Measuring Psychological Variables PDF
Document Details
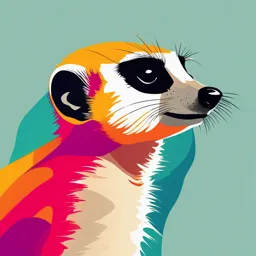
Uploaded by FlexibleWormhole6936
University of Galway
Dr. Jenny Groarke
Tags
Summary
This document discusses various aspects of measuring psychological variables, including theory, constructs, operational definitions, different scales of measurement, measurement error, and modalities of measurement. It also explores the critical concepts of validity and reliability in psychological measurement.
Full Transcript
Measuring Psychological Variables Dr. Jenny Groarke University ofGalway.ie Who am I? Lecturer in Psychology, UoG Honorary lecturer in Health Psychology, QUB Graduate of NUIG: BA, Hdip, PhD Music, Cancer, Emotions + Wellbeing, Technology Qual: Reflexiv...
Measuring Psychological Variables Dr. Jenny Groarke University ofGalway.ie Who am I? Lecturer in Psychology, UoG Honorary lecturer in Health Psychology, QUB Graduate of NUIG: BA, Hdip, PhD Music, Cancer, Emotions + Wellbeing, Technology Qual: Reflexive Thematic Analysis Quant: RCTs, Regression (SEM), Scale Development, ESM Outline Definitions: Theory, Construct, Operational definition Scales of measurement Measurement error Modalities of measurement Validity and reliability of measurement The Research Process Methods for evaluating claims Causal claims Experiments/RCTs bivariate/multivariate Association claims correlational research Frequency claims Surveys, observations Measuring Psychological Constructs Theory: A set of statements about the mechanisms underlying a particular behaviour, cognition, affective state. Variable: unit of measurement that has at least two levels/values Construct: A hypothetical construct is an explanatory variable which is not directly observable. Measuring Psychological Constructs Operational definition: procedure (or set of procedures) for quantifying a construct e.g., intelligence = scores on a standardised IQ test Measuring external manifestations of underlying construct 1. The operational definition is incomplete 2. The operational definition includes extra components Measuring Psychological Constructs How do I create an operational definition? Literature review: methods section Construct: Depression Clinical Interview Standardised Teacher Inventory Observations Categorical Vs Continuous Measurement Discrete Vs Continuous Frequencies Range [variability] Mode/Median – nonparametric tests Frequencies Mean Parametric tests Categorical variables Binary/dichotomous variable Modalities of Measurement Self-report measures + Direct, self-awareness - Bias Modalities of Measurement Physiological measures – HR, BP, GVSR, PET, fMRI + More objective - Expensive - Ecological validity - Operational definition Modalities of Measurement Behavioural measures – natural/structured: e.g., laughing vs RT + vast number of options, pick best for operationalization - Temporary or situational indicator of construct Measurement Procedure Using multiple measures + More confidence in validity of construct measurement - More complex multivariate statistical analysis - Two measures of the same thing may behave differently Measurement Procedure Sensitivity Measurement procedure must be sensitive enough to detect the magnitude of change we anticipate Range effects = incompatibility between the measurement procedure and the individuals measured ceiling effect = scores clustering at the high end of the scale floor effect = scores clustering at the low end of the scale Problem of Measurement Suppose we wished to measure the height of this glass and we obtained the following values: 10.1 cm 10.3 cm 11 cm 10.5 cm What is the real height of the glass? Problem of Measurement Can you think of any reasons why the values that we obtained for the height of the glass varied? Problem of Measurement Different angle of the ruler Different angle of viewing the ruler (parallax) Different person reading the measurement Most importantly, we assume that something remained constant…. What was that? Problem of Measurement We assume that the height of the glass did not change Problem of Measurement Every score on every measure is affected by two sources of variation: The characteristic that we wish to measure Any other variable that affects the score The effect of these other variables on the obtained score is called error Thus, each score = true measure of the characteristic + error Measurement Error Observer Environmental Participant error changes changes Validity of Measurement Validity = the degree to which the measurement procedure measures the construct it claims to measure Type of Description Validity Construct How well the variables are measured or manipulated Are the operational definitions applied in the study a good approximation of the constructs of interest? Internal The degree to which we can say variable A is responsible for variable B, and not some third variable C External The degree to which the results generalize to other populations, settings, situations Statistical How well the study minimizes the probability of Type I and Type II error Strength of association/effect size and its statistical significance Validity Causal Claims Association Claims Frequency Claims (e.g., music lessons increase IQ) (e.g., Smart phone use linked to (e.g., half of university students lower IQ) are depressed) Construct How well have you How well have you measured How well have you measured measured/manipulated the each of the two variables in the the variable in question? variables in the study? association? Internal Is there temporal precedence? Internal validity is not relevant Internal validity is not relevant Control for extraneous/confounding as there should be no causal as there should be no causal variables? claims claims External How representative is the sample, How representative is the How representative is the manipulations and measures? sample? sample – was it a random To what other settings/problems sample? might the association be To what other settings/problems generalized? might the estimate be generalized? Statistical What is the effect size? Is the What is the effect size? How What is the margin of error of difference statistically significant? strong is the association? the estimate? If there is a significant difference, If there is a significant what is the probability it is false? association, what is the (Type I) probability it is false? (Type I) If there is no difference, what is the If there is no association, what is probability it is false (Type II) the probability it is false (Type II) Construct Validity = is the appropriateness of inferences made based on observations or measurements (often test scores), i.e., whether a test measures the intended construct. Constructs are abstractions that are deliberately created by researchers in order to conceptualize the latent variable, which is correlated with scores on a given measure (although it is not directly observable) Measuring Psychological Constructs Psychological scales consists of a series of items, each of which constitutes an attempt to measure a construct Rosenberg Self-esteem scale "On the whole, I am satisfied with myself." "I feel that I have a number of good qualities." Validity of Measurement Construct Scores from a measurement procedure behave the same as the underlying construct Face Unscientific – measurement procedure ‘appears’ to measure what it claims to measure Content The test measures appropriate content for the construct (i.e., addresses all aspects of the construct) Predictive When scores from a measure accurately predict behaviour according to a theory Concurrent Scores from a new measure are closely related to scores on an established/gold-standard measure Convergent Strong relationship between scores from two (or more) different methods of measuring the same construct Divergent Weak or no relationship between scores on measures (or discriminant) of two different constructs Translation Validity Content validity involves examining the content of a measure (e.g., an IQ test) to test whether it covers a representative sample of the behaviour to be measured So, if you wish to test mathematical ability, then the content of the test should include mathematical problems and not general knowledge questions However, it is not always this simple. Content Validity Include a representative selection of items e.g., If a test of mathematical ability included only long division, then it would not constitute a representative sample of mathematical behaviour The apparent relevance of an item is not enough (this is face validity). e.g., If an item in a questionnaire asks: “Do you tell lies (a) often, (b) rarely or (c) not at all?”, simply responding (c) may not be an accurate measure of the person’s actual rate of lying