PSCI 2702 Textbook: Statistics for Social Research - PDF
Document Details
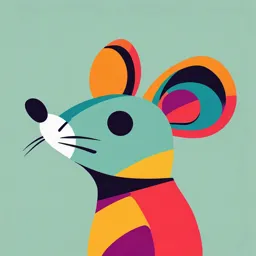
Uploaded by ConscientiousEvergreenForest1127
Toronto Metropolitan University
Tags
Summary
This textbook, "Statistics: A Tool for Social Research and Data Analysis", explores the role of statistics within social science research. It details how social scientists use statistical methods to verify ideas and theories, and highlights the importance of data analysis for the advancement of knowledge in this field. The book explains that without statistics, meaningful quantitative research is impossible.
Full Transcript
Book Title: eTextbook: Statistics: A Tool for Social Research and Data Analysis, Fifth Canadian Edition Chapter 1. Introduction 1.1. Why Study Statistics? 1.1. Why Study Statistics? Students sometimes approach their first course in statistics with questions...
Book Title: eTextbook: Statistics: A Tool for Social Research and Data Analysis, Fifth Canadian Edition Chapter 1. Introduction 1.1. Why Study Statistics? 1.1. Why Study Statistics? Students sometimes approach their first course in statistics with questions about the value of the subject matter. What, after all, do numbers and statistics have to do with understanding people and society? In a sense, this entire textbook will attempt to answer this question, and the value of statistics will become clear as we move from chapter to chapter. For now, the importance of statistics can be demonstrated, in a preliminary way, by briefly reviewing the research process as it operates in the social sciences. These disciplines are scientific in the sense that social scientists attempt to verify their ideas and theories through research. Broadly conceived, research is any process by which information is systematically and carefully gathered for the purpose of answering questions, examining ideas, or testing theories. Research is a disciplined inquiry that can take numerous forms. Statistical analysis is relevant only for those research projects where the information collected is represented by numbers. Numerical information is called data, and the sole purpose of statistics is to manipulate and analyze data. Statistics, then, are a set of mathematical techniques used by social scientists to organize and manipulate data for the purpose of answering questions and testing theories. Sometimes, the numbers obtained after applying these techniques are also called statistics. What is so important about learning how to manipulate data? On the one hand, some of the most important and enlightening works in the social sciences do not use any statistical techniques. There is nothing magical about data and statistics. The mere presence of numbers guarantees nothing about the quality 11 of a scientific inquiry. On the other hand, data can be the most trustworthy information available to the researcher and, consequently, deserve special attention. Data that have been carefully collected and thoughtfully analyzed are the strongest, most objective foundations for building theory and enhancing understanding. Without a firm base in data, the social sciences would lose the right to the name science and would be of far less value. Thus, the social sciences rely heavily on data analysis for the advancement of knowledge. Let us be very clear about one point: it is never enough merely to gather data (or, for that matter, any kind of information). Even the most objective and carefully collected numerical information does not and cannot speak for itself. The researcher must be able to use statistics effectively to organize, evaluate, analyze, and interpret the data. Without a good understanding of the principles of statistical analysis, the researcher cannot make sense of the data. Without the appropriate application of statistical techniques, the data remain mute and useless. Statistics are an indispensable tool for the social sciences. They provide the scientist with some of the most useful techniques for evaluating ideas, testing theory, and discovering the truth. The next section describes the relationships among theory, research, and statistics in more detail. Book Title: eTextbook: Statistics: A Tool for Social Research and Data Analysis, Fifth Canadian Edition Chapter 1. Introduction 1.2. The Role of Statistics in Scientific Inquiry 1.2. The Role of Statistics in Scientific Inquiry Figure 1.1 uses a wheel to graphically represent the role of statistics in the research process. The diagram is based on the thinking of Walter Wallace and illustrates how the knowledge base of any scientific enterprise grows and develops. One point the diagram makes is that scientific theory and research continually shape each other. Statistics are one of the most important means by 12 which research and theory interact. Let’s take a closer look at the wheel. Figure 1.1 The Wheel of Science Source: Adapted from W. Wallace, The Logic of Science in Sociology. Copyright © 1971 by Transaction Publishers. Reprinted by permission of the publisher. Because the figure is circular, it has no beginning or end, and we could begin our discussion at any point. For the sake of convenience, let’s begin at the top and follow the arrows around the circle. A theory is an explanation of the relationships among phenomena. People naturally (and endlessly) wonder about problems in society (such as prejudice, poverty, child abuse, or serial murders), and in their attempts to understand these phenomena, they develop explanations (“lack of education causes prejudice”). This kind of informal “theorizing” about society is no doubt very familiar to you. One major difference between our informal, everyday explanations of social phenomena and scientific theory is that the latter is subject to a rigorous testing process. Let’s take the problem of health inequality as an example to illustrate how the research process works. Why do people differ in their health? One possible answer to this question is provided by the materialistic theory. This theory was stated over 40 years ago in the groundbreaking Black Report, named after the physician Sir Douglas Black, on health inequalities in the United Kingdom. It has been tested on a number of occasions since that time. According to the materialistic theory, health is affected by social class. We often think it is the individual who chooses whether to have a healthy diet, participate in leisure exercise, protect their own health and prevent illness (e.g., annual physical, flu shot), and so on. The materialist theory asserts that such “individual choices” are constrained by one’s social class. Social class is linked to health by one’s access to resources and commodities such as health care; preventative health care services; adequate diet; and safe housing, living, and working conditions that are necessary to maintain and improve health. The materialistic theory is not a complete explanation of health inequality, but it can serve to illustrate a sociological theory. This theory explains the relationship between two social phenomena: (1) health inequality and (2) social class. People of a lower social class will have poorer health, and those of a higher social class will have better health. Before moving on, let’s examine theory in a little more detail. The materialistic theory, like most theories, is stated in terms of causal relationships between variables. A variable is any trait that can change values from case to case. Examples of variables are gender, age, ethnicity, and political party affiliation. (Remember that a variable must be able to vary—so if your study includes only women, then gender is not a variable.) In any specific theory, some variables will be identified as causes and others will be identified as effects or results. In the language of science, the causes are called independent variables and the 13 effects or result variables are called dependent variables. In our theory, social class is the independent variable (or the cause) and health is the dependent variable (the result or effect). In other words, we are arguing that social class is a cause of health conditions or that an individual’s level of health depends on their social class. Vadym Pastukh/www.shutterstock.com A variable is any trait that can change values from case to case, such as age, gender, ethnicity, or social class. Diagrams can be a useful way of representing the relationships between variables: Social class → Health Independent variable → Dependent variable X→Y The arrow represents the direction of the causal relationship, and X and Y are general symbols for the independent and dependent variables, respectively. So far, we have a theory of health and an independent and a dependent variable. What we do not know yet is whether the theory is true or false. To find out, we need to compare our theory with the facts—we need to do some research. The next step in the process is to define our terms and ideas more specifically and exactly. One problem we often face in conducting research is that scientific theories are too complex and abstract to be fully tested in a 14 single research project. To conduct research, one or more hypotheses must be derived from the theory (see Figure 1.1). A hypothesis is a statement about the relationship between variables that, while logically derived from the theory, is much more specific and exact. For example, if we wish to test the materialistic theory, we have to say exactly what we mean by social class and health. There has been a great deal of research on the effects of social class on health, and we must consult the research literature to develop and clarify our definitions of these concepts. As our definitions develop and the hypothesis takes shape, we begin the next step of the research process, during which we decide exactly how we will gather our data. We must decide how cases will be selected and tested and how the variables will be measured. Ultimately, these plans will lead to the observation phase (the bottom of the wheel of science), where we actually measure social reality. Before we can do this, however, we must have a very clear idea of what we are looking for and a well-defined strategy for conducting the search. To test the materialistic theory, we begin by considering people from all sections of society (rich, poor, healthy, unhealthy, and so on). Then, we need to decide what our measures of social class and health status are. In other words, we need to operationalize the concepts of social class and health status. For example, we may decide to use those measures used in the Black Report: occupation and chronic illness as measures of social class and health, respectively. We would then administer a survey that asked each person, “What is your current main occupation?” and “How many long-term or chronic medical conditions diagnosed by a health professional do you have?” Our goal would be to see whether people with higher occupational status have fewer chronic illnesses. Now, finally, we come to statistics. As the observation phase of our research project comes to an end, we are confronted with a large collection of numerical information or