Descriptive Statistics PDF
Document Details
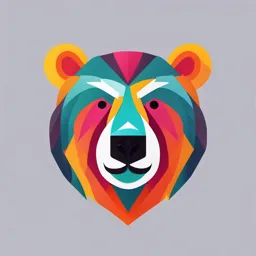
Uploaded by GratifyingHeliotrope8176
Tags
Summary
This document provides a basic introduction to descriptive statistical measures, focusing on measures of central tendency like the mean, median, and mode. It also contains examples of calculating these measures.
Full Transcript
Descriptive Statistical 1. Measures of central tendency measures 2. Measures of variation (dispersion) Measures of Central Tendency (location) for ungrouped data Measures of central tendency describe where the data are located. The most com...
Descriptive Statistical 1. Measures of central tendency measures 2. Measures of variation (dispersion) Measures of Central Tendency (location) for ungrouped data Measures of central tendency describe where the data are located. The most common measures of central tendency are: Arithmetic mean, median, mode. 32 Arithmetic Mean Arithmetic mean: σ 𝑛 𝑥𝑖 𝑥1+ 𝑥2 + …+ 𝑥𝑛 Let 𝑥1 , 𝑥2 , …, 𝑥𝑛 be a sample of size 𝑛. The sample mean (average) is: 𝑋ത = 𝑖=1 𝑛 = 𝑛 Examples: 1. What is the mean of the following numbers: 9, 3, 7, 3, 8, 10, 2 ? 9+3+7+3+8+10+2 Answer: 𝑋ത = =6 7 2. If the marks of five students (out of 100) are: 96, 94, 72, 52, 56, find the average of their marks. 96+94+72+52+56 Answer: 𝑋ത = = 74 5 33 3. A student got the following grades on his tests: 87,95,76 and 88. He wants his average to be 85 or better overall. What is the minimum grade he must get on the next test in order to achieve that average? 87+95+76+88+𝑥 Solution: 𝑋ത = ≥ 85 5 Properties of arithmetic mean 1. Uniqueness: for a given set of data there is one and only one mean. 2. Simplicity: it is so easy to compute the mean of any sample. 3. The value of each data item has an influence on the mean, thus the mean is affected by extreme values, this makes the mean, in some cases, not a good representative of the tendency of the values of the majority of the data. 34 Median Let 𝑥1 , 𝑥2 , …, 𝑥𝑛 be a sample of size 𝑛, and 𝑥(1) , 𝑥(2) , …, 𝑥(𝑛) be the sorted sample. The sample median is: 𝑥 𝑛+1 𝑖𝑓 𝑛 𝑖𝑠 𝑜𝑑𝑑 2 Median = ൞𝑥 𝑛 + 𝑥 𝑛+1 2 2 𝑖𝑓 𝑛 𝑖𝑠 𝑒𝑣𝑒𝑛 2 Example: Find the median of each of the following data sets: a. 20, 17, 56, 16, 29, 38, 7 b. 20, 17, 56, 32 Solution: a. 7 , 16 , 17 , 20 , 29 , 38 , 56 ⟹ 𝑚𝑒𝑑𝑖𝑎𝑛 = 20 20+32 b. 17 , 20 , 32 , 56 ⟹ 𝑚𝑒𝑑𝑖𝑎𝑛 = = 26 2 35 Example: Find the median for data in the following frequency table: Solution: 13 Total frequencies is 13 ⟹ = 6.5 ≈ 7. 2 So, the median is 𝑥7 = 15 Properties 1. Uniqueness. of Median 2. Simplicity. 3. Unlike the mean, it is not drastically affected by extreme values. 36 Mode The most frequent observation. Remark 1. There may be more than one mode. 2. Mode may not exist. Example: Find the mode of the following data sets: a. 8, 2, 4, 6, 8, 2, 1, 3, 6, 7, 8. b. 1, 2, 3, 4, 5, 6. Solution: a. 8 b. Mode does not exist. 