Content Validity It is also necessary to be careful not to over generalise from the items used in the test E.g., a multiple-choice spelling test may measure a person’s ability to recognise correctly spelled words but may not be a good measure of the person’s ability to spell words from memory Finally, irrelevant features of the content should not interfere with the measurement of the characteristic E.g., if items use words that are unfamiliar to some people, then they might not perform as well Face Validity The face validity of a test how valid a test seems (i.e., at face value) Thus, it is not an objective measure of validity However, a test with high face validity can often obtain more cooperation from subjects and thus be a more reliable and valid test E.g., if you are testing the math’s skills of business people, it might be better to write items in terms of business examples rather than using mathematical terminology as the respondents will “see the point” of the test Construct Validity Criterion-related validity concerns the effectiveness of a test in predicting an individual’s performance on particular activities (the criterion) Criterion-Related Validity Predictive Validity The performance on the test is compared to criterion performance at a later date E.g., if we developed a test in order to select the best candidates for a job, we would compare performance on the test to job performance Criterion-Related Validity Sometimes it is not practical to wait for a period of time to test the validity of a measure Concurrent validity A measure should correlate highly with other measures of the same construct E.g., if we have a new test for I.Q., it should correlate highly with previous tests for I.Q. (though not too highly or it may be redundant). Criterion-Related Validity Convergent validity A measure should correlate highly with other measures of related constructs E.g., a new IQ test should correlate with performance on other related variables such as reading skill, math’s ability and so on. Criterion-Related Validity Discriminant validity A measure should not correlate with other measures with which it should not correlate E.g., if we have a new test for I.Q., it should not correlate highly with a test for colour vision Criterion-Related Validity Criterion Contamination If a criterion performance is employed to test the validity of a test, then it is crucial that the criterion performance is not affected by test performance. E.g., Let's imagine we want to test the validity of a test to rate new employees by comparing test scores to an evaluation by a senior employee. If the senior employee knows the scores of each graduate, they will be likely to give better evaluations to the employees who received higher test scores Construct validity may include both translation and criterion- related approaches to validity Construct Construct validity is a theoretical Validity position and translation and criterion-related procedures may both be employed to test construct validity Validity of Measurement Construct Scores from a measurement procedure behave the same as the underlying construct Face Unscientific – measurement procedure ‘appears’ to measure what it claims to measure Content The test measures appropriate content for the construct (i.e., addresses all aspects of the construct) Predictive When scores from a measure accurately predict behaviour according to a theory Concurrent Scores from a new measure are closely related to scores on an established/gold-standard measure Convergent Strong relationship between scores from two (or more) different methods of measuring the same construct Divergent Weak or no relationship between scores on measures (or discriminant) of two different constructs Meehl and Cronbach (1955) proposed the following three steps to evaluate construct validity: 1. articulating a set of theoretical concepts and their interrelations 2. developing ways to measure the hypothetical constructs proposed by the theory 3. empirically testing the hypothesized relations Meehl and Cronbach (1955) A nomological network defines a construct by illustrating its relation to other constructs and behaviours: represents the concepts (constructs) of interest in a study/test, their observable manifestations and the interrelationship among them. examines whether the relationships between similar constructs are considered when examining relationships between observed measures of the constructs. Distinguish between measures of different constructs and different measures of the same construct Messick (1989) "... an integrated evaluative judgment of the degree to which empirical evidence and theoretical rationales support the adequacy and appropriateness of inferences and actions based on test scores…" “a single study does not prove construct validity. Rather it is a continuous process of evaluation, reevaluation, refinement, and development.” Attempted to combine realist (measuring what is really there) and constructivist (creating useful measures) approaches to validity Realist vs Constructivist Realist: "Height is a property of the glass for which we collect evidence” Constructivist: “Height is a conceptual tool we use to work with glasses and other objects effectively” Messick (1989) Presented a new conceptualization of construct validity as a unified and multi-faceted concept. Consequential – What are the potential risks if the scores are invalid or inappropriately interpreted? Is the test still worthwhile given the risks? Content – Do test items appear to be measuring the construct of interest? Substantive – Is the theoretical foundation underlying the construct of interest sound? Structural – Do the interrelationships of dimensions measured by the test correlate with the construct of interest and test scores? External – Does the test have convergent, discriminant, and predictive qualities? Generalizability – Does the test generalize across different groups, settings and tasks? Threats to Construct Validity Inadequate Preoperational Explication of Constructs Interaction of Different Treatments, Interaction of Testing and Treatment Restricted Generalizability Across Constructs, Confounding Constructs and Levels of Constructs The "Social" Threats to Construct Validity Hypothesis Guessing Evaluation Apprehension Experimenter Expectancies Reliability of Measurement Reliability of a measurement procedure is the stability and/or consistency of the measurement. If the same individuals are measured under the same conditions, a reliable measurement procedure produces identical (or nearly identical) measurements When error is large, reliability is low and vice versa Measuring Psychological Constructs Psychological scales consists of a series of items, each of which constitutes an attempt to measure a construct Rosenberg Self-esteem scale "On the whole, I am satisfied with myself." "I feel that I have a number of good qualities." If our scale is working well, then the responses should relate to one each other every item is measuring the same thing, so they should give the same answer (simplified) Reliability of Measurement 1. Successive measurements Test-retest reliability Alternate/parallel-forms reliability 2. Simultaneous measurements Inter-rater reliability 3. Internal Consistency Split-half reliability Test-Retest Reliability Participant WAIS (Time 1) WAIS (Time 2) 1 115 120 2 112 113 What kind of statistical test 3 104 110 would we employ 4 113 107 to test whether 5 102 108 the WAIS is a 6 120 118 reliable test? 7 103 105 Test-Retest Reliability We use correlation to measure whether the test is reliable. As the test should measure the same characteristic both times, then the correlation should approach 1 if it is a reliable test. Test-Retest reliability is a good measure of how generalisable the scores on a test are from time to time Considerations Must note the interval between the first test and the second test (some tests have high only short/medium term reliability, e.g., IQ) Practice effects can improve performance If intervals are short, then subjects may remember answers to particular questions Alternate-Forms Reliability In a variation of the test-retest reliability procedure, we can compare performance on alternate but equivalent forms of a test. For example, if we wish to examine vocabulary, we could ask the subject to define 40 words on one occasion and a different 40 words on a second occasion Considerations It is not always possible to find an alternate form of the test you use As with test-retest reliability, the interval between the application of the alternate forms must be noted Practice effects may occur if items are similar in both forms (e.g., types of reasoning problems) Items that seem to be of the same difficulty