Introduction to Statistics - Psychological Measurement - PDF
Document Details
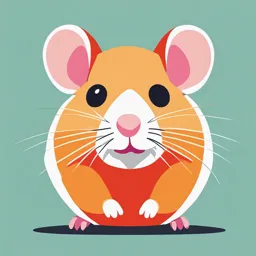
Uploaded by PrizeKineticArt7826
King's College London
2024
Moritz Herle
Tags
Summary
This presentation provides a basic introduction to statistics and psychological measurement, focusing on key concepts such as latent variables, factor analysis, and reliability and validity.
Full Transcript
IoPPN 14th November 2024 Moritz Herle Introduction to Statistics SGDP Centre Psychological Measurement This lecture Latent variables Factor analysis Cronbach’s alpha and other forms of reliability Test reliability and validity ...
IoPPN 14th November 2024 Moritz Herle Introduction to Statistics SGDP Centre Psychological Measurement This lecture Latent variables Factor analysis Cronbach’s alpha and other forms of reliability Test reliability and validity Think – Pair – Share Why do we need measurements and what are potential challenges in the fields of psychology and psychopathology? DD/Month/ Professor/Dr: Topic title: YYYY Questionnaires are everywhere! DD/Month/ Professor/Dr: Topic title: YYYY Latent variables Psychometrics is a scientific discipline concerned with the construction of assessment tools, measurement instruments, and formalized models that may serve to connect observable phenomena (e.g., responses to items in an IQ-test) to theoretical attributes (e.g., intelligence) Psychometrics and is generally using a Structural Equation Modelling (SEM) Framework Latent (similar to Twin Modelling) Variabl “Classical test theory”: An observed score on a test is the “true” score + e error The “true” score is a latent variable Some examples of latent variables: We can never Observ Intelligence measure any ed Happiness of these Extraversion variabl Depression directly: e Quality of life we have to Socioeconomic status make Liberalism/Conservatism inferences ε from observed Arithmet Specific variance + ic error Matrix Specific variance + Reasoni error ng Intelligence Specific variance + Vocabul error ary Digit Specific variance + Span error Factor analysis Fundamental point of factor analysis: maybe the variation that we see in a large number of observed variables really reflects the variation in a smaller number of latent variables Put another way: the reason that observed variables correlate together might be that they’re all associated with a latent variable In the previous slide, a proportion of the variance among the IQ tests was theorised to be explained by “intelligence” To what extent can we reduce the dimensions of our data to show these latent variables? Examples: The Big Five Inventory (BFI) Popular questionnaire to measure different aspects of personality 25 self-report items - Items cluster into 4 subscales (latent variables): - Openness to experience - Agreeableness - Conscientiousness - Neuroticism - Extraversion Exploratory vs. confirmatory Four factor-analysis questions we can ask of a multi-item dataset: 1. How many factors are there? (exploratory) 2. What are they? (exploratory) 3. How well does a model where we assume k factors fit to the data? (confirmatory) 4. How well does each individual item represent those factors? (both) We’re going to try this in the bfi dataset Steps of a factor analysis How many factors are there in the dataset? 1. Factor analysis extracts a set of new variables (factors) that summarise the overlap in variance among the individual items 2. Each factor has an eigenvalue: the amount of variance that it explains compared to one individual variable e.g. An eigenvalue of 3.5 means that the factor explains as much variance as 3.5 of the observed variables 3. The factors are lined up in order of their eigenvalues 4. We use some criterion (there are many) to decide how many factors to keep 5. Then we look at which observed variables are most strongly linked to each factor That is, their “factor loadings” (the correlation of each observed variable with the factor) The eigenvalue is the sum of the (squared) loadings on the factor Confirmatory factor analysis N E O A C N N N N N E E E E E O O O O O A A A A A C C C C C 1 2 3 4 5 1 2 3 4 5 1 2 3 4 5 1 2 3 4 5 1 2 3 4 5 ?? Arithmet Arrows are ic going in the opposite It’s easy to read too much direction Matrix into a factor analysis Reasoni The factors aren’t necessarily ng Intelligence Income “real” The interpretation isn’t Vocabul ary straightforward Job Latent vs. formative -> Status Socio- Digit Economic Span Status See Meehl (1993) Number of rooms For a better understanding of in home our measures, they need Number reliability and validity of cars owned Different kinds of reliability Now we have our subscales (latent factors) but are they any good? Is the test consistently measuring the same thing? Better reliability = more of the observed score consists of “true” variance rather than error Test-retest reliability (does the test measure the same thing over time?) Internal consistency (do the different parts of the test measure the same thing?) Split-half reliability (ditto) Interrater reliability (do different raters use the test in the same way?) Test-retest reliability Correlation of performance at time 1 with performance at time 2 Test-retest information for the WISC-IV -> Same tests repeated after average ~1 month Example of test-retest reliability Same test repeated after 79 years Source Cronbach’s alpha The most commonly-reported reliability measure See here for perspective from social/personality psychology Measures the internal consistency of a set of scale items To what extent do the items overlap in whatever they’re measuring? Can take values from 0 to 1 0 = no overlap at all; 1 = complete overlap Rule of thumb: minimum acceptable = Alpha for the other scales A: 0.70 C: 0.72 E: 0.76 N: 0.81 O: 0.61 Debate over Cronbach’s alpha Assumptions: Each item contributes ~equally to the total score (“tau equivalence”) Normally-distributed items Uncorrelated errors Unidimensionality These are often violated Can bias the alpha in unpredictable ways Some alternatives discussed here See also this defence of alpha Different kinds of validity Face validity: does the measure appear (on its face) as if it measures the construct of interest? Concurrent validity: does the measure correlate with other measures of the same construct? Predictive validity: does the test predict some relevant outcome in the future? Construct validity: (see next slide) There’s no simple test in R for any of these! Cronbach & Meehl (1955) Different ways of testing construct validity: 1. Group differences (e.g. a test of “attitudes towards going to church” should differ between churchgoers and non-churchgoers) 2. Internal structure (pattern of correlations/latent factors are theoretically expected – there should be convergent and discriminant validity) 3. Changes over time (a high test-retest correlation could be good, or bad, for validity) 4. Studies of process (if people keep misreading an item, there’s error added to their score and the validity is lower) The nomological net The interlocking network of measurable variables in which a construct occurs Built up over time by investigating the relation of that variable to many others Reliability and validity Source Summary What we’re normally trying to measure in psychology is a latent variable But what we can actually measure is an observed variable Factor analysis helps uncover patterns of correlation in a dataset, and points to latent variables Note: this was a very quick intro and factor analysis can get extremely complicated! There are many different ways of assessing reliability and validity in psychology For reliability: Cronbach’s alpha is most common, but it’s far from perfect For validity: the classic paper by Cronbach & Meehl is a must-read for anyone assessing any construct