data. If our sample consists of 2,000 people, we will have 2,000 completed surveys measuring social class and health. Try to imagine dealing with 2,000 completed surveys. If we ask each respondent five questions about their health, instead of just one question, we will have a total of 10,000 separate pieces of information to deal with. What do we do? We need to have some systematic way to organize and analyze this information; at this point, statistics are very valuable. Statistics provide us with many ideas about “what to do” with the data (we will begin to look at some of the options in Chapter 2). For now, let us stress two points about statistics. First, statistics are crucial. Simply put, without statistics, quantitative research is impossible. Without quantitative research, the development of the social sciences would be severely impaired. Only through the application of statistical techniques can mere data help us shape and refine our theories and understand the social world better. Second, and somewhat paradoxically, the 15 role of statistics is rather limited. As Figure 1.1 makes clear, scientific research proceeds through several mutually interdependent stages, and statistics become directly relevant only at the end of the observation stage. Before any statistical analysis can be legitimately applied, the preceding phases of the process must be successfully completed. If the researcher has asked poorly conceived questions or has made serious errors of design or method, then even the most sophisticated statistical analysis is valueless. As useful as they can be, statistics cannot substitute for rigorous conceptualization, detailed and careful planning, or creative use of theory. Statistics cannot salvage a poorly conceived or badly designed research project. They cannot make sense out of garbage. Conversely, inappropriate statistical applications can limit the usefulness of an otherwise carefully done project. Only by successfully completing all phases of the process can a quantitative research project hope to contribute to understanding. A reasonable knowledge of the uses and limitations of statistics is as essential to the education of the social scientist as is training in theory and methodology. As the statistical analysis comes to an end, we begin to develop empirical generalizations (see Figure 1.1). While we should be primarily focused on assessing our theory, we also look for other trends in the data. Assuming that we find that social class is linked to health in general, we might go on to ask if the pattern applies to all genders or to older respondents as well as to younger ones. As we probe the data, we might begin to develop some generalizations based on the empirical patterns we observe. For example, what if we find that social class is linked to health for older respondents but not for younger respondents? Could it be that material advantages and disadvantages have a cumulative effect on health, so that the social class—health link does not emerge until old age? As we develop tentative explanations, we will begin to revise or elaborate our theory. If we change the theory to take these findings into account, however, a new research project designed to test the revised theory is called for and the wheel of science begins to turn again. We (or perhaps some other researchers) will go through the entire process once again with this new and, hopefully, improved theory. This second project might result in further revisions and elaborations that will (you guessed it) require still more research projects, and the wheel of science will continue turning as long as scientists are able to suggest additional revisions or develop new insights. Every time the wheel turns, our understandings of the phenomena under consideration will (hopefully) improve. This description of the research process does not include white-coated, clipboard-carrying scientists who, in a blinding flash of inspiration, discover some fundamental truth about reality and shout, “Eureka!.” The truth is that, in the normal course of science, it is a rare occasion when we can say with absolute certainty that a given theory or idea is definitely true or false. Rather, 16 evidence for (or against) a theory gradually accumulates over time, and ultimate judgments of truth are likely the result of many years of hard work, research, and debate. Let’s briefly review our imaginary research project. We began with an idea or theory about social class and health. We imagined some of the steps we would have to take to test the theory and took a quick look at the various stages of the research project. We wound up back at the level of theory, ready to begin a new project guided by a revised theory. We saw how theory can motivate a research project and how our observations can cause us to revise the theory and, thus, motivate a new research project. Wallace’s wheel of science illustrates how theory stimulates research and how research shapes theory. This constant interaction between theory and research is the lifeblood of science and the key to enhancing our understandings of the social world. The dialogue between theory and research occurs at many levels and in multiple forms. Statistics are one of the most important links between these two realms. Statistics permit us to analyze data, to identify and probe trends and relationships, to develop generalizations, and to revise and improve our theories. As you will see throughout this textbook, statistics are limited in many ways. They are also an indispensable part of the research enterprise. Without statistics, the interaction between theory and research would become extremely difficult, and the progress of our disciplines would be severely hindered. (For practice in describing the relationship between theory and research and the role of statistics in research, see Problems 1.1 and 1.2.) Book Title: eTextbook: Statistics: A Tool for Social Research and Data Analysis, Fifth Canadian Edition Chapter 1. Introduction 1.3. The Goals of this Textbook 1.3. The Goals of this Textbook In the preceding section, we argued that statistics are a crucial part of the process by which scientific investigations are carried out and that, therefore, some training in statistical analysis is a crucial component of the education of every social scientist. In this section, we address the questions of how much training is necessary and what the purposes of that training are. First, this textbook takes the point of view that statistics are tools. They can be a very useful means of increasing our knowledge of the social world, but they are not ends in themselves. Thus, we will not take a “mathematical” approach to the subject. The techniques will be presented as a set of tools that can be used to answer important questions. This emphasis does not mean we will dispense with arithmetic entirely, of course. This textbook includes enough mathematical material to help you develop a basic understanding of why statistics do what they do. Our focus, however, will be on how these techniques are applied in the social sciences. 17 Second, all of you will soon become involved in advanced coursework in your major fields of study, and you will find that much of the literature used in these courses assumes at least basic statistical literacy. Furthermore, many of you, after graduation, will find yourselves in positions—either in a career or in graduate school—where some understanding of statistics will be very helpful or perhaps even required. Very few of you will become statisticians per se (and this textbook is not intended for the pre-professional statistician), but you must have a grasp of statistics in order to read and critically appreciate your own professional literature. As a student in the social sciences and in many careers related to the social sciences, you simply cannot realize your full potential without a background in statistics. Within these constraints, this textbook is an introduction to statistics as they are used in the social sciences. Its general goal is to develop an appreciation—a healthy respect—for statistics and their place in research. You should emerge from this experience with the ability to use statistics intelligently and to know when other people have done so (or not!). You should be familiar with the advantages and limitations of the more commonly used statistical techniques, and you should know which techniques are appropriate for a given set of data and a given purpose. Lastly, you should develop sufficient statistical and computational skills and enough experience in the interpretation of statistics to be able to carry out some elementary forms of data analysis by yourself. Book Title: eTextbook: Statistics: A Tool for Social Research and Data Analysis, Fifth Canadian Edition 1.4. Descriptive and Inferential Statistics 1.4. Descriptive and Inferential Statistics As noted earlier, the general function of statistics is to manipulate data so that research questions can be answered. In this section, we introduce the two general classes of statistical techniques that, depending on the research situation, are available to accomplish this task. Book Title: eTextbook: Statistics: A Tool for Social Research and Data Analysis, Fifth Canadian Edition 1.4. Descriptive and Inferential Statistics Descriptive Statistics Descriptive Statistics The first class of techniques is called descriptive statistics and is relevant in several different situations: 1. Statistics used when the researcher needs to summarize or describe the distribution of a single variable are called univariate (one variable) descriptive statistics. 2. Statistics used when the researcher wishes to describe the relationship between two or more variables are called bivariate (two variables) or multivariate (more than two variables) descriptive statistics. To describe a single variable, we arrange the values or scores of that variable so that the relevant information can be quickly understood and appreciated. 