, you will have perfect multicollinearity - this is sometimes called the dummy variable trap Why is there perfect multicollinearity here? Solutions to the dummy variable trap: 1 omit one of the groups (e.g. Freshmen), or 2 omit the intercept What are the implications of (1) or (2) for the interpretation of the coecients? Martin / Zulehner: Introductory Econometrics 14 / 24 Results from wage regressions ln(wage) = β0 + β1 x1 +... + βk xk + u Variable women men ∆ in PP # Observations 5.422 11.043 Adjusted R-square 0,638 0,619 Constant 1.625* 1.848* -0,223* Education (reference: compulsory school) Apprenticeship 0.180* 0.230* -0.050* BMS, nurse's training school 0.256* 0.284* -0.028* Highschool (AHS, BHS), university course 0.371* 0.431* -0.060* Work master craftsman's certicate 0.281* 0.295* -0.014 University of applied science, academy 0.415* 0.451* -0.036 University 0.538* 0.612* -0.074* University (Second degree) 0.616* 0.666* -0.050 dierences in coecients reect dierences in choice of school, profession and programme of study sample: full-time employees, priv. + pub. sector, ∗ signicant at the 95% level Martin / Zulehner: Introductory Econometrics 15 / 24 Estimated coecients from separate estimates Variable women men ∆ in PP Professional experience 0.045* 0.049* -0.004 squared × 100 -0.086* -0.096* 0.010 Duration of employment 0.008* 0.008* 0.000 squared × 100 -0.002 0.010* -0.012 Partnership 0.006 0.058* -0.052* Leading position 0.117* 0.092* -0.025 Firm Ratio of women to men -0,164* -0,221* 0,055* Wage of women/wage of men 0,024 -0,179* 0,203* no dierences in payment for experience and tenure between women and men married men earn 5% more than unmarried men, this does not apply to women a higher proportion of women in the rm leads to lower wages for both women and men sample: full-time employees, priv. + pub. sector, ∗ signicant at the 95% level Martin / Zulehner: Introductory Econometrics 16 / 24 Nonlinear regression functions Motivation ▶ the regression function so far has been linear in the X 's ▶ but the linear approximation is not always a good one ▶ the multiple regression model can handle regression functions that are nonlinear in one or more X What can we do about it? 1 Nonlinear regression functions - general comments 2 Nonlinear functions of one variable 3 Nonlinear functions of two variables: interactions 4 Application to the California Test Score data set Martin / Zulehner: Introductory Econometrics 17 / 24 The test score student to teacher ratio relation looks linear (maybe) Martin / Zulehner: Introductory Econometrics 18 / 24 But the test score income relation looks nonlinear Martin / Zulehner: Introductory Econometrics 19 / 24 Nonlinear functions of one variable We'll look at two complementary approaches: 1 Polynomials in x ▶ The population regression function is approximated by a quadratic, cubic, or higher-degree polynomial 2 Logarithmic transformations ▶ y and/or x is transformed by taking its logarithm, which provides a percentages interpretation of the coecients that makes sense in many applications Martin / Zulehner: Introductory Econometrics 20 / 24 Polynomials in X Approximate the population regression function by a polynomial: yi = β0 + β1 xi + β2 xi2 +... + βr xir + ui This is just the linear multiple regression model - except that the regressors are powers of x ! Estimation, hypothesis testing, etc. proceeds as in the multiple regression model using OLS The coecients are dicult to interpret, but the regression function itself is interpretable Example: test score income relation Incomei = average income in the i th district (thousands of dollars per capita) Quadratic specication: TestScorei = β0 + β1 Incomei + β2 Income2i + ui Cubic specication: TestScorei = β0 + β1 Incomei + β2 Income2i + β3 Income3i + ui Martin / Zulehner: Introductory Econometrics 21 / 24 Interpretation of the estimated regression function estimated regression line: test score = 607.93 (2.9) + 2.28 × income (0.27) - 2 0.0423 × income (0.0048) Martin / Zulehner: Introductory Econometrics 22 / 24 Interpretation of the estimated regression function testing the null hypothesis of linearity, against the alternative that the population regression is quadratic and/or cubic, that is, it is a polynomial of degree up to 3 : ▶ H : population coecients on Income2 = 0 and Income3 = 0 0 ▶ H : at least one of these coecients is nonzero 1 ▶ F(2, 416)=37.69 with Prob > F = 0.0000 The hypothesis that the population regression is linear is rejected at the 1% signicance level against the alternative that it is a polynomial of degree up to 3 Martin / Zulehner: Introductory Econometrics 23 / 24 Summary: polynomial regression functions yi = β0 + β1 xi + β2 xi2 +... + βr xir + ui Estimation: by OLS after dening new regressors The individual coecients have complicated interpretations ▶ To interpret the estimated regression function: ▶ plot predicted values as a function of x ▶ compute predicted ∆y /∆x for dierent values of x Hypotheses concerning degree r can be tested by t - and F -tests on the appropriate (blocks of ) variable(s). ▶ Choice of degree r ▶ plot the data; t - and F -tests, check sensitivity of estimated eects; judgement. ▶ Or use model selection criteria (later) Martin / Zulehner: Introductory Econometrics 24 / 24