may be easier or more difficult for certain subjects Inter-rater reliability = the degree of agreement between two observers who simultaneously record measurements R = correlation between the scores of the two observers % or by computing a percentage of agreement Split Half Reliability Both test-retest and alternate form reliability involve comparing two different administrations of a test (i.e., successive) Alternatively, we can compare the performance of subjects on equivalent items of the same test (inter-item reliability) we can avoid the practice and interval effects Split Half Reliability Measures of complex constructs usually contain multiple items Assumption is that each item, or group of items measures a part of the total construct. Thus, there should be some consistency between the scores for different items or groups of items Split Half Reliability To measure the split half reliability of a test, we compare the participants’ performances on two equivalent halves of the same test 1. The first problem is how to split the test in order to obtain two equivalent halves Q: What do you think would happen if we compared the first half of the test (e.g., items 1-20) to the second half (21-40) of a test? Split Half Reliability We rarely compare the first half to the second half because subjects performances are likely to differ because of factors such as warming up, practice, fatigue and boredom (reduce correlation) Split Half Reliability One popular method for obtaining two equivalent halves of a test is to compare scores on the odd items (e.g., 1, 3, 5 and so on) to scores on the even items (e.g., 2, 4, 6 and so on) We then compare scores on the equivalent halves by assessing the correlation between those scores Split Half Reliability Q Score For this short test, we could test the split half reliability by comparing subjects’ scores 1 5 on items 1, 3, 5 and 7 to their scores on 2 3 items 2, 4, 6 and 8. 3 4 4 3 5 4 Participant Half 1 Half 2 6 4 1 18 14 7 5 2 15 16 8 4 3 18 17 4 17 16 Cronbach’s Alpha There are many different methods that can be used to split a test in half The final method of measuring reliability that we will consider is to calculate Cronbach’s alpha (α) Cronbach’s alpha is the mean of all possible split half coefficients Cronbach’s Alpha So, with our short test, Cronbach’s alpha is the figure we would get if we worked out r for every possible split half (1,3,5,7 and 2,4,6,8; 1,2,3,4 and 5,6,7,8 and so on) and worked out the mean value Half 1=1,3,5,7; Half 2=2,4,6,8 Half 1=1,2,3,4; Half 2=5,6,7,8 Subject Half 1 Half 2 Subject Half 1 Half 2 1 18 14 1 15 17 2 15 16 2 15 18 3 18 17 3 14 17 And so on… 4 17 16 4 16 17 Luckily for us, SPSS calculates this value for us! Considerations Both split half and Cronbach’s alpha measure the inter-item reliability or internal consistency of a test This does not tell us how consistent it will be from time to time and place to place (use test-retest or alternate forms) Cronbach’s alpha is only suitable when the same characteristic is being measured throughout the test (homogenous). The validity of a measure concerns what it measures and how well it does so A test may be very reliable but not measure what it is supposed to measure This is because reliability is simply concerned Reliability and with the consistency of obtained results and Validity not whether those results are related to the characteristic being measured Reliability and Validity Independent but related criteria Reliability is a prerequisite for validity, a measure can’t be valid if it is not reliable BUT: there is no requirement for a measure to be valid for it to be reliable. Accuracy = the degree to which a measurement conforms to the established standard A measure could be reliable and valid – but not accurate Revision Quiz! https://b.socrative.com/login/ student/ GROARKE9410 Readings Wikipedia entries https://en.wikipedia.org/wiki/Construct_validity https://en.wikipedia.org/wiki/Test_validity Threats to construct validity http://www.socialresearchmethods.net/kb/consthre.php Anastasi, A., & Urbina, S. (1996). Psychological Testing (7th ed.). Pearson. (Chapters 5 & 6) Cronbach, L. J.; Meehl, P.E. (1955). "Construct Validity in Psychological Tests". Psychological Bulletin. 52 (4): 281–302. doi:10.1037/h0040957. PMID 13245896. Messick, S. (1995). "Standards of validity and the validity of standards in performance assessment". Educational Measurement: Issues and Practice. 14 (4): 5–8. doi:10.1111/j.1745-3992.1995.tb00881.x. Experimental Design Dr Jenny Groarke University University ofGalway.ie ofGalway.ie Lecture Outline Experimental Design Cause and Effect: Hume, Mill, Popper Types of Experimental Design Between-Subjects Within-Subjects Threats to validity External Internal University ofGalway.ie What are the similarities and differences between experimental and correlational research?? University University ofGalway.ie ofGalway.ie Cause and Effect Hume (1748): 3 criteria 1. Cause and effect must occur close together in time (contiguity) 2. The cause must occur before an effect does 3. The effect should never occur without the presence of the cause Cause is equated to high degree of correlation between University contiguous events ofGalway.ie Cause and Effect Hume (1748): 3 criteria to infer cause and effect 1. The tertium quid: the third variable problem 2. The direction of causality. University ofGalway.ie Cause and Effect Mill (1865) 1. Cause has to precede effect 2. Cause and effect should correlate 3. All other explanations of the cause-effect relationship must be ruled out University ofGalway.ie Cause and Effect Mill (1865) 1. The method of agreement: an effect should be present when the cause is present 2. The method of difference: when the cause is absent the effect must be absent also University ofGalway.ie Mill’s Logic Agreement: If X, then Y If “you increase physical activity”, then “you will lose weight” If X is regularly followed by Y, then X is sufficient for Y to occur University ofGalway.ie Mill’s Logic Disagreement: If not X, then not Y If “you do not increase steps”, then “you will not lose weight” If Y never occurs in the absence of X, then X is necessary for Y to occur For one event to cause another, it must be necessary University and sufficient for the event to occur ofGalway.ie Mill’s Logic 3. The method of concomitant variation: when the two previous relationships are observed, causal inference will be made stronger because most other interpretations of the cause-effect relationship have been ruled out University ofGalway.ie Cause and Effect? “Just because we observe that night always followed day in the past, does not prove that night will follow day again in future” - Attributed to Hume University ofGalway.ie Falsification Popper (1934) falsification of theoretical statements = the correct goal of experimentation Why? No number of observations of X before Y proves conclusively that X causes Y One observation of “not X then Y” or “X then not Y” proves it false Example: “All swans are white” No number of white swans proves this statement true, but one black swan proves this statement false. University ofGalway.ie Falsification If it is possible to increase physical activity and not lose weight (X then not Y) or it is possible to not increase physical activity and lose weight (not X, then Y) Then, increasing physical activity (X) does not cause weight loss (Y) University ofGalway.ie Falsification Is this simplistic? A little We can conclude that increasing physical activity (X) is not the sole cause of weight loss However, being hungry is not the sole cause of eating behaviour (there has to be something to eat) and, yet, we know it as a “cause” Increasing physical activity may interact with other behaviours to increase the likelihood of losing weight University ofGalway.ie Interrogating Causal Claims Rule Definition Covariance As A changes, B Changes (e.g., as A increases B increases and vice versa) Temporal A comes first in time, before B Precedence Internal There are no possible alternative Validity explanations for the change in B, A is the only thing that changed (randomUniversity ofGalway.ie assignment) Experiments To support a causal claim: Well-designed experiment in which variable A is manipulated and variable B is measured Variable A = IV Variable B = DV University ofGalway.ie Variables Experimental designs seek to establish whether a particular IV is the cause of a change in a particular DV If X then Y becomes If “change in IV”, then “change in DV” RQ: Does increasing physical activity reduce weight? University ofGalway.ie DISCUSS: 1. What is your operational definition (i.e., measurement procedure) for physical activity and weight? 