H&D 5 .pdf
Document Details
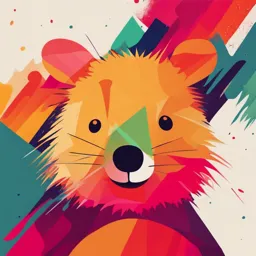
Uploaded by LogicalStarlitSky
Tags
Related
- Topnotch Medical Board Prep Preventive Medicine & Public Health Main Handout - April 2024 PDF
- Basic Epidemiologic Concepts and Principles PDF
- Epidemiologic Data Measurements PDF
- Chapter 251: Atrial Fibrillation (Harrison's Principles of Internal Medicine)
- Community Medicine - LECTURE 3 - Measures of Mortality.pdf
- Principles of infectious disease epidemiology 2024.pdf
Full Transcript
Sources of Variation Professor Dr. Zhian Salah Ramzi MBChB, MSc and PhD in Community Medicine Department of Clinical Sciences Objectives of lecture You should be able to: • Distinguish between ‘observed’ epidemiological quantities (incidence, prevalence, incidence rate ratio, etc.) and their ‘true...
Sources of Variation Professor Dr. Zhian Salah Ramzi MBChB, MSc and PhD in Community Medicine Department of Clinical Sciences Objectives of lecture You should be able to: • Distinguish between ‘observed’ epidemiological quantities (incidence, prevalence, incidence rate ratio, etc.) and their ‘true’ or ‘underlying’ values. • Discuss how ‘observed’ epidemiological quantities depart from their ‘true’ values because of random variation • Describe how ‘observed’ values help us towards a knowledge of the ‘true’ values by: 1-allowing us to test hypotheses about the ‘true’ values 2- allowing us to calculate a confidence interval that gives a range which includes the ‘true’ value with a specified probability. Parameters and estimates • Parameter numerical characteristic of a population • Statistics = a value calculated in a sample • Estimate a statistic that “guesstimates” a parameter • Example: sample mean “x-bar” is the estimator of population mean µ 0 Parameters and estimates are related but are not the same Parameters and statistics Parameters Statistics Source Population Sample Notation Greek (μ, σ) Roman (x, s) Random variable? No Yes Systematic and Random Variation New risk if • Systematic Variation: This is a consistent difference between the recorded value and the true value in a series of observations which results in some individuals being systematically misclassified. • Random Variation : Chance differences in the true and recorded values may result in an apparent association between an exposure and an outcome, and such variations may arise from unbiased measurement errors the true value Systematic and Random Variation • Some variation in diseases can be attributed to particular factors: for example, morbidity and mortality generally vary with age. The general increase in the incidence of cancer of the prostate with age is an example of systematic variation in disease incidence - As most diseases involve complex processes, some fluctuations cannot be explained. - In effect, things are affected by chance or random variation. Systematic and Random Variation - If a wheel comes off a lorry and causes a major road accident on the Malik Mahmood circular street , should we conclude that the MM circular street has suddenly got much more dangerous and advise people to choose a different route? - Of course not – we know that driving anywhere carries a small risk, that wheels occasionally fall off lorries and sometimes a pile -up results, and that there is no reason to conclude that the MM street is more or less dangerous than usual after the accident Systematic and Random Variation - Why do some heavy smokers live to 90 while some non -smokers die of lung cancer at 30? - Why do we occasionally see half a dozen or more road deaths in one day in certain district, and then none for weeks? - Why, if you check the prevalence of tuberculosis (TB) on several different occasions, does it fluctuate up and down? Systematic and Random Variation - If your grandfather lives to 90 despite smoking heavily, would you conclude that smoking is harmless, or would you just conclude that he was lucky? - Most people would sensibly put it down to simple luck. The amount of tobacco smoked is strongly associated with lung cancer: - increased use of tobacco is associated with an average increase in lung cancer incidence. - About half of all regular smokers will die prematurely with a smoking-related condition. • Random variation influences anything we observe, including epidemiological data on groups of people. For example, the incidence of TB varies with time and some of the variation is likely to be random variation Systematic and Random Variation • Random variation influences anything we observe, including epidemiological data on groups of people. • For example, the incidence of TB varies with time and some of the variation is likely to be random variation • Because of random variation, it is useful to distinguish between the ‘true’ or ‘underlying’ value of what we are measuring, and what we can actually go out and measure or observe (which we know may have been influenced by random variation). • In an epidemiological context we may get four cases of TB in one year and seven the next, but we do not necessarily conclude that the ‘true’ risk of TB has increased Systematic and Random Variation • Of course, what we really need to know about is the ‘true ’ risk – • There is no sense in reacting to random fluctuations in TB frequency, but we would want to know if the ‘true’ or ‘underlying’ risk is changing. • Perhaps there is some new risk factor, perhaps a pathogen has become more virulent, or perhaps some new public health measure is working. • Ideally, we would try to explain the systematic part of the variation, i.e. the variation that could be attributed to the new risk factor. • The table below gives a few more examples of things we might want to find out about, and of the sorts of observations we could make in each case Observations we can make, and the ‘true’ or ‘underlying’ things we are interested in What we might actually measure or observe What we really want to know 1- The proportion of people with diabetes in a random sample of 200 in Ranya The true prevalence of diabetes in Ranya 2- The difference in survival between 200 cases of breast cancer treated with Tamoxifen, and 200 untreated cases 3- The number of new cases of Tuberculosis notified in hawler in 2000 The effect on breast cancer survival if all breast cancer patients were treated with Tamoxifen The underlying risk or incidence of Tuberculosis in Hawler in 2000 Definitions Association • A statistical relationship between two or more variables Risk • Probability conditional or unconditional of the occurrence of some event in time • Probability of an individual developing a disease or change in health status over a fixed time interval, conditional on the individual not dying during the same time period Risk factors A risk factor refers to an aspect of personal habits or an environmental exposure, that is associated with an increased probability of occurrence of a disease. risk factors can usually be modified, intervening to alter them in a favourable direction can reduce the probability of occurrence of disease. The impact of these interventions can be determined by repeated measures using the same methods and definitions The chances of something happening can be expressed as a risk or as an odds: RISK = the chances of something happening the chances of all things happening ODDS= the chances of something happening the chances of it not happening Thus a risk is a proportion, But an odds is a ratio. An odds is a special type of ratio, one in which the numerator and denominator sum to one. Measuring the occurrence of disease or other health states is the first step of the epidemiological process. The next step is comparing occurrence in two or more groups of people whose exposures have differed. An individual can be either exposed or unexposed to a factor under study. An unexposed group is often used as a reference group. Measures of Effect The 2x2 table is formed when the “exposure” (i.e. cause) variable and “outcome” (effect) variable take a dichotomy of either absent or present. On the axis of exposure, we have two categories : -exposure present (E +) -exposure absent (E-). On axis of outcome, we have, -outcome present (O+) -outcome absent (O - ) . 2 2 table 2 umn Yess labile expof To answer whether smoking is a risk factor for IHD, ischemicheart Disease assume that a study was started with 5000 healthy adults and IHD was excluded at the start by means of relevant diagnostic work - up. These 5000 subjects were interrogated about smoking habits (exposure); it was found that there were 1500 smokers (E +) and 3500 non smokers (E-). These two groups (E+ and E - ) were then followed up for 10 years to see whether IHD (the outcome) developed (O +) or not (O - ). It was found that out of 1500 smokers (E +), 150 developed IHD (E + O +) while 1350 did not (E + O - ). On the other hand, out of the 3500 non smokers (E - ), 175 developed IHD (E - O +) while 3325 did not(E - O - ). General epidemiology - Family and Community Medicine department Two by two table for this situation is : Risk 80 REEF General epidemiology - Family and Community Medicine department a+b = Total number of subjects who have the exposure, irrespective of whether they develop the outcome or not, i.e. E+ (e.g. all smokers, whether they developed IHD or not, i.e.,.(1500 ) c+d = Total number of subjects who do not have the exposure, irrespective of whether they develop the outcome or not, i.e. E (e.g. all non smokers, whether they developed IHD or not, i.e. 3500). a+c = Total number of subjects who developed the outcome, irrespective of whether they had the exposure or not, i.e. O+ (e.g. all those who did develop IHD, whether they used to smoke or not, i.e. 325). b+d = Total number of subjects who did not develop the outcome, irrespective of whether they had the exposure or not, i.e. O - (e.g. all those who did not develop IHD, whether they used to smoke or not, i.e. 4675). a+b+c+d = The grand total of all subjects studied (or simply “n”) (e.g. 5000 in the present example). We can compare occurrences to calculate the risk that a health effect will result from an exposure. We can make both absolute and