Chapter 4: Descriptive Statistical Measures PDF
Document Details
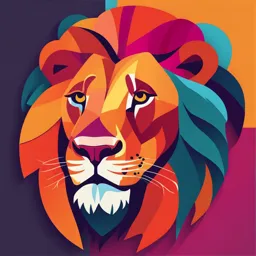
Uploaded by UnrivaledUnderstanding
Universiti Teknologi MARA, Johor
Nur Liyana binti Mohamed Yousop
Tags
Summary
This document provides an overview of descriptive statistical measures, including central tendencies (mean, median, mode), measures of dispersion (range, variance, standard deviation), z-scores, and the concept of outliers. It also touches upon normal distributions and skewness, along with practical examples, formulas, and Excel functions.
Full Transcript
CHAPTER 4 Descriptive Statistical Measures Prepared by: Nur Liyana binti Mohamed Yousop Population vs Sample Population Sample Definition The whole group that is being studied Subgroup of population Notation Parameter Statistics Mean μ x̅ Variance σ2 s2 Std. Dev σ s Sample Size N...
CHAPTER 4 Descriptive Statistical Measures Prepared by: Nur Liyana binti Mohamed Yousop Population vs Sample Population Sample Definition The whole group that is being studied Subgroup of population Notation Parameter Statistics Mean μ x̅ Variance σ2 s2 Std. Dev σ s Sample Size N n Population Proportion P p̂ Elements X x Descriptive vs Inferential Statistics Descriptive Statistics Inferential Statistics Definition • • Helps you describe, organize, and summarize the data. It presents information in a manageable form. • Descriptive statistics do not, however, allow us to make conclusions beyond • the data we have analysed or reach conclusions regarding any hypotheses we might have made. Inferential statistics, unlike descriptive statistics, is the attempt to apply the conclusions that have been obtained from one experimental study to more general populations. This means inferential statistics tries to answer questions about populations and samples that have not been tested in the given experiment. Measures of Central Tendency Definition In statistics, a central tendency (or measure of central tendency) provide a summary for the whole dataset with a single value that is derived from dataset. It may also be called a center or location of the distribution. Types of Measures of Central Tendency The middle value in a data set Mean Average of dataset Mode Data values that occur more often Median Measures of Dispersion Definition In statistics, dispersion (also called variability, scatter, or spread). Measure of dispersion describe the variation or spread of the observation around the mean in a dataset. Common examples of measures of statistical dispersion are the variance, standard deviation, and interquartile range. Range Difference between largest and smallest values in dataset. Rarely use in statistics Range = Largest value – Smallest value FORMULA: =MAX(data range) – MIN(data range) Variance The variance is the “average” of the squared deviations from the mean. Population In Excel: =VAR.P(data range) Sample In Excel: =VAR.S(data range) **Note the difference in denominators! https://www.mathsisfun.com/data/standard-deviation.html Example 1: Computing the Variance Purchase Orders Cost per order data FORMULA: Standard Deviation The standard deviation is the square root of the variance. ◦ Note that the dimension of the variance is the square of the dimension of the observations, whereas the dimension of the standard deviation is the same as the data. This makes the standard deviation more practical to use in applications. Population In Excel: =STDEV.P(data range) Sample In Excel: =STDEV.S(data range) Example 2: Computing the Standard Deviation Purchase Orders Cost per order data Using the results of Example 1, take the square root of the variance: Alternatively, use the STDEV.S function for the data range. Standard Deviation as a Measure of Risk Excel file: Closing Stock Prices Intel (INTC): Mean = $18.81 Standard deviation = $0.50 General Electric (GE): Mean = $16.19 Standard deviation = $0.35 INTC is a higher risk investment than GE. Coefficient of Variation The coefficient of variation (relative standard deviation) is a statistical measure of the dispersion of data points around the mean. The metric is commonly used to compare the data dispersion between distinct series of data. FORMULA: CV = Standard deviation / Mean measure risk per unit of return Normal Distribution and z-Score Normal Distribution @ Gaussian Distribution ➢ The normal distribution (bell shape distribution) is a probability function that describes how the values of a variable are distributed. ➢ Different normal distribution curves may have different sets of parameters of mean and standard deviation or both. ➢ All normal distribution can curves can be determined by standardized distribution, which is known as the standard normal probability distribution (μ=0 and σ=1) Normal Distribution @ Gaussian