37 Dispersion statistics summarize the scatter or spread of Measures of Variation (dispersion) data. Most of these functions describe deviation from a particular location. The most common measure of dispersion is range, variance, standard deviation, interquartile range and interpercentile range. Range Range = 𝑚𝑎𝑥𝑖𝑚𝑢𝑚 𝑜𝑏𝑠𝑒𝑟𝑣𝑎𝑡𝑖𝑜𝑛 − 𝑚𝑖𝑛𝑖𝑚𝑢𝑚 𝑜𝑏𝑠𝑒𝑟𝑣𝑎𝑡𝑖𝑜𝑛. 38 Example: Find the range for the following list of values: 13,18,13,14,16,14,21,13 Solution: Range = 𝑚𝑎𝑥𝑖𝑚𝑢𝑚 𝑜𝑏𝑠𝑒𝑟𝑣𝑎𝑡𝑖𝑜𝑛 − 𝑚𝑖𝑛𝑖𝑚𝑢𝑚 𝑜𝑏𝑠𝑒𝑟𝑣𝑎𝑡𝑖𝑜𝑛 = 21 − 13 = 8 Example: If Jordan's hottest temperature was 39.2° in 2018, and the range in temperature is 40.7°, what was the coldest temperature? Solution: Range = 𝑚𝑎𝑥𝑖𝑚𝑢𝑚 𝑜𝑏𝑠𝑒𝑟𝑣𝑎𝑡𝑖𝑜𝑛 − 𝑚𝑖𝑛𝑖𝑚𝑢𝑚 𝑜𝑏𝑠𝑒𝑟𝑣𝑎𝑡𝑖𝑜𝑛 40.7° = 39.2° − 𝑚𝑖𝑛 𝑚𝑖𝑛 = −1.5° 39 Variance Let 𝑥1 , 𝑥2 , …, 𝑥𝑛 be a sample of size 𝑛, the sample variance is: σ𝑛 ത 2 𝑖=1(𝑥−𝑋) 𝑆2 = (𝑛−1) 2 𝑛 σ𝑛𝑖=1 𝑥 2 − (σ𝑛𝑖=1 𝑥𝑖 ) = 𝑛(𝑛 − 1) Standard deviation Standard deviation is the square root of the variance. 𝑆 2 = ⃒𝑆⃒ 40 Example1: Suppose you are given the following data set: 3,2,1,4,5, find its variance and standard deviation. Solution: 2 𝑛 σ𝑛 2 𝑛 𝑖=1 𝑥 −(σ𝑖=1 𝑥𝑖 ) 5 55 − 15 2 𝑆2 = = = 2.5 𝑛(𝑛−1) 5 4 𝑆 = 2.5 = 1.58 41 Example2: The following data set represents daily wages for 7 workers: 4, 5, 6, 7, 8, 9, 10. What is the standard deviation of their wages? Solution: 2 2 𝑛 σ𝑛𝑖=1 𝑥 2 − (σ𝑛𝑖=1 𝑥𝑖 ) 7 371 − 49 2 𝑆 = = = 4.7 𝑛(𝑛 − 1) 7 6 𝑆 = 4.7 = 2.17 42 Percentiles, Deciles and Quartiles If we want to segment data into percentages we commonly are talking about quartiles, deciles and percentiles. Quartiles divide the data into four parts, with one-fourth of the data values in each part. The first quartile (denoted by 𝑄1 ) is the 25𝑡ℎ percentile. The second quartile (denoted by 𝑄2 ) is the 50𝑡ℎ percentile (the median). The third quartile (denoted by 𝑄3 ) is the 75𝑡ℎ percentile. Deciles divide the data into 10 equal parts. Percentiles divide the data into 100 parts. 43 Interquartile Range Interquartile Range equals the difference between 75th and 25th percentiles. 𝐼𝑄𝑅 = 𝑄3 − 𝑄1 How can you evaluate the interquartile range? 1. Order the data from least to greatest 𝑛 2. Find the observations in the first quartile 𝑄1 where its position is 4 3𝑛 3. Find the observations in the third quartile 𝑄3 where its position is 4 4. Apply 𝐼𝑄𝑅 = 𝑄3 − 𝑄1 44 Example: Find the interquartile of the following sets of data: 1) 2, 7, 5, 11, 5, 8, 10. Solution: 7 3 7 𝑛 = 7 → 𝑇ℎ𝑒 𝑜𝑟𝑑𝑒𝑟 𝑜𝑓 𝑄1 𝑖𝑠 = 1.75 ≈ 2 and the order of 𝑄3 𝑖𝑠 = 5.25 ≈ 6. 4 4 Ordered data: 2, 5, 5, 7, 8, 10, 11. So, 𝑄1 𝑖𝑠 5 ,𝑄3 𝑖𝑠 10 𝑎𝑛𝑑 𝐼𝑄𝑅 = 10 − 5 = 5 Solution: 25 3 25 𝑛 = 25 → 𝑇ℎ𝑒 𝑜𝑟𝑑𝑒𝑟 𝑜𝑓 𝑄1 𝑖𝑠 = 6.25 ≈ 7𝑡ℎ and the order of 𝑄3 𝑖𝑠 = 18.75. 4 4 So, 𝑄1 𝑖𝑠 2 𝑎𝑛𝑑 𝑄3 𝑖𝑠 4 𝑎𝑛𝑑 𝐼𝑄𝑅 = 4 − 2 = 2 45 Interpercentile Range The interpercentile range is the difference between two percentiles. 𝑟 The 𝑟 𝑡ℎ percentile is define d by 𝑃𝑟 = 100 × 𝑛 The 𝑚𝑡ℎ percentile to 𝑛𝑡ℎ percentile is 𝑃𝑛 − 𝑃𝑚 How to find interpercentile range? 1. Order data in an ascending order. 2. Find 𝑃𝑛 and 𝑃𝑚 positions. 