2. How will you determine causality through your research design? University ofGalway.ie Isolating cause Control conditions One condition in which the cause is present (e.g., treatment/intervention) One condition in which the cause is absent (i.e., control condition) University ofGalway.ie Isolating Cause Removing the tertium quid: 1. Controlling other factors 2. Randomisation University ofGalway.ie (Roughly) Equivalent terms Independent Dependent Variable Variable (IV) (DV) Cause Effect Predictor Outcome Factor Criterion Influence Measure of the University ofGalway.ie Phenomenon (Roughly) Equivalent terms Between participants, Within participants, subjects, groups subjects, groups Independent participants, Repeated Measures subjects, groups Non-related Related Non-matched Matched (pairs) University ofGalway.ie Which stats when? Categorical DV Continuous DV Chi square, Categorical IV Logistic (or more ANOVA, t test complex) regression Logistic (or more Correlation, Linear Continuous IV complex) regression Regression University ofGalway.ie Experimental Designs Quasi Experiments True Experiments Between-Participants Within-Participants University ofGalway.ie True Experiment For a true experiment, you must be able to allocate/assign participants to conditions randomly That means you must be able to manipulate the IV If you cannot do so, then it is a quasi-experiment E.g., using a subject variable such as gender, age etc as an IV University ofGalway.ie Quasi-Experimental Designs Experimenter can’t control the manipulation of the IV (practical or ethical reasons) e.g., ‘risky’ behaviour We can’t randomly assign people to engage in that, but we could compare people who already do engage in the behaviour with those who don’t University ofGalway.ie Quasi-Experimental Design 2. One group pre-test/post-test design subject to time effects Measurement Treatment Measurement 1. One group post-test design no way of knowing if treatment caused effect, or size of effect University ofGalway.ie Between-Subjects Designs We wish to determine whether or not increasing physical activity causes weight loss Adults with overweight/obesity 1. an experimental group and 2. a control group Experimental group will increase their average daily step count Control group will not University Afterwards, we will measure weight in Kg ofGalway.ie Between-Groups design + simplicity + less chance of practice and fatigue effects + when it is impossible for a pt to participate in all conditions - Expense in terms of time, effort and participant numbers University - Less sensitive to experimental manipulations ofGalway.ie One Factor Between-Subjects Design IV DV Group A Instructed to increase Weight (experimental) ave daily step count by 10% Group B Instructed to maintain Weight (control) current step count University ofGalway.ie Creating Equivalent Groups This is an experiment, because it was possible for people to be assigned randomly to different conditions Random assignment is used to minimise the possibility of participant variables that will impact the DV We must assume that the groups would have the same weight/BMI if one had not increased their stepcount University ofGalway.ie Random Assignment Random assignment is used to minimise the possibility of participant variables that will impact the DV – We must assume that the groups would have the same level of DV had they not been exposed to treatment/intervention University ofGalway.ie Random Assignment Does not guarantee that groups will be equal on all participant variables BUT it should spread any confounds equally among conditions Pure random assignment might mean that we have unequal numbers in our groups Block randomization ensures each condition is allocated a participant before the next participant is chosen (randomizer.org) University ofGalway.ie Matching When using small groups, random assignment may not create equivalent groups If there is a relevant variable that you wish to control for, then you may need to match the groups for that variable Examples: If using a brain measure, control for handedness If measuring reaction times, control for caffeine intake Weight/BMI - Control for height, current Level of PA? University ofGalway.ie Matching University ofGalway.ie Matching University ofGalway.ie Matching What should I match for? Know your dependent variable What influences it and in what way? You can never control for every possible variable in real experiments Matching participants takes time With small groups, it may not be possible to matchUniversity ofGalway.ie your groups Equivalent Groups Using equivalent groups allows you to control for selection effects University ofGalway.ie One Factor (IV) Between-Subjects Design IV DV Group A Increase PA Weight (experimental) Group B No PA increase Weight (control) Must be University equivalent Baseline PA, weight, gender? ofGalway.ie Within-Subjects Designs Controlling participant variables requires equivalent groups Alternatively, we can simply use the same participants in each condition These designs are called within-subject designs or repeated measures designs University ofGalway.ie Within-Subjects Designs + economy, smaller N + sensitivity - Conditions have to be ‘reversable’ - Higher participant burden - Sequence effects University ofGalway.ie One factor (IV) Within-Subjects Design IV level 1 DV IV level 2 DV Group A Control Test Treatment Test University ofGalway.ie Within-Subjects Designs Same sequence as one group pretest-posttest design, but rationale is different The “pretest” in this case is a measure of the DV at one level of the IV, not a measure of irrelevant variation Although the sequence of events is the same, the rationale will determine the statistical tests and conclusions drawn University ofGalway.ie Within-Subjects Designs Within-Subject designs have many advantages: Cheaper Easier to get sufficient numbers Tightest control of participant variables University ofGalway.ie Within-Subjects Designs However, within subject designs take longer to run per participant Such designs may result in sequence effects Sequence effects Prior exposure to the IV or DV 1. May modify the effect of the next level of the IVUniversity 2. May modify the next measure of the DV ofGalway.ie Sequence Effects Prior exposure to a level of the IV (xmg of drug/silence) May modify the effect of the next level of the IV (increase reaction/decrease reaction to music – carry over) May modify the next measure of the DV (effects of IV level 1 may carry over) University ofGalway.ie Sequence Effects Carryover effects IV level 1 DV IV level 2 DV Group A Control Test Treatment Test Expected effect University ofGalway.ie Sequence Effects Prior measurement of the DV (BP/RT/Self-report) 1. May modify the effect of the next level of the IV (increase reaction/decrease reaction- carry over effects) 2. May modify the next measure of the DV (practice effects) University ofGalway.ie Within-Subjects Designs Carryover effects IV level 1 DV IV level 2 DV Group A Control Test Treatment Test University ofGalway.ie Sequence Effects Carry-over effects: Any effect of a condition on the next condition Practice effects: The effect of previous measurement on future measurement of the DV Can increase or decrease performance/alter response Can occur in some between subject designs too University ofGalway.ie Counter-balancing To control for sequence effects, we counterbalance presentation of conditions (i.e., levels of the IV) When we measure a DV once for each condition, we typically try to achieve complete counterbalancing Each sequence is presented to an equal number of participants University ofGalway.ie Counter-balancing Complete Counterbalancing IV level DV IV level DV Group A Control Test Treatment Test Group B Treatment Test Control Test University ofGalway.ie Counterbalancing Complete Counterbalancing For 2 conditions, 2 sequences AB, BA For 3 conditions, 6 sequences ABC, ACB, BAC, BCA, CAB, CBA Number of sequences is the factorial of the number of conditions (3! = 3x2x1) For 4 conditions, 24 sequences For 6 conditions, 720 sequences So, complete counterbalancing becomes unwieldy quite quickly University ofGalway.ie Counterbalancing Partial Counterbalancing If you have a number of conditions (4 or more), you should consider partial counterbalancing e.g., Latin Square In a balanced Latin Square, each condition occurs equally often in every sequential position and every condition precedes and follows every other conditionUniversity ofGalway.ie exactly once Latin Square Example 6x6 Latin square (for 6 conditions) ABFCED BCADFE CDBEAF DECFBA EFDACB FAEBDC Then, assign equal number of participants to each row University ofGalway.ie Latin Square Examples University ofGalway.ie Mixed Designs Both between group and repeated measures designs have