18 Many of the statistics that might be appropriate for this summarizing task are probably familiar to you. For example, percentages, graphs, and charts can all be used to describe single variables. To illustrate the usefulness of univariate descriptive statistics, consider the following problem: Suppose you wanted to summarize the distribution of the variable “family income” for a community of 10,000 families. How would you do it? Obviously, you couldn’t simply list all incomes in the community and let it go at that. Imagine trying to make sense of a list of 10,000 different incomes! Presumably, you would want to develop some summary measures of the overall income distributions—perhaps an arithmetic average or the proportions of incomes that fall in various ranges (such as low, middle, and high). Or perhaps a graph or a chart would be more useful. Whatever specific method you choose, its function is the same: to reduce these thousands of individual items of information into a few easily understood numbers. The process of allowing a few numbers to summarize many numbers is called data reduction and is the basic goal of univariate descriptive statistical procedures. By contrast, bivariate and multivariate descriptive statistics are designed to help the investigator understand the relationship between two or more variables. These statistics, also called measures of association, allow the researcher to quantify the strength and direction of a relationship. These statistics are very useful because they enable us to investigate two matters of central theoretical and practical importance to any science: causation and prediction. These techniques help us disentangle and uncover the connections between variables. They help us trace the ways in which some variables might have causal influences on others, and, depending on the strength of the relationship, they enable us to predict scores on one variable from the scores on another. Note that measures of association cannot, by themselves, prove that two variables are causally related. However, these techniques can provide valuable clues about causation and are therefore extremely important for theory testing and theory construction. For example, suppose you were interested in the relationship between “time spent studying statistics” and “final grade in statistics” and had gathered data on these two variables from a group of university students. By calculating the appropriate measure of association, you could determine the strength of the bivariate relationship and its direction. Suppose you found a strong, positive relationship. This would indicate that “study time” and “grade” were closely related (strength of the relationship) and that as one increased in value, the other also increased (direction of the relationship). You could make predictions from one variable to the other (“the longer the study time, the higher the grade”). As a result of finding this strong, positive relationship, you might be tempted to make causal inferences. That is, you might jump to such conclusions as “longer study time leads to (causes) higher grades.” Such a conclusion might make a 19 good deal of common sense and would certainly be supported by your statistical analysis. However, the causal nature of the relationship cannot be proven by the statistical analysis. Measures of association can be taken as important clues about causation, but the mere existence of a relationship can never be taken as conclusive proof of causation. In fact, other variables might affect the relationship. In the example above, we probably would not find a perfect relationship between “study time” and “final grade.” That is, we would probably find some individuals who spend a great deal of time studying but receive low grades, and some individuals who fit the opposite pattern. We know intuitively that other variables besides study time affect grades (such as efficiency of study techniques, amount of background in mathematics, and even random chance). Fortunately, researchers can also incorporate these other variables into the analysis and measure their effects. Book Title: eTextbook: Statistics: A Tool for Social Research and Data Analysis, Fifth Canadian Edition 1.4. Descriptive and Inferential Statistics Inferential statistics Inferential statistics Inferential statistics, the second class of statistical techniques, become relevant when we wish to generalize our findings from a sample to a population. A population is the total collection of all cases that the researcher is interested in and wishes to understand better. Examples of possible populations are all eligible voters in Canada, unemployed youth in the Greater Toronto Area, and students enrolled at the University of British Columbia. Monkey Business Images/Shutterstock.com A population is the total collection of all cases that the researcher is interested in understanding better—for example, all school-aged children in Canada. However, it is impossible to test every case in such a population, so researchers turn to inferential statistics—using information from a sample subset of the population to make generalizations. Populations can theoretically range from inconceivable in size (all humanity) to quite small (all professional hockey players residing in the City of Ottawa) but are usually fairly large. In fact, they are almost always too large to be measured. To put the problem another way, social scientists almost never have the resources or time to test every case in a population. Hence the need for inferential statistics, which involve using information from a sample (a carefully chosen subset of the population) to make inferences about a population. Because they have fewer cases, samples are much cheaper to assemble, and—if the proper techniques are followed—generalizations based on these samples can be very accurate representations of the population. There are two general uses of inferential statistics: estimation and hypothesis testing. While many of the concepts and procedures involved in inferential statistics may be unfamiliar, most of us are experienced consumers of them— 20 most familiarly, perhaps, in the form of public opinion polls and election projections. When a public opinion poll reports that 36 percent of the Canadian electorate plans to vote for a certain political party, it is essentially reporting a generalization to a population (the “Canadian electorate,” which numbered about 27 million people in 2019) from a carefully drawn sample (usually about 1,500 respondents). Book Title: eTextbook: Statistics: A Tool for Social Research and Data Analysis, Fifth Canadian Edition 1.4. Descriptive and Inferential Statistics The Descriptive–Inferential Statistics Divide The Descriptive–Inferential Statistics Divide The textbook is divided into four parts, with each part focusing on one or both of the two fundamental (descriptive and inferential) classes of statistics. Part 1 reviews statistical procedures that describe the distribution of a single variable (i.e., univariate descriptive statistics). These procedures include frequency distributions and graphs (Chapter 2), measures of central tendency and dispersion (Chapter 3), and Z scores and the normal curve (Chapter 4). Part 2 of the textbook provides a transition from descriptive to inferential statistics. Chapter 5 looks at the logic behind inferential statistics, and Chapter 6 looks at one of its two main applications: estimation. In Part 3 we turn our attention to bivariate relationships, where both measures of association (descriptive statistics) and hypothesis testing (the other main application of inferential statistics) are discussed. This part of the textbook is organized according to the level of measurement of the variables (to be discussed in Section 1.6) whose relationship we are going to examine. We begin with a discussion of hypothesis testing for nominal or ordinal variables in Chapter 7. Next, we look at measures of association designed for situations where both variables are measured at the nominal level in Chapter 8 and the ordinal level in Chapter 9. Before we move on to situations where both variables are measured at the interval-ratio level (Chapter 13), we first consider measures of association and hypothesis testing for situations where we have a combination of nominal, ordinal, and interval-ratio variables, in Chapters 10, 11 and 12. Part 4 introduces multivariate descriptive statistics used to describe the relationship between three or more interval-ratio variables. Partial correlation and multiple regression (Chapter 14) are multivariate extensions of the techniques introduced in Chapter 13. In summary, Figure 1.2 provides a diagram outlining the structure of this textbook, in which descriptive and inferential statistical procedures are divided by type of analysis (univariate, bivariate, multivariate) and uses of inferential statistics (estimation, hypothesis testing). As we will see in the next two sections of this chapter, the nature of the variable(s) under consideration largely determines what statistical procedure to use in our research. (For practice in describing different statistical applications, see Problems 1.3 and 1.7.) 21 Figure 1.2 An Overview of Statistical Procedures, by Type of Analysis and Inferential Statistic Book Title: eTextbook: Statistics: A Tool for Social Research and Data Analysis, Fifth Canadian Edition Chapter 1. Introduction 1.5. Discrete and Continuous Variables 1.5. Discrete and Continuous Variables In Chapter 2, you will begin to encounter some of the broad array of statistics available to social scientists. One aspect of using statistics that can be puzzling is deciding when to use which statistic. You will learn specific guidelines as you go along, but we will introduce some basic and general guidelines at this point. The first of these concerns discrete and continuous variables; the second, covered in the next section, concerns level of measurement. A variable is said to be discrete if it has a basic unit of measurement that cannot be subdivided. For example, number of people per household is a 22 discrete variable. The basic unit is people, a variable that will always be measured in whole numbers: you’ll never find 2.7 people living in a specific household. The scores for a discrete variable will be zero, one, two, three, or some other whole integer. Other discrete variables include number of siblings, children, or cars. To measure these variables, we count the number of units for each case and record results in whole numbers. A variable is continuous if its measurement can be subdivided infinitely—at least in a theoretical sense. One example of a continuous variable is time, which can be measured in minutes, seconds, milliseconds (thousandths of a second), nanoseconds (billionths of a second), or even smaller units. In a sense, when we measure a continuous variable, we are always approximating and rounding off the scores. We could report somebody’s time in the 100-metre dash as 10.73 seconds or 10.732451 seconds, but, because time can be infinitely subdivided (if we have the technology to make the precise measurements), we can never report the exact time elapsed. Since we cannot work with infinitely long numbers, we must report the scores on continuous variables as if they were discrete. The distinction between these two types of variables relates more to measuring and processing the information than to the appearance of the data. This distinction between discrete and continuous variables is one of the most basic in statistics and will constitute one of the criteria by which we will choose among various statistics and graphic devices. (For practice in distinguishing between discrete and continuous variables, see Problems 1.4, 1.5, 1.6, 1.7, and 1.8.) Book Title: eTextbook: Statistics: A Tool for Social Research and Data Analysis, Fifth Canadian Edition 1.6. Level of Measurement 1.6. Level of Measurement A second basic and very important guideline for the selection of statistics is the level of measurement or the mathematical nature of the variables under consideration. The best way to identify a variable’s mathematical nature is to look at its response categories. Response categories are a variable’s possible attributes, qualities, or characteristics. For example, the