HSERV 533 Midterm Review PDF
Document Details
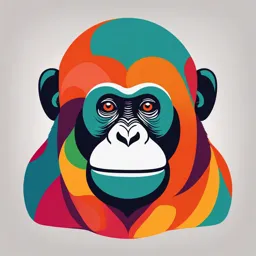
Uploaded by KnowledgeablePiccolo
Tags
Summary
This document is a midterm review for a public health course (HSERV 533). It covers various concepts including study designs, outbreak investigations, and measures of disease occurrence. The topics discussed are designed to prepare students for a midterm exam, and include relevant case studies and examples.
Full Transcript
Win 24: HSERV 533 Midterm Review Review Topics Study designs Outbreak investigations Measures of morbidity/ Measures of disease occurrence Screening Types of variables Sample statistics and describing distributions Hypothesis testing Measures of association & Tests of statistical significance Study...
Win 24: HSERV 533 Midterm Review Review Topics Study designs Outbreak investigations Measures of morbidity/ Measures of disease occurrence Screening Types of variables Sample statistics and describing distributions Hypothesis testing Measures of association & Tests of statistical significance Study Designs How we obtain our data and the structure of our studies determine the types of inferences we can make from our studies Grimes DA and Schulz KF (2002) An overview of population health research. Lancet 359:57-61. Study Designs Comparison of Designs Cross-sectional Data collected on both exposure and outcome status at the same time Can be used to estimate prevalence and identify risk factors needing urgent attention Can be difficult to establish temporal relationship (I.e. hard to determine if exposure came before the outcome) Cannot estimate risk ----why not? Case-control People with a particular condition or disease (cases) are selected and compared to people without the particular condition or disease (controls) We consider those with and without disease (the outcome) and then look back at exposures Useful for rare outcomes Cannot directly calculate risk----why not? Cohort Compares incidence of disease in the exposed group and unexposed group We consider groups that are exposed and unexposed and then look to see who gets the outcome Know that exposure comes before the outcome (can establish a temporal relationship) Useful for rare exposures CAN estimate risk The exposure/independent variable is NOT randomly assigned in any of the above designs Types of Study Designs- Organized by strength of evidence each type provides-> Stronger evidence as you move up the pyramid So far, we’ve focused on case series, cohort, case-control, and crosssectional studies*. More to come! Case 1: case-control Case 3: cross-sectional Ecologic, Cross-Sectional Case 1: case series *Note: Focus on the strengths (and application) and limitations of the designs we’ve covered Outbreak Investigations Outbreak Investigations Steps in an investigation—See case #1 Can involve different study designs depending on the stage of the investigation Key components (hint hint) Case definitions Confirmed vs suspected cases Epidemic curves (type of histogram) Includes incident cases over time Shape (distribution) can help us understand trends and give clues about the source (e.g. point, propagated, intermittent) Measures of morbidity/ Measures of disease occurrence Format and formulas for quantifying the presence of a condition of interest Measures of morbidity/ Measures of disease occurrence Prevalence Point Prevalence Period Prevalence Incidence Cumulative Incidence Incidence Rate (Incidence Density) Note: Know how to calculate these measures! Hint: think about what goes into the numerator and denominator for each Prevalence Prevalence is the measure of persons in a population who have a particular disease at a specified point in time or over a specified period of time. cases of a disease present in the population during a specified time period Prevalence = x 10n number of persons in the population during the specified time Point vs. Period Prevalence Point prevalence The proportion of people who have a disease at a point in time. Period prevalence The proportion of people who have a disease at any time during a certain period of time. Incidence Incidence is the number of new cases of disease in a population at risk over a period of time Measure of risk Two types of incidence measures: Cumulative Incidence Incidence Rate (aka Incidence Density) Cumulative Incidence (Incidence Proportion) Cumulative Incidence is the proportion of a population that becomes diseased over a specified period of time. Often reported as a % or standardized (usually a multiple of 10) Cumulative Incidence # of new cases of disease occurring during a specified time period = # of persons at risk of developing the disease during that time period Incidence Rate (aka Incidence Density) Incidence rate is the number of new cases of a disease that occur in a population at risk per person-time of observation. # of new cases of disease Incidence Rate = person-time of observation in the population at risk of developing the disease Relation between Incidence and Prevalence The Bathtub Analogy Cure Incidence Prevalence Death Prevalence = Incidence x Duration of Disease P=IxD Screening PH tool to decrease morbidity and mortality Screening Screening: using tests to detect the