weaknesses Between group designs must assume equivalent groups Repeated measures carryover and practice effects Mixed designs use both types of factors Repeated measures factor = Time Between groups factor = Intervention Vs Control University ofGalway.ie More Complex Designs Randomised, pretest-posttest, control-group design Solomon’s four-group design University ofGalway.ie Pretest-Posttest Control Group Designs DV IV DV Group A Pretest Treatment Posttest (experimental) Group B Pretest No treatment Posttest (control) University ofGalway.ie Pretest-Posttest Designs Pretest results can be used to more accurately judge the effects of the IV It is possible to use the pretest as a covariate (ANCOVA) If participants score high in the pretest and high in the posttest, then we have less confidence in the effect of the IV The correlation between pre and posttest is excluded in University this ofGalway.ie test Multilevel, completely randomised, between-subjects design Group A Pretest Treatment 1 Posttest (experimental 1) Group B Pretest Treatment 2 Posttest (experimental 2) Group C Pretest No treatment Posttest (control) University ofGalway.ie Solomon’s four-group design Experiencing the pretest might affect affect post-test Might be the same in the experimental and control groups, but Pretest might interact with the experimental manipulation to confound effect Sequence in Solomon’s design protects against this University ofGalway.ie Solomon’s four-group design 3 Control Groups – Pretest-posttest only, Treatment- posttest only and Posttest only Carryover effects Group A Pretest Treatment Posttest Group B Pretest Posttest Group C Treatment Posttest Group D Posttest University ofGalway.ie Interrogating Causal Claims Rule Definition Construct Validity How well were the variables measured and manipulated? Internal The degree to which we can say variable A (IV) is responsible for variable B (DV), and not some third variable C (confound) External Validity To what people or settings can you generalise the causal claim? (random sampling and ecological validity) Statistical Validity How well do the data support the causal claim? (statistical significance, effect size)University ofGalway.ie Internal Validity If scores on your measures are not due to your manipulations but are instead actually caused by other factors, then they lack ‘internal’ validity -> addressed by good experimental design University ofGalway.ie Threats to Internal Validity Confounding variables: Internal validity is threatened when causality cannot be confidently attributed to changes in the IV because of other simultaneous changes University ofGalway.ie Threats to Internal Validity Group Threats: Baseline differences in our experimental and control groups Participants can vary in many unique characteristics, some learned and some inherent If there is an unequal distribution of these subject- related variables across experimental groups -> possible threat to internal validity University ofGalway.ie Selection differences Can produce group threats. e.g., using volunteers for one group and non- volunteers for the other, or using college students for one group and patients for another group University ofGalway.ie Selection differences If scores on the DV differ between the groups, the discrepancy may be due to the independent variable or to the subject- related variable. University ofGalway.ie Selection differences Before Randomly assign participants to conditions Match groups (assign equal distribution to each level of IV) Use variable as part of model, as predictor After Use variable as covariate Temper claims (i.e., limitation of the study) University ofGalway.ie Regression to the mean Subjects with extreme scores on a first measure of the dependent variable tend to have scores closer to the mean on a second measure. "Take any dependent measure that is repeatedly sampled, move along it as in a time dimension, and pick a point that is the "highest (lowest) so far. On the average, the next point will be lower (higher), nearer the general trend." Campbell (1969, p. 414) University ofGalway.ie Regression to the mean If we had abnormally high scores at Time 1, then we would expect them to stabilize at Time 2. This would confound the expected effect of the Maths Education intervention University ofGalway.ie Regression to the mean Before Be careful if you are using an “abnormal” sample Know your measures well Compare your Time 1 scores to expected norms (if they are quite different, you may have this problem) After identify abnormal individual scores (i.e., outliers), because shifts in these may bias overall interpretation University ofGalway.ie History Outside events may influence subjects in the course of the experiment or between repeated measures of the dependent variable. If an event occurs for one group of participants (or one condition) but not another, it will affect internal validity University ofGalway.ie History Once again, if scores on the dependent measure differ at these two times, the discrepancy may be due to the independent variable or to Event A (e.g., a relevant news story on the day Group 1 is tested). University ofGalway.ie History Before Good research design requires that you explicitly identify relevant historical variables Standardise exposure across groups (if unavoidable for one group, then expose other group) Tightly control experimental environment After Post hoc questionnaire/interview Temper claims University ofGalway.ie Maturation Participants may change in the course of the experiment or between repeated measures of the dependent variable due to the passage of time per se. Some of these changes are permanent (e.g., biological growth), while others are temporary (e.g., fatigue). University ofGalway.ie Maturation If scores on the dependent measure differ at these two times, the discrepancy may be due to the independent variable or to naturally occurring developmental processes University ofGalway.ie Maturation Before Use control group Use variable as part of model, as predictor After Use variable as covariate Temper claims University ofGalway.ie Selection-Maturation Interaction Selection and maturation do not necessarily operate in isolation. They sometimes combine to produce effects that reduce validity. University ofGalway.ie Instrumentation The reliability of the instrument used to gauge the dependent variable or manipulate the independent variable may change in the course of an experiment. Examples include changes in the calibration of a mechanical measuring device as well as the proficiency of a human observer or interviewer (confirmation bias). For this reason, you must report the reliability of your questionnaires University ofGalway.ie Repeated Testing The prior measurement of the dependent variable may affect the results obtained from subsequent measurements. This is particularly likely when using questionnaires with memorable questions (e.g., emotionally sensitive questions) or answers (e.g., riddles, logic puzzles) University ofGalway.ie Repeated Testing and Instrumentation Before Know the procedures/questionnaires that you will use well (use pilot studies etc) Research test-retest reliability and interrater reliability of measures Ensure that all staff are trained Use computer-controlled experiments After Evaluate the reliability of your measures (e.g., test-retest reliability, Cronbach’s alpha for questionnaires and inter-rater University ofGalway.ie reliability for observations) Differential Mortality “selective attrition” In the course of an experiment, some subjects may drop out before it is completed. If participants in one group are more likely to discontinue their participation part way through an experiment than participants in another group then this will threaten validity Example: Anti-depressant medication University ofGalway.ie Selective Attrition Before Know your measures well Compare the total N for both groups at both times Know your population well After Intention to treat/per protocol analysis Either eliminate randomly from Time 1 or construct missing values for Time 2 Temper claims University ofGalway.ie Reactivity and Experimenter Effects Measuring a person’s behaviour can change it e.g., 24-hour Food Recall ‘Evaluation Apprehension’ ‘social desirability’ University ofGalway.ie Reactivity and Experimenter Effects The experimenter’s age, race, sex and other characteristics may affect the results they obtain Experimenters can subtly, unconsciously bias the results the obtain by the way they interact with the participants University ofGalway.ie Reactivity and Experimenter Effects Expectations of an outcome by persons running an experiment may significantly influence that outcome. As with instrumentation, the reliability of the