response categories of a variable measuring a person’s first-learned language might be “English,” “French,” “English and French,” and “Neither English nor French.” In other words, where the discrete–continuous distinction looks at a variable’s unit of measurement, the level of measurement distinction looks at a variable’s response categories. Variables at the highest level of measurement have numerical scores and can be analyzed with a broad range of statistics. Variables at lower levels of measurement have “scores” that are really just labels, not numbers at all. Using numerical variables with statistics that require non-numerical variables is inappropriate and often completely meaningless. When selecting statistics, you 23 must be sure that the level of measurement of the variable justifies the mathematical operations required to compute the statistic. For example, consider the variables “age” (when measured in years, rather than in year ranges) and “income” (when measured in dollars, rather than in dollar ranges). Both of these variables have numerical scores and could be summarized with a statistic such as the mean or average (e.g., “The average income of this city is $93,000”; “The average age of students on this campus is 21.7 years”). However, the mean or average would be meaningless as a way of describing gender or area codes, which are variables with non-numerical scores. Your personal area code might look like a number, but it is merely an arbitrary label that happens to be expressed in digits. These “numbers” cannot be added or divided, and statistics like the average cannot be applied to this variable: the average area code of a group of people is a meaningless statistic. Determining the level at which a variable has been measured is one of the first and most foundationally important steps in any statistical analysis, and we will consider this matter at some length. We will make it a practice throughout this textbook to introduce level-of-measurement considerations for each statistical technique. The three levels of measurement, in order of increasing sophistication, are nominal, ordinal, and interval-ratio. Each is discussed separately. To reiterate an important point, we need to examine a variable’s response categories— rather than the variable’s definition—in order to identify the variable’s level of measurement. Book Title: eTextbook: Statistics: A Tool for Social Research and Data Analysis, Fifth Canadian Edition 1.6. Level of Measurement Nominal Level of Measurement Nominal Level of Measurement Variables measured at the nominal level simply classify observations into “scores” or categories. Examples of variables at this level are gender, area code, province of residence, religious affiliation, and place of birth. At this lowest level of measurement, the only mathematical operation permitted is comparing the relative sizes of the categories (e.g., “there are more females than other genders in this residence”). The categories or scores of nominal- level variables cannot be ranked with respect to one another and cannot be added, divided, or otherwise manipulated mathematically. Even when the scores or categories are expressed in digits (such as area codes or street addresses), all we can do is compare relative sizes of categories (e.g., “the most common area code on this campus is 416”). The categories themselves are not a mathematical scale: they are different from one another but not more or less, or higher or lower, than one another. There are differences in terms of gender, but no category has more or less gender than any other. In the same way, an area code of 604 is different from but not “more than” an area code of 416. 24 Although nominal variables are rudimentary, we need to observe certain criteria and procedures in order to ensure adequate measurement. In fact, these criteria apply to variables measured at all levels, not just nominal variables. First, the categories of nominal-level variables must be mutually exclusive so that no ambiguity exists concerning classification of any given case. There must be one and only one category for each case. Second, the categories must be exhaustive; that is, a category must exist for every possible score that might be found, even if it is only another or miscellaneous category. Third, the categories of nominal variables should be relatively homogeneous; that is, our categories should include cases that are comparable. To put it another way, we need to avoid categories that lump apples with oranges. There are no hard and fast guidelines for judging whether a set of categories is appropriately homogeneous. The researcher must make that decision in terms of the specific purpose of the research. Categories that are too broad for some purposes may be perfectly adequate for others. Table 1.1 demonstrates some errors of measurement in four different schemes for measuring the variable “major field of study at university.” Scale A in the table violates the criterion of mutual exclusivity because of the overlap between the categories “Social sciences” and “Sociology.” Scale B is not exhaustive because it does not provide a category for those majoring in a field other than the eight listed. Scale C uses a category (“Non-business”) that is too broad (not homogeneous enough) for many research purposes. Scale D meets the basic criteria of mutually exclusive, exhaustive, and homogeneous. It is important to point out that, while satisfactory, the categories in Scale D may still be too general for some research projects. For example, it is not possible using this scale to investigate differences between social science disciplines such as anthropology, criminology, economics, geography, political science, 25 psychology, and sociology. Table 1.1 Four Scales for Measuring Major Field of Study for University Students Scale A (not Scale B (not Scale C (not Scale D (an mutually exhaustive) homogeneous) adequate scale exclusive) Business Business Business Business Humanities Humanities Non-business Humanities Health Health Health Education Education Education Engineering Engineering Engineering Physical Physical Physical sciences sciences sciences Math/computer Math/computer Math/compute science science science Social sciences Social sciences Social sciences Sociology Other Other Numerical labels are often used to identify the categories of variables measured at the nominal level. This practice is especially common when the data are being prepared for computer analysis. For example, the various major fields of study might be labelled with a 1 indicating “Business,” a 2 signifying “Humanities,” and so on. Remember that these numbers are merely labels or names and have no numerical quality to them. They cannot be added, subtracted, multiplied, or divided. The only mathematical operation permissible with nominal variables is counting and comparing the number of cases in each category of the variable. Book Title: eTextbook: Statistics: A Tool for Social Research and Data Analysis, Fifth Canadian Edition 1.6. Level of Measurement Ordinal Level of Measurement Ordinal Level of Measurement Variables measured at the ordinal level are more sophisticated than nominal- level variables. They have scores or categories that can be ranked from high to low so that, in addition to classifying cases into categories, we can describe the categories in terms of “more or less” with respect to one another. Thus, with variables measured at this level, not only can we say that one case is different from another; we can also say that one case is higher or lower, or more or less, than another. For example, the variable “socioeconomic status (SES)” is usually measured at the ordinal level. The categories of the variable are often ordered according to the following scheme: 4. upper class 3. middle class 2. working class 1. lower class Individual cases can be compared in terms of the categories into which they are classified. Thus, an individual classified as a 4 (upper class) ranks higher than an individual classified as a 2 (working class), and a person classified as a 1 (lower class) ranks lower than a person classified as a 3 (middle class). Other variables that are usually measured at the ordinal level are attitudes and opinions—for example, prejudice, alienation, or political conservatism. The major limitation of the ordinal level of measurement is that a particular score represents only position with respect to some other score. We can distinguish between high and low scores, but the distance between the scores cannot be described in precise terms. Although we know that a score of 4 is more than a score of 2, we do not know if it is twice as much as 2. Because we don’t know the exact distances from score to score on an ordinal scale, our options for statistical analysis are limited. For example, addition and 26 most other mathematical operations assume that the intervals between scores are exactly equal. If the distances from score to score are not equal, 2+2 might equal 3 or 5 or even 15. Thus, strictly speaking, statistics such as the average or mean (which requires that the scores be added together and then divided by the number of scores) are not permitted with ordinal-level variables. The most sophisticated mathematical operation fully justified with an ordinal variable is the ranking of categories and cases (although, as we will see, it is not unusual for social scientists to take some liberties with this strict criterion). Reading Statistics 1. Introduction By this point in your education, you have developed an impressive array of skills for reading words. Although you may sometimes struggle with a difficult idea or stumble over an obscure meaning, you can comprehend virtually any written work that you are likely to encounter. As you continue your education in the social sciences, you must develop an analogous set of skills for reading numbers and statistics. To help you reach a reasonable level of literacy in statistics, we have included a series of boxed inserts in this textbook called “Reading Statistics.” These will appear in most chapters and will discuss how statistical results are typically presented in the professional literature. Each box will include an extract or quotation from the professional literature so that we can analyze a realistic example. As you will see, professional researchers use a reporting style that is quite different from the statistical language you will find in this textbook. Space in research journals and other media is expensive, and the typical research project requires the analysis of many variables. Thus, a large volume of information must be summarized in very few words. Researchers may express in a word or two a result or an interpretation that will take us a paragraph or more to state. Because this is an introductory textbook, we have been careful to break down the computation and logic of each statistic and to identify, even to the point of redundancy, what we are doing when we use statistics. In this textbook we will never be concerned with more than a few variables at a time. We will have the luxury of analysis in detail and of being able to take pages or even entire chapters to develop a statistical idea or analyze a variable. Thus, a major theme of these boxed inserts is to summarize how our comparatively long-winded (but more careful) vocabulary is translated into the concise language of the professional researcher. When you have difficulty reading words, your tendency is (or at least, should be) to consult reference books (especially dictionaries) to help you identify and analyze the elements (words) of the passage. When you have difficulty reading statistics, you should do exactly the same thing. One tip that might be helpful is to write out a list of your questions as you read and attend classes. Then, make sure that you obtain answers to your satisfaction. Another helpful tip is to ask questions as they arise, so that your questions do not build up as you go along. We hope you will find this textbook a valuable reference, and if you learn enough from it to be able to use any source to help you read statistics, it will have fulfilled one of its major goals. 