relative comparisons; the measures describe the strength of an association between exposure and outcome. Relative comparisons Relative risk and odd ratio Attributable risk Absolute comparisons IF Risk difference Attributable fraction (exposed) Population attributable risk To determine the rates of disease by person, place and time Absolute risk (incidence, prevalence) To identify the risk factors for the disease Relative risk (or odds ratio) To develop approaches for disease prevention Attributable risk/fraction To identify the risk factors for the disease Relative risk (RR), odds ratio (OR) RR = ratio of incidence of disease in exposed individuals to the incidence of disease in non-exposed individuals (from a cohort/prospective study) If RR > 1, there is a positive association If RR < 1, there is a negative association OR = ratio of the odds that cases were exposed to the odds that the controls were exposed (from a case control/retrospective study) – If OR > 1, there is a positive association If OR < 1, there is a negative association Relative risk The relative risk (also called the risk ratio) is the ratio of the risk of occurrence of a disease among exposed people to that among the unexposed. The risk ratio is a better indicator of the strength of an association than the risk difference, because it is expressed relative to a baseline level of occurrence. The risk ratio is used in assessing the likelihood that an association represents a causal relationship. Relative risk For example, the risk ratio of lung cancer in long-term heavy smokers compared with non-smokers is approximately 20. This is very high and indicates that this relationship is not likely to be a chance finding. The Relative Risk (RR) gives an idea of the number of times that the Incidence is likely to be more among those who have the exposure compared to those who do not have the exposure. This equation is the Relative Risk (RR) or the “Risk Ratio”. Thus, RR = Incidence of outcome among those having the Exposure (IE) Incidence of outcome among those not having the Exposure (IO or INE) In prospective studies. the product of the study can be summarized as a 2 x 2 contingency table illustrated by the following generic form: Risk Factor Present Absent Total Cases a c a+c Primary Outcome Controls Total b a+b d c+d b+d n=a+b+c+d e.g: In The above 2 x 2 table tells us that the incidence of IHD (over a 10 year period) was 10% among smokers (150 out of 1500) while it was 5% among non smokers (175 out of 3500). When asked if smoking is a risk factor for IHD, would be “yes, it is two times more among smokers”. This conclusion of “two times” more calculated simply dividing 10% by 5%. Incidence of the outcome (IHD) among those with the exposure (smokers)”, (i.e. 10%) is divided by the “incidence of the outcome (IHD) among those without the exposure (non - smokers) (i.e. 5%). What happens if the RR is equal to one or less than one ? : The incidence (i.e. Risk) of developing IHD among both, smokers and the non smokers is the same. So if RR is less than one( e.g 0.5), thus Smoking reduces the risk of getting IHD by half. If RR is less than 1, it indicates declining risk, i.e. a protective effect. smoking protects against IHD by 50%. (i.e. reduces the risk of IHD by half). further the RR is from one (towards zero), more is the strength of this protective association. Primary Outcome Risk Factor HIV Positive HIV Negative Total 30 or older a = 39 b = 816 855 under 30 c = 18 d = 623 641 Total 57 1439 n = 1496 ODDS RATIO Used by epidemiologists in studies looking for factors which do harm, it is a way of comparing patients who already have a certain condition (cases) with patients who do not (controls) – a “case–control study”. One boy is born for every two births, so the odds of giving birth to a boy are 1:1 (or 50:50) = 1⁄1 = 1 If one in every 100 patients suffers a side-effect from a treatment, the odds are 1:99 = 1⁄99 = 0.0101 The odds ratio is used to estimate the population relative risk when data is obtained in a retrospective study. In a retrospective study, the data can also be displayed in the form of a 2 x 2 contingency table: Risk Factor Present Absent Total Cases a c a+c Primary Outcome Controls Total b a+b d c+d b+d n=a+b+c+d The odds ratio in a case control study is calculated by the For example, we can take 50 persons diagnosed with IHD and 50 without IHD, obtain the history of smoking during past 10 years from them and set the data into the following 2x2 table The above setting is the classical “case control study” . The most important difference is that in this example we are not following up, in a FORWARD manner, the 2 groups of subjects (smokers and non - smokers developing or not developing IHD); but since the outcome (IHD) had already occurred, we are picking up “samples” of cases and healthy persons (50 each) and going BACKWARD from outcome to exposure. In our present example, the odds ratio And since the OR is a valid estimator of RR, we would conclude that the risk of IHD due to smoking is 1.94 times as compared to non - smoking. Primary Outcome Risk Factor HIV Positive HIV Negative Total 30 or older a = 39 b = 816 855 under 30 c = 18 d = 623 641 Total 57 1439 n = 1496 True value ?? • We consider several population characteristics we might want to know about: • The frequency of new cases (the incidence), • The proportion of people affected at a point in time (the prevalence) • The extra risk associated with an exposure (the incidence rate ratio or relative risk; also the SMR) • The reduction in risk associated with a new treatment (again the incidence rate ratio or relative risk). Probabilis True value ?? Er •We always want to find out the true value of these epidemiological measures but in each case we are limited by the measurements we can make or the events we can observe and count. Random variation?? • We hope to get approximately the right answer, but we know that our result might have been distorted by random variation. • We might observe a prevalence of diabetes of 30 per 1,000 in our sample, but we cannot say for sure that the true prevalence throughout Kalar takes this value. • We might observe that the new drug reduces the risk of death by half in our study, but we cannot be quite sure of its effect on the risk of death in the whole population of people with the disease. Hypothesis testing • What can we say, then? Informally we might say “the true prevalence is probably fairly close to 30 per 1,000” or “the new drug roughly halves the risk of death” but these are very vague statements. • We need to be able to make a more precise statement about what we are trying to measure, based on observed data. • In the ‘Hypothesis Testing’ section, we will consider how to use observed data to test hypotheses about what we have measured. • In the ‘Estimation’ section, we will consider how to use observed data to provide a range within which the true value of the summary measure is likely to lie. Hypothesis testing • Hypothesis testing is an assumption about a population parameter • This assumption may or may not be true •Hypothesis testing refers to the formal procedures used by statisticians to accept or reject statistical hypotheses Example: Imagine that we observe an incidence rate ratio (IRR) for childhood leukemia of 4 in a town with a nuclear power station compared with another town with no power station. This suggests that childhood leukemia incidence may be quadrupled in the town with the power station. Hence we suspect the power station to be the cause of the increase in IRR. However, we know that random variation could explain the observed IRR. How can we be sure that the ‘true’ IRR is raised at all? How do we know that it is not just a chance finding? Maybe the power station is innocent after all: what information do we have? f f f poe i IRR ( Incidence Rate Ratio) Suppose that the IRR of 4 was observed in one of two ways: Example 1: in Town A, 2 in 5,000 children developed leukemia in a particular year, whereas in Town B, 1 in 10,000 children did. Example 2: in Town C, 40 in 100,000 children developed leukemia in a particular year, whereas in Town D, 5 in 50,000 children did. Interpretation The argument has this structure: Hypothesis: no real excess risk of leukemia near the power station, i.e. consider ‘true’ IRR for leukemia = 1. Observation: observed IRR for leukemia = 4. Probability of observing IRR of 4 by chance if ‘true’ IRR = 1 Example 1: about 1 in 4, i.e. quite high. Example 2: less than 1 in 10,000, i.e. very low. Interpretation Conclusion: Example 1: insufficient evidence against the hypothesis of no real excess risk, i.e. chance could explain the observed difference. Example 2: strong evidence against the hypothesis of no real excess risk, i.e. chance is unlikely to explain the observed difference and so some factor other than chance is likely to be the reason Hypothesis testing This process is known as hypothesis testing. The more improbable the observation, under the assumption that the hypothesis is true, the stronger the evidence against that hypothesis. This probability is known as the p-value.(is the probability of obtaining test results at least as extreme as the results actually observed during the test, assuming that the null hypothesis is correct). The smaller the p-value, the stronger the evidence against the hypothesis. A common convention is to regard p-values greater than 0.05 (5%) as providing ‘little or no evidence’ against the hypothesis. When a p-value is less than 0.05, the data are said to show statistically significant evidence against the hypothesis; equivalently we say that we reject the hypothesis (reject null hypothesis). True value in epidemiological measure With this approach, we can start to make simple concrete statements about the ‘true’ value of epidemiological measures based on observed data in the following 5 examples: Example 1: “the incidence of leukemia in Town A is not statistically significantly higher than in Town B (p > 0.25)”, i.e. chance is likely to be responsible for the observed difference. Example 2: “the incidence of leukemia in Town C is statistically