likely presence of diseases or conditions before illness or symptoms Goal-> reduce morbidity and mortality Does NOT affect the current state of disease Consider true positives, true negatives, false positives, and false negatives (with respect to “truth” or “gold standard”) Key measures in screening: Sensitivity Specificity Positive predictive value Negative predictive value Comparison of Results of a Screening Test with Actual Disease Status Population (“Truth”) or “Gold Standard” Test Screening Test Result Positive “Likely” Negative “Unlikely” Diseased Not Diseased Have disease and have positive test result = true positive (TP) No disease but have positive test result = false positive (FP) Have disease but have negative test results = fal se negative (FN) No disease and have negative test result = true negative (TN) Diseased= TP+FN Not Diseased= FP+TN 19 Sensitivity The ability of a test to identify correctly those who have the disease. people with the disease detected by the screening test Sensitivity = x 100 total number of people tested with the disease TP Sensitivity = x 100 TP + FN 20 Specificity The ability of a test to identify correctly those who do not have the disease. people without the disease who are negative by the screening test Specificity = x 100 total number of people tested without the disease TN Specificity = x 100 TN + FP 21 Positive Predictive Value Proportion of positive tests that are truly positive. people with the disease tested positive by the screening test PPV = x 100 total number of people tested positive by the screening test TP PPV = x 100 TP + FP 22 Determinants of Positive Predictive Value Prevalence of disease in the population tested. Higher prevalence in the screened population leads to a marked increase in the positive predictive value using the same test. The usefulness of a test changes as the clinical situation changes. 23 Negative Predictive Value Proportion of negative tests that are truly negative. NPV = people without the disease tested negative by the screening test x 100 total number of people tested negative by the screening test TN NPV = x 100 TN + FN 24 Putting it all together example Types of Variables Types of Variables Categorical- Three types of categorical variables Binary (also known as dichotomous): variable with only two mutually exclusive options Eg. Yes/No, Diseased/Not Diseased, Dead/Alive, UW student/ Non-UW student Ordinal: ordered or “ranked” variable; the difference between values is not always the same E.g. Placement in a marathon race -> The time difference between the first place and second place may be different than the time difference between the second place and third place Nominal: values that are not defined by order; labels or names E.g. Racial group, favorite color, birth state Continuous- variable that can take on an unlimited number of values between the highest and lowest possible values; a one-unit difference means the same no matter where you are on the scale E.g. weight in grams, time it takes to run a mile, systolic/diastolic blood pressure Sample Statistics and Describing Distributions Characterizing our samples and gaining insight from known properties of distributions Sample Statistics Sample statistics: Describing a distribution Mean: is the average of the numbers It is the sum of all the values, divided by the number of values Median: is the middle value of a sorted list of numbers. In other words, it is the value below which, 50% of the values lie To find the Median, place the numbers in value order and find the middle. Mode: is the value that appears the most often To find the Mode, put the numbers in order, then count how many of each number. A number that appears most often is the mode Note: If you just saw the shape a distribution, what could you say about the mean, median, mode, and skew? Sample statistics…continued Inter-Quartile Range: the middle 50% of a sorted list of numbers This is the range between the top 25 percentile and the bottom 25th percentile It tells us where ‘most’ of the data lies Box-and-Whiskers plot tell us: Skew: median pulled towards the top (negative skew) or the bottom values (positive skew) Outliers: extreme high/low values in our dataset Quartiles http://flowingdata.com/2008/02/15/how-to-read-and-use-a-box-and-whisker-plot/ A Box and Whiskers plot shows the distribution of an entire dataset at a glance! Frequently used Distributions* Binomial- distribution of a binary random variable The experiment consists of n (fixed) identical trials Only two possible outcomes on each trial The probability of success (A), denoted by p, remains the same from trial to trial The probability of failure (B), denoted by q, q = 1- p Normal- potential distribution of a continuous variable Frequently called the “Gaussian distribution” or bellshape curve The distribution is symmetrical about its mean and bell shaped The mean, the median and mode are almost equal. It is unimodal Figure shown at right *There are other types of distributions, but we are focusing on these for the course Sample statistics…continued Standard Deviation: is a measure of how spread out numbers are. SD is a characteristics of your sample/ data set You calculate the