Statistics PDF
Document Details
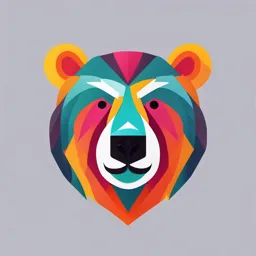
Uploaded by ReachableSard9497
Salawag National High School
Tags
Related
Summary
This document explains the fundamental concepts of statistics, including different types of data, measures of central tendency, dispersion, various statistical methods and distributions. It provides a concise overview that can be beneficial for learners of different educational levels, such as undergraduates.
Full Transcript
# Statistics Statistics is defined as the process of collection, organization, analysis, interpretation, and presentation of data. ## Types of Statistics - Descriptive Statistics - Inferential Statistics ## Descriptive Statistics The descriptive statistics is a type of statistics that is used t...
# Statistics Statistics is defined as the process of collection, organization, analysis, interpretation, and presentation of data. ## Types of Statistics - Descriptive Statistics - Inferential Statistics ## Descriptive Statistics The descriptive statistics is a type of statistics that is used to describe the data collected and summarize the data and its properties using the measures of central tendencies and the measures of dispersion. ## Inferential Statistics Inferential statistics is used to draw conclusions from the data. Inferential statistics requires statistical tests performed on samples, and it draws conclusions by identifying the differences between the 2 groups. ## Kind of Data - Qualitative data is when the data is descriptive or categorical. - Quantitative data is when the data is numerical information. ## Qualitative/Categorical Data Qualitative or Categorical Data is a data that cannot be measured or counted in the form of numbers. The categorical information involves categorical variables that describe the features such as a person's gender, home town etc. ## Nominal Data Nominal data are used to label variables without any quantitative value. Common examples are: Male/female, hair color, nationalities, name of people. ### Examples of Nominal Data | COLOR: | Nationality | |---|---| | Blonde | American | | Black | German | | Brown | Filipino| ## Ordinal Data Ordinal data/variable is a type of data that follows a natural order. Ordinal scales are often used for measures of satisfaction, happiness, and so on. ### Example: - Very likely, - Likely, - Neutral, - Unlikely, - Very unlikely ## Quantitative Data Quantitative data can be expressed in numerical values, making it countable and including statistical data analysis. For example, the price of a phone, the computer's ram, the height or weight of a person, etc. falls under quantitative data. ## Quantitative Data Can Be Classified The discrete data contain the values that fall under integers or whole numbers. The discrete data are countable and have finite values; their subdivision is not possible. ### Examples Of Discrete Data - Total numbers of students present in a class - Cost of a cell phone - Numbers of employees in a company - The total number of players who participated in a competition - Days in a week ## Interval Data Interval data is a type of data which is measured along a scale, in which each point is placed at an equal distance (interval) from one another. Interval data is one of the two types of discrete data. An example of interval data is the data collected on a thermometer - its gradation or markings are equidistant. ### Example of Interval Data - IQ Test - NAT Test - AGE - Temperature, in degrees Fahrenheit or Celsius (but not Kelvin) ## Ratio Scale Ratio scale are continuous data. It is a quantitative scale where there is a true zero and equal interval between neighboring points. ### Example of Ratio Data - Weight, height, length - Temperature in Kelvin - Area ## Quantitative Data Can Be Classified Continuous data are in the form of fractional numbers. It can be the height of a person, the length of an object, etc. Continuous data represents information that can be divided into smaller levels. ### Examples of Continuous Data - Height of a person - Speed of a vehicle - "Time-taken" to finish the work - Wi-Fi Frequency ## Measures of Central Tendencies Measures of central tendency are the values that describe a data set by identifying the central position of the data. There are three main measures of central tendency - Mean, Median and Mode. ### The Three Measures of Central Tendencies - Mean is the sum of all observations divided by the total number of observations. - Median is the middle or central value in an ordered set. - Mode is the most frequently occurring value in a data set. ## Measures of Relative Position Measure of position determine the position of a single value in relation to other values in a sample or population data set. ## Quartiles Quartiles are values that divide your data into quarters. The values that divide each part are called first, second, and third quartiles; and they are denoted by Q1, Q2, and, Q3 respectively. 1 2 3 4 5 6 7 8 Q1 Q2 Q3 ↓ ↓ ↓ ## Percentile Percentiles are a measure of the relative standing of observation within a data. Percentiles divide a set of observations into 100 equal parts and percentile scores are frequently used to report results from national standardized tests such as NAT, GAT, etc. ## Decile Decile is a method that is used to divide a distribution into ten equal parts. When data is divided into deciles, a decile rank is assigned to each data point in order to sort the data into ascending or descending order. *Formula for decile Dk = * $k/10(n + 1)$ ## Dispersion in Statistics Dispersion in statistics is a way of describing how spread out a set of data. It is the state of data getting dispersed, stretched, or spread out in different categories. ## Types of Measures of Dispersion Range is the measure of the difference between the largest and smallest value of the data variability. The range is the simplest form of Measures of Dispersion. Range = Largest scores - lowest scores ## Types of Measures of Dispersion Variance (σ^2): can be calculated by obtaining the sum of the squared distance of each term in the distribution from the Mean, and then dividing this by the total number of the terms in the distribution. Population variance: σ^2 = Σ(x - μ)^2 / N ## Random Variable Random variable is a variable that is used to quantify the outcome of a random experiment. It is variable whose possible values are determine by chance. ## Discrete Random Variable A discrete random variable is a variable that can take on a finite number of distinct values. For example, the number of children in a family can be represented using a discrete random variable. ## Discrete Probability Distribution A list of all possible values of a discrete random variable along with their corresponding probability is called a discrete probability distribution. ## Properties of Discrete Random Variable - The probability of each value of a discrete random variable is between 0 and 1 inclusive. 0≤P(x) ≤ 1 - The sum of all the probabilities is 1. ΣP(x) = 1 ## Continuous Random Variable A random variable that can take on an infinite number of possible values is known as a continuous random variable. An example of a continuous random variable is the weight of a person. ## Probability Mass Function Probability mass function can be defined as the probability that a discrete random variable will be exactly equal to some particular value. ## Probability Mass Function Properties - P(X = x) = f(x) ≥ 0. This implies that for every element x associated with a sample space, all probabilities must be positive. - Σf(x)=1. The sum of all probabilities associated with x values of a discrete random variable will be equal to 1. ## Normal Distribution The normal distribution is a bell-shaped, symmetrical distribution in which the mean, median and mode are all equal. If the mean, median and mode are unequal, the distribution will be either positively or negatively skewed. ## Z-SCORE Z score is also known as a standard score and is used to represent the number of standard deviations by which a raw score is above or below the mean. Z = (X - μ) / σ