instrument used to gauge the dependent variable or deliver the independent variable is suspect Must be aware of this potential risk (researchers are human!) University ofGalway.ie Reactivity and Experimenter Effects ‘Demand characteristics’ – participants behave in ways to give the experimenter the data they ‘want’ (good participants) or not. -> Double blind University ofGalway.ie Finally For a variable to confound your results, it must explain variation in your DV Many variables covary with levels of your IV, you need to identify those relevant to your study Not all simultaneous variables are bad! University ofGalway.ie Types of Variation Systematic Unsystematic University ofGalway.ie External Validity If your findings are only valid for the specific situation within which you obtained them, then they lack ‘external’ (or ecological) validity Experimental effects can be very reliable (i.e., reproducible) without being very valid in ‘everyday’ contexts University ofGalway.ie Threats to External Validity Overuse of special participant groups: Overreliance on undergraduates, and volunteers Restricted number of participants: Underpowered to identify statistically significant (small) effects University ofGalway.ie Threats to External Validity Maximising Generality Before: Representative sampling (typical of the population) Random or Stratified After: Replications - settings/circumstances/populations University ofGalway.ie Threats to Internal Validity University ofGalway.ie Reducing Variance Random/stratified selection protects external validity Random assignment Reduces threats to internal validity from regression to the mean and attrition Control group Reduces threats to internal validity from instrumentation, history, and maturation University ofGalway.ie Why did my experiment show a null result? Not enough variability between levels Ineffective manipulation of IV a 1 day workshop did not change DV but a 12 week intervention might Measurement of DV not sensitive Researchers measured change in BMI, enough instead of Kg Ceiling or floor effects on the IV Cancer survivors with obesity have high health literacy Ceiling or floor effects on the DV Step count is already high Too much variability within levels Measurement error Multiple sources of random error Individual differences Scores on DV are influenced by individual differences in motivation/ability Situation variability External influences on DV What are the similarities and differences between experimental and correlational research?? University University ofGalway.ie ofGalway.ie Correlational & Experimental Research Both attempt to assess the relationship between two variables. Conclusions of both are limited by the quality of the measurement (validity, reliability) The experimental method manipulates the presumed causal variable, and the correlational method measures it. Different statistical tests, r or B vs F or t are not that different Correlational & Experimental Research Only experiments can assess causality Reasons for not knowing causal direction in correlational studies o Third-variable problem o Unknown direction of cause Experimental manipulation creates levels that may be unrealistic [high v low, continuum] An experiment can determine whether one variable can affect another, but not how often or how much it actually does, in real life. For that, correlational research is required. Correlational & Experimental Research Complications with experiments o Uncertainty about what was really manipulated ▪ A version of the third-variable problem o Can create unlikely or impossible levels of a variable o Often require deception o Not always possible to conduct expt Experiments are not always better. An ideal research program includes both designs. Further Reading Goodwin, C. J. (2007). Research In Psychology: Methods and Design, 5th Edition. Wiley. (Chapters 5-8) University ofGalway.ie N of 1/ Single Case Designs University University ofGalway.ie ofGalway.ie Lecture Outline What is a single case design? Reading and Interpreting Graphs Single case designs A-B and withdrawal designs Multiple baseline design Alternating Treatments Changing Criterion Single-case experimental designs studying prospectively and intensively a single person or small group of persons over time; measuring repeatedly and frequently the outcome in all phases of the study; sequentially applying and/or withdrawing the intervention University ofGalway.ie Single-case experimental designs A wide variety of designs that use a form of experimental reasoning called baseline logic to demonstrate the effects of the independent variable on the behaviour of individual subjects. Baseline logic entails three elements—prediction, verification, and replication—each of which depends on an overall experimental approach called steady state strategy. Steady state strategy entails exposing a subject to a given condition while trying to eliminate or control any extraneous influences on the behaviour and obtaining a stable pattern of responding before introducing the next condition. University ofGalway.ie Background Most evidence derived from between-person designs In an effective intervention, some intervention participants who did not benefit, or who experienced harm, and there will some control participants who did benefit. Only when response distributions are non-overlapping can we presume ‘most’ people will benefit from the intervention University ofGalway.ie Background N-of-1 designs: response to treatment is evaluated for one person. Patient/individual heterogeneity is common: Can one treatment model serve an entire population? Is a personalized model needed for each person? Or different models for different subgroups? Generalisable knowledge is not the goal University ofGalway.ie Background Less influenced by recruitment problems Lower risk of type 2 error Sample size = number of data points Power from number of repeated measures not number of pts University ofGalway.ie Background Single case designs do not necessarily focus on one participant could be one ward/one hospital could be one shop/retailer could be one group of people/context could even be one intervention group need to be similar enough to be replications University ofGalway.ie When and Why? What situations are suitable? Few participants Extended contact e.g., mHealth pilot interventions with daily data precision medicine/health Similar to Qualitative (and works well with it) University ofGalway.ie When and Why? What questions? Effectiveness of individualised intervention (precision medicine/health psychology, ABA, educational psych) Questions of dynamics (change over time) Which intervention components are effective? University ofGalway.ie When and Why? In medicine, N-of-1 are gold-standard for generating evidence for individual treatment decisions. Typically, 1-3 patients, repeated measures, sequential and randomized introduction of an intervention Not case reports, a priori hypotheses = experimental designs University ofGalway.ie When and Why? evaluating the efficacy of a current intervention for one particular patient in daily clinical practice to provide the best treatment based on evidence rather than clinical impressions; University ofGalway.ie When and Why? conducting research in a clinical setting (outside a research team) with a single or few patients; piloting a novel intervention, or application/modification of a known intervention to an atypical case or other condition/type of patients that the intervention was originally designed for; University ofGalway.ie When and Why? investigating which part of an intervention package is effective; working with rare conditions or unusual target of intervention, for which there would never be enough patients for a group study; University ofGalway.ie When and Why? impossibility to obtain a homogenous sample of patients for a group study; time limitation (e.g. a study needing to be completed within 8 months, e.g. for a dissertation/thesis...), or limited funding not allowing recruitment of a group University ofGalway.ie Analysis N-of-1 Statistics Some novel statistical approaches to N-of-1 data will not cover these today See: Vieira, McDonald, Araújo-Soares, Sniehotta & Henderson (2017) Naughton & Johnston (2014) University ofGalway.ie Visual Analysis As behaviour change is a dynamic and ongoing process, the behaviour analyst (the practitioner or researcher) - must maintain direct and continuous contact with the behaviour under investigation. Graphs are major devices in SCRD, allow the analyst to organise, store, interpret and communicate results of work. Visual Analysis systematic form of examination to interpret graphically displayed data. University ofGalway.ie Benefits of Visual Analysis 1. Plotting each measure of behaviour on a graph right after observation provides the analyst (researcher/practitioner/patient) with immediate access to an ongoing visual record of the participants behaviour – responsive. 