3. Apply 𝐼𝑃𝑅 = 𝑃𝑛 − 𝑃𝑚 Example: Find 35% to 65% interpercentile of the following data set: 2, 2, 5, 7, 7, 8, 8, 9, 10, 12 Solution: 35 × 10 = 3.5 ≈ 4 then 𝑃35 = 7 100 65 × 10 = 6.5 ≈ 7 then 𝑃65 = 8 100 𝑃65 − 𝑃35 = 8 − 7 = 1 46 Coefficient of Variation The Coefficient of Variation denoted by C.V., is a unit free measure that is used to compare the amount of dispersion between two different sets of data with (possibly) different means and different units. 𝑆 The coefficient of variation is given by C.V= 𝑋ത × 100 Example: The following table summarizes the data collected about the weights of two samples of human males. Which of the samples is more dispersed? Solution: 10 C.V for sample 1 is 145 × 100 = 6.9 10 C.V for sample 2 is 80 × 100 = 12.5 Hence, sample 2 is more spread. 47 48 Counting techniques that are used to determine the number of Chapter Two outcomes of an experiment include: Counting Techniques 1. Product rule. 2. Permutations 3. Combinations Product rule: If an experiment consists of 𝑘 steps, of which the first can be made in 𝑛1 ways, the second can be made in 𝑛2 ways,..., the 𝑘th can be made in 𝑛𝑘 ways, then the whole choice can be made in 𝑛1 × 𝑛2 ×...× 𝑛𝑘 ways. 62 Example: How many different routes can we take in travelling from town A to C if there are 2 roads from A to B and 3 roads from B to C, as shown in the diagram. Solution: Number of routes from town A to B is 2 Number of routes from town B to C is 3 Number of routes from town A to C is 2 × 3 = 6 Example: A club has 5 members. In how many ways can the club choose a president and a vice- president if everyone is eligible? Solution: Number of ways of selecting a president is 5. Number of ways of selecting a vice-president is 4. Number of ways of selecting a president and a vice-president is 5×4 = 20 ways 63 Example: How many license-plates with 4 letters chosen from {𝑎,𝑏,𝑐,𝑑,𝑒} can be composed in each case: 1) with repetition. 2) without repetition. Solution: 1) 5 × 5 × 5 × 5 = 625 license-plates. 2) 5 × 4 × 3 × 2 = 120 license-plates. Example: A box contains 3 red, 4 white and 5 black balls. Four balls are drawn from the box. In how many ways can we perform this experiment if: a. Balls are drawn with replacement. b. Balls are drawn without replacement. c. The first is red, the second is white, the third is red and the fourth is white (with replacement). Solution: a. Number of choices = 12 × 12 × 12 × 12 = (12)4 = 20736 b. Number of choices = 12 × 11 × 10 × 9 = 11880 c. Number of choices= 3 × 4 × 3 × 4 = 144 64 𝑛! (read as 𝑛 factorial) is a product of 𝑛 with each positive counting number less than 𝑛. 0! = 1 1! = 1, 2! = 2, 3! = 6, 4! = 24, 5! = 120 In general,𝑛! = 𝑛 × 𝑛 − 1 × 𝑛 − 2 × ⋯ × 1 65 A permutation is an arrangement of objects without repetition where order is important. Remark: 1. A permutation of 𝑛 objects, arranged into one group of size 𝑛, without repetition, and order being important is 𝑛! 2. A permutation of 𝑛 objects, arranged in groups of size 𝑘, without repetition, and order being important is 𝑛! 𝑃(𝑛, 𝑘) = (𝑛 − 𝑘)! 66 Example: In how many ways can we ask 5 students 5 different questions ? Solution: 5! = 120 Example: In how many different ways could 4 items be arranged in 4 places ? Solution: 4! = 24 Example: Find all two-letter permutations of the letters {𝐴,𝐵,𝐶} Solution: 3! 