difference between each value in your sample, and the mean, sum up the squares of all those differences, divide that by the sample size, and square root that entire number Standard Error: is a measure of how precise an estimate our sample really gives us. SE for continuous variables: SE = SD / √n SE for binary variables: SE = √[ p * (1 – p) / n ] we’d expect 95% of the sample means would fall between ± two standard errors from the underlying population mean SD SD/SE Takeaways SD= measure of dispersion-> how spaced out your data values are SE= measure of precision -> how precise an estimate our sample gives us (in relationship to the “true” population parameter) Standard errors of the mean follow a normal distribution if your sample size is large enough (about 30, but you don’t need worry about why we use this number) SE decreases as sample size increases More advanced note: This is why small differences can be statistically significant in large datasets We care about SEs because we use them to calculate 95% CIs (or any confidence intervals, 95% is most common) We use confidence intervals (or p-values) to make decisions about our hypotheses and what conclusions we can draw from our data Confidence intervals Confidence intervals: measure of uncertainty of an estimate Confidence intervals use the (sample statistic), the appropriate z or t value depending on your confidence level, and your standard error to form a range of values around your sample statistic Eg. 95% CI for a sample mean: Mean - (1.96*SE), Mean + (1.96*SE) Confidence intervals continued The most commonly used confidence levels are 95% (aka 95% CI) *For this course, focus more on the use and interpretation of CIs, rather than the calculations (your statistic software will do this for you) Interpretations for a 95% CI: If we repeated our study 100 times, the interval constructed would include the true mean (μ)/proportion 95 times OR We are 95% confident that the population parameter (true value of the population) is contained within the constructed interval Hypothesis Testing How do we know if our results are due to true differences or by chance? Hypothesis testing Null hypothesis (Ho) Formalized skepticism: No difference, no effect, no association, equal/same Ho is true until the evidence against it becomes overwhelming Statistics has ways of testing Ho Alternative hypothesis (Ha) Researchers' ideas It is the proposition you are trying to prove Complementary to each other Hypothesis testing-Examples from Case 1 and 3 Case 1 Ho: Well water at the fair was not the risk factor/source of diarrheal disease. Ha: Well water at the fair was the risk factor/source of diarrheal disease. Case 3 Ho: Starting Right has no effect on the proportion of low birth weight Ha: Starting Right has an effect on the proportion of low birth weight Case 3 Ho: Mean birthweight in counties implemented starting right is equal with the mean weight birthweight in non-starting right implemented counties. Ha: Mean birthweight in counties implemented starting right is not equal to the mean weight birthweight in non-starting right implemented counties. Significant level vs P-value Significant level (α) (1-confidence level) For 95% confidence level, α = 1-0.95= 0.05 = 5% Decided before the start of the study Ztabulated, Z1-α/2 = 1.96 , Z1-α = 1.64 Used to decide significance Rejection region Rejection region To be or not to be…statistically significant We can determine statistical significance in two ways: 1. Compare our p-value to our alpha level Typically we use an alpha level of 0.05 or 5% If our p-value is LESS THAN alpha (0.05) -> We reject the null hypothesis If our p-value is GREATER THAN or equal to alpha (0.05) -> We fail to reject the null hypothesis 2. Determine if our confidence interval includes our “null value” If our CI does NOT include the null value -> We reject the null hypothesis If our CI does include the null value -> We fail to reject the null The null value will differ depending on your measure of association and how you have constructed your hypotheses Type I and Type II errors Type I-often equated with “false positives” – happen in hypothesis testing when the null hypothesis is true but rejected Type II- sometimes known as “false negatives”- happen when the null hypothesis is false and you subsequently fail to reject it Graphical Representation of Type I & II Errors Measures of association How do we know if variables are related to each other? In causal framework, does the exposure cause the outcome? Measures of Association Relative Risk Note: Know how to calculate this based on a 2x2 table! Odds Ratio Note: Know how to calculate this based on a 2x2 table! Proportions (difference in proportions) Means (difference in means) Correlation Tests of statistical significance How we determine if our measures of association is statistically significant Four Questions 1. Is there a difference between groups (an association between the exposure and outcome)? If so, 2. In what direction is it? 3. How big is it? 4. Is it statistically significant? Note: Use the measure of association to answer the first 3 Qs, and then test for statistical significance Relative Risk (Risk Ratio): Formula using 2x2 table Numerator