significantly higher than in Town D (p < 0.001)”, i.e. some factor other than chance is likely to be responsible for the observed difference. True value in epidemiological measure Example 3: “ if the incidence of tuberculosis in Basra is statistically significantly higher than elsewhere in the Iraq (p= 0.01)”, it means some factor other than chance is likely to be responsible for the observed difference. Example 4: “the mortality rate from pneumoconiosis is statistically significantly higher in Barnsley than in Nottingham (p=0.05)”, i.e. some factor other than chance is likely to be responsible for the observed difference. Example 5: “patients on neutron therapy did not live statistically significantly longer than those on conventional radiotherapy (p=0.4)”, i.e. chance is likely to be responsible for the observed difference. Null hypothesis In each case we have tested a hypothesis about the ‘true ’ value. The hypothesis that we usually test is the null hypothesis , i.e. the hypothesis that the two groups do not differ. The hypothesis in the power station example (IRR=1) was a null hypothesis. In Examples 2, 3 & 4 above, the observed data is deemed to have provided sufficient evidence against the null hypothesis. In Examples 1 & 5, the observed data was insufficient to conclude against the null hypothesis. Null hypothesis A hypothesis test can lead to one of two conclusions: a) there is some evidence against the hypothesis (perhaps strong if p < 0.02), but not that the hypothesis is definitely false there is little or no evidence from the data considered against the hypothesis, but not that the hypothesis is true. b) if there are few data available, then the result of the hypothesis test will reflect this lack of information, e.g. there were only 3 cases observed in Example 1. However, if there were a lot of data, then we might conclude that there is little point in conducting further studies Null hypothesis • if Example 5 was based on 240 deaths out of 600 patients (40%) on neutron therapy and 255 deaths out of 600 patients (44%) on radiotherapy, it would suggest that we are unable to reject the null hypothesis despite having plenty of data with which to do so, and we might conclude that there is little point in pursuing the more expensive neutron therapy. • Hypothesis tests do not quantify the evidence in favor of the null hypothesis. Estimations If our data provide strong evidence against the hypothesis that Basra residents and other Iraq residents are at equal risk of tuberculosis (p=0.01) we may report that Basra residents are at a higher risk of TB than other Iraq residents but we cannot say anything about how much higher that risk is likely to be. If the data do not provide sufficient evidence that a new treatment is beneficial, we wish to know whether this is because there are too few data or whether the new treatment is unlikely to be of any value (see ‘In conclusion (b)’ paragraph above). It would be very useful to define a range within which the excess risk, or possible benefit, are likely to lie. An extension of the hypothesis testing approach allows us to do this. • Imagine that a study observes an incidence rate ratio (IRR) of 1.3 for tuberculosis in residents compared with other Iraq residents. Consider the following sequence of statements: A series of hypothesis tests about the ‘true’ excess risk of TB in Basra Hypothesis IRR (a) IRR = 0.8 (20% reduced risk) (b) IRR = 0.9 (10% reduced risk) (exactly the same risk, (c) IRR = 1.0 i.e. the ‘null hypothesis’) (d) IRR = 1.1 (10% increased risk) (e) IRR = 1.2 (20% increased risk) (f) IRR = 1.3 (30% increased risk) (g) IRR = 1.4 (40% increased risk) (h) IRR = 1.5 (50% increased risk) (i) IRR = 1.6 (60% increased risk) Probability of observing IRR = 1.3 Reject assuming ‘hypothesis IRR’ is true (p-value) hypothesis? 0.0001 Rejected 0.002 Rejected 0.01 Rejected 0.1 0.2 0.5 0.2 0.1 0.01 Not Rejected Not Rejected Not Rejected Not Rejected Not Rejected Rejected The null hypothesis (c) is rejected (p=0.01). However, here we have not sought to test just one hypothesis about the ‘true’ excess risk, but a whole range Hypothesized values for the ‘true ’ IRR between 1.1 and 1.5 are ‘consistent with’ the data, and hypothesized values outside this range are ‘not consistent with’ the data. We can say that the ‘true’ IRR probably lies somewhere between 1.1 and 1.5, i.e. Basra residents are very likely to be at between 10% and 50% increased risk. This is much more useful than the null hypothesis test, which simply states that Basra and other Iraq residents probably do not have exactly the same risk Error factor • In practice, we use statistical theory which shows that we can be 95% sure that the ‘true’ value of any measure used in this module lies somewhere between [Observed value ÷ e.f.] and [Observed value × e.f.] ..............