𝑃(3,2) = = 6 (3−2)! 67 If a word has 𝑁 letters, 𝑘 of which are unique, and let {𝑛1 ,𝑛2 ,...,𝑛𝑘 } be the Remark frequencies of letters, then the total number of distinguishable permutations is given by: 𝑁! 𝑛1 !𝑛2 !…𝑛𝑘 ! Example: Find all permutations of the letters of 'Bob'. Solution: 𝑁! 3! = (2!)(1!) = 3 𝑛1 !𝑛2 !…𝑛𝑘 ! Letter “B” is repeated twice and letter “O” is repeated once. 68 Example: Find all permutations of the letters of 'Statistics'. Solution: 𝑁! 10! = (3!)(3!)(2!)(1!)(1!) = 50400 𝑛1 !𝑛2 !…𝑛𝑘 ! Letter “S” is repeated three times Letter “T” is repeated three times Letter “I” is repeated twice Letter “A” is repeated once Letter “C” is repeated once. 69 Combinations An arrangement of objects without repetition where order is not important. The only difference in the definition of a permutation and a combination is whether order is important. A combination of 𝑛 objects, arranged in groups of size 𝑘, without repetition is: 𝑛! 𝑃(𝑛,𝑘) 𝐶(𝑛,𝑘) = 𝑛 𝑘 = (𝑛−𝑘)!𝑘! = 𝑘! Example: If we pick a team of 3 people from a group of 10. How many choices do we have? Solution: 10 10! 10! 10×9×8×7! 10×9×8 𝐶(10,3) = 3 = = 7!3! = = = 120 10−3 !(3)! 7!3! 6 70 Example: In choosing 3 desserts from a menu of 7. How many choices do we have? Solution: 7 7! 7×6×5×4! 7×6×5 𝐶(7,3) = 3 = 4 !(3)! = 4!3! = 6 = 35 1. 𝑛 1 =𝑛 Note 2. 𝑛 =1 𝑛 3. 𝑛 𝑘 = 𝑛 𝑛−𝑘 4. If 𝑛 𝑘1 = 𝑛 𝑘2 , then either 𝑘1 =𝑘2 , or 𝑘1 +𝑘2 = 𝑛. 71 Combinations are used in the binomial expansion theorem from Pascal's Triangle algebra to give the coefficients of the expansion (𝑎 + 𝑏)𝑛. They also form a pattern known as Pascal's Triangle Each element in the table is the sum of the two elements directly above it. Each element is also a combination. The 𝑛 value is the number of the row (start counting at zero) and the 𝑘 value is the element in the row (start counting at zero). Pascal's Triangle illustrates the symmetric nature of a combination. 72 Example: A coach wants to compose a team of 4 members. If there are 4 tennis players and 5 athletes, in how many ways can he compose such a team consisting of each of the following? 1. Two tennis players and two athletes. 2. At least two tennis player. 3. At most one athlete. Solution: 1. 4 2 5 2 = 6 × 10 = 60 2. 4 2 5 2 + 4 3 5 1 + 4 4 5 0 = 6 × 10 + 4 × 5 + 1 × 1 = 81 3. 4 3 5 1 + 4 4 5 0 = 4 × 5 + 1 × 1 = 21 73 Example: Out of 8 questions, 4 are scientific and 4 are literature, in how many ways can a student choose 4 of them in each case: 1. Three scientific questions and one literature question. 2. At least 3 literature questions. 3. At most 2 literatures. Solution: 4 4 1. 3 1 = 16 2. 1 4 4 3 + 4 0 4 4 = 16 + 1 = 17 3. 4 2 4 2 + 4 3 4 1 + 4 4 4 0 = 6 × 6 + 4 × 4 + 1 × 1 = 53 74 Additional Exercises 1. What order could 16 pool balls be in? 2. How many ways can first and second place be awarded to 10 people? 3. How many permutations of 3 different digits are there, chosen from the ten digits 0 to 9 inclusive? 4. How many different committees of 5 people can be chosen from 10 people? 