27 Book Title: eTextbook: Statistics: A Tool for Social Research and Data Analysis, Fifth Canadian Edition 1.6. Level of Measurement Interval-Ratio Level of Measurement Interval-Ratio Level of Measurement The categories of nominal-level variables have no numerical quality to them. Ordinal-level variables have categories that can be arrayed along a scale from high to low, but the exact distances between categories or scores are undefined. Variables measured at the interval-ratio level not only permit classification and ranking but also allow the distance from category to category (or score to score) to be exactly defined. That is to say, interval-ratio variables are measured in units that have equal intervals. For example, the variable “number of people in a family” is measured at the interval-ratio level because it has equal intervals—a family of two is one more than a family of one, a family of three is one more than a family of two, and so on. Other examples of interval-ratio variables are income in dollars (e.g., 10,000 dollars is one more dollar than 9,999 dollars, 43,289 dollars is one more dollar than 43,288 dollars, etc.), number of annual visits to a dentist, and number of years married. We should point out that statisticians sometimes separate or distinguish between the interval level (equal intervals) and the ratio level (equal intervals plus a true zero point, indicating the absence or complete lack of whatever is being measured). As an example, “year of birth” is, strictly speaking, an interval-level variable because, while its unit of measurement (years) has equal intervals (the distance from year to year is 365 days), it does not have a true zero and can represent values below zero (e.g., Julius Caesar, the ancient Roman leader, was born in 100 BCE, or 100 years prior to “Year 0” on the Western calendar used around the world today). On the other hand, “age in years” is a ratio-level variable because it has both equal intervals and an absolute zero point—age zero literally means that there is zero of that variable. The same cannot be said for “year of birth.” Because we find the distinction between the interval and ratio levels to be unnecessarily cumbersome in an introductory textbook, we will treat these two levels as one. Furthermore, most statistical analysis that is appropriate for interval variables is also appropriate for ratio variables. As an overview of our discussion, Table 1.2 presents the basic characteristics of the three levels of measurement. It also shows that the number of permitted mathematical operations increases as we move from nominal to ordinal to interval-ratio levels of measurement. Ordinal-level variables are more sophisticated and flexible than nominal-level variables, and interval-ratio-level variables permit the broadest range of mathematical operations. Table 1.2 Basic Characteristics of the Three Levels of Measurement Level of Examples Measurement Mathematical Measurement Procedures Operations Permitted Nominal Gender, race, Classification Counting religion, into number of marital status categories cases in each category of the variable; comparing sizes of categories Ordinal Socioeconomic Classification All operations status (SES), into above as well attitude and categories as judgments opinion scales plus ranking of “greater of categories than” and with respect “less than” to one another Level of Examples Measurement Mathematical Measurement Procedures Operations Permitted Interval-Ratio Age, number All of the All of the of children, above plus above plus all income description of other distances mathematical between operations scores in (addition, terms of subtraction, equal units multiplication, division, square roots, etc.) Book Title: eTextbook: Statistics: A Tool for Social Research and Data Analysis, Fifth Canadian Edition 1.6. Level of Measurement Level of Measurement and Discrete versus Continuous Variables Level of Measurement and Discrete versus Continuous Variables The distinction made earlier between discrete and continuous variables is a concern only for interval-ratio-level variables. Nominal- and ordinal-level variables are discrete, at least in the way they are usually measured. For example, to measure marital status, researchers usually ask people to choose 28 one category from a list: married, living common law, widowed, and so on. What makes this variable discrete is that respondents can place themselves in a single category only; no subcategories or more refined classifications are permitted. Interval-ratio-level variables, on the other hand, can be either discrete (number of people in a family) or continuous (income or age). Remember that because we cannot work with infinitely long numbers, continuous variables have to be rounded off at some level and reported as if they were discrete. The distinction relates more to our options for appropriate statistics or graphs, not to the appearance of the variables. Book Title: eTextbook: Statistics: A Tool for Social Research and Data Analysis, Fifth Canadian Edition 1.6. Level of Measurement Level of Measurement: A Summary Level of Measurement: A Summary Levels of measurement can be depicted as steps on a ladder, as illustrated in Figure 1.3. As we climb up the ladder, each step possesses the characteristics of the preceding step, plus adds a new characteristic. The bottom or first step of the ladder is the nominal level. Nominal-level variables simply classify observations into categories. The second or middle step of the ladder is the ordinal level. Ordinal-level variables classify observations into categories, but the categories also have an intrinsic ordering—they can be naturally ranked from low to high. The top or third step of the ladder is the interval-ratio level. Interval-ratio variables classify observations into ordered categories or scores, but there is also an equal distance between any two adjacent categories or scores. Figure 1.3 Characteristics of Levels of Measurement Thus, to ascertain the level of measurement of a variable, you need to ask two successive questions about its categories (or scores). The first is, “Do the categories of the variable have an intrinsic order?” If the answer is no, it is a 29 nominal-level variable, and no further question is asked. For example, gender is a nominal variable because its scores do not have an intrinsic order—they can be stated in any order: (1) male, (2) female, (3) other; (1) other, (2) female, (3) male; and so on. If the answer to the first question is yes, then the variable is at least ordinal, so a second, follow-up, question is asked: “Is there an equal distance between categories of the variable?” If the answer to the second question is no, it is an ordinal-level variable; if the answer is yes, it is an interval-ratio variable. Consider a scale measuring self-rated health with the following categories: (1) poor, (2) fair, (3) good, (4), very good, and (5) excellent. Individuals in excellent health are healthier than those in very good health, individuals in very good health are healthier than those in good health, and so on. Scores on self-rated health have an intrinsic order; however, the distance between adjacent scores is unequal. For instance, the distance between a score of 4 (very good) and a score of 5 (excellent) is not the same as the distance between a score of 1 (poor) and a score of 2 (fair). That is, people with scores of 4 and 5 are likely to be in a very similar state of health, while people with scores of 1 and 2 are likely to be in quite different states of health—the distance in health status between a score of 4 and a score of 5 is narrower than the distance between a score of 1 and a score of 2. Thus, self-rated health is only an ordinal-level variable. 30 To help find the level of measurement of a variable, Figure 1.4 provides a flow chart of the two questions and how to proceed when answered. The One Step at a Time box further demonstrates how the two questions can be used to determine the level of measurement of three different variables that measure health. As you can see in this demonstration, the level of measurement is not an inherent feature of what a variable measures but is instead a characteristic of the variable’s response categories. Figure 1.4 Finding the Level of Measurement of a Variable Remember that the level of measurement of a variable is important because different statistics require different mathematical operations. The level of measurement of a variable is the key characteristic that tells us which statistics are permissible and appropriate. Ideally, researchers use only those statistics that are fully justified by the level-of-measurement criteria. In this imperfect world, however, the most powerful and useful statistics (such as the mean) require interval-ratio variables, while most of the variables of interest to the social sciences are only nominal (gender, marital status) or ordinal (attitude scales). Relatively few concepts of interest to the social sciences are so precisely defined that they can be measured at the interval-ratio level. This disparity creates some very real difficulties for social science research. On the one hand, 31 researchers should use the most sophisticated statistical procedures that are fully justified for a particular variable. Treating interval-ratio data as if they were only ordinal, for example, results in a significant loss of information and precision. Treated as an interval-ratio variable, the variable “age” can supply us with exact information regarding the differences between the cases (e.g., “Individual A is three years and two months older than Individual B”). Treated only as an ordinal variable, however, the precision of our comparisons suffers and we can say only that “Individual A is older than (or greater than) Individual B.” On the other hand, given the nature of the disparity, researchers are more likely to treat variables as if they were higher in level of measurement than they actually are. In particular, variables measured at the ordinal level, especially when they have many possible categories or scores, are