2.Direct and continual contact with the data in a readily analysable format enables the researchers to explore interesting variations in behaviour as they occur 3.Visual analysis of graphed data is quick and is relatively easy to learn. University ofGalway.ie Benefits of Visual Analysis 4.Graphs enable and encourage independent judgements and interpretations of the meaning and significance of behaviour change. 5.Visual Analysis can provide feedback to the people whose behaviour the graphs depict (self-monitoring/management). 6.Visual Analysis facilitate the communication, dissemination, and comprehension of behaviour change among a wide variety of recipients (e.g., professionals, parents, government agents responsible for policymaking). University ofGalway.ie Is this intervention working? Number of Steps Goal = increase steps Time University ofGalway.ie Is this intervention working? Number of Steps Goal = increase steps University ofGalway.ie Line Graphs SCRD primarily uses line graphs Each point on a line graph shows the level of some quantifiable dimension of the target behaviour (i.e., the dependent variable ) in relation to a specified point in time and/or environmental condition (i.e., the independent variable ) in effect when the measure was taken. Typically use equal interval scale A condition change line indicates that an independent variable was manipulated at a given point in time. University ofGalway.ie Features of a Line Graph University ofGalway.ie University ofGalway.ie University ofGalway.ie University ofGalway.ie Line Graphs University ofGalway.ie What characteristics of behaviour can you see from reading a graph? Changes in: 1. Variability 2. Trend 3. Level How those changes occur: 1. Sudden 2. Gradual 3. Delayed University ofGalway.ie Variability Level Considerations Before beginning your intervention the data should have no physical trend and physical variability should be small relative to the expected change. If your baseline data are unstable, there are 3 ways to reduce the variability: 1. analyse and remove the sources of variability 2. wait for stability 3. change temporal unit of measurement University ofGalway.ie Examining your Data 1. There are no changes: Wait, there may be a delayed effect of the intervention Change treatment Change elements of the treatment (eg., dose) 2. Stop intervention if deterioration (and adverse consequences) 3. Continue if improvement University ofGalway.ie Single case Designs Once we choose an intervention, we must then decide how to implement that intervention Most importantly, we need to do so in such a way as to ensure that we can tell whether the intervention is working or not The experimental designs used for this work are called single case designs University ofGalway.ie Types of SCED Reversal Multiple Baseline Alternating treatment Changing Criterion University ofGalway.ie Single case Designs The simplest design is the A-B design. In this we simply try the intervention and look for a change in behaviour A stands for a baseline phase in which we measure the behaviour but do not intervene B stands for the phase during which the intervention is in place University ofGalway.ie Baseline A baseline condition does not necessarily mean the absence of instruction or treatment per se, only the absence of a specific independent variable of experimental interest. The independent variable should be introduced when stable baseline responding has been achieved. The independent variable should not be introduced when an ascending or descending baseline indicates improving performance. The independent variable should be introduced when an ascending or a descending baseline indicates deteriorating performance. An independent variable should not be imposed on a highly variable, unstable baseline University ofGalway.ie A-B Example Percent Attending Behavior 100 80 60 40 Baseline Reinforcement Contingency 20 0 5 9 15 20 No. of 10-min. Observation Sessions Walker, H.M., & Buckley, N.K. (1968). The use of positive reinforcement in conditioning attending behavior. Journal of Applied Behavior Analysis, 1, 245-250 A-B Drawback The A-B design does not allow us much control. The problem is that the behaviour may have changed because of a some other simultaneous occurrence. Internal Validity University ofGalway.ie A-B Design A-B design is not wholly satisfactory because (a) the investigator does not know how it worked (b) and therefore, the investigator cannot predict the long-term success for the client Two main extraneous sources of control: Maturation: physical changes in the client History: environmental events occurring concurrently with the experiment Single case designs are means to enhance internal validity (increase our confidence that the intervention caused the change) University ofGalway.ie Withdrawal Designs The simplest way to examine whether an intervention is working is to remove the intervention (withdrawal or reversal) If the intervention was the reason for the behaviour change, then behaviour should revert to its initial level. This is called an A-B-A design A : Baseline B : Intervention A : Baseline University ofGalway.ie A-B-A Example Increasing Classroom Attending 100 Percent Attending 80 Behavior Extinction 60 Reinforcement Baseline Contingency 40 20 0 5 9 15 20 25 30 35 No. of 10-min. Observation Sessions Walker, H.M., & Buckley, N.K. (1968) The use of positive reinforcement in conditioning attending behavior. Journal of Applied Behavior Analysis, 1, 245-250 Withdrawal Designs Naturally, if an intervention does work, it is unethical to deny the client the treatment Thus, in practice, the intervention is usually re-administered This is called an A-B-A-B design A: Baseline B: Intervention A: Baseline B: Intervention University ofGalway.ie A-B-A-B Example Withdrawal Designs There are a number of variations of the withdrawal design: B-A-B Design B : Intervention A : Baseline B : Intervention This design can be used to for two reasons: 1. To examine whether an intervention that is already in place is working 2. When a behaviour is so severe it requires immediate intervention University ofGalway.ie Withdrawal Designs A-B-A-B-C-B-C Design A : Baseline B : Intervention 1 A : Baseline B : Intervention 1 C : Intervention 2 B : Intervention 1 C : Intervention 2 This design is used to compare 2 approaches. However, there may be sequential effects that are not highlighted by this design University ofGalway.ie Withdrawal Designs Advantages: the most robust way to measure the effectiveness of an intervention (Kazdin, 1994) Disadvantages withdrawing treatment may result in harm to the client ‘carryover effects’: Some behaviours/outcomes trained during interventions may not decrease during the next baseline phase (e.g., social skills, beliefs underlying behaviours) University ofGalway.ie Activity University ofGalway.ie Multiple Baseline Designs If we cannot remove treatment, we must find another way to provide a control for maturation and history Multiple baseline designs provide this control by comparing the effect of the intervention across cases, behaviours, or settings If we wish to examine the effect of an intervention across cases, we introduce the intervention for one case while the others remain on baseline University ofGalway.ie Multiple Baseline Across cases 100 Strength of Belief (0 75 Ron - 100) 50 25 0 4 9 14 19 Baseline Cognitive Therapy 100 Strength of Belief (0 - 100) 75 Jim 50 25 0 5 9 14 19 100 Strength of Belief (0 75 Gary - 100) 50 25 0 5 0 13 18 Multiple Baseline Designs If the intervention results in a marked change in observed responding on its introduction and not before, then we can have confidence in its effect. The more often, this effect is replicated, the more confidence we may have We may also examine the effect of an intervention across behaviours. To do this, we introduce the intervention for one behaviour while the others remain on baseline University ofGalway.ie Multiple Baseline Across Behaviors 100 Strength of Belief (0 75 Verbal Aggression - 100) 50 25 0 4 9 14 19 Baseline Intervention 100 Strength of Belief (0 75 Vandalism - 100) 50 25 0 5 9 14 19 100 Strength of Belief (0 75 Physical Aggression - 100) 50 25 0 5 0 13 18 Multiple Baseline Designs Finally, we can examine the effect of the intervention in the same case, the same behaviour but in different contexts We introduce the intervention for one context while the others remain on baseline University ofGalway.ie Multiple Baseline Across Settings Multiple Baseline Designs Maturation and history: Multiple