5. An encyclopedia has eight volumes. In how many ways can the eight volumes be replaced on the shelf? 6. A restaurant offers 5 choices of appetizer, 10 choices of main meal and 4 choices of dessert. A customer can choose to eat just one course, or two different courses, or all three courses. Assuming all choices are available, how many different possible meals does the restaurant offer? 75 Chapter Three Elements of Probability 76 Definitions 1.An experiment a process that yields an observation (outcome). 2. When the outcomes of an experiment vary randomly, then the experiment is called a random experiment. 3. A sample space (denoted by Ω ) is a collection of all possible outcomes of the random experiment. Examples: 1. In rolling a fair die once and observing the outcomes, the sample space is Ω = 1,2,3,4,5,6 2. In tossing an unbiased coin twice and observing the outcomes, the sample space is Ω = {(𝐻, 𝐻), (𝐻, 𝑇), (𝑇, 𝐻), (𝑇, 𝑇)} 77 An event is a subset of a sample space, and commonly denoted by 𝐴. Events Note that: ∅ and Ω are two events. Example: An experiment of tossing three balanced coins and the observed face of each coin is of interest. Write the event 𝐴 in each of the following cases: 1. Obtaining at least two heads. 2. Obtaining at most one head. Solution: Ω = {(𝐻,𝐻,𝐻),(𝐻,𝐻,𝑇),(𝐻,𝑇,𝐻),(𝐻,𝑇,𝑇),(𝑇,𝐻,𝐻),(𝑇,𝐻,𝑇),(𝑇,𝑇,𝐻),(𝑇,𝑇,𝑇)}. 1. 𝐴 = {(𝐻,𝐻,𝐻),((𝐻,𝐻,𝑇),(𝐻,𝑇,𝐻),(𝑇,𝐻,𝐻)}. 2. 𝐵 = {(𝐻,𝑇,𝑇),(𝑇,𝐻,𝑇),(𝑇,𝑇,𝐻),(𝑇,𝑇,𝑇)} 78 Theoretical notations of events Suppose that Ω is the sample space of some random experiment. Let 𝐴1 and 𝐴2 be two events in Ω. 1. 𝐴1ҧ (complement of 𝑨𝟏 ) consists of all out comes in Ω which are not in 𝐴1. 2. An event 𝐴1 is said to be a subset of 𝐴2 (denoted by Let 𝐴1 𝐴2 ) if every outcome in 𝐴1 is in 𝐴2. 3. The intersection of 𝐴1 and 𝐴2 (denoted by 𝐴1 ∩ 𝐴2 ) consists of the outcomes that belong to both 𝐴1 and 𝐴2. 4. The union of 𝐴1 and 𝐴2 (denoted by 𝐴1 ∪ 𝐴2 ) consists of the outcomes that belong to 𝐴1 or 𝐴2. 5. Two events Let 𝐴1 and 𝐴2 are said to be disjoint if 𝐴1 ∩ 𝐴2 = ∅. 79 Venn diagrams 80 Classical Probability If the sample space of an experiment consists of 𝑁 finite sample points which are equally likely, then for any event 𝐴, we define the probability of 𝐴 as: 𝑛(𝐴) 𝑛𝑢𝑚𝑏𝑒𝑟 𝑜𝑓 𝑜𝑢𝑡𝑐𝑜𝑚𝑒𝑠 𝑖𝑛 𝐴 𝑃 𝐴 = = 𝑛(Ω) 𝑛𝑢𝑚𝑏𝑒𝑟 𝑜𝑓 𝑜𝑢𝑡𝑐𝑜𝑚𝑒𝑠 𝑖𝑛 Ω Example: Suppose we roll a fair once. What is the probability of getting each of the following events? 𝐴1 : 𝑂𝑑𝑑 𝑛𝑢𝑚𝑏𝑒𝑟 , 𝐴2 : 𝑁𝑢𝑚𝑏𝑒𝑟 𝑙𝑒𝑠𝑠 𝑡ℎ𝑎𝑛 3 , 𝐴3 : 𝑁𝑢𝑚𝑏𝑒𝑟 𝑔𝑟𝑒𝑎𝑡𝑒𝑟 𝑡ℎ𝑎𝑛 8 Solution: Ω = 1,2,3,4,5,6 𝑛 𝐴1 3 1 1. 𝐴1 = 1,3,5 ⟹ 𝑃 𝐴1 = 𝑛 Ω =6=2 𝑛 𝐴2 2 1 2. 𝐴2 = 1,2 ⟹ 𝑃 𝐴2 = =6=3 𝑛 Ω 𝑛 𝐴3 0 3. 𝐴3 = = ∅ ⟹ 𝑃 𝐴3 = =6=0 𝑛 Ω 81 Suppose that Ω is the sample space of some random experiment. Let 𝐴 and 𝐵 be two events in Ω, then: 1. 0𝑃 𝐴 1 2. 𝑃(Ω) = 1 and 𝑃(∅) = 0 3. 𝑃(𝐴𝐵) = 𝑃(𝐴) + 𝑃(𝐵) − 𝑃(𝐴𝐵). Joint events 4. If 𝐴𝐵=∅, then 𝑃(𝐴𝐵) = 𝑃(𝐴) + 𝑃(𝐵) because 𝑃 𝐴𝐵 = 0 5. 𝑃(𝐴𝐵) ҧ = 𝑃(𝐵) − 𝑃(𝐴𝐵) and 𝑃(𝐴𝐵) ത = 𝑃(𝐴) − 𝑃(𝐴𝐵) 6. 𝑃 𝐴ҧ = 1 − 𝑃 𝐴 7. 