often treated as if they were interval-ratio because the statistical procedures available at the higher level are more powerful, flexible, and interesting. This practice is common, but researchers should be cautious in assessing statistical results and developing interpretations when the level-of-measurement criterion has been violated. Level of measurement is a very basic characteristic of a variable, and we will always consider it when presenting statistical procedures. Level of measurement is also a major organizing principle for the material that follows, and you should make sure you are familiar with these guidelines. (For practice in determining the level of measurement of a variable, see Problems 1.4, 1.5, 1.6, 1.7, and 1.8.) One Step at a Time Finding the Level of Measurement of a Variable Health1, Health2, and Health3 are three variables that measure health, but each of these variables measures health in a different way. Health1 Health1 is based on the question, “In the past 12 months, did you contact a medical practitioner to discuss your health?” The response categories of Health1 are 1 = No 2 = Yes Question 1: Are the response categories ordered? SHOW ANSWER Health2 Health2 is based on the question, “In the past 12 months, how many times did you contact a medical practitioner to discuss your health?” The response categories of Health2 are 1 = 0–1 2 = 2–3 3 = 4–5 4 = 6 or more Question 1: Are the response categories ordered? SHOW ANSWER Question 2: Are there equal distances between the response categories? SHOW ANSWER 32 Health3 Health3 is based on the question, “In the past 12 months, how many times did you contact a medical practitioner to discuss your health?” The response categories of Health3 are 1=0 2=1 3=2 4=3 5=4 6=5 7=6 8=7 and so on, with each code value representing the specific number of times the respondent contacted a medical practitioner. Question 1: Are the response categories ordered? SHOW ANSWER Question 2: Are there equal distances between the response categories? SHOW ANSWER Transformation of Variable Level of Measurement What the above examples also illustrate is that it is often possible to “transform” a variable’s level of measurement from a higher level to a lower level. This procedure can be done simply by collapsing or grouping the response categories of the higher-level variable. For example, Health3 (interval-ratio) could be transformed into an ordinal variable by collapsing/grouping its values to correspond to the values of Health2, or it could even be transformed into a nominal variable by collapsing/grouping its categories to correspond to the values of Health1, as follows: Health3 Health2 Health1 Code Variable Code Variable Code Variable Value Value Value 1 0 1 0–1 1 No 2 1 2 Yes 3 2 2 2–3 4 3 5 4 3 4–5 6 5 7 6 4 6 or more 8 7 Note, however, that transformation is a downward-sloping, one-way street based on the simplification of variable response categories. So an interval- ratio variable can be transformed into an ordinal or a nominal variable, and an ordinal variable can be transformed into another ordinal or a nominal variable. However, an interval-ratio variable can never be transformed into another interval-ratio variable, an ordinal variable can never be transformed into an interval-ratio variable, and a nominal variable can never be transformed into an ordinal or an interval-ratio variable. 33 Book Title: eTextbook: Statistics: A Tool for Social Research and Data Analysis, Fifth Canadian Edition Chapter 1. Introduction Summary Summary 1. In the context of social research, the purpose of statistics is to organize, manipulate, and analyze data so that researchers can test their theories and answer their questions. Along with theory and methodology, statistics are a basic tool by which social scientists attempt to enhance their understanding of the social world. 2. There are two general classes of statistics. Descriptive statistics are used to summarize the distribution of a single variable and the relationships between two or more variables. Inferential statistics provide us with techniques by which we can generalize to populations from random samples. 3. Two basic guidelines for selecting statistical techniques were presented. Variables may be either discrete or continuous and may be measured at any of three different levels. At the nominal level, we can compare category sizes. At the ordinal level, categories and cases can be ranked with respect to one another. At the interval-ratio level, all mathematical operations are permitted. Interval-ratio-level variables can be either discrete or continuous. Variables at the nominal or ordinal level are almost always discrete. Glossary Continuous Data Data reduction Dependent variables Descriptive statistics Discrete Hypothesis Independent variables Inferential statistics Interval-ratio Level of measurement Measures of association Nominal Ordinal Population Research Response categories Sample Statistics Theory Variable Multimedia Resources 34 Visit the companion website for the fifth Canadian edition of Statistics: A Tool for Social Research and Data Analysis to access a wide range of student resources: www.cengage.com/healey5ce. Problems 1.1 In your own words, describe how statistics might be used to measure changes in divorce rates over time. Using the wheel of science (Figure 1.1) as a framework, explain how statistics can be used to link theories about family change with research. 1.2 Find a research article in any social science journal. Choose an article on a subject of interest to you, and don’t worry about being able to understand all of the statistics that are reported. a. How much of the article is devoted to statistics per se (as distinct from theory, ideas, discussion, and so on)? b. Is the research based on a sample from some population? How large is the sample? How were subjects or cases selected? Can the findings be generalized to some population? c. What variables are used? Which are independent and which are dependent? For each variable, determine the level of measurement and whether the variable is discrete or continuous. d. What statistical techniques are used? Try to follow the statistical analysis and see how much you can understand. Save the article and read it again after you finish this course to see if you understand more. 1.3 Distinguish between descriptive and inferential statistics. Describe a research situation that would use both types. 1.4 Below are some items from a public opinion survey. For each item, indicate the level of measurement and whether the variable is discrete or continuous. (HINT: Remember that only interval-ratio-level variables can be continuous.) a. What is your occupation? b. How many years of school have you completed? c. If you were asked to use one of these four names for your social class, which would you say you belonged in? upper middlez working lower d. What is your age? e. In what country were you born? f. What is your grade point average? g. What is your major? h. The only way to deal with the drug problem is to legalize all drugs. strongly agree agree undecided disagree strongly disagree i. What is your astrological sign? j. How many siblings do you have? 1.5 Below are brief descriptions of how researchers measured a variable. For each situation, determine the level of measurement of the variable and whether it is continuous or discrete. 35 a. Gender. Respondents were asked to select a category from the following list: female male other b. Honesty. Subjects were observed as they passed by a spot on campus where an apparently lost wallet was lying. The wallet contained money and complete identification. Subjects were classified into one of the following categories: returned the wallet with the money; returned the wallet but kept the money; did not return the wallet. c. Social class. Subjects were asked about their family situation when they were 16 years old. Was their family very well off compared to other families? about average? not so well off? d. Education. Subjects were asked how many years of schooling they and each parent had completed. e. Household size. Subjects were asked, “How many people reside in your household?” 1 person 2 people 3–4 people 5 or more people f. Student seating patterns in classrooms. On the first day of class, instructors noted where each student sat. Seating patterns were remeasured every two weeks until the end of the semester. Each student was classified as same seat as last measurement; adjacent seat; different seat, not adjacent; absent. g. Physicians per capita. The number of practising physicians was counted in each of 50 cities, and the researchers used population data to compute the number of physicians per capita. h. Physical attractiveness. A panel of 10 judges rated each of 50 photos of a sample of people for physical attractiveness on a scale from 0 to 20, with 20 being the highest score. i. Number of accidents. The number of traffic accidents for each of 20 busy intersections in a city was recorded. Also, each accident was rated as minor damage, no injuries; moderate damage, personal injury requiring hospitalization; severe damage and injury. 1.6 For each of the first 20 items in the General Social Survey (see Appendix G), indicate the level of measurement and whether the variable is continuous or discrete. 1.7 For each research situation summarized below, identify the level of measurement of all variables, and indicate whether they are discrete or continuous. Also, decide which statistical applications are being used: descriptive statistics (single variable), descriptive statistics (two or more variables), or inferential statistics. Remember that it is quite common for a given situation to require more than one type of application. a. The administration of your university is proposing a change in parking policy. You select a random sample of students and ask each student whether they favour or oppose the change. b. You ask everyone in your social research class to tell you (1) the highest grade they ever received in a math course and (2) their grade on a recent statistics test. You then compare the two sets of scores to find out whether there is any relationship. c. A relative is running for mayor and hires you (for a huge fee, incidentally) to question a sample of voters about their concerns in local politics. Specifically, they want a profile of the voters that will tell exactly what percentage belong to each political party; what percentage are male, female, or other gender; and what percentage favour or oppose the widening of the main street in town. d. Several years ago, a country reinstituted the death penalty for first-degree homicide. Supporters of capital punishment argued that this change would reduce the homicide rate. To investigate this claim, a researcher has gathered information on the number of homicides in the country for the two-year periods before and 36 after the change. e. A local automobile dealer is concerned about customer satisfaction. They want to mail a survey form to all customers for the past year and ask them if they are satisfied, very satisfied, or not satisfied with their purchases. 