Risk of disease in exposed Denominator Risk of disease in unexposed = a a+b c c+d Interpretation of the Relative Risk In a Cohort Study RR > 1 = Having the exposure is associated with a higher incidence of disease RR = 1 = No association, or no difference in incidence of disease between the two exposure groups (null value) RR < 1 = Having the exposure is associated with a lower incidence of disease Example of Calculating a Relative Risk Risk of Disease in the smokers Relative = Risk of Disease in the non-smokers Risk (RR) 84 3000 = Know how to interpret your measures! RR = 87 5000 1.6 28 per 1,000 = 17.4 per 1,000 “Smokers have a 1.6 times greater risk of developing CHD than non-smokers” Is the inverse true? Do non-smokers have 1.6 times less risk (or 60% decreased risk) of CHD than smokers? Hint: NO. Odds Ratio: The 2x2 Table for a Case-Control Study Cases Controls Exposed Number of cases exposed Number of controls exposed Total Exposed Unexposed Number of cases not exposed Number of controls not exposed Total Unexposed Total Cases Total Controls Estimating Risk in a Case-Control Study-> Calculating an Odds Ratio Cases Controls Exposed A B Not Exposed C D A C B Odds of exposure in the controls = D Odds of exposure in the cases = = “Odds a case is exposed” = “Odds a control is exposed” Estimating Risk in a Case-Control Study-> Odds Ratio Odds a case is exposed Odds Ratio (OR) = Odds a control is exposed Odds Ratio (OR) = A C B D AD = BC Example of Calculating an Odds Ratio Odds a case was exposed Odds Ratio (OR) = Odds a control was exposed Know how to interpret your measures! Odds Ratio = 26 1 53 87 = 42.7 “Children who had Reye’s Syndrome were 42.7 times more likely to have used salicylates compared to children who did not have Reye’s Syndrome.” Interpreting Ratios (either RRs or ORs) 10 3.0 weak positive association 1.1 10.9 0.33 0 increasingly strong positive association, possible “risk” factor no association weak negative association increasingly strong negative association, possible “protective” factor Interpretation of Relative Risk Infinity! RR 0 Interpretation Testing the statistical significance of RRs and ORs Relative Risk and Odds Ratios use a chi-square test to test for statistical significance You do NOT need to know how to do this. Your trusty software package will do this for you and will give you a 95%CI and p-value for these measures DO understand that these measures have a “null value” of 1. If your confidence interval for an RR or OR contains or spans the value of 1, the measure is not considered to be statistically significant You may also compare the p-value to your alpha level Typically we look to see if the p-value is LESS THAN 0.05 (Other)Measures of Association with Binary Outcomes/Dependent Variables You can use RRs or ORs (depending on your study type) or… Proportion One-sample z test Independent variable is a constant Tests whether the sample proportion equals the specified proportion Difference in Proportions Two-sample z test Independent variable is a binary variable Tests whether two sample proportions are equal to each other (could also be framed as testing whether the difference between two proportions is equal to zero). *These are equivalent statements Note: RRs and ORs are relative measures of association (think division); and a difference in proportions is, well, a difference (think subtraction) Measures of Association with Continuous Outcomes/Dependent Variables Mean One-sample t test Independent variable is a constant Tests whether the sample mean equals the specified value Difference in means Two-sample t test Independent variable is a binary variable Tests whether two sample means are equal to each other (could also be framed as testing whether the difference between two means is equal to zero). *These are equivalent statements ANOVA Independent variable is a categorical variable Tests whether all the means are equal to each other Correlation (r) Independent variable is also a continuous variable Significance tested using a t-distribution. Don’t worry about how to calculate this, your software will do this for you! The closer r is to -1 or 1, the stronger the association. The closer r is to 0, the weaker the association. Categorical Independent and Categorical Dependent Variables Want to see if two categorical variables are associated with each other? Or Want to know if the distribution of one categorical variable is related to the distribution of another categorical variable? Chi-square test! Your software will calculate the p-value for this test If your p-value is less than alpha (usually 0.05), we say that there appears to be an association between these two variables Analytic tools at a glance…putting it all Dependent Variable (Outcome) together Measure of Association Binary Continuous proportion mean one sample z-test one-sample t-test Test of Statistical Significance Constant Independent Variable (Exposure or Predictor) RR or OR Binary χ2 test difference in means Difference in proportions two-sample t-test two-sample z-test Categorical Chi-Square difference in means Can be used with categorical outcomes too! ANOVA Correlation (r) Continuous Stay tuned…for logistic regression in Case 6 t-test