𝑃(𝐴 ത = 𝑃(𝐴 ∪ 𝐵) = 1 − 𝑃(𝐴 ∪ 𝐵) and 𝑃(𝐴ҧ ∪ 𝐵) ҧ 𝐵) ത = 𝑃(𝐴 ∩ 𝐵) = 1 − 𝑃(𝐴 ∩ 𝐵) Disjoint events 82 Example: Given an experiment such that 𝑃(𝐴) = 0.5 , 𝑃(𝐵) = 0.25 and 𝑃 𝐴𝐵 = 0.2. Evaluate each of the following: 1.𝑃 𝐴𝐵ത 2. 𝑃 𝐴ҧ ∪ 𝐵ത 3.𝑃 𝐴𝐵 4. 𝑃 𝐴ҧ 5. Are A and B disjoint? Justify your answer. Solution: 1. 𝑃 𝐴𝐵ത = 𝑃 𝐴 − 𝑃 𝐴𝐵 = 0.5 − 0.2 = 0.3 2. 𝑃 𝐴ҧ ∪ 𝐵ത = 𝑃 𝐴 ∩ 𝐵 = 1 − 𝑃 𝐴 ∩ 𝐵 = 1 − 0.2 = 0.8 3. 𝑃 𝐴𝐵 = 𝑃 𝐴 + 𝑃 𝐵 − 𝑃 𝐴𝐵 = 0.5 + 0.25 − 0.2 = 0.55 4. 𝑃 𝐴ҧ = 1 − 𝑃 𝐴 = 1 − 0.5 = 0.5 5. No. 𝐴 and 𝐵 are joint because 𝑃(𝐴𝐵) = 0.2 83 Number of Number of outcomes of a random experiment is outcomes (𝒏𝒖𝒎𝒃𝒆𝒓 𝒐𝒇 𝒐𝒖𝒕𝒄𝒐𝒎𝒆𝒔 𝒐𝒇 𝒂 𝒔𝒊𝒏𝒈𝒍𝒆 𝒕𝒓𝒊𝒂𝒍)𝒏𝒖𝒎𝒃𝒆𝒓 𝒐𝒇 𝒕𝒓𝒊𝒂𝒍𝒔 1. If we roll a fair die twice, we will get (6)2 = 36 outcomes. Examples 2. If we toss a balanced coin 5 times, we will get (2)5 = 32 outcomes 84 Example: An experiment of tossing a balanced coin four times, the event 𝐴 is getting at least one head, 𝐵 getting exactly three heads. Calculate 𝑃(𝐴) and 𝑃(𝐵). Solution: 𝑛 Ω = 16 𝐴ҧ = { 𝑇, 𝑇, 𝑇, 𝑇 } 1 𝑃(𝐴) = 16 15 hence, 𝑃 𝐴 = 16 𝐵 = {(𝐻, 𝐻, 𝐻, 𝑇), (𝐻, 𝐻, 𝑇, 𝐻), (𝐻, 𝑇, 𝐻, 𝐻), (𝑇, 𝐻, 𝐻, 𝐻)} 4 1 𝑃(𝐵) = = 16 4 85 Conditional Let B be an event such that 𝑃(𝐵) > 0. The conditional probability of an event 𝐴 Probability given 𝐵 is: 𝑃 𝐴⃒𝐵 = 𝑃 𝐴∩𝐵 𝑃 𝐵 The conditional probability of an event 𝐵 given 𝐴 provided that 𝑃 𝐴 > 0 is 𝑃(𝐴 ∩ 𝐵) 𝑃 𝐵⃒𝐴 = 𝑃(𝐴) Example: Let 𝐴 and 𝐵 be two events in Ω such that 𝑃(𝐴𝐵) = 0.4 and 𝑃 𝐴 ∩ 𝐵 = 0.2. Calculate 𝑃 𝐵ത. Solution: 𝑃 𝐵ത = 1 − 𝑃 𝐵 = 0.5 Example: Let 𝐴 and 𝐵 be two events in Ω such that 𝑃(𝐴𝐵) = 0.4 and 𝑃 𝐴 = 0.2 and 𝑃 𝐵 = 0.3. Calculate 𝑃(𝐵𝐴). Solution: 𝑃(𝐵𝐴) = 0.6 86 Example: An experiment of picking randomly a transistor from a box containing 100 transistors, some of which were produced by factory 1 and the rest by factory 2. Some are defective and some are good (non-defective). If 𝐴 the event of obtaining a defective one, 𝐵 is the event that transistor was produced by factory 1. Use the accompanying table to evaluate: 1.𝑃 𝐴 2. 𝑃(𝐴𝐵) 3. 𝑃(𝐵𝐴) 4. 𝑃(𝐴 ∩ 𝐵) ത Solution: 20 1. 𝑃 𝐴 = 100 = 0.2 15 𝑃 𝐴∩𝐵 2. 𝑃(𝐴𝐵) = = 100 60 = 0.25 𝑃 𝐵 100 15 𝑃 𝐴∩𝐵 3. 𝑃(𝐵𝐴) = 𝑃 𝐴 = 100 20 = 0.75 100 5 1 4. 𝑃 𝐴 ∩ 𝐵ത = 100 = 20 87 𝐴 and 𝐵 are said to be independent events if 𝑃(𝐴 ∩ 𝐵) = 𝑃 𝐴 𝑃 𝐵 Example: In rolling a fair die once, if 𝐴 = 1,2 and 𝐵 = {2,4,5}. Are 𝐴 and 𝐵 independent? Solution: Ω = 1,2,3,4,5,6 𝐴 ∩ 𝐵 = {2} 1 𝑃 𝐴 = 3 1 𝑃 𝐵 = 2 1 𝑃 𝐴∩𝐵 = =𝑃 𝐴 𝑃 𝐵 6 So, 𝐴 𝑎𝑛𝑑 𝐵 are independent. 88 Example: A box contains eight tickets, each labeled with a binary number. Two are labeled 111, two are labeled 100, two 010 and two 001. If we draw randomly one ticket, the events are: 𝐴: the first digit is one , 𝐵: the second digit is one , 𝐶: the third digit is one Evaluate each of the following: 1. 𝑃 𝐴 , 𝑃(𝐵) and 𝑃(𝐶). 2. 𝑃 𝐴 ∩ 𝐵 ∩ 𝐶 3. 𝑃(𝐶𝐵) 4. Are A, B and C independent? Solution: 4 1. 𝑃 𝐴 = 𝑃 𝐵 = 𝑃 𝐶 = 8 = 0.5 2 2. 𝑃 𝐴 ∩ 𝐵 ∩ 𝐶 = 8 = 0.25 2 𝑃 𝐶∩𝐵 3. 𝑃(𝐶𝐵) = = 8 4 = 0.5 𝑃 𝐵 8 1 4. No. They are dependent because 𝑃 𝐴.𝑃 𝐵.𝑃 𝐶 = 8 = 0.125 but 𝑃 𝐴 ∩ 𝐵 ∩ 𝐶 = 0.25 89 Example: If a fair die is rolled twice, show that the two events 𝐴 and 𝐵 are independent where 𝐴: 𝑇ℎ𝑒 𝑠𝑢𝑚 𝑜𝑓 𝑡𝑤𝑜 𝑎𝑝𝑝𝑎𝑟𝑒𝑛𝑡 𝑓𝑎𝑐𝑒𝑠 𝑖𝑠 8. 