1.8 For each research situation below, identify the independent and dependent variables. Classify each in terms of level of measurement and whether or not the variable is discrete or continuous. a. A graduate student is studying sexual harassment on university campuses and asks 500 students if they have personally experienced any such incidents. Each student is asked to estimate the frequency of these incidents as either “often, sometimes, rarely, or never.” The researcher also gathers data on age, gender, and major to find out whether there is any connection between these variables and frequency of sexual harassment. b. A supervisor in the Solid Waste Management Division of a municipal government is attempting to assess two different methods of garbage collection. One area of the city is served by “traditional” trucks with two-person crews, and the rest of the city is served by new “high-tech” trucks with a single-person crew. The assessment measures include the exact number of complaints received from the two different areas over a six- month period, the amount of time per day required to service each area, and the cost per tonne of garbage collected. c. The adult bookstore near campus has been closed by the city council. Your social research class has decided to poll the student body and get their reactions and opinions. The class decides to ask each student if they support or oppose the closing of the store, how many times each one has visited the store, and if they agree or disagree that “pornography is a direct cause of sexual assaults.” The class also collects information on the gender, exact age, religious and political philosophy, and major of each student to see if the opinions are related to these characteristics. d. For a research project in a political science course, a student has collected information about the quality of life and the degree of political democracy in 50 nations. Specifically, the student used infant mortality rates to measure quality of life and the percentage of all adults who are permitted to vote in national elections as the measure of democratization. The student’s hypothesis is that quality of life is higher in more democratic nations. e. A highway engineer wonders if a planned increase in speed limit on a heavily travelled local avenue will result in any change in number of accidents. They plan to collect information on traffic volume, exact number of accidents, and number of fatalities for the six-month periods before and after the change. f. Students are planning a program to promote “safe sex” and awareness of a variety of other health concerns for university students. To measure the effectiveness of the program, they plan to give a survey measuring knowledge about these matters to a random sample of the student body before and after the program. g. Several provinces have drastically cut their budgets for mental health care. Will this increase the number of people who are homeless in these provinces? A researcher contacts a number of agencies serving people who are homeless in each province and develops an estimate of the size of the population before and after the cuts. h. Does tolerance for diversity vary by race, ethnicity, or gender? A cross section of ethnic, racial, and gender groups was sampled. Respondents were asked about their interests in and appreciation of social and culture groups other than their own. 37 You Are the Researcher Introduction to the Canadian General Social Survey, Canadian Community Health Survey, and SPSS Two types of exercises are located at the end of each chapter of this textbook. While the “Problems” exercises are based on fictitious data for the most part and are designed to be solved with a simple hand calculator, the “You Are the Researcher” computer-based exercises provide a more realistic experience and use actual social science data from two leading Canadian social surveys, the Canadian Community Health Survey (CCHS) and the Canadian General Social Survey (GSS). The GSS is an annual public opinion poll, administered by Statistics Canada, of a representative sample of Canadians. It has been conducted since 1985 and explores a broad range of social issues. Each year the survey collects information on specific aspects of society relevant to social policy. Since survey topics are often repeated every few years, GSS data can also be used to track trends and changes in these characteristics over time. The GSS has proven to be a very valuable resource for testing theory, for learning more about Canadian society, and for informing public debates. The 2018 cycle of the GSS is used in this textbook. It collected information on charitable giving and volunteering in Canada. Using this data set, you can describe reasons for volunteering, main volunteer activities, quality of volunteer experience, financial giving to charities, and reasons for giving. You can further examine whether variables such as gender, age, and education are associated with giving and volunteering in Canada. There are two versions of the GSS data set. The first version, named 2018_GSS_Full.sav, contains the original random sample. The second (2018_GSS_Shortened.sav) is a shortened version with 1,500 cases randomly selected from the original random sample. The latter was created to simplify computer output. The CCHS provides information on the determinants of health, health status, and use of health care services for a random sample of Canadians. The CCHS is conducted annually by Statistics Canada. The 2018 CCHS is provided here. Using CCHS data, you can describe many aspects of the health of Canadians and the factors that determine it, such as health care access, alcohol and tobacco use, stress, and socioeconomic status. The 2018 CCHS contains dozens of variables for a sample of thousands of Canadians. As with the GSS, we provide both the complete version of the CCHS (2018_CCHS_Full.sav), containing the original random sample, and a shortened version (2018_CCHS_Shortened.sav), with 1,500 randomly selected cases. The four databases (2018_GSS_Shortened.sav, 2018_CCHS_Shortened.sav, 2018_GSS_Full.sav, and 2018_CCHS_Full.sav) and the user’s guides for the 2018 GSS (2018_GSS_Guide.pdf) and 2018 CCHS (2018_CCHS_Guide.pdf) can be downloaded from the student companion website at www.cengage.com/healey5ce and the instructor website at login.cengage.com. The user’s guides provide a detailed description of each survey; however, for quick reference, a list of the variables in each database is shown in Appendix G. Two additional surveys, the 2017 Aboriginal Peoples Survey (2017_APS_Full.sav) and the 2016 Canadian Census (2016_Census_Full.sav), are also supplied with the textbook. While these data are not used in the end-of- 38 chapter sections, they provide further opportunity to practise statistical and computing skills on real-life data. One of the challenges with using real-life data is that they very often contain too many cases to be analyzed with just a simple calculator. Computers and statistical software packages are almost always needed to analyze the data. A statistical software package is a set of computer programs for data analysis. The advantage of these packages is that, since the programs are already written, you can capitalize on the power of the computer with minimal computer literacy and virtually no programming experience. This textbook uses IBM SPSS Statistics for Windows, referred to in this book simply as SPSS. Version 27 of SPSS is used, though all recent versions of SPSS are compatible with the textbook. In the “You Are the Researcher” section at the end of each chapter, we will explain how to use SPSS in various ways to manipulate and analyze the GSS or CCHS data, and we will illustrate and interpret the results. Be sure to read Appendix F, which gives a general introduction to SPSS, before attempting any data analysis. A manual for Stata, another commonly used statistical computer program, is also available for this textbook. The manual mirrors the “You Are the Researcher” sections in the textbook but with Stata instruction and data. It can be downloaded from www.cengage.com/healey5ce and is accompanied by Stata versions of the 2018 CCHS and GSS data sets. 39 Book Title: eTextbook: Statistics: A Tool for Social Research and Data Analysis, Fifth Canadian Edition Chapter 2. Basic Descriptive Statistics: Percentages, Ratios and Rates, Tables, Charts, and Graphs 2.1. Percentages and Proportions 2.1. Percentages and Proportions Consider the following statement: “Of the 113 survey respondents, 56 are female.” While there is nothing wrong with this statement, the same fact could have been more clearly conveyed if it had been reported as a percentage: “About 50% of survey respondents are female.” Percentages and proportions supply a frame of reference for reporting research results in the sense that they standardize the raw data: percentages to the base 100 and proportions to the base 1.00. The mathematical definitions of proportion and percentage are Formula 2.1 f Proportion (p) = n Formula 2.2 f Percentage(%) = ( ) × 100% n where f = frequency, or the number of cases in any category n = the number of cases in all categories To illustrate the computation of percentages, consider the data presented in Table 2.1. How can we find the percentage of males in the sample? Note that 42 there are 53 males (f = 53) and a total of 113 cases in all (n = 113). So, f 53 Percentage % = ( ) × 100% = ( ) × 100% = 0.4690 × 100% = 4 n 113 Table 2.1 Gender of Respondents Using the same procedures, we can find the percentage of females: f 56 Percentage % = ( ) × 100% = ( ) × 100% = 0.4956 × 100% = 4 n 113 and the percentage of other genders: f 4 Percentage % = ( ) × 100% = ( ) × 100% = 0.0354 × 100% = 3 n 113 All three results could have been expressed as proportions. For example, the proportion of females in Table 2.1 is 0.4956 : f 56 Proportion(p) = = = 0.4956 n 113 Percentages and proportions are easier to read and comprehend than frequencies. This advantage is particularly obvious when attempting to compare groups of different sizes. For example, Table 2.2 provides data on major fields of study from the 2019 Postsecondary Student Information System, an annual Statistics Canada survey of postsecondary students and institutions. Gender comparisons, however, are difficult because the total numbers of male (243,405) and female (319,935) students are very different. It is not evident which gender has the higher relative number of, say, majors in business, management, and public administration. Calculating percentages eliminates the difference in size of the two groups by standardizing both distributions to the base of 100. The same data are presented in percentages 43 in Table 2.3, making it easier to identify both differences and similarities 44 between the genders. We now see that a higher percentage of males (25.57%) than females (23.33%) study business, management, and public administration at Canadian postsecondary institutions, even though the absolute number of males (62,229) is considerably smaller than that of females (74,640). How would you describe the differences in the