Descriptive Statistics - Measures of Variability and Position PDF
Document Details
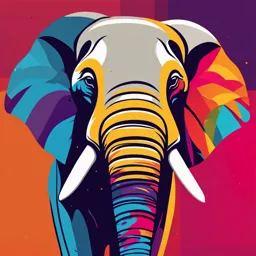
Uploaded by SincereKazoo3282
University of the Cordilleras
Tags
Summary
This document is a lesson on descriptive statistics, focusing on measures of variability and position. It covers key concepts such as standard deviation and variance with examples. It also includes formulas for calculations of statistical measures.
Full Transcript
MODULE 3 DESCRIPTIVE STATISTICS Lesson 3.2. MEASURES OF VARIABILITY AND POSITION MEASURES OF VARIABILITY Variability provides a quantitative measure of the degree to whic...
MODULE 3 DESCRIPTIVE STATISTICS Lesson 3.2. MEASURES OF VARIABILITY AND POSITION MEASURES OF VARIABILITY Variability provides a quantitative measure of the degree to which scores in a distribution are spread out or clustered together. The table below to familiarize symbols used to describe values Standard Total number of Variance Mean Deviation scores/observations Population µ N Sample s s2 n Example: The mean score for each quiz is 7. However, even though the two quizzes have the same mean score, you can see that the distributions are quite different. The students' scores on Quiz 1 are more clustered together, whereas the students' scores on Quiz 2 are more spread out. The students' scores on Quiz 1 are less variable than the students' scores Quiz 2, even though the mean score is the same on the two quizzes. Three frequently used measures of variability are the: A. Range o The distance from the largest score to the smallest score in a distribution. o The larger the range, the more spread out the data are; the smaller the range, the more clustered the data are. o The problem with using the range as a measure of variability is that it is completely determined by the two extreme values and ignores the other scores in the distribution. o Example: On Quiz 1, shown in Figure 1, the lowest value is 5, and the highest value is 9. Therefore, the Range on Quiz 1 is 4. Range = Highest value – Lowest Value B. Standard Deviation o Standard Deviation is an especially useful measure of variability especially when the distribution is normal ("bell shaped") or approximately normal because the standard deviation affects the shape of the "bell curve." o Standard deviation uses the mean of the distribution as a reference point and describes whether the scores are clustered closely around the mean or are widely scattered. 1. Raw Score Method Formula: 𝛴𝑋 2 − (𝛴𝑋)2 SD = √ ⃑⃑⃑⃑⃑⃑⃑⃑⃑⃑⃑⃑⃑ 𝑛 𝑛−1 Scores (X) Squared Score (X2) 8 64 7 49 10 100 15 225 14 196 ΣX = 54 ΣX2 = 634 𝛴𝑋 2 − (𝛴𝑋)2 SD = √ ⃑⃑⃑⃑⃑⃑⃑⃑⃑⃑⃑⃑⃑ 𝑛 𝑛−1 634 − (54)2 =√ ⃑⃑⃑⃑⃑⃑⃑⃑⃑⃑⃑⃑ 5 5−1 = √12.7 SD = 3.56 2. Deviation Score Method Formula: ∑(𝑿− 𝑿 )𝟐 SD = √ 𝒏−𝟏 Deviation Score Squared Deviation Scores (X) (x - x) Score (x - x)2 8 -2.8 7.84 7 -3.8 14.44 10 -0.8 0.64 15 4.2 17.64 14 3.2 10.24 x = 10.8 ∑x - x = 0 Σ (x - x)2 = 50.8 ∑(𝑿− 𝑿 )𝟐 SD = √ 𝒏−𝟏 50.8 =√ 5 −1 SD = 3.56 3. ORGANIZED DATA Formula: 𝑛 (𝑓𝑋 2 ) − (𝛴𝑓𝑋)2 SD = √ ⃑⃑⃑⃑⃑⃑⃑⃑⃑⃑⃑⃑⃑⃑⃑⃑⃑⃑⃑⃑⃑⃑⃑⃑⃑⃑⃑⃑⃑⃑⃑⃑⃑⃑⃑⃑⃑⃑⃑⃑ 𝑛 ( 𝑛 − 1) Example: Class Intervals f CM (X) fX fX2 CM * fX 3–5 10 4 40 160 6–8 12 7 84 588 9 – 11 8 10 80 800 12 – 14 6 13 78 1,014 15 – 17 4 16 64 1,024 Σf = 40 (n) ΣfX = 346 ΣfX2 = 3,586 40 (3,586) − (346)2 SD = √ ⃑⃑⃑⃑⃑⃑⃑⃑⃑⃑⃑⃑⃑⃑⃑⃑⃑⃑⃑⃑⃑⃑⃑⃑⃑⃑⃑⃑⃑⃑⃑⃑⃑⃑⃑⃑⃑⃑⃑⃑⃑⃑⃑⃑⃑⃑ 40 ( 40 − 1) (143,440) − (119,716) =√ ⃑⃑⃑⃑⃑⃑⃑⃑⃑⃑⃑⃑⃑⃑⃑⃑⃑⃑⃑⃑⃑⃑⃑⃑⃑⃑⃑⃑⃑⃑⃑⃑⃑⃑⃑⃑⃑⃑⃑⃑⃑⃑⃑⃑⃑ 40 (39) SD = 3.90 C. Variance o The variance of a set of scores is just the square of the standard deviation or the average squared distance from the mean. Standard Deviation How to get the variance Variance s = 3.90 (3.90)2 s2 = 15.21 Reporting Standard Deviation In reporting the results of a study, the researcher often provides