𝐵: 𝑇ℎ𝑒 𝑠𝑢𝑚 𝑜𝑓 𝑡𝑤𝑜 𝑎𝑝𝑝𝑎𝑟𝑒𝑛𝑡 𝑓𝑎𝑐𝑒𝑠 𝑖𝑠 3. Solution: Ω = { 1,1 , 1,2 , … , 6,6 } 𝑁: 𝑁𝑢𝑚𝑏𝑒𝑟 𝑜𝑓 𝑜𝑢𝑡𝑐𝑜𝑚𝑒𝑠 𝑖𝑛 Ω 𝑖𝑠 36 5 𝐴= 2,6 , 6,2 , 3,5 , 5,3 , 4,4 ⟹ 𝑃(𝐴) = 36 2 𝐵= 1,2 , 2,1 ⟹ 𝑃(𝐵) = 36 10 𝐴 ∩ 𝐵 = ∅ ⟹ 𝑃 𝐴 ∩ 𝐵 = 0 and 𝑃 𝐴 ∩ 𝐵 = 0 ≠ (36)2 = 𝑃 𝐴)𝑃(𝐵 Hence, 𝐴 𝑎𝑛𝑑 𝐵 𝑎𝑟𝑒 𝑑𝑒𝑝𝑒𝑛𝑑𝑒𝑛𝑡. 90 In a random experiment with a sample space Ω, if 𝐴 is an event an 𝐵1 ,𝐵2 , … ,𝐵𝑛 Low of Total are events such that : Probability i. 𝐵1 ∪ 𝐵2 ∪ ⋯ ∪ 𝐵𝑛 = Ω ii. 𝐵𝑖 ∩ 𝐵𝑗 = ∅ 𝑓𝑜𝑟 𝑎𝑙𝑙 𝑖 ≠ 𝑗 Then 𝑃 𝐴 = 𝑃 𝐴⃒𝐵1 × 𝑃 𝐵1 + 𝑃 𝐴⃒𝐵2 × 𝑃 𝐵2 + ⋯ + 𝑃(𝐴⃒𝐵𝑛 ) × 𝑃(𝐵𝑛 ) Example: In a class, 30% of students are IT, 50% are math’s and 20% are physics. Suppose that 60% of IT, 80% of math’s and 90% of physics students are females (𝐹). Find 𝑃 𝐹. Solution: 𝑃 𝐹 = 𝑃 𝐹⃒𝐼𝑇 × 𝑃 𝐼𝑇 + 𝑃 𝐹⃒𝑀𝑎𝑡ℎ𝑠 × 𝑃 𝑀𝑎𝑡ℎ𝑠 + 𝑃 𝐹⃒𝑝ℎ𝑦𝑠𝑖𝑐𝑠 × 𝑃 𝑝ℎ𝑦𝑠𝑖𝑐𝑠 = 0.60 × 0.30 + 0.80 × 0.50 + 0.90 × 0.20 = 0.76 91 Chapter Four 1. Univariate Distribution Discrete Definition: A random variable X is a function (mapping) defined Random over a sample space Ω that associates a real number with each (𝑋: Ω → ℝ and 𝑋(𝜔) = 𝑥 ) possible outcome 𝜔 in Ω Variables 92 Example: 1 𝑖𝑓 ℎ𝑒𝑎𝑑𝑠 In tossing an unbiased coin once, let 𝑋 =ቊ 0 𝑖𝑓 𝑡𝑎𝑖𝑙𝑠 i.e. 𝑋 is quantifying outcomes of a random experiment. Discrete Random Variables If a set of all possible values of a random variable 𝑋 is a countable set 𝑥1 , 𝑥2 , … , then 𝑋 is called a discrete random variable. The function 𝑓(𝑥) = 𝑃[𝑋 = 𝑥] assigns the probability to each possible value 𝑥 will be called the discrete probability density function (pdf). 93 Example: In tossing a balanced coin 3 times. Let 𝑋 to be number of heads, find the pdf of 𝑋 in each case: 1. 𝑓(𝑥) = 𝑃[𝑋 = 0] 2. 𝑓(𝑥) = 𝑃[𝑋 = 1] 3.𝑓(𝑥) = 𝑃[𝑋 = 2] 4. 𝑓(𝑥) = 𝑃[𝑋 = 3] Solution: Ω = { 𝐻,𝐻,𝐻 , 𝐻,𝐻,𝑇 , 𝐻,𝑇,𝐻 , 𝐻,𝑇,𝑇 , 𝑇,𝐻,𝐻 , 𝑇,𝐻,𝑇 , 𝑇,𝑇,𝐻 , 𝑇,𝑇,𝑇 } 1 1. 𝑓 𝑥 = 𝑃 𝑋 = 0 = 8 3 2. 𝑓 𝑥 = 𝑃 𝑋 = 1 = 8 3 3.𝑓 𝑥 = 𝑃 𝑋 = 2 = 8 1 4. 𝑓 𝑥 = 𝑃 𝑋 = 3 = 8 94 1. 0 ≤ 𝑃 𝑋 = 𝑥 ≤ 1 2. σ 𝑃 𝑋 = 𝑥 = 1 3. 𝐹 𝑥 = 𝑃 𝑋 ≤ 𝑥 = σ 𝑓(𝑥) (Cumulative distribution function) 4. 𝑃 𝑋 > 𝑥 = 1 − 𝑃 𝑋 ≤ 𝑥 1 𝑥 Example: A discrete random variable 𝑋 has a pdf 𝑓(𝑥). If 𝑓 𝑥 = ቐ𝑘 2 𝑥 = 1,2,3. Find the constant 𝑘. 0 𝑜𝑡ℎ𝑒𝑟𝑤𝑖𝑠𝑒 1 1 1 2 1 3 7 8 Solution: 𝑘 +𝑘 +𝑘 = 1 , thus 𝑘 =1 ⟹𝑘=7 2 2 2 8 Example: Suppose that the random variable 𝑋 represents the number of children in 100 families, find 𝑘. Solution: σ 𝑃 𝑋 = 𝑥 = 1. Hence, 0.44 + 0.34 + 0.11 + 0.06 + 0.02 + 0.01 + 0.01 + 𝑘 = 1 , 𝑠𝑜 𝑘=0.01 95 Example: Consider the following table, find each of the following: 1. 𝑃 𝑋 = 4 2. 𝑃 𝑋 ≤ 2 3. 𝐹 𝑥 4.𝑃 𝑋 > 2 5. 𝑃 2 ≤ 𝑋 < 4 6. 𝑃[𝑋 𝑖𝑠 𝑜𝑑𝑑⃒𝑋 > 2] Solution: 7 1. 𝑃 𝑋 = 4 = 𝑓 4 = 16 1 3 4 1 2. 𝑃 𝑋 ≤ 2 = 𝑓 1 + 𝑓 2 = 16 + 16 = 16 = 4 1 3 12 3 4. 𝑃 𝑋 > 2 = 1 − 𝑃 𝑋 ≤ 2 = 1 − + = 16 = 4 16 16 3 5 8 1 5.𝑃 2 ≤ 𝑋 < 4 = 𝑓 2 + 𝑓 3 = 16 + 16 = 16 = 2 5 𝑃[ 𝑋 𝑖𝑠 𝑜𝑑𝑑 ∩ 𝑋>2 ] 5 6.