(1) where e.f. = error factor calculated from the data. This range is known as a 95% confidence interval – a widely used statistical measure. The method of calculating confidence intervals differs according to the measurement. Equation (1) above applies to many common epidemiological measures. The following examples show how to work out 95% confidence intervals for the incidence rate, prevalence, incidence rate ratio (IRR) and standardised mortality ratio (SMR). a) 95% Confidence Interval for incidence rate Example : • If we observe 300 heart attacks in a population of 50,000 over an 18 month period, the incidence rate would be: 300 /(50,000 × 1.5) = 0.004 heart attacks per person per year or ‘4 per 1,000 person/years’. • This is the observed incidence rate, and we are interested in the ‘true’ value The error factor for an incidence rate is given by: 1 • error factor = Exp { 2X -------------} -------------- (2) d where d is the number of events observed and exp means exponential (which can appear on your calculator as ex or Inverse ln) 95% CI for incidence rate N.B. – d here is 300 (the number of events) and not 4 (the rate of events). 1 error factor = Exp { 2X -------------} = 1.122 300 The error factor is 1.122, 4 ÷ 1.122 = 3.6 , 4 X 1.122 = 4.5 Hence the 95% confidence interval is 3.6 to 4.5 per 1,000 person-years. In plain English, we are 95% sure that the ‘true’ or ‘underlying’ incidence rate is somewhere between 3.6 and 4.5 cases per 1,000 person-years. I hope you will agree that this is more useful than saying “we think the incidence rate is probably somewhere around 4 per 1,000 person/years”. b) 95% Confidence Interval for Prevalence Conveniently, the error factor for prevalence is identical to Equation (2). again be sure to remember that d is the number of cases and not the prevalence. • If we observe 80 cases of breast cancer in 1,500 elderly women, the prevalence is 80 / 1,500 = 0.053 = 53 per 1,000. The error factor is 1.25 and the 95% confidence interval for the ‘true’ prevalence is from (0.053 ÷ 1.25) to (0.053 × 1.25), From 0.042 to 0.066. We can be 95% sure that the ‘true’ or ‘underlying’ prevalence is somewhere between 42 and 66 per 1,000 elderly women C. 95% Confidence Interval for an Incidence Rate Ratio (IRR) • For an incidence rate ratio (IRR), the error factor needs to take account of the number of events in both populations (d1 and d2) and is given by: • For example, we observe 8 strokes in 80 men over 10 years and 5 strokes in 200 women over 5 years. What is the ‘true’ incidence rate ratio (IRR) between men and women? The observed IRR is (8 ÷ 800) / (5 ÷ 1,000) = 2.0. 1 1 error factor = Exp { 2X ---------- + ----------} d1 ------------------------------ (3) d2 The error factor (calculated using d1 = 8 and d2 = 5) is 3.13. The 95% confidence interval extends from 0.64 to 6.25 and we are 95% sure that the ‘true’ or ‘underlying’ IRR lies somewhere within this range D. 95% Confidence Interval for an SMR(Standardized Mortality Ratio) • You will recall that the SMR is given by O/E. Despite the complexity of calculating the SMR itself, calculating a 95% confidence interval is child’s play. The error factor for an SMR is simply: 1 error factor = Exp { 2X ------------- } --------------- (4) O Where O (i.e. the number of deaths observed in the local population) is the value used to calculate the SMR itself The calculation of the 95% confidence interval is as in Equation (1) and again we can be 95% sure that the ‘true’ or ‘underlying’ SMR lies somewhere within this range 4. Relationship between Hypothesis Testing and Estimation On the whole, estimation is considered a better approach than hypothesis testing because it is far more informative. However, testing the null hypothesis is still often done when comparing two groups, since the first question to consider when we observe an apparent difference between groups is “can we plausibly attribute this entire result to chance?” If we can, there may be little point in going any further. • There is a simple ‘Rule of Thumb’ that links estimation with the null hypothesis test. 4. Relationship between Hypothesis Testing and Estimation You will recall that the 95% confidence interval is simply the range of ‘true’ values that are ‘consistent with’ the observed data. If the null hypothesis value (1.0 for an IRR, 100 for an SMR) lies within the 95% confidence interval, then the null hypothesis value is ‘consistent with’ the observed data, the p-value is greater than 0.05 So we cannot reject the null hypothesis. If the null hypothesis value lies outside the 95% confidence interval, the null hypothesis value is ‘not consistent with’ the observed data, the p-value is less than 0.05 and we reject the null hypothesis For example, in the example of the IRR for strokes, 1.0 lies within the 95% confidence interval (0.64 to 6.25) so a ‘true’ IRR of 1.0 is ‘consistent with’ the observed data. The p-value is greater than 0.05, and we have insufficient evidence to reject the null hypothesis that men and women are truly at equal risk of stroke. Note that this does not mean that the null hypothesis value is the ‘true’ value for the IRR for stroke between men and women, it is one of many potential ‘true’ values between 0.64 and 6.25 that are ‘consistent with’ the observed data Thank you