Distribution Standardized Values A standardized value, commonly called a z-score, provides a relative measure of the distance an observation is from the mean, which is independent of the units of measurement. z-score tells you the score lies on the normal distribution and it also tells you how many standard deviation is above or below mean. For example: • z-score of 1 is 1 standard deviation above mean • z-score of -1 is 1 standard deviation below mean If the z-score is ± 3, it tells you that the value is much higher or lower (outlier). Standardized Values The z-score for the ith observation in a data set is calculated as follows: (formula for sample) ◦ Excel function: =STANDARDIZE(x, mean, standard_dev). Properties of z-Scores The numerator represents the distance that xi is from the sample mean; a negative value indicates that xi lies to the left of the mean, and a positive value indicates that it lies to the right of the mean. By dividing by the standard deviation, s, we scale the distance from the mean to express it in units of standard deviations. Thus, a z-score of 1.0, means that the observation is one standard deviation to the right of the mean; a z-score of 1.5, means that the observation is 1.5 standard deviations to the left of the mean. Example 3: Computing z-Scores Purchase Orders Cost per order data =(B2 - $B$97)/$B$98, or =STANDARDIZE(B2,$B$97,$B$98). z-Scores Tables Outliers Identifying Outliers Some typical rules of thumb: There is no standard definition of what constitutes an outlier. ** An observation point/s that is different from the other points • z-scores greater than +3 or less than -3 • Extreme outliers are more than 3*IQR to the left of Q1 or right of Q3 • Mild outliers are between 1.5*IQR and 3*IQR to the left of Q1 or right of Q3 Example 6: Investigating Outliers Home Market Value data None of the z-scores exceed 3. However, while individual variables might not exhibit outliers, combinations of them might. ◦ The last observation has a high market value ($120,700) but a relatively small house size (1,581 square feet) and may be an outlier. Measures of Shape Definition Skewness Kurtosis Measures of shape describe the distribution (or pattern) of the data within a dataset. Skewness Skewness describes the lack of symmetry of data. ◦ Distributions that tail off to the right are called positively skewed; those that tail off to the left are said to be negatively skewed. Positively skewed Symmetrical Coefficient of Skewness Coefficient of Skewness (CS): Excel function: =SKEW(data range) CS is negative for left-skewed data. CS is positive for right-skewed data. |CS| > 1 suggests high degree of skewness. 0.5 ≤ |CS| ≤ 1 suggests moderate skewness. |CS| < 0.5 suggests relative symmetry. Example 4: Measuring Skewness Purchase Orders database Cost per order data: CS = 1.66 (high positive skewness) A/P terms data: CS = 0.60 (moderate positive skewness) Kurtosis Kurtosis refers to the peakedness (i.e., high, narrow) or flatness (i.e., short, flat-topped) of a histogram. The coefficient of kurtosis (CK) measures the degree of kurtosis of a population. CK < 3 indicates the data is somewhat flat with a wide degree of dispersion. CK > 3 indicates the data is somewhat peaked with less dispersion. Excel function: =KURT(data range) Shape and Measures of Location Comparing measures of location can sometimes reveal information about the shape of the distribution of observations. For example, if the distribution were perfectly symmetrical and unimodal, the mean, median, and mode would all be the same. If it were negatively skewed, we would generally find that mean < median < mode Positive skewness would suggest that mode < median < mean Excel Descriptive Statistics Tool This tool provides a summary of numerical statistical measures for sample data. Data > Data Analysis > Descriptive Statistics Enter Input Range Labels (optional) Check Summary Statistics box The data must be in a single row or column. If the data are in multiple columns, the tool treats each row or column as a separate data set Example 5: Using the Descriptive Statistics Tool Purchase Orders database Note: Results of the Analysis Toolpak do not change when changes are made to the data. Practice: Closing Stock Prices Use the Descriptive Statistics tool to summarize the mean, median, variance and standard deviation of the closing price of stock. Practice: Sale Transactions dataset Use Pivot Table to find the number of sale transactions by product and region, total amount of revenue by region, and total revenue by region and product in the Sales Transactions database. Using PivotTable, find the average and standard deviation of sales by source (Web or e-mail). Do you think this information could be useful in advertising? END OF CHAPTER 4