descriptive information for both central tendency and variability. The dependent variables in psychology research are often numerical values obtained from measurements on interval or ratio scales. With numerical scores the most common descriptive statistics are the mean (central tendency) and the standard deviation (variability), which are usually reported together Example: Children who viewed the violent cartoon displayed more aggressive responses (M = 12.45, SD = 3.7) than those who viewed the control cartoon (M = 4.22, SD = 1.04). When reporting the descriptive measures for several groups, the findings may be summarized in a table. Refer to the table below for an example. Lesson 3.3. MEASURES OF POSITION Measures of position is used to describe the position of a data value in relation to the rest of the data. TYPES OF MESURES OF POSITION A. Quartiles o Values of the variable that divide the ranked data into quarters; each set of data has three quartiles. Formula: L – lower boundary of interval that contains the i th quartile iN − 𝑐𝑓𝑏 i – class size Qi = L + i ( 4 ) F - frequency of the interval that contains the ith quartile ̅̅̅̅̅̅̅̅̅ 𝑓𝑚 N – total no. of observations fm - the cumulative frequency ith quartile cfb – cumulative frequency of the class before the Qi class Example: Compute for the 1st, 2nd, and 3rd quartiles Class Intervals f cf % CP 3–5 10 10 25 25 6–8 12 22 30 55 9 – 11 8 30 20 75 12 – 14 6 36 15 90 15 – 17 4 40 10 100 Σf = 40 (N) Σp = 100 CI/Quartile Qn f (fm) cfb Class 1(40) Q1 ( ) 10 3-5 10 0 4 2 (40) Q2 ( ) 20 6-8 12 10 4 3 (40) Q3 ( ) 30 9 - 11 8 22 4 Q1 Q2 Q3 iN iN iN − 𝑐𝑓𝑏 − 𝑐𝑓𝑏 − 𝑐𝑓𝑏 Q1 = L + i ( 4 ) Q2 = L + i ( 4 ) Q3 = L + i ( 4 ) ̅̅̅̅̅̅̅̅̅ 𝑓𝑚 ̅̅̅̅̅̅̅̅̅ 𝑓𝑚 ̅̅̅̅̅̅̅̅̅ 𝑓𝑚 1(40) 2 (40) 3 (40) −0 − 10 − 22 = 2.5 + 3 ( 4 ) = 5.5 + 3 ( 4 ) = 8.5 + 3 ( 4 ) ̅̅̅̅̅̅̅̅̅ 10 ̅̅̅̅̅̅̅̅ 12 ̅̅̅̅̅̅ 8 10 − 0 20 − 10 30 − 22 = 2.5 + 3 ( ̅̅̅̅̅̅̅̅̅) = 5.5 + 3 ( ̅̅̅̅̅̅̅̅̅ ) = 8.5 + 3 ( ̅̅̅̅̅̅ ) 10 12 8 Q1 = 5.5 Q2 = 8 Q3 = 11.5 * 25% of the scores fall below 5.5 * 50% of scores fall below 8 * 75% of scores fall below 11.5 * 25% of the test takers obtained * 50% of the test takers * 75% of the scores fall below a score below 5.5 obtained a score below 8 11.5 B. Percentiles o A measure that divides the distribution into 100 equal parts. Further, it is the value on the measurement scale below which a specified percentage of the scores in the distribution fall. Formula: L – lower boundary of interval that contains the i th percentile i – class size kN − 𝑐𝑓𝑏 F - frequency of the interval that contains the ith percentile Pi = L + i (100 ) N – total no. of observations ̅̅̅̅̅̅̅̅̅ 𝑓𝑚 fm - the cumulative frequency leading up to the interval that contains the ith percentile cfb – cumulative frequency of the class before the Pi class Example: Compute for the 15th, 50th, and 95th percentiles Class f cf rf p CP Intervals 15 – 17 4 40 0.10 10% 100% 12 – 14 6 36 0.15 15% 90% 9 – 11 8 30 0.20 20% 75% 6–8 12 22 0.30 30% 55% 3-5 10 10 0.25 25% 25% ∑f = 40 ∑rf = 1.00 ∑p = 100% CI/Quartile Pn f (fm) cfb Class 15 (40) P15 ( ) 6 3-5 10 0 100 50 (40) P50 ( ) 20 6-8 12 10 100 95 (40) P95 ( ) 38 15 - 17 4 36 100 P15 P50 P95 kN kN kN − 𝑐𝑓𝑏 − 𝑐𝑓𝑏 − 𝑐𝑓𝑏 P15 = L + i (100 ) P50 = L + i (100 ) P95 = L + i (100 ) ̅̅̅̅̅̅̅̅̅ 𝑓𝑚 ̅̅̅̅̅̅̅̅̅ 𝑓𝑚 ̅̅̅̅̅̅̅̅̅ 𝑓𝑚 15 (40) 50 (40) 95 (40) −0 − 10 −36 = 2.5 + 3 ( 100 ) = 5.5 + 3 ( 100 ) = 14.5 + 3 ( 100 ) ̅̅̅̅̅̅̅̅̅̅̅̅̅ 10 ̅̅̅̅̅̅̅̅̅̅̅̅̅̅̅ 12 ̅̅̅̅̅̅̅̅̅̅̅̅̅̅̅ 4 6−0 20 − 10 38 − 36 = 2.5 + 3 ( ̅̅̅̅̅̅̅̅̅) = 5.5 + 3 ( ̅̅̅̅̅̅̅̅̅ ) = 14.5 + 3 ( ̅̅̅̅̅̅̅̅̅̅) 10 12 4 P15 = 4.3 P50 = 8 P95 = 16 * 15% of the scores fall below 4.3 * 50% of scores fall below 8 * 95% of scores fall below 16 * 15% of the examinees scored * 50% of the examinees * 95% of the examinees below 4.3 scored below 8 scored below 16