Power & Sample Size Calculations PDF
Document Details
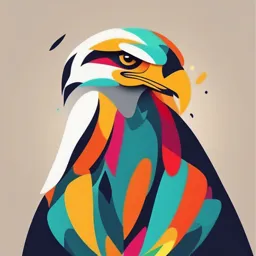
Uploaded by WorldFamousZombie1045
UCL
Tags
Summary
This document discusses power and sample size calculations in research, covering different scenarios such as continuous and binary outcomes. It explains crucial concepts like alpha, beta, and standard deviations, providing formulas and examples for various situations, including loss-to-follow-up considerations. It's useful for researchers designing studies.
Full Transcript
**[Core Principles of Mental Health Research]** **[Power & Sample Size]** [Alpha Criterion & Hypothesis Test:] - Red lines at 1.96 SD above/below mean - Alpha =.05 assumes 5% chance of a type I error - Centred on 0 = null is true [Alternative Hypothesis:] - Purple line represent sampl...
**[Core Principles of Mental Health Research]** **[Power & Sample Size]** [Alpha Criterion & Hypothesis Test:] - Red lines at 1.96 SD above/below mean - Alpha =.05 assumes 5% chance of a type I error - Centred on 0 = null is true [Alternative Hypothesis:] - Purple line represent sampling distribution for mean difference in null hypothesis is true - For pragmatic reasons we assume null is true (but alternative could be true) - True difference between groups is 6 points therefore alternative hypothesis centred on 6 - These curves represent mutually exclusive worlds [Power:] - Pink area represents power of test - Green area (was type I area, contributes to power now) - Yellow area is type II error (50% probability of making a correct decision/50% chance we make a type 2 error) [Factors which affect power:] - Level of statistical significance (alpha) -- larger alpha = increased power BUT increases probability of making a type I error - Effect size to detect (difference between groups) -- increased power BUT can only detect large intervention effects - Standard deviation (SD) of the outcome -- depends on sample size - Sample size - How could we increase power? [Factors which determine sample size:] - Clinically important effect - Smallest difference between groups which is necessary to detect - Usually determined from prior studies or in consultation with clinicians or researchers - Standard deviation of the outcome - Usually based on previous literature or a pilot study - Often assumed to be the same in both groups - Power to detect the specified difference if a true difference exists - Usually specified as 80 or 90% - Level of statistical significance - Usually specified as 5% [Continuous Outcomes -- Comparison of Two Means:] Power is a probability -- sample size calculations calculates instead of power - The required sample size per group is: Where: - [**μ**~**2**~**−μ**~**1**~ ]{.math.inline}is the true difference between the means in the two groups - [**σ**]{.math.inline} is the standard deviation of the outcome - [**α**]{.math.inline} is the significance level - [**1** **−** **β**]{.math.inline} is the statistical power - [**z**]{.math.inline} is the Z score from a normal distribution [Binary Outcomes -- Comparison of two proportions ] - The requires sample size per group is: Where: - [**p**~**1**~ ]{.math.inline}is the true proportion in the control group - [**p**~**2**~]{.math.inline} is the true proportion in the treatment group - [**α**]{.math.inline} is the significance level - [**1** **−** **β**]{.math.inline} is the statistical power - [**z**]{.math.inline} is the Z score from a normal distribution [Similarities between formulae:] - General principle [Sample Size -- information required:] - What is the primary outcome measure? - How will the data be analysed? - What results are expected in the control group? - How small a treatment difference needs to be detected? - With what degree of certainty? [More Complicated Scenarios:] - Loss to follow up - Unequal treatment group sizes - Adjustment for baseline - Very common with continuous outcomes - Specify correlation between baseline and follow up - Usually reduces the required sample size - Hierarchal data structures: - Examples: multicentre trials, patients within GP practices, patients treated by the same therapist - Specify intraclass correlation (ICC) for patients within clusters - Usually increases the required sample size - Time to event analysis [Loss to follow up:] - In the CBT for depression in adults with cancer study, it is expected that 25% of patients will be lost to follow up - The required sample size calculated for the study was 126 - If 25% drop out, that only leave 75%, or 94 patients whose data can be analysed - The power to detect the specified clinically important difference will be less than 80% specified Problem: loss to follow up reduces the power of a trial Solution: increase initial number of patients recruited to allow sufficient power after loss to follow up [Adjustment for loss to follow up:] - If your sample size calculation says you need 100 participants, how many extra participants do you need to recruit to account for 20% loss to follow up? - Increase by 20% ??? - 120% of 100 (or 1.2 x 100) = 120 - How many of 120 participants will remain after 20% attrition? - 100% -- 20% = 80% (or 1 -- 0.2 = 0.8) - 80% of 120 (or 0.8 x 120) = ??? - Increase by 25% ??? - 125% of 100 (or 1.25 x 100) = 125 - How many of 125 participants will remain after 20% attrition? - 80% of 125 (or 0.80 x 125) = ??? - If proportion lost is Q, multiply the required sample size (calculated using the relevant formula) by 1/(1-Q) - Sample size = 100, attrition = 20% 2n x 1/(1-Q)\ = 100 x 1/(1-0.2)\ = 100 / 0.80 = 100 / 4/5\ = 100 x 1.25 = 100 x 5/4\ = 125 - Sample size = 126, attrition = 25% 2n x 1/(1-Q)\ = 126 x 1/(1-0.25)\ = 126 / 0.75 = 126 / 3/4\ = 126 x 1.33 = 126 x 4/3\ = 168 - If loss to follow up only expected in one study arm, apply correction to one arm only [When P\>0.05:] - There are a number of possible reasons, including - True difference between groups is smaller than specified in the sample size calculation - Standard deviation is greater than specified in the sample size calculation - There is no true difference between groups in the population - There is a true difference between groups, but this result is a Type II error, for example one of the 10% of studies expected to produce a false negative result when power is specified as 90% - It's not possible to distinguish between these reasons [Avoid Post-Hoc Power Calculations:] - Some journals request post hoc sample size calculations - Probabilities are not meaningful after the event - Before you flip a coin, the probability of getting "heads" is 0.5 - After you have flipped a coin the result is not in doubt -- it is either 0 (if you got "tails") or 1 (if you got "heads") - Post hoc power calculations are not informative - They cannot help distinguish between possible reasons for the expected result not being found - Post hoc power for a trial with p\>0.05 will always be low - **AVOID** post hoc power calculations - A priori: perform and report power/sample size calculations - Post hoc: use 95% confidence intervals to shed light on results