𝑃 𝑋 𝑖𝑠 𝑜𝑑𝑑⃒𝑋 > 2 = = 16 12 = 12 𝑃[𝑋>2] 16 96 If 𝑋 is a discrete random variable with pdf 𝑓 𝑥 , then the Expected value expected value of 𝑋 is defined by 𝐸 𝑋 = 𝜇 = 𝑥𝑓 𝑥 Example: A box contains four cards. Two are labeled with number 2, one is labeled with a 4, and the other with an 8. The experiment of choosing a card at random can be associated with a discrete random variable 𝑋 having distinct values on the four cards. Evaluate the expected vale of 𝑋. Solution: 𝑥 = 2, 4 and 8 with 1 1 𝑓(2) = 2 , 𝑓(4) = 𝑓(8) = 4 1 1 1 𝜇 = 𝐸 𝑋 = (2 × ) + (4 × ) + (8 × ) = 4 2 4 4 97 𝑥 Example: Let 𝑋 be a random variable with discrete pdf 𝑓(𝑥) = 8 if 𝑥 = 1,2,5 and 𝑧𝑒𝑟𝑜 otherwise. Calculate 1.𝑃[𝑋 = 2] 2. 𝑃[𝑋 > 2] 3.𝐸 𝑋 Solution: 2 1 1. 𝑃 𝑋 = 2 = 8 = 4 3 5 2. 𝑃 𝑋 > 2 = 1 − 𝑃 𝑋 ≤ 2 = 1 − =8 8 1+2×2+5×5 30 3. 𝐸(𝑋) = = = 3.75 8 8 98 Variance & Standard If 𝑋 is a discrete random variable with pdf 𝑓 𝑥 , then the variance of 𝑋 is defined by 𝑉𝑎𝑟 𝑋 = 𝜎 2 = 𝐸 𝑋 2 − 𝐸 2 𝑋 Deviation = σ 𝑥 2 𝑓 𝑥 − σ 𝑥𝑓 𝑥 2 Standard deviation= 𝜎 2 = ⃒𝜎⃒ 1 1 Example: Let 𝑋 be a random variable such that 𝑓(0) = 𝑓(2) = 4 , 𝑓(1) = 2 , find the mean and standard deviation of 𝑋. Solution: 1 1 1 𝐸(𝑋)= (0 × 4) + (2 × 4) + (1 × 2) = 1 2 2 1 1 1 1 1 1 𝑉𝑎𝑟 𝑋 = 𝜎 2 = 𝑥 2 𝑓 𝑥 − 𝑥𝑓 𝑥 = 02 × + 22 × + 12 × − (0 × ) + (2 × ) + (1 × ) 4 4 2 4 4 2 3 1 = − 1 = 2 2 1 Standard deviation= 𝜎 2 = 2 99 Example: If the random variable 𝑋 has the following CDF: 0 𝑥 70 = 𝑃 𝜎 > 20 = 𝑃 𝑍 > 0 = 0.5 𝑛 10 𝑛 10 ത 𝑋−𝜇 73−70 ത 𝑋−𝜇 73−70 b) 𝑍 = 𝜎 = 20 = 1.5 𝑠𝑜 𝑃 𝑋ത < 73 = 𝑃 𝜎 < 20 = 𝑃 𝑍 < 1.5 = 0.9332 𝑛 100 𝑛 10 141 If the sample size is small and we want to estimate the mean of a normally distributed population, then we use the t-distribution. Definition: If 𝑥1 ,𝑥2 , … ,𝑥𝑛 is a random sample drawn from a population which is normally distributed with mean 𝜇 and variance 𝜎 2 , ത 𝑋−𝜇 then 𝑆 ~𝑡𝑛−1 where (𝑛 − 1) is the degrees of freedom (d.f) ൗ 𝑛 1. The distribution curve for 𝑡 - distribution is a bell-shaped curve symmetric about the 𝑡 = 0 Properties of 2. For 𝑛 ≥ 30, the 𝑡 -distribution is very closed to the standard 𝑡 -distribution normal distribution. 3. The shape of 𝑡 -distribution depends on the sample size 𝑛. To compute probabilities, one can use the 𝑡 -distribution tables that give the cumulative probability. 142 Example: Find the probability of each of the following: 1. 𝑃 𝑡 ≥ 2.571 2. 𝑃 𝑡 ≤ 1.372 3. 𝑃 𝑡 ≤ −1.645 Solution: 1. 𝑃 𝑡 ≥ 2.571 = 0.025 , 𝑛 = 5 2. 𝑃 𝑡 ≤ 1.372 = 1 − 𝑃 𝑡 ≥ 1.372 = 1 − 0.1 = 0.9 ,𝑛 = 10 3. 𝑃 𝑡 ≤ −1.645 = 𝑃 𝑡 ≥ 1.645 = 0.05 ,𝑛 = ∞ Example: There are 250 cats at a cat show who weigh an average of 12 pounds, with a standard deviation of 8 pounds. If 4 cats are chosen at random, what is the probability they have an average weight of greater than 7 pounds. Solution: Number of cats that were chosen is 4 ത 𝑋−𝜇 7−12 𝑡= 𝑆 = 8 = −1 ⟹ 𝑃 𝑡 ≥ −1 = 𝑃 𝑡 ≤ 1 = 1 − 0.01 = 0.99 𝑛 4 143 Chi-Square If 𝑥1 ,𝑥2 , … ,𝑥𝑛 is a random sample drawn from a population with mean Distribution (𝑛−1)𝑆 2 𝜇 and variance 𝜎. If 𝑆 is the sample variance, then 𝜒 = 2 2 2 has 𝜎2 a sampling distribution called the chi-square distribution with (𝑛 − 1) degrees of freedom. 1. The curve of 𝜒 2 distribution is skewed to the right. 2. To compute probabilities, one can use the 𝜒 2 -distribution tables that give the cumulative probability. 3. The mean of a chi-square distribution equals 𝑛 and the variance equals 2𝑛. 144