baselines control for maturation and history because the intervention shows a marked effect on its introduction for each case, behaviour, or setting. It would be unlikely for biological or environmental effects to occur numerous times within the one individual or across individuals at the very point at which the intervention was introduced The more replications of the effect, the firmer the case for the intervention University ofGalway.ie Multiple Baseline Designs For this reason, however, it is crucial for a multiple baseline that all variables other than that systematically manipulated are held constant. Thus, we could not use a multiple baseline across cases and behaviours (physical aggression in Jim and verbal aggression in Mary) It would not be clear whether differences in the observed behaviour were due to the individual, the behaviour, or the intervention. University ofGalway.ie Multiple Baseline Designs Advantages: Multiple baseline designs allow us to examine the effectiveness of an intervention without removing it This is particularly useful for measuring the effectiveness of skills training generalisation University ofGalway.ie Multiple Baseline Designs Disadvantages: If behaviour requires immediate intervention, then multiple baseline would not be suitable It is possible to get cross-contamination across behaviours and contexts (generalisation) and comparable cases may be difficult to find University ofGalway.ie Alternating Treatment Design University ofGalway.ie Alternating Treatment Design If immediate intervention is required, we may choose an alternating treatment design In an alternating treatment design, the effects of two or more interventions are compared within the same phase Each contingency/treatment usually presented at random One of the contingencies may be a baseline University ofGalway.ie Alternating Treatment Example 90 % of Time on Task 68 45 Ritalin Pill Placebo 23 0 10 12 11 2 3 5 7 9 1 4 6 Days 8 Alternating Treatment Example 40 Daily Anxiety Ratings 33 Benzo Pill Placebo 25 No Pill 18 10 2 3 5 6 8 1 4 7 9 10 11 12 DAYS Alternating Treatment Example Alternating Treatment Design Advantages Allows two or more active treatments to be compared Does not require withdrawal of treatment Comparisons can be made over a much shorter period (cheaper for client) Do not need a formal baseline to make valid inferences Can be useful for targets that are changing naturally University ofGalway.ie Alternating Treatment Design Disadvantages If the intervention has a lasting effect then there may be no difference between the intervention and baseline conditions (use multiple baseline instead) Changing Criterion Design A changing criterion design begins with an A-B stage, but sequential performance criteria are specified i.e., successive goal levels of the target behaviour are specified and reinforcement is contingent on achieving the criterion in each phase As the criterion increases/decreases so should the level of the target behaviour University ofGalway.ie Changing Criterion Criteria for successive stages Changing Criterion Criteria for successive stages Changing Criterion Design Like the multiple baseline design, if behaviour changes with each change in the level of the intervention, then we have experimental control Also, the more replications of this effect, the more confident we can be about the experimental control University ofGalway.ie Considerations The changing criterion design is less satisfactory than withdrawal or multiple baseline designs in ruling out the influence of extraneous events that give rise to the observed behaviour change If an extraneous event is having a gradual effect on behaviour, then it is likely to change in the same direction as the gradually increased intervention would predict If the behaviour closely matches the criterion, then this concern is lessened University ofGalway.ie Considerations Alternatively, this design may be strengthened by making bidirectional changes in criterion That is, the criterion may be made less stringent (a partial withdrawal) and then more stringent If the target behaviour increases and decreases with the criteria in place then it is highly unlikely that any other event is controlling the behaviour University ofGalway.ie Considerations Finally, care must be taken when choosing the criteria for each phase If the criterion is too stringent, then we may lose control completely as the client will not reach the criterion and not be reinforced If the criterion is not stringent enough, then treatment will take longer and be more expensive University ofGalway.ie Review Single case Designs Allow us to measure whether an intervention worked A-B is the simplest form, but provides no control for history or maturation Withdrawal or reversal designs: A-B-A etc Advantages and disadvantages of withdrawal designs Multiple baselines Across cases, behaviours, and settings Useful for irreversible changes Requires baseline phase Alternating Treatments University ofGalway.ie Helpful Reading Cooper, Heron & Heward (2020) Applied Behaviour Analysis. Third Edition. - Available through Library. Part 3 – Evaluating and Analysing Behaviour Change Dallery, J., & Raiff, B. R. (2014). Optimizing behavioral health interventions with single-case designs: from development to dissemination. Translational behavioral medicine, 4(3), 290-303. Kratochwill, T. R., Hitchcock, J., Horner, R. H., Levin, J. R., Odom, S. L., Rindskopf, D. M., & Shadish, W. R. (2010). Single-case designs technical documentation. What works clearinghouse. University ofGalway.ie Single case Designs Single case Designs Single case Designs Reading Graphs Reading Graphs Reading Graphs Reading Graphs Reading Graphs Reading Graphs Chi Square and non-parametric statistics Dr Jenny Groarke University University ofGalway.ie ofGalway.ie Lecture Outline Non-parametric statistics Chi Square Frequencies Observed scores, expected scores Significance Other Non-parametric tests University ofGalway.ie Non-Parametric Statistics Used when the data cannot be measured on a quantitative scale, or when The numerical scale of measurement is arbitrarily set by the researcher, or when The parametric assumptions such as normality or constant variance are seriously violated. University ofGalway.ie Test for Normality 1 To test the normality of variables A and B, we will use the Explore function Under Analyze, choose Descriptive Statistics and then Explore In the dialogue box, enter the total variables for A and B into the Dependent List Under Plots, choose Stem and leaf and Histogram University ofGalway.ie Non-Gaussian Distributions University ofGalway.ie Test for Normality 2 Analyze → Descriptive Statistics → Explore Check Skew and Kurtosis values Calculate the z score for skewness and kurtosis by dividing the skew/kurtosis value (e.g., 0.258) by its standard error (e.g., 0.717) If the z score is above 1.96, then the data are significantly different from normal University ofGalway.ie Data not Normal!! Don’t panic! If you find your data do not fit a normal distribution Check your work (correct column, data inputted correctly etc) Speak to your supervisor Read about your variable (is it usually normal?) Solutions Transform data (ask your supervisor) Use non-parametrics University ofGalway.ie Rank order tests University ofGalway.ie Chi Square Chi Square is a non-parametric test However, unlike other non-parametric tests, chi square deals with frequencies rather than ranked scores Chi square is denoted by χ2 University ofGalway.ie Which stats when? Categorical DV Continuous DV Chi square, Categorical IV Logistic (or more ANOVA, t test complex) regression Logistic (or more Correlation, Linear Continuous IV complex) regression Regression University ofGalway.ie Chi Square – When to use Chi square is used when participants are allocated to categories Data can be displayed in a contingency table or a crosstabulation Chi Square tests to see if an association exists between variables Always two-tailed University ofGalway.ie Chi Square It is typically used for the all-or-none behaviour of participants (e.g. smoking vs non-smoking) Samples of data which involve frequencies rather than scores, chi square test may be used Chi square test is formally described as comparing an observed frequency distribution to an expected frequency distribution University ofGalway.ie Chi Square For the Chi square test, the DV measures how many participants in each group will fall into certain categories (frequencies) This, therefore, cannot be determined beforehand, as in other experimental designs Consequently, you have to test quite a lot of people to make sure that you have sufficient number turn out to be allocated to each category A minimum of 20 (usually) University