other major fields? (For practice in computing and interpreting percentages and proportions, see Problems 2.1 and 2.2.) Table 2.2 Major Field of Study for Female and Male Postsecondary Students Source: Statistics Canada. (2019). Postsecondary Student Information System. Table 2.3 Major Field of Study for Female and Male Postsecondary Students (Based on Table 2.2) Applying Statistics 2.1. Communicating with Statistics Not long ago, in a large social service agency, the following conversation took place between the executive director of the agency and a supervisor of one of the divisions: Executive director: Well, I don’t want to seem abrupt, but I’ve only got a few minutes. Tell me, as briefly as you can, about this staffing problem you claim to be having. Supervisor: Unfortunately, I just don’t have enough people to handle our workload. Of the 177 full-time employees of the agency, only 50 are in my division. Yet 6,231 of the 16,722 cases handled by the agency last year were handled by my division. Executive director (smothering a yawn): Very interesting. I’ll certainly get back to you on this matter. How could the supervisor have presented the case more effectively? Because the supervisor wants to compare two sets of numbers (the supervisor’s staff versus the total staff and the workload of the supervisor’s division versus the total workload of the agency), proportions or percentages would be a more forceful way of presenting results. What if the supervisor had said, “Just over 28% of the staff is assigned to my division, but we handle more than 37% of the total workload of the agency”? Is this a clearer message? The first percentage is found by f 50 %=( ) × 100% = × 100% n 177 = 0.2825 × 100% = 28.25% and the second percentage is found by f 6,231 %=( ) × 100% = ( ) × 100% n 16,722 = 0.3726 × 100% = 37.26% Here are some further guidelines on the use of percentages and proportions: 1. When working with a small number of cases (say, fewer than 20 ), it is usually preferable to report the actual frequencies rather than percentages or proportions. With a small number of cases, the percentages can change drastically with relatively minor changes in the data. For example, if you begin with a data set that includes 10 engineers and 10 sociologists (i.e., 50% of each of the two professions) and then add another sociologist, the percentage distributions will change noticeably to 52.38% sociologists and 47.62% engineers. Of course, as the number of observations increases, each additional case will have a smaller impact. If we started with 250 engineers and 250 sociologists and then added one more sociologist, the percentage of sociologists would change by only a tenth of a percent (from 50% to 50.1% ). 2. The first guideline leads to a follow-up, general best practice guideline about reporting results. Always report the number of observations along with proportions and percentages. This permits the reader to judge the adequacy of the sample size and, conversely, helps prevent the researcher from lying with statistics. Statements like “two out of three 45 people questioned prefer courses in statistics to any other course” might impress you, but the claim would lose its gloss if you learned that only three people were tested. You should be extremely suspicious of reports that fail to state the number of cases that were tested. 3. Percentages and proportions can be calculated for variables at the ordinal and nominal levels of measurement, even though they require division. This is not a violation of the level-of-measurement guideline (see Table 1.2). Percentages and proportions do not require the division of the scores of the variable (as would be the case in computing the average score on a test, for example) but rather the number of cases in a particular category (f) of the variable by the total number of cases in the sample (n). When we make a statement like “ 43% of the sample is female,” we are merely expressing the relative size of a category (female) of the variable (gender) in a convenient way. One Step at a Time Finding Percentages and Proportions 1: Determine the values for f (number of cases in a category) and n (number of cases in all categories). Remember that f will be the number of cases in a specific category and n will be the number of cases in all categories and that f will be smaller than n, except when the category and the entire group are the same. Therefore, proportions cannot exceed 1.00 , and percentages cannot exceed 100.00%. 2: For a proportion, divide f by n. 3: For a percentage, multiply the value you calculated in step 2 by 100%. Book Title: eTextbook: Statistics: A Tool for Social Research and Data Analysis, Fifth Canadian Edition 2.2. Ratios and Rates Ratios Ratios Ratios are especially useful for comparing categories of a variable in terms of relative frequency. Instead of standardizing the distribution of the variable to the base 100 or 1.00 , as we did in computing percentages and proportions, we determine ratios by dividing the frequency of one category by the frequency of another. Mathematically, a ratio can be defined as Formula 2.3 f1 Ratio = f2 where f1 = the number of cases in the first category f2 = the number of cases in the second category 46 To illustrate the use of ratios, we will use actual information from the 2019 Canadian Election Study, which is an inter-university project that regularly conducts a survey of Canadian voters on a variety of political issues. One of the survey questions asked, “Thinking about government spending, should the federal government spend more or about the same as now or less on crime and justice.” Of those polled, 1,442 people said that the federal government should spend more, while 1,890 said it should spend about the same as now or less. What is the relative size of these two groups? To find the ratio of those who think the government should spend more (f1) to those who think the government should spend about the same as now or less (f2) , divide 1,442 by 1,890 : f1 1,442 Ratio = = = 0.76 f2 1,890 The resultant ratio is 0.76 , which means that for every Canadian who thinks the government should spend about the same as now or less on crime and justice, there are 0.76 Canadians who think the government should spend more. Ratios can be very economical ways of expressing the relative predominance of two categories. In our example, it is obvious from the raw data that Canadians who think the government should spend more on crime and justice are outnumbered by Canadians who think the government should spend about the same as now or less. Percentages or proportions could have been used to summarize the overall distribution (e.g., “ 43.28% of Canadians think more should be spent on crime and justice, compared to 56.72% who think spending should remain the same as now or less”). In contrast to these other 47 methods, ratios express the relative size of the categories: they tell us exactly how much one category outnumbers (or is outnumbered by) the other. Applying Statistics 2.2. Ratios Indigenous Peoples are one of the fastest growing populations in Canada. One way to express this growth is to calculate the ratio of Indigenous (f1) to non-Indigenous (f2) persons at different points in time. For example, there were 1,172,785 Indigenous and 30,068,240 non-Indigenous persons in Canada in 2006, or a ratio of f1 1,172,785 = = 0.039 f2 30,068,240 By 2016, census data showed that there were 1,673,785 Indigenous and 32,786,285 non-Indigenous persons in the population. The ratio is f1 1,673,785 = = 0.051 f2 32,786,285 So, for every non-Indigenous person, there were 0.039 and 0.051 Indigenous persons in the population in 2006 and 2016 respectively. To eliminate the decimal points, we can multiply these ratios by 100 and report the values as 3.9 and 5.1 —for every 100 non-Indigenous persons in the population, the number of Indigenous persons grew from 3.9 to 5.1 over this 10-year period. Source: Data from Statistics Canada, 2006 and 2016 Census of Population. Ratios are often multiplied by some power of 10 to eliminate decimal points. For example, the ratio computed above might be multiplied by 100 and reported as 76 instead of 0.76. This would mean that, for every 100 Canadians who think the government should spend about the same as now or less on crime and justice, there are 76 Canadians who think more should be spent. To ensure clarity, the comparison units for the ratio are often expressed as well. Based on a unit of ones, the ratio of Canadians who think more should be spent to Canadians who think about the same as now or less should be spent would be expressed as 0.76:1. Based on hundreds, the same statistic might be expressed as 76:100. (For practice in computing and interpreting ratios, see Problems 2.1 and 2.2.) Book Title: eTextbook: Statistics: A Tool for Social Research and Data Analysis, Fifth Canadian Edition 2.2. Ratios and Rates Rates Rates Rates provide still another way of summarizing the distribution of a single variable. Rates are defined as the number of actual occurrences of some phenomenon divided by the number of possible occurrences per some unit of time. Formula 2.4 factual Rate = fpossible where factual = the number of actual occurrences of a phenomenon fpossible = the number of possible occurrences of the phenomenon Rates are usually multiplied by some power of 10 to eliminate decimal points. For example, the crude death rate for a population is defined as the number of 48 deaths in that population (actual occurrences) divided by the number of people in the population (possible occurrences) per year (the term “crude” is used because it is not adjusted for any underlying characteristics of the population such as the age or sex distribution). This quantity is then multiplied by 1,000. The formula for the crude death rate can be expressed as Number of deaths Crude death rate = × 1,000 Total population Applying Statistics 2.3. Rates In 2020, there were 374,885 births in Canada, within a population of 38,005,238. In 1972, when the population of Canada was only 22,218,463 , there were 351,256 births. Is the birth rate rising or falling? Although this question can be answered from the preceding information, the trend in birth rates is much more obvious if we compute birth rates for both years. Like crude death rates, crude birth rates are usually multiplied by 1,000 to eliminate decimal points. For 1972: 351,256 Crude birth rate = × 1,000 = 15.81 22,218,463 In 1972, there were 15.81 births for every 1,000 people in Canada. For 2020: 374,885 Crude birth rate = × 1,000 = 9.86 38,005,238 In 2020, there were 9.86 births for every 1,000 people in Canada. With the help of these statistics, the decline in the birth rate is clearly expressed. Source: Statistics Canada. Table 17-10-0008-0 Estimates of the components of demographic growth, annual. One Step at a Time Finding Ratios and Rates To Find Ratios 1: Determine the values for f1 and f2. The value for f1 is the number of cases in the first category, and the value for f2 is the number of cases in the second category. 2: Divide the value of f1 by the value of f2. 3: You may multiply the value you